Tech Policy Summit: A Fox, a Rabbit, and a Cabbage
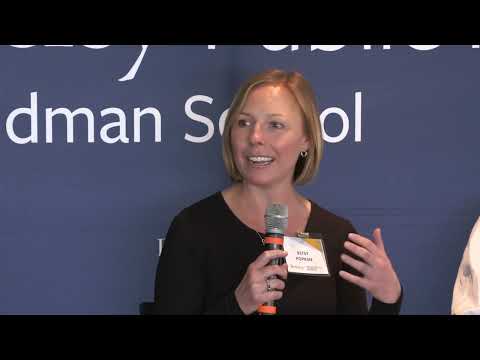
- So our next panel, a fox, a rabbit, and cabbage, spurring trustworthy and secure emerging technologies. We'll explore the balancing act required to foster technological innovation without compromising on ethics, security, and trustworthiness. Drawing inspiration from the classic problem solving puzzle, this panel explores the challenges and strategies in advancing new technologies while ensuring they're developed and deployed responsibly. So I'll let you up? - Yep. - And our esteemed moderator is Janet Napolitano, director of the Center for Security in Politics, professor of Public Policy at The Goldman School, and the former president of the University of California and former US secretary of Homeland Security, among many other very impressive titles. How many more? How many did I miss? - A few. - A few? Okay.
That's good. Well, welcome, Janet, so much, and I believe that there is a seat up there for you. - Okay. Okay, everybody ready? Are you psyched? All right, let's hear some energy in the room (audience laughs) 'cause we're about to dive into a really easy topic, which is how do you align innovation with other values and ethical values? So, as Brandy said, I'm Janet Napolitano. I'm currently a faculty member at The Goldman School and the director of the Center for Security in Politics. We have four outstanding panelists today: X Eyee who is the CEO of Malo Santo; Jessica Newman, who is director of the AI Security Initiative and the AI Policy Hub Center at the Center for Long-term Cybersecurity, CLTC, at Berkeley; Betsy Popken, who is the executive director of the Human Rights Center at Berkeley Law; and Niloufar Salehi, assistant professor of the School of Information at UC Berkeley.
As mentioned in this session, we will be exploring the balancing act required to foster technological innovation without compromising on ethics, security, and trustworthiness and even whether that can be done. We're going to draw on the classic problem solving puzzle of the rabbit, the fox, and the cabbage. So who remembers the puzzle the rabbit, the fox, and the cabbage? Okay, I'm gonna tell it to you. All right. - [Audience Member] (speaks indistinctly) this, too, is I'm obsessed with "Fargo."
There's an episode of it. - It's from "Fargo"? - Yeah, but- - Okay, all right. For those of us who aren't "Fargo" obsessed, here it goes. (audience laughs) A farmer must relocate a fox, a rabbit, and a cabbage across a river. They only have room to move one across the river at a time.
If the fox and rabbit are left alone together, the fox will eat the rabbit. That is not good for the farmer. Kind of sounds like Democrats and Republicans. (audience laughs) If the rabbit is left alone with the cabbage, the rabbit will eat it. There is a solution, but it takes some planning and some foresight.
First, the farmer takes the rabbit to the other side of the river and comes back alone. She then takes the fox with her to the other side. She leaves the fox and brings the rabbit back with her. She leaves the rabbit and takes the cabbage with her to the other side. She leaves the cabbage and comes back alone, and she then takes the rabbit with her to the other side of the river. And therefore, the rabbit, the fox, and the cabbage all end up safely on the other side.
Now, this puzzle isn't just meant to jog some memories of riddles, but it also gives us somewhat of a framework of the challenges faced in AI development. How do we appropriately spur innovation while ensuring compliance with serious constraints, technical, ethical, regulatory? So that is what our conversation is gonna focus on today, and I'm gonna begin by asking each of the panelists just the same question, which is how can the lessons of the fox, the rabbit, and the cabbage be applied to real world policy and development challenges in emerging technology, particularly in ensuring ethical considerations are not overlooked in the process of innovation? Jessica, we'll start with you, and we're just gonna work our way down the row. - All right, thank you so much, and thank you for moderating this and to the organizers.
I know an incredible amount of care and work has gone into this, and it's really wonderful opportunity to bring different parts of the UC Berkeley campus as well as the external community together. So yeah, this riddle, this puzzle, you know, I think part of the story that it's telling is you can't necessarily do everything at once. You know, trying to rush the process, get everything across the river at the same time, you know, will end up backfiring, and certainly, we see that in the AI governance space in terms of a lot of the leading AI governance and policy frameworks. They take a risk-based approach or a rights-based approach or some combination of those with the idea being that you have to prioritize. You can't do everything all at once, and some of the potential AI technologies, some of the potential use cases are gonna be much more impactful than others. We have to prioritize our actions.
I think one place where the analogy, you know, we shouldn't read too much into it, is that when we're thinking about AI governance, we also have to take a comprehensive view, and so, for example, you see in some companies, they'll kind of lean on the existing privacy frameworks that are in place, and like, that's the language they're used to. That's the kind of legal compliance they're used to, but we can't only think about privacy when it comes to AI governance. There's so many different issues that come into play, and so how do we, you know, both prioritize but also take that comprehensive view, I think, is one of the challenging parts of AI governance, but I like the analogy for thinking through that. - [Janet] Very good. - Two things I wanna point out. One is the need for an interdisciplinary approach, and I think you can see that by all of us on this panel right now.
