What is experimental statistics?
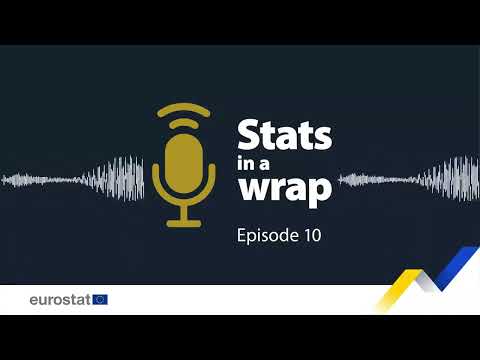
Stats in a Wrap, the new podcast series from Eurostat. Welcome to another episode of Stats in a Wrap, the podcast series from Eurostat, the statistical office of the European Union. With this series, we want to immerse ourselves in the world of official statistics and to pick its most delicious morsels and striking flavours to give you, our listeners, insights into the unexpected, the quirky and downright peculiar perspectives that only the numbers can reveal. I’m Jonathan Elliott, your host for this episode.
And today is something of a special day because the podcast is celebrating the start of its second season and its 10th episode, yay! And to mark the new season, we’ve got something of an unusual addition this week, because instead of our usual two contributors, we have not three, not four, not even five, but six statisticians in the wrap café today. There must be a collective noun for stats people - if you know it, please tell us or make one up. Yes, it’s something of an experiment today to have this many people which is just as well because the subject of today’s podcast is experimental statistics. The phrase is a curious one and conjures images of mad scientists labouring late in laboratories with test tubes. - In fact, Eurostat’s icon and logo designating stats that are experimental features a test tube.
So what on earth are experimental statistics? Should we trust them? Who does them and why and how? We’ll be finding out from our experts. I’d like to introduce our three teams. This is not a competition.
They may be teams but they’re not competing with each other, at least not officially. There are two from Eurostat and one from the Danish government statistics department: Statistics Denmark. Welcome, everybody. Let’s start with our guests from Denmark, Soren Anderson and Kirsten Balling.
Folks, great to have you on the show. Thank you very much for joining us. Would you like to say what it is that you both do at statistics, Denmark, Soren? Well, my job is to be director for the Department of Business Statistics, which include conduction of a large number of business surveys and the collection of data across the whole office. Lovely, Kirsten.
Well, for 25 years, I’ve been doing statistics in the economic sphere, economic statistics, you know, the things like growth figures and bills and payment and price statistics and national accounts. That kind of statistics. Lovely, and are you both in Copenhagen? Have I got it right? That's right. So joining Soren and Kirsten are the first of our two Eurostat teams comprising Agnieszka Zajac and Albrecht Wirthmann. Your official job title is head of unit methodology and innovation.
And Agnieszka is in the same unit. And so you’re both sort of right at the heart, if I got this right, of what experimental stats are, and what they do, is that fair to say, are you keepers of the flame of experimentation? That’s fair to say. And we’re struggling hard to bring new things into statistics.
And also keep statistics up to date with the developments that are now taking place. Well, you have to move with the times, innovation is vital and modernization is too, you’re at the cutting edge and great we’ll be hearing more from you in a minute. And our last two contributors, which I’m calling team tourism, they are from the tourism statistics department of Eurostat. Christophe Demunter, an old friend of Stats in a Wrap, and his colleague, Simon Bley.
Welcome, gentlemen, you are the brains behind the highly experimental and fascinating collaborative economy project, which looks at statistics on short stay accommodation, Airbnb, booking.com, and so on, basically people who rent out their flats to tourists about which we know very little, at least in official terms. But you are about to or actually in the process or have done, you have changed this. So it’s really huge.
I mean, this for example, is it true that every minute nearly 100 bookings are made on Airbnb, booking.com, TripAdvisor and Expedia in Europe alone? Is that true? Christophe? It’s an amazingly high number. And it actually shows how important the segment of the accommodation market is. Of course, it also shows how important it is that we actually include it in our statistics. And that’s just in the 27 member states.
