New technology in blood test risk stratification
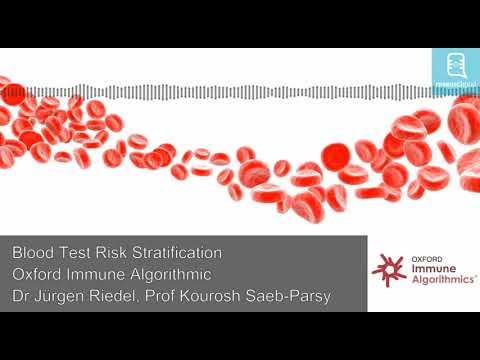
[Music] hello i'm well welcome to research pod you may have heard of the hippocratic oath a historic guide to medical ethics from which we draw the quote first do no harm you may not know the line further down the text i will remember that i do not treat a fever chart a cancerous growth but a sick human being today i'm talking with dr jurgen riedel and professor kiroshai parsi of oxford immune algorithmics about a more precise personalized and preventative approach for immune health monitoring when coupled with ai and machine learning technologies their work on proactive patient-centric approaches in medicine is part of a significant shift and managing health before it turns into illness [Music] i'm maliorgan riedel i'm the co-founder and cto of oxford new algorithmics we are a biomed company i am passionate about data in all formation information and certainly human health and beyond and garage hello hello my name is kurosai parsi i'm professor of transplantation at the university of cambridge and chief medical officer at oxford immune algorithmics we're talking about the work on blood screening and algosite it makes a lot of sense how you get to that from the immune algorithmic side of things coming from a biological background jurgen if we could hear a little bit more about how your experience and interest in data has led you to i'm going to be honest a little bit of a niche interest sure i mean i started as a computer software developer so i came into that world of data and discovered quite early that data is powerful driver in any kind of business i started working for investment banks private banks and that led me further to look into data more deeply so i think about data patterns to basically understand what is the essence of data for any kind of businesses and that led me to business intelligence look at databases so i became a database expert but it also led me to the point of how to visualize and make a story out of data now the other part of my passion about data is also because i'm a physicist by training and the physicist like to understand what are the underlying principles that govern the creation but also the uses of data so those combined brought me to this point of conversions which then led me to the application of not only data for businesses data and natural sciences but also in living organisms and life itself to understand what governs information in living structures how data and how information can help to improve people's lives using artificial intelligence system biology physics medicine to create precision medicine tools and garage i imagine it's something of the reverse for you coming from a very wet science to the dry and hard bioinformatics that we're talking about today that's right as a doctor i've always been fascinated by the way that we monitor our health status and how we diagnose disease and my interest here arose because it always seemed to me strange how we categorize patients blood results into a binary format where results are classified as normal versus abnormal except the reference ranges that we use for these categories are actually very broad so you can have very large changes in some of the lat test values and still remain within the normal range to me this is a very crude way of approaching the analysis of the data what makes more sense i think is to understand the baseline values for a given individual so that we can start detecting deviation from that baseline now if we're going to do that at large scale we will end up generating huge volumes of data and then the challenge becomes how do we acquire this data how do we analyze it how do we integrate it with other relevant data such as health status and clinical science and this is where the problem or the challenge has two facets one is the technology required to gain access to that data and then the other equally important and perhaps more difficult challenge is how do we analyze this data and this is where artificial intelligence and machine learning and the tools we are developing with oia coming [Music] they mentioned the idea of having deviations from the norm and a personalized approach to health kent personalized medicine being the big umbrella concept the utopian future of medicine that you are treated to return to the best that you can be in terms of any blood score metabolic function any disease state there's a lot of data and there's a lot of people so in terms of how we can put that across an entire lifetime as well the philosophy of biological markers over time just to kind of expand on what is normal versus disease versus just getting a bit older perhaps that's right so you're absolutely correct in that this idea of personalized medicine has been talked about for a long time but we don't really apply it to everyday delivery of health care what do i mean by that so let's take the medical test or diagnostic test or blood test that is most frequently performed in the world that's something called the full blood count so this is the blood test where a small sample of blood is taken and then we count the number of white cells in somebody's blood now this is the most commonly performed test worldwide several billion are performed every year so this is something that we should be thinking about well how do we personalize the analysis of the data we get here but we don't at the moment as we said earlier what we do is we categorize the results we get from a patient into this large reference values so an approach to personalization of that would be to say to understand what the number of a particular subset of white blood cells are in my blood let's take lymphocytes they're a type of white blood cell important in many conditions including cancer and fighting infection what we argue is that we