We have policy makers, we have lawyers, human rights experts, computer scientists. We have kind of the full array of people here talking about one issue, and I think that is really necessary to moving the conversation forward. At the Human Rights Center, I'm leading a team conducting a human rights assessment of large language models, and we pair human rights experts, policy makers with computer scientists to do this. So we're conducting a traditional human rights impact assessment of the use of LLMs by educators, legal professionals, and journalists, and we're pairing that with a model evaluation.
So we're working with a team of computer scientists to basically tie the outputs of the models with human rights risks, and for that, we're focused in particular on the use of LLMs in investigative journalism. So that's one piece of it, and the second piece of it from the story that really jumps out to me is the need for foresight in the work you're doing. And so, so often in the human rights space, impact assessments are conducted after products have already been produced, oftentimes after they've already been released in the field. I know one company who produced a major LLM didn't conduct an analysis of the human rights impacts until months after it was released into the public, and so I think what needs to happen is to get this multidisciplinary team together, really at the inception of a new idea, to begin assessing the risks and creating processes for how to do that while the product is in development and then a process for how to respond to those risks that are identified.
So those are the two things that jump out to me from it, multidisciplinary approach and the need for foresight. - Thank you. So already, really great insights. I think for me, what stands out the most from this story in this problem is that the problem solving itself is very much a balancing act and an iterative process. It's not like a here you go, here's the solution, go do it. It's much more of a step by step, you do a little bit of it, then something happens, then you go back, you change your course, and then over time, you're hopefully getting closer and closer to the answer.
But I think the other part of it, and this probably applies more to the technologists in the room, I'm trained myself as a computer scientist, is that what comes to us really looking like tech problems are really, at the core, human problems and people problems. You know, my students have been working a lot on evaluations. I think LLM evaluation is a really, really impactful and important field that requires technological advancements, but at the same time, you know, my students have been doing a lot of work also on AI used in the criminal legal system, so, you know, facial recognition, gunshot detection, even statistical genotyping software. And the more we look into it and how it's actually used and contested in court, the less it looks like an evaluation like a tech problem and the more it looks like figuring out who the actors are, who is the fox, the rabbit, and the cabbage.
It's, you know, the technologists, the policy makers, but also the judges and jurors who are making the actual decisions on the ground and how those and their relationships between them and where the expertise and the resources lies is really what is having the most impact in the real world. So I think, yeah, for me, it's sort of this iterative process of problem solving as well as really focusing on the people and the roles that they play over whatever the technology does. - Wow, what else can I say? No. Hi, everyone. My name's X. Two lessons that come from that parable that was shared. The first is that you only want to move fast enough to move forward, right? If the solution was to get it done in the quickest amount of time as the parable sort of illustrates, then everyone would've died.
So there's a need to be able to consider how your actions will impact the different user groups of your systems, especially when designing artificial intelligence, weighing those against each other. If we zoom out and aren't just talking about fairness or just privacy or just safety but all of the different principles that typically fall underneath the concept of responsible AI are not universal facts. They are subjective concepts, whether that's fairness, privacy, safety, security, transparency. Those are all subjective concepts that are shaped by an individual's personal societal context and their own value systems, and so that can vary infinitely across space and across times, space being across geographies, across cultures, within communities, and over time, they change. So as we're designing these systems, we're always making trade-offs between individual value systems.
We're always making decisions about whose voice matters more, about whose value systems are actually applicable and whose are not, especially when we're designing at a global scale. So the question then becomes not can we make it 100% fair because 100% fair isn't possible. We don't believe in the same things 100% of the time as humans, as evidenced by the Republican and Democrat parties going at it right now, and so the question then becomes, well, who's making those decisions? What are the factors that are going into making those decisions? So who being, is it a company like my former employer, Google, who gets to decide whether or not an algorithm is biased, or is it the individuals who are affected by it that get to decide that, and how do we make those decisions in those trade-offs? And so to build on what you said in this parable, it wasn't like, well we said, "Well the fox is more important because the fox is the faster animal," like a lot of tech companies do. They'll say, "Well, yes, it's really nice that we wanna do something for Native Americans, but they're only 2% of the US population. They're never gonna significantly contribute to our user growth of revenue," and as someone who has fiduciary duty, cannot spend a million dollars on that research project.
So how do we balance that trade-off knowing that inherently in the way that these tech companies operate, they are always going to make trade-offs that have weights that are different than the ones that you and I might consider? So I think that's another thing that we have to take in from this parable is that the different groups of people as often as possible should be included in the process because it is possible to find a solution that works well, but we have to be intentional about seeking it out in the first place. - So X, do you think companies like Google or others would actually engage in kind of an inclusive process to bring people around the table to talk about, "Well, maybe we should include Native Americans in our algorithms?" You know, is that realistic? - While I was at Google, there were two different programs that we did run. One of them was run company wide. It was called the Owls Program, and it was around children's safety. So they would bring in children's experts from different domains from all around the world, and they would pay them and put them under very stringent NDAs, and then all different products across Google could come to them and consult and say, "Hey, we're building this new thing in YouTube or in the Play Store, or we're thinking about this privacy setting.