I mean, we’re not talking globally here. We’re talking Europe. That’s in the European Union. So while you were asking the question, and I was answering, maybe 100 trip bookings were made for the weeks and months to come. Fantastic. Great.
Well, we’ll be coming back to the project and unpacking how it was done, and the challenges it phased in its development later. But first of all, let’s have a look at where experimental stats have come from and why they’re becoming more and more important. We have to go to the gurus, the keepers of the flame Agnieszka and Albrecht, you have the overview, the source data used for statistical analysis is becoming much, much more diverse than it used to be. I mean, when you hear about the traditional sources of data, censuses and registers, it seems incredibly old fashioned. And now we’re inundated with streams of information from everything from satellites to smart meters, and even mobile phone activity. But how does this play into why experimental stats are becoming more important? Experimental statistics are becoming more important because there is a need for faster statistics.
We saw that with the crises, the pandemic crisis, but also now with the energy crisis, that there is a need for data immediately, so not only one year after the event happens. Politicians and society need this data immediately for solving the problems. And this is where we try then to step in to find new data sources. I’d definitely rather wait to get quality data, but it’d be nice to have if it’s going to take a long time, I’d like to have updates, maybe release some of the findings a bit sooner than the rest.
If it means to just keep like the trust in. I wouldn’t want incomplete data, because you can’t really use that very well. But I’d rather wait. So timeliness is critical here. But the touchstone of official statistics has traditionally been accuracy.
The technical definition of experimental stats are that they’re ones that are in the research and development stage where they don’t have to meet all the quality criteria, which are normally required, and these are laid down in regulation 223/2009, no less. And they are, let us remember: timeliness, relevance, accuracy, punctuality, accessibility and clarity, comparability and coherence. Agnieszka, could you just help us out a bit with what makes experimental statistics? Yes. So as you rightly said, experimental statistics are in the research and development phase. They are produced in a robust statistical quality context but they are of a little bit lower level of maturity, as compared to European statistics in terms of harmonization, coverage, or methodology.
So in general, we say that they can score higher in terms of relevance and timeliness, but fall a little bit short, maybe on other quality criteria, like accuracy or comparability. Fascinating if we were all in academia, but we’re in official statistics here. I mean, I can hear voices of conservative anxiety saying, this is all very interesting, but frankly, you know, keep it in academia, we need official statistics, we need it to be absolutely perfect. How would you respond to that? I mean, what do you say to people who think experimental statistics can’t be trusted? I think we need both: We need the traditional, absolute accurate statistics and probably also slow statistics for fundamental things like the total count of the population, which has some consequences on the money that is distributed within the European Union. But we need, as well, fast statistics where users are aware that probably it’s not accurate at the 1% level, but which gives them the direction of the development of the problem.
So when you are, for example, in a pandemic situation, you are taking some actions, and you want to know: do the actions contribute to improve the situation or do they worsen the situation? And there we can say, at least with this type of statistics, they are improving or they are worsening the situation. So the answer that we are giving is then adequate or should be adequate to the needs for the data. I think in the case of like a pandemic or a very urgent emergency, it’s very difficult to rely on qualitative data because it just takes so much longer to gather it. I think we cannot, we need to also look at very short data, very current data. But I think if I want to make up my mind about the topic, I’d rather look at data that has been developed for a longer time in a process that takes more time than just a quick decision or a quick thought about the issue.
That’s fascinating. I mean, I will go on about my favourite analogy that experimental stats are a bit like trial pharmaceuticals. I mean, if you’re lying on your deathbed and somebody comes along and says, this is very, very good medicine, but it hasn’t gone through all the time. Give it to me now! I don’t care if you know, it’s gonna save my life, fantastic, because there are statistics which are good. But if they come in two months’ time, they’re useless.
So they have a sell by date on it, even if they’re not ideal and the pandemic, I mean, Albrecht, just unpack for us a little bit about the pandemic, because clearly there was a desperate need for accurate information wasn’t there and, and you guys must have been feeling the heat, somewhat. Yes, this is true, there was a big need for data on infections, then, for example, on the number of people that got infected, on available beds in the hospitals, also on the people who died. We struggled hard to get these data not only using the fancy new data sources, but also going directly to the hospitals and organizing the data flows better so that we instead of getting the data a quarter after, but getting the data one week or on the day after they were collected.
