Erez Dagan: The Key Technologies for Unlocking AVs at Scale
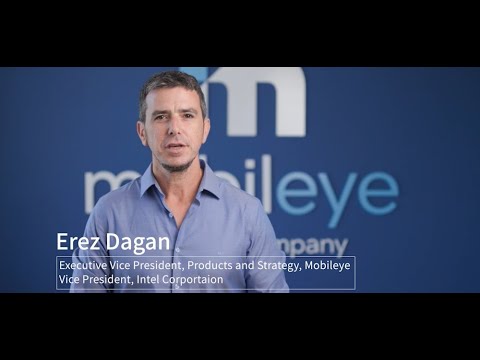
Thank you for the opportunity to speak at the Car of the Future summit by Reuters. In my short talk today I will share with you what we at Mobileye identify as the core technological enablers of autonomous driving at scale. At Mobileye we have been harnessing computer vision and machine learning to promote driver assistance and road safety since 1999. Today our three major business pillars are ADAS, the bread and butter, crowdsourced AV mapping, which targets autonomous driving, driver assistance, and smart cities markets and our self-driving full-stack solution targeting the consumer AV and mobility as a service markets. The combination of all three cortec engines is imperative to unleashing AVs at scale. The first
element is the RSS formal safety model. It's an explicit digitally interpretable and enforceable model for the safety of the decision making of the autonomous vehicle. The second principle is the realization that the AV-ADAS divide is not scope of capabilities, not a different scope of capabilities but rather the mean time between failures in executing those capabilities. We are relying on what we call True Redundancy™ - truly redundant perception subsystems which perceive the environment in parallel to one another and allowing us to both perceive the environment with low failure rate as well as prove that our system has lower failure rate of that of a human driver which allows us in turn to take the driver into a eyes off mind off position. The third element is enabling or unleashing seamless geographical scalability. Autonomous driving has to be deployable
anywhere and in order to make that a reality we've designed a crowdsourced mapping solution which utilizes the plethora of driver assistance cameras that are traveling out there to harvest information and build automatically high refresh rate AV maps to cater for that interest of seamless geographical scalability of the autonomous driving system. What do we mean by True Redundancy? Having two perception subsystems, one comprised of cameras only, and the other one comprised of radar and lidar sensors only, both creating a comprehensive view of the environment covering the four elements that constitute the environment the road users the road boundaries the road geometry and the road semantics. Being able to do that independently in two subsystems gives rise to both the very low failure rate or high mean time between failure that our system yields as well as to the provability of our systems - our ability to prove that our system is exceeding the capabilities of a human driver in terms of a failure rate. Having
two independent subsystems proven on order of magnitude of 10,000 hours each would give rise to a proven safety case of the perception system, of the overall perception systems which is the square around 100 million hours of driving. Coming to design a computer vision subsystem which meantime between failures is at the order of 10,000 hours is not a trivial undertaking. It entails a combination of several standalone mutually informative computer vision cores.