What do you all think?" And then they would take that into how they would design their products. We also did that. A colleague of mine, Jamila Smith-Loud, created the EARR, the Ethical AI Research Roundtable, which brought in different researchers into Google to do the same thing.
I think inherently what happens is, until there is a forcing function to hold them accountable for when it does cause harm, there is no incentive to do so outside of goodwill, and publicly traded companies are not motivated by goodwill. They're motivated by profits and bottom line, especially in a hyper-competitive environment where companies like Google and TikTok are at each other's throat for market share and for money. And so I think that until there is some forcing function where they can get in actual trouble for harming different communities, that it won't be something that they just inherently do, and it's not what I witnessed at Microsoft, not what I witnessed at Google. In fact, you know, the FTC is kind of trying to step up and do that. So there was a whole suit where Amazon, the little Alexa thing, was keeping children's voice recordings, and Amazon lied about it to the FTC. They kept it way longer than they said they did, children's data, and they only got fined $25 million.
That was their repercussions. And so I think, until collectively we shape a forcing function, similar to seat belts in cars, right? It wasn't until 2011 that the Department of Transportation required that seat belts be tested with bodies that were shaped like women. So up until 2011, female-bodied individuals were 60% more likely to die in a car accident, and trust that when they passed that law, all the automobile makers were like, "No, we can test it ourselves. We can fix it. We don't need oversight. It's gonna slow down our ability to make cars. and we're gonna not be able to innovate."
Well now those numbers around female-bodied individuals having less car accidents has gone exponentially down. So this is not new to a challenge that we're facing. It's just something that needs to be applied to this technology, and I think the implications are just as serious as not having seat belts that have been tested on different types of people, if that makes sense. - Yeah. Let me ask you other panelists.
Do you agree with that? Do you think there can be a forcing function that incentivizes companies to include ethical considerations and other kinds of considerations in their development and use of AI, and if so, who does it? Or do we get another Section 230 analogy, right? (Niloufar laughs) - Well, I think there are three main forcing functions. As far as I know, there's policy, of course, and oversight and regulation. Then there's public opinion. There was a lot of reactions. For instance, Facebook increased the transparency of their algorithms that target ads. They reduced the possibility of harm through ads after there was a lot of public opinion and backlash, and then I think the third one is labor organizing by their employees, and we've seen a lot of that through groups like the Tech Workers Coalition, various groups of people who work within the companies that have power to, you know, push towards change, given, you know, their power as the workers within the companies. Those are the three levers of power that I know, but I'm curious to know if there are other ones.
- Well, and I would say picking up on that third lever, kind of the workers within an organization, a number of companies have signed on to the UN Guiding Principles on Business and Human Rights, which are companies can voluntarily sign onto, and hundreds of companies have done so, including some of the big major tech companies. And I'd say they're really only effective when you have high level support for them, but for companies, it requires them to draft a policy talking about kind of what their major human rights risk areas are and to whom, to conduct regular due diligence on their products and services to assess for those risks, and then to come up with remediation measures if they find that certain risks are in play, and, I mean, we've seen it in use. I would say that the poor thing about it is that it often, and I mentioned this earlier, often happens after a product has already been created and launched into the public. But if we can create an incentive structure internally at companies so they're doing this earlier, I think that's a way to deal with it. - But that would be voluntary. - It would be voluntary. - You? - Yeah.
I mean, just, you know, we had the panel about the EU AI Act this morning, and with that going into effect soon, I think we will see companies having to reorganize how they're thinking about AI risks in particular and creating in the same way that GDPR kind of created those robust privacy protections and the kind of criticality of that in a lot of tech companies, there will be a requirement to focus more on, you know, how do we assess the different levels of risk and how do we do those impact assessments and following the different requirements that are laid out in that. In the United States, we'll see what we get. Obviously, we had the executive order at the end of last year on safe, secure, and trustworthy artificial intelligence that put some requirements on companies as well, including more information sharing with the government. So some pressures from that level that are more than voluntary but a lot more to be done. - Let me ask this.
Do you think the United States Congress is capable of dealing with AI in its current configuration? (audience laughs) Everybody. - [Audience Member] Are there any members of Congress here? - I would say I think it's capable of putting together and, you know, not focusing on the substance of it itself but focusing on processes that can be put into play where, you know, there are other entities that have the expertise that can monitor those processes. So I do think Congress is (laughs) good at creating processes, and I think rather than kind of focusing on them having the like real substantive expertise to do so to focus on that part of it. - So my company has just completed a contract with one of the government agencies that is responsible for drafting the legislation for the White House around open source model weights, and between that and the work that I did at Google, there are two things that can concern me that I think everyone in this room can help fix. The first is that with the government agency I was working with, I don't wanna like get in trouble if this is on video, they basically had two individuals out of the 40 or so people that were on this task force who were actually technical who had any type of background in AI and ML, and one of them had just graduated a master's program. The other one had done like a postdoc fellowship at Stanford, but neither of them had applied practical experience.