Do you think we’re in a culture now where the need for rapid information, people’s expectations to have information quickly, is making experimental statistics more relevant, because suddenly you’ve got debates now, which can’t wait for the information, can’t wait for the statistics, are we seeing a trend here, Albrecht? There is clearly a trend, we see more and more data coming. And also people now, if they don’t know anything, they just go on internet searches and then get the answers immediately. So there is an attitude of getting answers immediately. And this also impacts, I think, official statistics and then we have to follow this. On the other hand, what I also can see is that people are confused about the wealth of the information.
And they are asking: Is this information correct? Can we rely on this information? And there I see an additional role then by the statistical offices to make a quality stamp on certain information. And that’s critical, isn’t it? Because if you can supply information showing how you created these stats, then that sort of protects their usefulness, doesn’t it? Yes, we are following a kind of an open source approach there. We try to make this data or the calculation of the data as transparent as possible, we have a discussion on scientific methodology, we try to document the methodology, the algorithms, also to have some scrutiny by the public and to make transparent the way the data is generated. To educate and also to get proposals for improvements.
I don’t really trust information that I read on Facebook, because for the last few years, there’s a lot of fake news on it. And you often see articles that you cannot trust, in my opinion, because you don’t know if everything’s like trustworthy. So I do not trust information that I find on Facebook. Christophe, if I may just jump in here. We talked about this before, when you said that the importance of this kind of information is almost to get ahead of the game, because there's plenty of statistics out there.
There’s loads of information, but none of it is official. Some of it is quite dangerously inaccurate. So is there a sort of need for official statistics, and there’s people who work with it to try and keep up perhaps with what could be fake news or just, you know, irresponsible information? Yes, certainly. I think it links to what Albrecht said earlier on.
There’s some kind of market for data. And there’s no monopoly for official statistics. Official statistics are made by the government, but it’s not the only statistics around. When people want to know which artist is singing a song on the radio, they use Shazam. They have the answer in two seconds.
Basically, for data needs they want to say they have a question and they want to find the data immediately. That’s what Albrecht pointed out people go to the internet, and there’s all kinds of data. So in a way, we have to safeguard that there is also some official data for whatever questions people might have, and preferably in a timely manner. And this is probably where experimental statistics can help to offer statistics that were previously not available, which is exactly the case with the projects that Simon and I are talking about.
There was no data previously, on platforms. There are data providers, like inside Airbnb, AirDNA, but their objectivity is not always clear to users. So this might be where we step in with official data, we do get hits from the platform’s directly, but we do have a whole battery of tools to test the data to process the data. So what comes out of it at the end, what we publish, we assume it’s reliable, impartial data. I trust the statistics and data from the government. Because I come from Sweden, and in general, there is, often the data is correct, and we have an opportunity or possibility to cross check.
So coming to Copenhagen now, because I think there’s a side to experimental statistics, which is, well creative and innovative, and it engages totally other stakeholders. Kirsten, you’ve described this field as a playground or a sandbox, where you can work with universities, academics, and well, theoreticians to look for new methods and new techniques that help stats reveal things they normally can’t. I mean, you and your colleagues are opening up new frontiers, right? There’s another kind of experiment to statistics where the statistic being compiled is to a large extent moving away from being directly an observation of what you want to say something about.
By moving in that direction, one would traditionally have said, that this is not statistics, this is modelling. But there are phenomena that you cannot hope to be able to observe directly and still want to be able to describe statistically. An example could be when you want to say something about climate footprint, the consumption we have in Denmark has, globally, then you need to know something about the CO2 emissions in China, caused by producing the consumption goods that we import from China. And China is not likely to observe this for us, letting us conduct a survey in China to tell us this. So we have to do something else to be able to provide our decision makers with information on what are the consequences of the consumption we have in a rich society such as Denmark, on the global climate.