need to understand what the number of those cells in my blood are and then be able to track them over time what that allows us to do or what that will allow us eventually to do is to understand when i start to deviate from that baseline and this may be an early marker of disease or it may be a marker of response to treatment or as you say it may be my marker of aging or some other physiological process that you're not even aware of right it may be to do with the seasons or maybe to do with the time of day but gathering this longitudinal data is at the core of personalizing our approach to this particular blood test and if we were going to personalize anything i would argue well why not start with the most commonly performed test worldwide right that would be a good starting point it's a test that's commonly performed it has utility in other words it has value clinicians use it they make decisions from it so this would be a good start important for personalization of approach to blood testing it's not the end of it but we believe it's a really important and good first step well i feel i'd be remiss if i want to mention that we're recording this in march 2022 what feels like kind of at the tail end of a global pandemic though numbers are rising and that's all based on people doing regular tests which i suppose is a very slim slice of personalized medicine in action if i could maybe just pick your brains about how the pandemic has fit into this worldview and how that's fitting into healthcare systems as well great point in the pandemic really does exemplify how our current approach while it has been effective is arguably not sustainable or at best inefficient so the way that we have dealt with a pandemic a series of measures but we know that vaccination has been really important has had a very beneficial impact but naturally the way we had to respond to it was to say well let's vaccinate everybody as many times as is required and we did that in the uk and in other countries whereby everybody had a first dose then everybody had a second dose a third dose and so on the problem with this approach is that it is not sustainable if we have further waves of either this virus or others the idea that we take a population of 60 million people in the uk and actually we vaccinate everybody every few months it's both economically and logistically a very difficult task we know that different people respond differently to either the virus or the vaccination so a personalized approach would be to say well we need to understand how a person's immune response evolves to being vaccinated or to having the virus once we understand that we will be able to distinguish who requires for example another dose of a vaccine booster or who is at risk of contracting the virus or at risk of actually having clinical symptoms from it so a personal approach to this would be to say rather than vaccinating everybody every three months we do regular longitudinal monitoring of the immune system we understand how for example the levels of the antibodies that are generated after vaccination on the level of lymphocytes white blood cells to the vaccine changes with time once we've collected some of that data then we will understand better who needs repeat vaccination most or who is at greater risk so the idea here again is not to treat every member of the population exactly the same but starts to delve into the detail of who is at risk who needs particular or additional protection now this concept actually in the context of viruses is not new we've been doing that for decades for things like hepatitis b vaccines so many people particularly clinicians who are exposed to the virus potentially are vaccinated against hepatitis b what we don't do is to say well every clinician needs to be vaccinated every several years right what we say is once you've had two doses of vaccine you get blood tests and if the levels of the antibody aren't high enough then you get a booster so the idea is to have the same approach to the pandemic but in order to do that we need to gather the data so that we understand what these thresholds are and this is the importance of generating data being able to track it and importantly being able to analyze it when thinking about whole body wellness there's immunity there's inflammation there's metabolic markers i suppose to give a kind of a brief overview of the human biome and various microbiomes they're in how can one estimate a decent well-being baseline for someone what's an out of the box human look like so human physiology is of course very complex we have different organs and different systems in our body the immune system is one of them but we have the cardiovascular system which is the heart and the vasculature respiratory system the lungs we have the renal system so the kidneys the liver and so on and of course these are all interconnected and they interact with each other so to get a really detailed picture of the human health or disease we would need to capture data on all of these systems naturally that's a complex task and we have to start somewhere now our proposition is that the immune system is a good place to start the reason for that is there is now increasing evidence that the immune system actually contributes to and impacts the well-being and the function of all other organ systems in the human body of course we've long known that the immune system is important for infections right responding to viruses or bacteria we've over the last few decades began to understand the role of the immune system in either the emergence of cancers but also the response to cancers but there's not good evidence that the immune system is also important in our susceptibility to other diseases that historically were not thought to be immune mediated so these are things like heart disease or mental disorders depression