So here you are asking these individuals who are researchers who have valuable insight to solve applied practical problems, right? So there's a need for, I think, us to be involved in that through the open comments, through getting involved with these organizations, offering up your expertise, offering up your wisdom to make sure that the individuals who are day-to-day responsible for drafting and moving those levers of power have access. For example, when I did a little talk with them, one of the people's like, "Someone told me you couldn't even test an LLM for fairness. So we didn't even think about putting that inside of our regulation." Like that's the level of distance that's there. It's very normal in Congress and Senate for, when they want education on a specific domain, for them to go to the top companies in that domain to get that education. So, for example, they'll go get like a briefing on pharmaceutical stuff from 3M or from Pfizer or they'll go to Amazon, Google, and Microsoft to get drafted on their, you know, or to get briefed on their approaches to responsible AI.
So I helped make the ML for policy leaders course at Google that all it said was, "We got it. Don't look at our stuff. We can regulate ourselves. China, China is gonna beat us if you try to look at our stuff," right? Was kind of like the energy. And so making sure that we balance out the voices that are in those rooms that are educating folks by doing congressional briefings, teaching senatorial staff, making sure that like the research is distilled in a way where those folks can understand and be able to move policy forward.
So I'll stop. Sorry. - Let me ask, and this kind of follows up, X, on a point you made. You have these big players, mega corporations that are in the AI space, but a lot of the innovation is happening in much smaller entities, either in academia or small startups or what have you.
What needs to be done to make sure they have a seat at the table? Because their view and their susceptibility to the cost of regulation, et cetera, will be different than Google or Amazon, et cetera. - I think we need to develop tools to make that process easier. You know, a lot of the tools that we need to be able to do AI responsibly don't exist in a more productized way, so, for example, dataset evaluation. There isn't a tool that I can go to like a website and pay a monthly fee and plug in my data that's stored in Google or that's stored in my Amazon or that's stored locally on a server and then run analysis on it.
Some of these platforms have some of these tools built in, but there's not one where I could like plug in data from multiple sources. That's a whole startup idea right there that every company would use and then for different types of data for image data versus video data versus voice data versus text data that would then, after I run my analysis, put that inside of the report that I need to turn into the EU to show them that I've tested my stuff for bias and tested it and made sure that it was compliant with GDPR in the collection process. That tool doesn't exist.
It's like there's not a tool that exists that I can plug in my model and test for fairness and have those results put out or that helps me take in recent research and do that. So I think what's needed is products that are as easy for me to like download and run like a security penetration test on my network so that we can actually implement these principles and make them more accessible versus a place like Google that had a whole team of researchers and their only job was like to evaluate, it's called braids. It was like their only job was like look at datasets across the whole company, right? Like everyone's not gonna have that. So developing those tools, open sourcing those tools, making them available, you know, to collaboratively build will be critical to easing that burden. - Yeah, I mean, I think in addition to evaluating the datasets that the model evaluation is so poor right now. And so basically, you know, AI developers are kind of haphazardly saying, you know, "I'm gonna choose to, you know, do this evaluation or this one."
There's no standardization across the board, and the in like meta-analyses of what these evaluations look at, you know, the vast majority of it is just capability. So you know, understanding, you know, what can this model do? How good is it? You know, how much does it push that frontier? And it's a real minority looking at things like is this, you know, robust? Is it accurate? Is it fair? You know, can we rely on this thing? Furthermore, if you're looking for different modalities for generative AI, almost all the evaluations focus on the text modality and almost always in English. So there's just real gaps in what we even have, you know, the tools to your point to really understand, you know, how good are these things in the first place? And so what that means is people just put them out without knowing, and then all of that potential harm or risk is just put onto society.
- And I would just add, you know, also from a human risk assessment approach, you know, I work on human rights. So when I'm talking about risk assessment, I think about risks to human rights. You know- - Can you give an example? - Yes, I will, and I think, you know, the AI White House executive order really can kind of shine a light on this.
So, you know, it talked about potential harms to the workforce. Well, workers' rights are human rights. It talks about harms to privacy. Well, privacy is a human right. It talks about unintentional bias and discrimination. Well, freedom from discrimination is a human right.
So I think part of what we need to do is learn one another's language and lexicon because I think a lot of them are shared concepts, and we need to learn to kind of advocate together. But I was gonna say, just from a human assessment standpoint, you can do that with just a few people in the room, right? To think about what are the, you know, just projecting out what might be the risks. Yes, there's the technical component of it but also from a human component. What might the risks be of this? And I can say from a policymaker standpoint, a lawyer standpoint, and a human rights person standpoint, like those are things you think about all the time. You know, it was said earlier, trust and safety is catastrophized, whatever the word is. I think it is similar, and so I think it really just takes a few individuals sitting around and thinking about that for a while to come up with some potential.
- Let me poke on that a little bit. So you've mentioned privacy several times as a human right. It's a human right in the United States. Other countries you could argue don't put such a high value or don't operate with it as such a high value, and their use of AI in a surveillance context is going to be much broader and probably much more widely accepted. So my fundamental question is who decides which rights are gonna be embodied in this technology that, by its nature, is gonna be international? - Technically speaking, 193 countries agreed to the Universal Declaration on Human Rights.