And in that case, we have to rely on modelling, we have to rely on international cooperation, because we cannot do this alone. We don’t know everything about China, for instance. And you can say why policymakers want this data when it is compiled using models and international corporations where you let go of some of the control of the production process of this data. And I’ll say they do that because they want all the other quality criteria coming out of statistics from independent statistical bureaus, like for instance, that it’s pre-announced, it’s produced repeatedly.
It’s followed by metadata, it’s free of political influence, and it’s made available to all users at the same time, to mention some of the more important ones. So, if we want to compile the footprint of our consumption, we just have to do something else than what we have traditionally done when we have been producing statistics. In doing that, we have to cooperate in different ways, we have to use new techniques, we have to use new ways of compiling statistics. And that is by nature experimental because we’ve never been there before. With friends and family I share a lot but when it comes to the government or others, otherwise, I would not like to share personal private data like medical history or medications or whatever but I don’t mind sharing what I earn or my utility bills or yeah, how much electricity I use and so forth. If I do recycling and whatever.
Soren, at Statistics Denmark, is there a risk that organizations like yours, you know, an official body that has to look highly correct in all that it does? It could risk appearing, well, a little unprofessional because it embraces these non-conventional techniques? We have traditionally been cautious of going down this way of the experiments because we were worried that it could jeopardize our reputation. What we saw during the pandemic is, also Albrecht was mentioning before, it was actually a breakthrough for the experimental way in the sense that it added to our credibility and it added to our relevance, and the respect that many stakeholders showed in statistical agencies that we could actually produce usable information in new ways in a very rapid time. Information was really desperately needed for the policymakers. Some people may say that statisticians are not born courageous, they become courageous over time.
And I think in this interaction with our stakeholders it also proved that we were courageous together, and it actually proved a success. So now we have an appetite for more. Fantastic. So well, you’re more collaborative and more fearless. Because you’re not sitting in this professional straitjacket of ultimate perfection and utter accuracy. You can, if you need, say, guys, it’s a little bit of give and take here, there’s a little bit of flexibility, you just have to accept the fact that we won’t get it completely perfect.
Well, we won’t and we’ll do the best we can. I am fascinated by the Smart Meter story in Denmark: For every household and business in the country. Correct me if I’ve got this wrong. You’ve got a reading from their smart meters every 15 minutes coming into your statistical office.
Soren has sort of opened his mouth and shock I fear I might have my facts wrong, Soren, help me. Well, it’s actually, you almost wouldn’t believe it. It’s not every 15 minutes, it’s every 15 seconds. my God. Right! Yeah, it is actually quite incredible. Hi, it’s milliseconds! Hey, what hey! It’s an experimental podcast.
In Denmark, all households and all establishments, which obviously all of them consume electricity, they have these smart meters installed to measure the electricity consumption. And these meters, the so called smart meters, they read the consumption every 15 seconds and the data are collected. And this data has been made available to Statistics Denmark, actually started just before the pandemic hit us. And we have then been using these data for different types of experimental statistics.
And I could get back into that in a second. But actually, this is a very good example of a new data source that has multiple purposes for us. Simon Bley from Team tourism, do come in here.
I’m fascinated by this. Because I’m thinking about the German way of doing this. And in Germany, I get a letter, once a year telling me please read your meter and tell us what it says once, so you have every 15 seconds, not once per year, get the data. I can comfort you.
It was like that only a couple of years ago, so. So it’s probably coming to you as well. I think all of this data is used by companies and it’s also sold by companies from me, like for example, if it just sent a message or a text message to a friend and that friend is on the other side of the world. I think that data is used in some way. Maybe not knowing what I said but for sure knowing that I send a message to another country, to another location and I think those companies yeah make their money with selling it. And not everybody in Denmark is a huge fan of Statistics Denmark getting their meter data every 15 seconds.
It’s quite controversial. Well, actually, I think it’s not controversial that we get the data, the observations, they are automatically collected by this private data holder that is kind of responsible for electricity supply across the energy market in Denmark. And they have the obligation to make these data available for research.