diabetes and so on what's more we now recognize that the immune system is also important in normal daily function things like sleep or mental performance or physical performance or recovery from injury or exercise so actually the immune system has an integral role to play in a variety of different conditions so from that perspective again if we were starting somewhere and we said look we want to generate comprehensive longitudinal data starting with one system in the human physiology we feel that the immune system is a good place to start that doesn't mean that it is not important to measure blood tests that tell us about kidney function or liver function but it just means that if we're going to start somewhere we think the immune system is a good place to start and the immune system is also particularly complex it has many different components of different types different cells and different cell types that have different functions different soluble factors so different small molecules or different proteins so its complexity also means that it lends itself well to analysis using a sophisticated approach which is why again it becomes a good target for applying artificial intelligence machine learning and advanced bioinformatics tool to understand the immune system and its implications for both health and disease which i suppose is where jurgen your experience and fascination and mastery of all things the data comes in that's correct and i think i just wanted to continue what has elaborated that the immune system is a complex system right i mean the whole notion of complex system is not new i mean we know whether it's a complex system right like a flock of birds they are complex a school of fish they're complex systems right but yet you know beside all the complexity which we know exists especially of human body is it almost like the prime sample of complexity if you include the brain right the notion of using data also has the idea of there are underlying rules which govern data or generating the data we observe right and so that's basically the approach we also utilize if we talk about machine learning and that is one way to look at it in the statistical point of view so you sample a lot of states of the system which is like measurable so blood tests different tests even like sensory input and so on but then on the other hand you also then have the notion of simple patterns you discover in those complex data sets right which are statistically derivable you can have inference and say okay if i see that kind of pattern then i would say the other and if i observe pattern a i would observe pattern b in a given time frame afterwards right now that doesn't really tell you much about the data and underlying principle why the data is generated so if you look a little further and think about ai not a statistical tool i mean the eyes by the way is a universe of different disciplines and one approach is what we are pursuing is looking at generating models of data right so we're looking at data and basically look at what kind of sort of structures or principle can generate the data it does explain it and therefore we have sort of a causal relationship between events occurring during a period of time and that's certainly also very important if you look at the immune system because if you want to say you observe a spike of white blood cells over a given time frame you certainly would like to know if that spike appears again or when it dies down but also you would like to know what the underlying cause right because that's really if you know the cause and you can prevent all the other spikes in the future right you don't have to wait until they come forth right so that's the idea we pursue and i think that's very where we argument the existing machine learning with our causal ai which we call it on eurosymbolic ai and basically mine those structures which explains the data and therefore give us a more holistic view of a patient or a user and so the idea also that is underneath is that having that model we can also create a baseline and we talked about it because the baseline precision medicine what does it really mean it means that we create like an individual model of a person right and we know persons are not the same and we accept it in many other areas like in social settings and political settings we accept it in even in education nowadays which is also was a long struggle but in medicine we are sort of struggling with that notion that personalization is important i mean that is what we want to change and but that is strange in lots of ways because that's like for us i would say the most important essence we have a good life good health but yet we try to generalize it population-wise and so on that's where again the fascination of information and data really merged together within need of personalized medicine and also for improved health this is about a fundamental change in our mindset in terms of gathering data our approach to health care historically and this is true for centuries if not decades is to only gather data when things go wrong right so the process of gathering data starts when a patient presents with a problem with a medical problem and we say okay what what information do we have let's do all these blood tests well actually we should be gathering data long before these events occur that will allow us to better understand what it is to be healthy we can then hopefully start to detect deviations from that healthy baseline sooner rather than having to wait until a patient actually has symptoms people will say well there's no need to measure this particular blood test because this patient is healthy well we would challenge that and we'd say well they may be healthy in that they don't have any overt clinical symptoms but unless we gather this data we won't really start to understand