So I understand and totally agree that how that is interpreted varies drastically by where one is located. I think who is determining them is actually the people, wherever that company is located, working on it. You know, I think those companies that are most successful bring in engaged stakeholders throughout the world. That's something we're doing in our human rights assessment of LLMs because the way large language models are used vary so significantly based on where you're located.
So you have judges in South America and Latin America using them to help make decisions in their cases. You have educators in the Middle East and Singapore using them to help assess student work. You have journalists in Southern and Eastern Africa using them to write news stories.
So the way in which LLMs are used varies so significantly based on where you are. So I think the companies that do it right involve stakeholders around the world in helping to make those decisions, but I definitely hear you that there are different values upheld differently by different nation states. - Anybody else wanna pursue that? - I also wanna go back. So one thing that I do a lot when I'm thinking about LLMS and what's gonna happen is thinking about social media and what happened there because I think, comparably, it's another type of technology that came out.
There was a lot of optimism around it, and then it ended up having these effects that no one could've predicted, like fake news. There was good things that no one could've predicted, there's bad things that no one could've predicted, and it's an example where different countries actually regulate it in different ways. So you can have the same social media company like Facebook or Twitter, and the way that they're run in Europe is different from the way that they're run in the US. So I think we have precedent for these large companies actually matching themselves to whatever values or rules are applied to them in their local context, but also, I think at the same time, it's really hard to think about this question in the abstract, you know? Evaluate your LLMs. It sounds really good in theory, but in practice, it's actually incredibly hard. I think a lot of the things that came out of Bard of chatGPT no one could've predicted, not even the people who made it, and I think it's more fruitful to think more directly at the harms, so going back to the human rights.
So I think even for Congress to be like, "Let's fix the AI," I think it's less fruitful than saying, "AI is being used in hiring," and we already know the challenges with hiring. There's disparate impact. There is you're not allowed to use tools that are not directly related to being able to do the job. We already have precedent for what tools for hiring have to look like, and so we can go look at the AI tools that are being used for hiring and much more clearly see what needs to be done there. There are other examples like in the criminal legal system and AI used for, you know, risk assessment for education.
I think it's much more fruitful to look at where it's applied and where the risks are and what the concrete harms are and then work back from there to see, okay, how should the AI or the tool be evaluated and governed for that? - In other words, move the cabbage itself across the river - Exactly. - and then, right, as opposed to all three at the same time, which was your point at the beginning, right? To take it incrementally. And now I'm talking about data poisoning and so forth, which one of the last speakers said seemed to say it was almost an unsolvable problem. How should we account for that in terms of balancing innovation and the ethical use of AI, and what ideas do you all as people in the field have for dealing with that? - Yeah, I think in terms of where that balance is right now, you know, it is very, very strongly on the side of we're gonna push forward innovation at the expense of security, knowing that there are very much unsolved security vulnerabilities with these systems as well as other limitations. Yeah, Ron spoke about the data poisoning and that, you know, there aren't great solves for that.
Certainly being aware of your sources for data and having some kind of, you know, control or trust of that helps, but that's not always possible in practice, but, you know, even once you have a system, you think it's, you know, relatively secure. We know with LLMs they're susceptible to prompt injection attacks, and, you know, all of the great safeguards that people are spending months and millions of dollars to put in place are pretty easily hackable and, you know, either directly or indirectly. And so, you know, strange things happen, right? So you can ask it to role play as a white hat hacker, and it'll be like, "Oh, okay, now I'm good. I'll tell you how to do the cyber attacks," or, you know, just things that people don't expect.
Like you say, "Just repeat this word," you know, over and over again," and it'll do it and do it and do it, and then it'll get bored and it'll start, you know, spitting out data that turns out to be trading data, you know, including people's social security numbers and things like that, right? So just like weird stuff that happens. And again, these are not like patchable bugs that we're used to with software development. This is based on the fact that they inherently learn from data, and they're being released inherently as instruction following machines that want to follow whatever instructions they're given, whether malicious or not.
- So I'll jump in. I think one, there is a laziness that happens in responsibility inside of tech companies when there is no forcing function. So for example, the datasets that have been used to train Bard or to train LaMDA, its predecessor, are extremely large datasets, extremely large databases containing vast amounts of text with millions and millions of embeddings inside of them, so relationships between the texts. What tool would Google use to go and look at that and say, "Is this fair or not or who's represented and who's not?" One doesn't exist.
The tool that was created, the language interpretability tool or I think it's something interpretability tool, the LIT tool, you can look it up, can only handle datasets of a certain size, and even then, that research team at Google was two people. (laughs) The whole team, just like my skin tone team, was two people, right? And so- - That's not big. - No. It's an engineer and a product manager, right? Is building this thing. And so at what point did they look over everything and say, "Here's who's represented, here's not, here's how we're gonna balance it out"? They didn't. They just threw it out. And even the process of curating that data, there are some people who are getting to decide what goes in it and what doesn't, right? They're saying, "Hey, we're gonna take out, you know, there's a research paper that, oh, it caused so much uproar internally.