They didn’t have the skills and the infrastructure to do so. But they knew that Statistics Denmark was very experienced in providing high volume data for research purposes. They said could you please be the custodians of these data for research and statistics? And we of course said yes, we would be happy to do so. And we have had no problems with citizens or enterprises not being happy with us having the data and making them available for research in a confidential manner. So it’s not that we can see an individual identified person using much electricity at some strange point in time. I don’t know whether that would be controversial.
But all the safeguards of the confidentiality it is ensured. Perfect, perfect. I’ll come to more of this shortly. But we’re long overdue to talk to Christophe and Simon, about their amazing project, which is a very good working example of experimental statistics in action.
I’ll ask Christophe to tell how he and his team collaborated with Airbnb and other huge private companies that typically, Eurostat don’t deal with on a day to day basis. Christophe! It took a while with lots of trial and error, which is probably part of producing experimental statistics, as you pointed out, it’s not easy to get in touch with them. I mean, there’s not like an info@airbnb.com address where you can send your data requests. After some attempts in the end it worked out with the help of our colleagues in DG GROW, the colleagues in Brussels who work on industrial policy, single market and so on, because they have contacts with the platforms for other reasons. With their help, we managed to get in touch with the right people. Before it was basically Eurostat data freaks talking with data freaks in the platform.
And we all like data, and it was all nice. But when it comes to sharing data, you need other people to be involved. For us, it was amazing. On the side of the platforms, I mean, there’s investor relations departments who are very sensitive to sharing data.
Everything they send to us is to be published only after their financial reporting, contracts people, legal people. So it was a whole battery of people going beyond what we can do in terms of, I mean, knowing the data and the methodologies. But in the end, it worked out. And I mean, there seems to be a win-win for us.
And for them to share the data. I think they’re happy that their segment is now better represented in official data. Also, that is an official source compared to some of the other sources I mentioned earlier on, they’re happy to show that they are cooperating with public authorities. This is how this is what keeps us going for the time being. You’re asking them to supply you with bookings, and footfall and page impressions and all of that kind of thing. But, to what extent were they going to give you the metadata, the actual kind of detail of how they got hold of this information? Exactly how reliable it is.
Because, normally, what you would expect from your national offices, when you have the people you normally work with, you get an awful lot of detailed information about the information. Did you get that from Airbnb and booking.com? We’re getting metadata information. But of course, if you compare it to the kind of information we get from the national statistical institutes, in other data streams then, it’s, of course, it’s more limited. That may also be one of the reasons why this is labelled as experimental. I mean, the main problem for us was to get the data and to get it out.
And we also collect the metadata on our end. So information about, for example, how they attribute stays to a certain month, if they overlap, if they go, I don’t know, if there’s a stay that goes from the end of May to the beginning of June, do you then add the stay to May? Or do you add it to June? Or do you split it? Or how do you do that? Which kinds of listings do you add to your dataset? For example, I don’t know you could think about houseboats or tree houses? Are they included in the dataset? These kinds of information we collect from them and they tell us. Yes, but of course, in official statistics, I would say this was much more rigorous.
How did you find culturally your ability to work with organizations which are in the private sector, driven by market forces and so on that totally different to Eurostat. What was the kind of cultural match? Like, do they do they speak your language? Did you find it easy to kind of communicate what you needed and to get into a useful dialogue, because they’re, they’re quite different organizations - Eurostat and Airbnb and Expedia and so on. I remember one anecdote from the early days when we were talking about statistical disclosure controls. So basically saying, if you’re in a cell or in a geographical area where the data that you’re collecting where the numbers are very small, and you risk that individuals are sort of detected by that because if it’s just I don’t know three nights and you know, a guy that offers an apartment in that area, then you know, these three nights must have been at this place, right. So usually, what’s done is that if the number is under a certain threshold, then the cell or the observation is not published.