at what point some of these parameters start to change before they present those symptoms or we shouldn't really measure this because we don't know what it means well to me that is precisely the reason why we should be measuring it if we don't know what it means we will never know what it means unless we start measuring it and we start correlating it with things that we do understand like clinical symptoms now of course this has to be done in a responsible manner we need to be careful that the data is managed appropriately the data is secure people's confidentiality isn't breached and also that people aren't exploited through the process of donating their data you know whether that's financial exploitation or otherwise so we have a responsible approach to this but our fundamental principle is that we need to gather this data not just during disease but also during health in order to be able to make diagnosis earlier and to guide management of disease more effective prevention being better than cure even down at the molecular level absolutely [Music] to bring all of this to a very specific example the paper that was published recently on blood test risk stratification to summarize how one gets from a fluid sample to data to analysis to color scoring maybe hand off between you as that literal handoff of sample works that sounds like a good approach i can start with the concept of what we're trying to achieve that when we are monitoring different types of data it can be difficult to really follow all these trends and be able to assimilate a message from these trends in a way that is simple to understand and has utility if we were to you know present a clinician with 10 000 different data points and say look you just look at all those things and process them in your mind and come to a solution well that's not impossible but it is a challenge the idea is for us to combine different data or even data sets into an easily understandable signal that can help a clinician manage their patients better we already do this in different concepts so we have for example something like an early warning score the idea with an early morning score is that it's a collection of physiological parameters from a patient when put together just gives a signal to a clinician or to an observer whether this patient is getting better or worse this has been done now for many years for monitoring things like blood pressure temperature and heart rate the idea is that if you were to monitor each of these individually you put them together to give you an early warning score and if they're going in the wrong direction right if they're deviating from normal towards abnormal that means your patient is getting worse this is a red flag that you should be attending to this patient whereas if they're going in the right direction they're getting better well your patient is responding to treatment so that's the concept of creating a score except that type of approach has not previously been applied to blood results and actually blood results are much more complex because there's more of them different blood tests are done performed in different contexts so what we've done is we have applied this concept to collection of different blood tests think of these as a basket of blood tests where we are combining the blood results that fall into a basket because they're related to each other and then quantifying how these blood results both individually but also as a set how they deviate from first of all what the population reference values are how they deviate from that person's individual or personalized reference values and also how they change over time so by monitoring this deviation over time we can get a feel for whether this patient is getting better or worse or we could triage patients based on who needs most attention so that's the concept of creating a score and we've done that for different baskets of blood tests so we've done them for example for all the components of a full blood count also known as a complete blood count which has the red cells and the white cells in it and this gives us something called an immune scope but in principle we can do this and we do this for for example blood tests that are related to monitoring the health of the kidneys or the health of the liver so that's what the concept of a score is it's a tool that brings together information in a sophisticated manner but actually makes the information then more accessible to clinicians and it becomes a tool for the clinician to use to manage their patients more effectively so the idea is to take the baskets and the analyte species that those are the subunits of the tests that is like the number of white blood cells red blood cells different type of blood cells and so on and then we basically take the data and transform it into an immune space we call it so where we basically map the data which have different units you know i mean the accounts sometimes they are like a concentration and map them into a unified immune space it's like an abstract mathematical space and then basically apply the analysis how much each of the data points delayed from the mean we start with the population meters course explained and basically give the deviation sort of a value and this value in total if the whole basket is one number can basically be a number between 0 and 10 right so that's where basically zero is it's not good and 10 is very good your smack on you know the standard right now that certainly is not true because there are no standard humans right therefore what we do and we observe them data over a period of time so we collect different samples over a period of time we look at longitudinal data and basically adapt from that population base reference values which is huge to a personalized the sub range if you will so basically if you think about a band from min