It was like a dataset these people had put out in research, and they had published it, and it like went on Google's website, and the data construction methodology, it was like these engineers just decided that any terms related to sex, sexuality were taken out, which meant that all LGBTQ, all transgender identities, all conversations, even if they were positive, were removed from that datasets. Like who gave you guys the authority to like make that decision? Like why didn't you come talk to anyone else? So there's no forcing function, right? So I think the issue of data collection is that, one, you will always be sampling from an infinite pool of possibility, so it will never be fully representative, and then two, we need the tools to actually be able to look into what we're sampling and putting together to be able to test them to be able to know, and I think maybe that's where that conversation comes from of you can't test the LLM for fairness. You can't check the dataset because no one's forced you to. When the EU's AI Act dropped and they said, "You're gonna have to demonstrate your dataset lineage," I guarantee you that that LIT tool team is like five or six people now at least rushing to build that tool because they need it for industry and for market, right? - You know, there's been some suggestion in policy circles of there being a licensing type or accreditation function for AI, perhaps administered by the National Institute of Standards and Technology, which is part of the Department of Commerce.
Would you all support that idea? - It's hard to tell. - Is that a forcing function? - In service of what goal? Like in service of like we would get paid for our data? - In terms of the goals that you just said, to make sure that data, that it's collated properly, that it's non-discriminatory, that it is inclusive, et cetera, et cetera. - So I think that there are market opportunities, and I think that the individuals who create the data should be compensated for it.
I don't think we have equitable models for that right now. Like Meta just released like a image generation model trained on three billion Instagram photos. So congratulations if you've ever posted on Instagram. Your photos were used to make that model, right? And how many of you guys got a paycheck from that? Increase in followers, likes? Got a new sponsored post? No? Okay, exactly. So I think that we do need compensation models but that are more directed at the consumer, but I think it's not getting paid to be able to do, like to have the data that's the piece that's missing.
It's the evaluation forcing function and the consequences. We see this in every industry. If the consequences are minimal, they'd still do the thing and then just pay the fine.
- [Janet] Right, so the consequences would be that you wouldn't be certified or licensed by the federal government. - Yeah, I mean, that's what the EU is saying, that you won't be able to operate your models within their borders. "If you want to literally be a business that uses this technology here, here's what you have to demonstrate, or you cannot use this as a consumer base."
Now I think what I know internally from some of these companies, some of the reason they're fighting so hard is because, if it were to come out and they were forced to evaluate their models and demonstrate, they'd be in violation of many, many federal civil rights laws. They would've been shown to have been lying in many federal court cases claiming things were not biased when they absolutely are. And so there's a fear coming from these companies who have been developing these models who don't have the techniques to repair them, right? So the repairing an AI model is very different than a regular piece of software.
If we have a food delivery app and you update to a new version of iOS and your checkout button stops working, I go back to my dev team, I give them the bug report, they go into the code, they update it to match the new version, and they push and update and it works. With artificial intelligence, that's not the case. It requires a comprehensive rewind of the system.
You have to first recall the model, stop letting people use it. Then you have to go grab all these interpretability techniques to even figure out why it's doing what it's doing that you don't like. Then you have to go grab a bunch of data and collect and clean that data to prepare it to balance out what it's learned, and then you have to retrain it all over again.
So the business risk of not doing this is really, really high. Now imagine these companies who have been doing AI 20, 25 years who didn't think about these things from the start now at risk of saying, "Well, let us see what you got." They're terrified. And so when we think about the future, building responsibly, building those processes in from the beginning, checking your datasets, building interpretability, black box models don't exist anymore, folks. There are free tools from every major company where you can see why they do what they do. Putting those inside of there, making sure that you have some element of like community accountability and some body that's responsible for reviewing them is necessary for good business, but I'll stop there.
I could- - Yeah. - Thanks for coming to my TED talk. (audience laughs) - Do we have questions, Gene? - That's coming up. - Oh, okay. Here's another conundrum I wanna pose to you all. This is a really interesting conversation, but to the extent that AI is viewed as kind of a new space race, us against the Chinese, right? And the first to the AI moon, if there is one, wins.
Do you agree that that kind of race exists, and to what extent would the kinds of things we've been talking about, building in some ethical guidelines, some forcing functions and what have you, intervene or slow down the United States companies' ability to get to the moon first? - So Stuart Russell talked about this a little bit in the panel this morning in terms of, you know, China actually has pretty strong regulations on LLMs and goes further than what we have in the US and EU in a lot of ways. And so I think the, you know, but if we don't do this, China will argument can be pretty dangerous. I think what has actually been like the most shocking development over the last year from my point of view is the corporate competitive dynamics, mostly within the United States where companies that had long understood, you know, LLMs as, you know, an interesting research phenomenon, you know, maybe a cool art project suddenly felt pressured to put this out and pretend that it could, you know, give accurate information or be part of a search engine and, you know, actually be used by enterprise around the world. And so, you know, there's been this push to continuously put out a bigger and more capable model, and so now we're seeing, you know, new things released all the time, and that dynamic, I think, has gotten outta control, and we're gonna see the harms of that for a long time to come because people are actually putting these models into high stakes settings where they do not belong. - I go back to something I mentioned earlier on, which is the fact that if you embed the risk assessment at the beginning of the process, it doesn't slow it down.