One of these cores is the vidar which produces a high angular resolution and high accuracy depth perception around the vehicle utilizing the multiple cameras placed around the vehicles and their overlap regions. This standalone computer vision engine is of course independent of the more classical pattern recognition methods that we deploy in the surrounding of the vehicle which perceive the vehicles based on the objects of the environment based on their appearance rather than their depth. Another such standalone comprehensive perception engine is designed to directly yield a semantically explicit representation of the host surroundings. As you could see in this slide,
it outputs a top view of the environment road users, road boundaries and even the velocity of vehicles around the host. The resulting computer vision subsystem is actually a product of itself. This is the SuperVision™ product which we designate as a premium driver assistance solution. It is a stand-alone end-to-end stack comprised of computer vision REM™ and mobilized driving policy in RSS layers. The Road Experience Management technology or REM is mobilized crowdsourced AV mapping technology which is designed to seamlessly enable AV everywhere. The technology is comprised of three core stages - the harvesting, the aggregation
and the localization. By harvesting we refer to the process of perceiving the environment through single camera equipped vehicles or driver assistance vehicles, identifying the road geometry and road semantics around us in a way that allows us to transmit with very low bandwidth this information up to the cloud. We interpret the road geometry and road semantics out of the video stream, pack it in small packets of 10 kilobytes per kilometer and shoot them out to the cloud for aggregation of a map. The second stage, the aggregation of the map is taking these snippets of information turning them into a coherent map of the environment - AV map, which contains the road geometry and road semantics with a very high level of accuracy as well as landmarks which will then be used to localize the vehicle within the map, which brings us to the third stage which is localization. An autonomous vehicle or a premium ADAS vehicle such as the SuperVision system
would consume the RoadBook by pulling it from the cloud, identifying landmarks in its vicinity, localizing itself inside of the RoadBook and then gaining that electronic horizon with rich semantics that the AV map has to offer. Having the crowdsourcing technology rely on camera only agents is critical - it's critical for the scalability that we just mentioned but it is done while not compromising on the accuracy. The accuracy of the map is actionable we can drive a vehicle, we can demonstratively drive a vehicle based on the on this map. The road geometry nuances as well as the semantic nuances are well captured to provide a full view of the electronic horizon as it's called. The map is not only accurate - it entails rich semantic layers which leverages both explicit and implicit cues captured by the crowd which allows us to generalize to the driving cultures and traffic rules across the globe. Such semantic information
for example could be the common driving speed, the stopping points within a junction through which human agents are investigating the junction without taking unnecessary risks and could also be the association of different traffic lights to different lanes. Our large harvesting fleet today allows us to harvest 0.7 billion kilometers of road globally, 8 million kilometers of road covered daily. By 2024 based on the set of agreements that we have with our partners we foresee one billion kilometers of road to be covered on a daily basis. Truly redundant
perception system in combination with the AV maps yield a robust model of the environment, covering the road users the road geometry the road boundaries and road semantics. A faithful model of the environment is not sufficient and to safeguard the vehicle decision making from causing an accident we have formulated RSS - an explicit model for a road user's duty of care. Beyond complying with the RSS contract in our own systems we are driving its standardization across the globe. The resulting robustness, geographical scalability, safety and agility of our AV system is not theoretical. It is clearly demonstrated through
our AV deployments in multiple cities across the globe, starting in Israel, Munich, Detroit and shortly upcoming in Tokyo, Shanghai, Paris and New York. Thank you all for your time today. Look for our AVs on the streets and feel free to reach out to continue the conversation. Thanks Erez for that presentation that was brilliant if may I ask - how do you envision the arrival of autonomous vehicles playing out in other words how long until we will be able to actually ride in or purchase a fully autonomous vehicle and what needs to happen before that dream comes true? Great question so there are two streams that are going to bring about autonomy to the end users - first one is robotaxis where a user simply can ride an autonomous vehicle. We are planning to launch such a service already by 2022 next year and we see the industry probably opening up in a larger scale for robotaxis in around 23 24 time frames.