So it’s kind of marked as confidential. We’ve had a big meeting with representatives from all four platforms. And we wanted to discuss our approach here, how we do this, this statistical disclosure control. And we came prepared, of course, we had this big paper, this big plan, how we would do this lots of individual steps, et cetera, et cetera. And we were starting to present this, but then at some point, they said: Yeah, I mean, that’s nice and all but why don’t we just wait until we did our quarterly reporting. And then I don’t know we give you another six weeks or so.
And then you just publish whatever you want to publish, then we don’t have to do with all these fuzzy calculations. We don’t care really then. So I think that story really shows that there’s a different mentality going on there’s. Christopher’s it’s a novelty.
I mean, do you feel that you’re going into territory, which normally, public bodies don’t do? And if so, do you think there’ll be more of this kind of thing? Because it’s clearly been a success so far, hasn’t it? Yeah, I mean, we’re publishing data. So that’s probably the best indicator that the project actually works and produces what it’s intended to produce. For a long time statistical offices have been using or reusing administrative data from social security organizations to produce earnings statistics, for instance, the last year the reuse of privately held data has become a hot topic, just because there’s a few of these companies out there who sit on a goldmine of data, that’s, of course, relevant for official statistics.
Our small recommendation platforms project is an example. But there’s many other things and I think this is basically one of the things that Albrecht’s unit is, is working on. And our project and tourism statistics was is basically a proof of concept or use case. So maybe Albrecht wants to elaborate more on the, let’s say, the overall Eurostat or European statistical systems strategy in reusing privately held data. I think I do trust private owned companies. I mean, it depends on the company, for sure.
But also depends on the research behind it. And the company. I think if there was official research that’s backed up, and it’s, it looks legit. I’ve got no reason not to believe it, for sure, I reckon. Albrecht at Eurostat’s innovation unit. I’ve been meaning to ask: the private sector, it sits on zettabytes of data.
And you would love to get some of it, I presume that Eurostat would love to be able to enter into partnerships here to help public policy. What is the picture about the relationship between the Commission and big companies like that? We’ve done quite a number of experiments, for example getting data from online job advertisements, we have been in contact with mobile network operators to explore some mobile phone data. We have some examples of producing experimental statistics out of it.
But for others, we are far from it. So for example, with mobile phones, there is a big reluctance in working together with statistical offices. So far, the production of statistics is rather limited to some areas.
And it could be broader. Absolutely right, which brings us neatly, I think, to Denmark - back to Copenhagen and their smart meters. We left them dealing with this tsunami of data coming at them every 15 seconds.
That happened a few years ago, from 1 million smart meters suddenly, as a statistical office in, in Copenhagen, dealing with an enormous amount of information, and then having to work out what to do with it. The volumes of these data are enormous. So what we actually do is also that we aggregate it to be able to handle it, including ourselves.
So the first thing we do when we receive this data is that we aggregate it to manageable sizes. And then what happened is that we had access to these data just before the pandemic that was at a time where we were beginning to have these data deliveries. And then during the COVID period enterprises, they were allowed the possibility to delay their declarations and payments of VAT, and I’ll get to the smart meters in just a second. And normally here in statistics, we use the detailed VAT data as a key data source for our short term business statistics and for national accounts. So it’s a key data source for, for really key statistical information. Especially, we use the VAT data in the services sector, which was severely hit by the COVID lockdown.
Now then, with the absence of the VAT data, comes the smart meter data into question because we used the change in electricity consumption compared to the situation before the lockdown as a proxy for change in economic activity and the enterprises. In this way, we could ensure the continuity of the short term indicators which were becoming even more important than usually due to the pandemic’s high and abrupt impact on the economy. Now, after the pandemic, we now conduct various other experiments with this smart meter data.
One example is we look into, how we can use them for making statistics about the construction sector much more timely, because now we can immediately with the smart meter data, identify when smart meters are being installed at new building sites, and when electricity is beginning to be used at that spot. If I may mention just one more example. Most recently, we have entered a data sharing agreement with another private holder of data that is the business association for companies on the market for electric vehicles and their charging stations. Via this agreement, we will have data on the infrastructure for charging points.
And together with data on the electricity consumption, we can now deliver new insights on the transition of the Danish transport sector, from fossil fuel vehicles to electric vehicles. We use this smart meter information to produce some fun facts. During the year, for instance, we look at where people actually spend Christmas.