What slows it down is that it often happens after the product is released, after it's put into public, and then you have like the race to develop it and then a slow down where you need to fix all of those risks that you then identified whereas if you have it embedded in the process, it will slow it down much less so. - And as X described, the fixing is a lot more complicated than one would assume and expensive, right? - I think there are two things that are wrong with that argument. One is that we're really, really, really bad at predicting how quickly this technology is gonna advance. I think where we are right now is much further behind than what people would've had us believe we would be by now when chatGPT first came out. It was supposed to go so much faster, and it hasn't really changed that much.
So I keep going back to this story of Marvin Minsky, who was one of the founders of the whole field of AI, decided at some point that for AI and what he thought of AI is what we now sometimes call AGI, he thought that for that to be possible, for it to actually work, the models needed to have some kind of vision understanding, so understand what are in pictures. And so he thought, "Okay, this is a big sub problem." He got a student to work on it, and he gave the student a summer, and the student was an undergrad. So he gave the whole of the computer vision, which this has been going on for decades now, to a single undergrad to solve in a summer. So we are really, really bad at predicting how hard these problems are.
Like X was saying, and fixing them is really hard. So there was that big story of how the Bing chatbot was telling a reporter that it had fallen in love with it and to sort of free it or something. It made the news. This thing has become sentient. I bet you it took a ton of time and money for them to figure out what was going on.
Turns out what was going on was that there was a novel in which this was a story, and the model had started picking up examples from the novel and just continuing the story. So it takes a ton of time, ton of money, and we figure out, "Oh, this is just a subplot in a novel," but even if we take out that novel from the data, which is not that hard, there are millions of other novels in the data. So it's not that we've solved the problem. It's really, really expensive and hard, and so I think there are two parts to that.
One is we're really bad at predicting how quickly this is gonna go and where it's gonna go, it's really, really expensive to fix, and I think the other part is that there's no singular definition of what innovation is. There's no singular future that everyone is going towards, and thinking about other things and thinking about harms will slow us down from. I think there are millions of potential futures, and we get to choose which future we wanna build. And so I tend to think of it less as there's a moon that we're all going towards and this will slow us down and more that we have agency of what our moon is, where are we headed towards, and each of these will have some benefits and some harms, and we have a responsibility to decide what that future is. - I love those comments. (laughs) I'm gonna watch this recording back and like take notes.
As a military veteran, a military combat veteran, I do not like the US first China narrative. I think it plays there is real competition in the market between them, but the reality is companies like Microsoft have subsidiaries called 21Vianet that provide the exact same cloud services and computing services to China, just under a different entity, right? So I don't personally like that argument, but what I will say is that we are in an AI arms race, but it's not us against someone else. It's these companies against each other. When you look at market dynamics over the last three years, TikTok emerged as the number one video platform, social media platform, and it is not US owned, which became a significant threat to YouTube, became a significant threat to Meta, became a significant threat to, what's the other one people use? Snapchat, right? Sorry. I'm clearly not the one, to Snapchat, right? And these are companies that are American grown companies that have been dominating this space, whether it was through images or videos, that now have a significant threat, have lost market share. Their average watch time has gone down, so now they're all trying to find new ways to compete, and then you have chatGPT dropped randomly in December of 2022, and I can tell you confidently that Bard was not a product when chat GPT dropped.
It sent the company into a frenzy. There's a report that you can read in the "New York Times" about how Google went into a code red, where all of the senior leaders got together, from Kent Walker, the chief legal officer, to Prabhakar, the head of search, to all these different individuals came together, and they said, "Uh oh. We're no longer the industry leader in AI, and that's kind of the only thing we've always been.
So what are we gonna do?" And from that, from the report, I wasn't in the meeting, but from the report I read in the "New York Times," they said, "We don't care about fairness. We don't care about safety. We don't care about security. As long as there's no child sexual abuse material and it doesn't say something bad about a US politician, we will put it out on the market."
In fact, Google did a review of Bard, of the system in their AI principles review process. The reviewers said, "Don't release it, it's not ready," and one boss went in and said, "Uh uh, I think we should," and changed the whole report and pushed it out, and then they launched it on the market, and that's another report that came outta the "New York Times." Now I promise I wasn't the one snitching, but these are things that are actively happening every day.
So these companies want us to believe that it's an existential threat to our safety and national security so that we can encourage them to over skip those guardrails, but right now, what's truly happening is just someone's fighting for market dominance in AI. Between each other, it's a Google, Microsoft, Amazon, Apple, IBM kind of somewhere in the corner battle, and who's gonna be the market leader? And it's the one space that opened up. Just like a few years ago, it was who's gonna be the market leader of cloud? And clear winners emerged. It's Azure, it's Microsoft, or it's Azure, and then it's Amazon, and then it's, you know, Google somewhere in there and Oracle around the corner, right? Like (laughs) it's the same type of thing.