or maximum and you look at your data points you will fluctuate not between those absolute population minimum maxima but in a more narrow band right you may be on the upper or lower end of the population band but your band is much smaller and you fluctuate ideally only very minimal and that's true for the immune system because the immune system is precisely there to buffer all the tubular things happen in the body and keep the body like can even heal right so that's the whole idea about the new system so if there are any kind of perturbations they are mostly dealt with but if they are too big you know the immune system somehow caves in and has to react more dramatically and that we would see in the slight deviation from the mean and basically what we do we calculate the the mean for the individual band not the population band therefore we are much more tuned into what is really a real deviation of the person's baseline and if that is happening we flag it and we have like a color code for the green everything is good yellow is your above one standard deviation or within one sun division of the absolute minimum maximum of your band and then red is if you go beyond right and that again is captured into one number and we also have the color bandwidth gives you an indicator also from one which direction you're going from red to yellow and green or you move away from green to red and that is very important information for physicians because they can try ash fishes much more efficiently faster and don't have to juggle with so many different plots and diagrams and i think the danger is also that lots of tendencies are overlooked because somebody you have a tunnel view you only look at certain analytes because it makes sense maybe the patient has certain conditions but overlooked a totally different range of analytes which might be very important for that use case so that's basically what we do with the paper we describe the method how we do it how we set up this score space how we calculate the score and so on but all of that process of taking all of that data all of those data points and coming up with green good red bad how much time does that take to kind of put that into clinical process of patient is admitted to hospital gets blood taken within maybe the first four hours how quickly can someone have some actionable data coming out of that so the good thing about our score is that it can be generated as quickly as the blood results are available now generating one score is valuable but the real value comes from tracking this score right so that whether you can see that it's getting better or worse by that i mean it's going towards normality or away from normality so that's why again the longitudinal monitoring is important but there is also a way of using the score as a tool to triage patients what i mean by that is imagine that you're seeing a hundred patients which sometimes can happen in the clinic and then you've got a set of blood results for 100 patients and you're thinking well which one should i start with in terms of reviewing the data well at the moment you either do them alphabetically or by random selection or based on the time that you saw them in clinic another way of doing it if a score was automatically generated for every patient so well i'll start with the ones whose blood results are most abnormal or the score is worst if you like and that means that you can focus on those patients first review their blood results and then gradually move towards the ones that have more normal blood results so those are sort of two applications of either an individual score or at score as it evolves over a period of time another type of use of this score is that actually as a clinician eventually you can create your own basket of blood cells let's say that for my patients i'm really interested in what happens to their let's say liver function test as well as a subset of their white blood cells and maybe their hemoglobin right actually you can create a basket that has those components in it for yourself for your patients and then you can monitor that so there is flexibility in the system of course over a period of time the idea is to validate clinically the utility of these scores what it means is when you track them over a period of time and then they start correlating with how patients respond to treatment or how their condition evolves then that allows us to modify the score improve it and make it more useful exactly how it was done with the early warning scores that we were talking about earlier so those have evolved over a period of time if you had a basket that had three different components in them so let's say it was heart rate blood pressure and temperature well you could give these equal weight right you could say look actually they contribute a third to my score each or you could say actually it looks like temperature is really important right so let's give two points to temperature and 1.2 blood pressure and heart rate or vice versa and the weighting and the way you combine these could evolve over time or you could say look actually maybe we should add you know another component to the score which is let's say level of pay so these scores are designed to evolve over time and be refined and optimized and actually the algorithms over time may be able to do that right it may be that actually the algorithms can be designed in a manner that they learn they corporate data and say like actually you know this component in the basket doesn't really seem to correlate much with a health outcome so we can reduce its priority or you can remove it from the basket but this other parameter is important thinking forwards where do you seek the future for that branching out possibly bringing it out into society thinking of long-term disease some kind of life-limiting condition where they are doing