So we look at how the consumption of electricity changes from being usually very much in the big cities. And then we can see when people are moving to their second home, and now they start using more electricity there. So we can show a picture of where people actually are moving from and to, to spend Christmas. And we also have another piece of fun fact we use. We try to look at when people put the turkey in the oven.
We can see it from electricity consumption that it differs from different parts of the country when people start cooking for the Christmas dinner. Kirsten, can we also see whether people are having duck or turkey or roast pork? Eh, no, we’re working on that. Well, I suppose they have different cooking times. Don’t they? Experimental statistics of the length of cooking on a Christmas day to work out which animal is in the oven.
And then of course, you’ve got vegetarians to think about, all in the cities, I expect. So I’m quite sure that data is being used without my knowledge. Frequently.
I do not really know what kind of data but where I live, what I do where and when I use my bank account. Yeah, what I buy, things like that it’s probably used all the time, especially for, I know companies buy this kind of information to sell your products and so forth. But hopefully it will not be used to do anything harmful. I have to touch on, being a journalist, I’m always looking for controversy, so forgive me, and I know we’ve already touched on this, but there is a hotly debated topic isn’t there, about official, that is to say, government access to information about our private energy use, so to say, consumer energy use. Kirsten, do you find this causing concern? When people get to know what you can use this data for then you have to be very particular in explaining how it is actually used.
Because, as Soren said, we can look at a particular address and we can actually see when is the light turned on and turned off by looking at the electricity consumption. If we are not relied on as an institution to actually care about this data in a way so that we do not use this for playing big brother watching you, then you could run into problems. So that’s why it’s so important when we have access to this data that we use what we have learned about data and data security for many years, also to protect this data.
It’s not a new thing that we know controversial things about people. We’ve known about controversial things about people for years, because we have access to administrative data. So we know how to care about this data in a secure way.
So that’s very important to tell people that they are not being overlooked by big brother, because we have access to this data. We’re just producing statistics in a more efficient and less costly way. Albrecht, I have to ask you, the world of private data is enormous and getting bigger with big data and new technologies and so on and public statistical bodies have to move with the times, they have to stay ahead of the game. They’re situated within enormous institutional frameworks, which don’t move very fast, let’s be honest, they can when they have to, COVID is a perfect example, but most of the time, they evolve quite slowly, I mean, is this a problem? Our experimentation showed that there are huge potentials outside: COVID, the crisis also showed that we are able to use this potential for creating statistics. We are working together with the member states to create this critical mass of introducing new statistics.
At the same time, we are also working on the legislation to improve the situation. We are feeling the support then, of the heads of the statistical offices, and we also have the demand of the users for providing new statistics. All together we think that this creates the condition really for modernizing official statistics and then for succeeding, of getting these new data insights, the official statistics.
Albrecht, thank you and sadly, thank you all. Because our experimental episode must come to an end, we are out of time. The results of the experiment, peer reviewed with metadata, of course, will be pinned up on the Wrap Cafe noticeboard so do drop by to inspect them.
I’d just like to say a huge thanks to our contributors from Luxembourg Eurostat team tourism Christophe and Simon. Thanks for having us. The keepers of the flame, Agnieszka and Albrecht. It was a great pleasure. And our smart meter watchers in Copenhagen, Kirsten, and Soren at Statistics Denmark.
Thank you all. Thank you. If you’ve enjoyed the show, don’t forget to share with friends and colleagues where Stats in a Wrap can be found on Spotify, Apple, Google, and all the usual places. And if you’d like to know more about the subjects discussed today, just search Stats in a Wrap - Eurostat. And of course, join us for the next episode when the wrap cafe will be dishing up more flavoursome insights. This time about how Eurostat tracks and checks the government finances of all 27 members of the European Union, if you think that must be like well sorting apples from pears, and at the same time herding cats, you’d be wrong. It’s much, much more complicated than that.
Join us then but for now from all of us in the cafe - goodbye.
2023-05-23 12:15