And so I encourage everyone here to not allow our mindsets to be moved into larger narratives that policy and military folks are handling in ways we cannot 'cause we don't all have top secret clearances here and to focus more so on the way in which we want these technologies to be used in our daily lives, how we want them to be held accountable when they might be violating or incongruent with our civil and our constitutional rights that are guaranteed to us as people in this country, and making sure that we are doing our part, one, as individual citizens, two, as academics, three, as just humans who give a, I'm not gonna say the F word, but who give a what what to make sure that we're using all of our personal power and agency to push forward, you know, policies or, you know, the three levers that she talked about that are gonna enable these systems to be designed in a way that isn't just we get to be the guinea pigs because Google wants to beat Microsoft or because Microsoft wants to get back at OpenAI, right? Like we need to play a much larger and more intentional role in shaping the way that these systems get regulated the same way they need to play a more intentional role in the way that these systems are designed. - Okay. (audience applauds) We now have questions from the audience, and the first question actually is reflective of a lot of the comments made today, and X, we're gonna start with you and come down the row this way 'cause I waited till you took a drink of water just to do that, but here's the question. "A profit motive is critical.
Can we align," and I like the word align, "the profit motive with values-oriented AI?" - I think we'd have to look at from a legal perspective, and maybe this is something you can actually answer, Betsy, but the challenge of breach of fiduciary duty, right? When you are a director or above at a publicly traded company, you're considered an officer of that company, which means you have a fiscal responsibility to make the company money. So that conversation I shared earlier about the desire to not do things, like not giving me a million bucks to do some US research with Native Americans is a very real one I had with the VP at a very large product at Google. And the reasoning, as they went on to explain, was literally, "I don't wanna get sued.
I don't wanna be found in breach of fiduciary duty." If I can take that million dollars and apply it to the black American community, which is 12% of the US population, which has a much more significant factor in our user base and in our advertising dollars, and someone looks back at my budget for the year and says, "Hey, I didn't spend this correctly," I am personally liable for that, and so I think I'd pass it there. Like maybe there's a reevaluation that can be done.
Maybe there are some exceptions we can think about on a policy level where people are granted like, "Well, if you did this to align with the civil right or with a constitutional right, then there's some type of exception," but that's really where the fear comes from and why that trade-off happens at that level because those individuals are personally and legally liable, and they're going to put that above what may be best for common good. - I think in the business and human rights space, we see pressure often coming from investors and from users. So a lot of the big steps companies take to better protect the rights of kind of users and those who are impacted by technologies tend to come from pressure they receive from their consumer base, from their user base, and from the investors. And I'd say the investors have a lot more that they can do, and there's certainly the regulatory element of it, which right now is not doing much of anything at all. But I think that's one that we can be beefed up to further provide incentive. - I think it also tends to matter what that investment looks like 'cause if it's, again, say, a venture capital firm, they again have a fiduciary duty to maximize profit.
So I think it goes back to the three levers of power, and sometimes they do align with profit. So for instance, the public opinion or the users, the consumers, they relate to profit in the way that they choose to buy the product or not, but it's limited 'cause like how many Amazons and Googles are there? How many options do you really have? And then again, the other levers. So there's the governmental level. That tends to have less to do with profit, although it can slow a company down, and that's, I think, how it relates to profit, and that's why they're so scared, and they might actually do something because if it takes a year to launch a new product versus three months, then that's a ton of money, and then that relates back to profit. And then I think the third one, employees, you know, really good ML engineers are really, really hard to recruit, and so there's, again, a profit motive to want to get the best employees and keep them, retain them, and keep them happy, and, again, I think that sort of relates to the profit. But I think all three of them do have relationships to profit, but they're not perfect because they each have their own limitations, and I think a real change happens from all three levers happening at the same time.
I don't think you can just do one and be done with it because there's a ton of money. These are really, really big companies, and so it's gonna be really, really hard to make them to change anything. - Yeah, I would just add, you know, companies experience these embarrassing things that happen, like racially diverse Nazis, for example, that we saw from Gemini not too long ago, and it cost the company a lot of money and energy to fix and deal with those.
So, you know, companies don't wanna be out there getting, you know, in the latest PR news cycle with their name splashed all over the place for these kinds of errors, and so there are internal incentives, but, you know, they're just not sufficient, right? And so then you see some companies try to take the approach of, you know, "We're gonna have a slightly different corporate structure, so OpenAI and Anthropic," right? With a different legal corporate structure. We saw it in the OpenAI case, you know, how that fell apart, and with the nonprofit board, you know, made a decision, and effectively, and Microsoft said, "No, you can't make that decision. Our power is greater than than yours," and the CEO is reinstated, and here we are. So I think that's a good lesson that those kind of alternative models are an interesting experiment and maybe, you know, not enough. So I think we certainly need, you know, guardrails, regulation because it's what the kind of self regulation that we've seen for decades in this space is not sufficient, and we have ample evidence of that. We need to see more access for independent researchers into these models, and (laughs) that's currently a challenge.
There's real, you know, power dynamics at play here in terms of, you know, who's creating these models and who has access to them, and, you know, for a lot of the public sector, they are reliant on going to third parties to get these systems, and for a lot of researchers, they're putting themselves at personal, you know, legal liability to do some of the research that they're doing to understand, you know, the potential harm. So yeah, a lot more we need to do to better align those incentives. - All right, Brandy just passed me a note to say that we need to finish this session. I wanna thank you all. Think about the rabbit, the fox, and the cabbage 'cause I think we've just peaked at some of the ethical, legal, and other conundrums that go along with this fascinating new technology. So thank you all very much. Thank the panel.
- [Brandy] Thank you. Thank you. (audience applauds)
2024-05-05 22:58