that monitoring already and that you have perhaps something to action more readily out of that when you can turn up with a very simple score of the doctors and say i know for a fact that my potassium is off or something i've taking that to a lifestyle approach towards health rather than just an incidence base that's exactly right it's about being proactive in measuring health and generating data and monitoring that data rather than reactive and actually we normally not only are we reactive we actually we react very late our trigger for a reaction is normally a health event right it's a bit like you know you start looking at the stable door once we find that all the horses are missing right whereas actually what we should be doing and saying well actually let's have a system where we look at the hinges and the locks on the door once a year and once we know that they're loosening we can do something about it before the horse is at fault the health mot i think i've heard it talked about i've heard about that for years and years and years but the phrase is out there that's right so the concept of doing that type of health assessment on a regular basis has been around for a long time but actually the concepts now need to be actioned right that means that we need to create mechanisms for generating and gathering the data and then mechanisms for analyzing all of this data right there's no point doing blood tests all the time if this information is never integrated gets lost nobody analyzes it then that has no utility so this is where oxford immune algorithmics is making an impact is to create those strategies in the infrastructure first of all to generate the data itself through some of our point-of-care blood testing technologies that we're creating generating the platform where all of the data can be hosted in a secure way and then the critically important algorithms and artificial intelligence and machine learning approaches to analyze a data machine learning and artificial intelligence has been presented as a developing concern for the future through various opportunities and various gun-wielding robots so could maybe press jurgen for a little bit of your thought on the near future or far future of how that comes together in terms of having personal models for health but then just like the sheer volume of data that's going to be rendered for the next couple of generations of people who will have this as an opportunity from birth over the course of their life this machine learning ai you may not need to look in the far future 10 years already is a giant leap for imagination but i think they are like as you said i mean yesterday uh opportunities but also negative size obviously and those information on knowledge can be misused by creating evil machines which is not help but control people and basically come back to the whole system that other few people control the masses right now we argue that we certainly acknowledge it but also we see the other side of the potential precisely that can help mankind to improve health that helps and not a good health system creates lots of issues and i think that's where we would see that the ai can really make a fundamental difference so that the planet to really use of data and also to connect different data points diseases so networks and see like a holistic picture which is like population and global but also extremely individualized very laser focused and that's our hope to improve the way we actually looking at wellness health to sustain a better life and also to create new opportunities for mankind if you will and well the hope is that that really eradicates most of the issues and we want to eradicate all of it but if you exaggerate that that can be a brighter future for us and the other chances really also having lots of data is also to really deal with it in the responsible way how we eliminate bias how we actually may not need that many data but learn how to use little but have better data i think that's also another point i mean we certainly need to also improve the way we collect data that is also a very important part of improving ai and to really build proper models about our world and help us to improve rather than creating like giant bias machines you can actually think about those large language models which are now very popular who actually can answer you all kind of questions but they are certainly i mean they create art they create programs but they are just language models which are extremely biased the way the social interactions are set up so we need to really take that not as a blueprint but you have to go to the direction where we curate data and i think really look at data responsibility and i think that would be a new domain to conquer i think we are going away from this hunter gathering of data to really creating a farming you can almost basically think about data farms you go from uncurated to creative data to data plants into really have data mines to really understand and prepare data so that can be used responsible ai purposes so i would say that is like a challenge we have to do in the next years to pose a final challenge i recently moved house i moved from one city within central england to another the mismatch between the health systems in one city and another has caused no end of problems and that's through systems compensated through a national health service i am not having to pay for going to doctors to make these appointments to get kidney tests and had kidney stone it was horrible kind of embedding this into a sociological approach to health in a society level approach unifying health systems and accounting for costs and access across even a fairly modern society such as in england where there is still a large inequity of access to healthcare of access to the facilities that enable people to seek healthcare things like child care or paid time off work where does the whole discussion that we've had so far fit within what needs to transform in a society in a health care system in a public health care model that lots of the world still doesn't have access to the inequality and access to healthcare is very apparent across the world and actually that drives some of our attempts and inspirations for developing new technologies we talk about democratizing our access to healthcare and the way we fulfill that promise is to say can we create technologies that allow people to have access to for example blood tests remotely without needing complicated expensive machines and laboratory equipment can it be done at the point of care in a village in remote rural areas so we are trying to address those challenges by creating some of those technologies and then in terms of the data analytics again our systems are designed to be based in the cloud so that actually anybody from any part of the world as long as they have access to internet then they can utilize these analytical tools and it doesn't require very expensive purchases this is very different to some of the other solutions that have been generated so electronic medical record systems that sometimes cost hospitals tens if not hundreds of millions of hundred dollars so again our approach is to democratize access both to the technologies that help generate the data but also the technologies that help analyze that data and our systems are designed so that they can be integrated into other existing laboratory data systems yeah i think that's really also for us very important to make the technology for everybody i think we actually develop technology which is actually utilizing low end technology that is feasible and that is affordable but very important and i think that's very busy at the we are not at the edge of latest technology but we are edge of converging existing technology to penetrate areas or markets which would be very difficult to penetrate with high cost machines which the health care system is basically saturated with this humongous machines centralized labs you know where there are only a few people have to excellent healthcare excellent machines outer worldly machines but 99 doesn't right and i think that's a very huge challenge i mean even think about fresh water this is a huge issue right so think about if there would be machines distributed just to create fresh water right now the drinking water that would change the landscape some similar we want to do with access to precision healthcare mainly through the use of blood tests which are very important any kind of i mean especially malaria for instance it's so huge issues still and any other kind of avoidable diseases if you will but extremely disturbing for many developing regions that can be addressed and also be eliminated right and i think that's what's for us i really also was a driving force i mean certainly you can add machines to existing super high tech health care systems but i think we see the potential on the other on the 99 as well right where we can make a global impact is there anything more immediate that you happen to be working on any new applications any upcoming publications as well that if anyone wants to see what is next for algosite or oxford immune algorithmics that they can keep an eye on that might be coming up anything on the horizon so one of the things that we're continuously working on is to use existing clinical and health data to validate our protein algorithms so we've been getting access to anonymized historical data from different sources and one of the things that we will be working on in due course is to use that historical data some of which is longitudinal to generate insights into the things that we talked about early diagnosis response to treatment and of course optimize our approach and our scores and ensure that they correlate with clinical endpoints that are of importance for patients and for clinicians the other direction is to i mean we talked about the immune system so that we explore the new system through the most common blood tests but the other next direction is also vertical i mean look deeper into the immune system look at the differentiation the new system the sub systems if you will and basically then go the horizontal way again and basically create new scores new site connect the different scores to gain more insight into the immune system and also to create links to multiple diseases which i think they are still very unknowns and for instance the other dimension i think we briefly mentioned is a microbiomic looking at the body as as a collection of entities which is based on our cells but there are a host of other single organisms inside our body which are very fundamentally involved in connected immune system which potentially is very much an integral for awareness and also to connect that and expand our understanding of the immune universe i think it's important that we make health care a really individual issue it is arguably a fundamental right to be healthy right i think that is something the empowerment we see in it and i think that's the dimension we may also be aware on is that we go away from large health systems which have like old networks old approaches outdated approaches and basically go towards a more individualized health care access and also the emphasis from seek to health care and i think that is sort of in conjunction with all the technology advances we all assume ai will bring us but we need also to change systems with it that's really the most fundamental aspect of it and i think with together that is a very important aspect of our new future we need to gather as much longitudinal data in a responsible manner so that we understand health better and can prevent and treat diseases more effectively [Music]
2022-06-20 10:48