The important next phase of autonomy which already has some sprouts is the introduction of consumer autonomous vehicles. Right now it's consumer autonomy in certain restricted function restrictions or OBD restrictions such as on highways or traffic jams and ultimately this will get us point to point autonomously. Now as for the question of what needs to happen to make that a mass-market reality - of course there are several factors I think primarily I would denote the regulatory discussion that needs to take place, I think I also regarded it in my talk - as for the RSS that contract between the autonomous vehicle and the society that's using the street along with that autonomous vehicle - there has to be a very clear interpretable contract of what is a safe negotiation of road users. Right now this contract is very vague in many ways. It's common practice of humans but expecting computers to take part in that
contract we really need to formalize it and make it something that that the vehicles can rigorously follow to have that market acceptance and all of the safety and efficiency benefits that autonomy can bring in. I think the second challenge that we need to overcome is a geographical scalability - how do we introduce autonomy everywhere not just in the pre-designated geo-fenced areas or roads. Okay, and for instance, while the world waits for AVs to arrive, are there other ways in which the industry and the public could benefit now or in the near future from the technologies being developed for self-driving vehicles? Most certainly - actually Mobileye takes pride in the fact that we trickle down - a lot of the value that we produce while developing the autonomous driving technologies we trickle them down into our driver assistance proposition. For example, our mapping was originally intended to cater only for autonomous vehicles and today is already marketed as part of premium driver assistance systems, and even the RSS model that I talked about which is that contract between the vehicle and the other road users is already being deployed as part of a driver assistance system, so imagine a world where this contract that the autonomous vehicle that was developed originally for autonomous vehicles can now safeguard the conduct of human drivers so a human is fully in control but there is a very clear notion of what is the boundary line between assertive driving and dangerous driving and the vehicle can inhibit certain actions to make sure that the human driver does not overstep and violate that important safety contract, so that's another example.
There are many other examples that concern our perception systems that evolve in giant leaps towards autonomous driving and these values were migrated into the driver assistance arena offering advanced functionalities such as animals detection, free space detection, the REM mapping that I mentioned earlier and then that safety contract of what actions are safe versus actions that are not safe on the most comprehensive sense. Okay, another thing, you mentioned REM, Mobileye seems to be taking a clean sheet approach to mapping for AVs. In what way do REM and the resulting Roadbook differ from other mapping solutions? Excellent, so first and foremost the fact that it is crowdsourced allows us to benefit from a fleet of millions of vehicles equipped with a single camera, no other sensing device, in order to continuously update the map. A map - critical attribute of a map - is that it has to faithfully reflect the reality. If there is a change or a construction in the road that happened five hours ago, the only way to get a map which is faithfully reflecting reality is to have a crowd-sourced approach to it, scanning the roads continuously and updating our understanding of the road structure. So that's the first element - the fact that it's crowdsourced allows that
high refresh rate and seamless geographical scalability anywhere that our driver driving assistance cameras are traveling is being mapped on the way. The second element of the map has to do with the second unique aspect of our mapping approach has to do with the semantics that we are extracting. When you are driving on the road there are explicit cues such as the road lines and the lane marks sorry or the pavement structure or the traffic signs next to you which are very explicit but there is implicit semantics that is very critical to the decisions while driving and I'll give a few examples - the association of which traffic light belongs to which of the lanes seems like a very simple assignment problem that humans solve very easily but when you really try to make a computer resolve that problem in real time you run into difficulties. What a map can do is encode this
information from the crowd that travels the road and understand which lane corresponds with which of the traffic directives - that's a very critical element. Another nice example is when a human is investigating a junction, entering a junction to investigate whether a vehicle is coming, there is a very specific pattern of how do we proceed into the junction while minimizing risks and maximizing our visibility and that pattern of the places in which human drivers are stopping within certain complex junctions is very valuable semantics that we can harvest from the crowd and then have very useful for the autonomous vehicle. So the approach we call them not HD maps but rather AV maps - this category of the crowdsourced rich semantic maps. Okay, but Erez, Mobileye is not the only company pursuing self-driving technologies, right, how does Mobileye's approach stand out from other well-known players in this field? What factors give Mobileye an edge over the competitors? So I won't repeat - I'll just denote the ones that are already mentioned so the REM mapping is one critical factor, the RSS which is the decision-making or driving policy contract of safe negotiation, that's a key factor that enables both the safety as well as the agility of our vehicles, so sitting in the vehicles you will experience a very human-like drive because the boundary line between assertive and dangerous is very clearly formulated and we can maximize the assertiveness and agility. Another factor which is critical and very very fundamental to our approach is the True Redundancy - the True Redundancy between having two perception systems completely independently perceiving the world and giving us both high robustness of our environment model, the environment model is correct to a higher degree and with a lower failure rate and in addition it gives us the ability to validate our perception system with a much more pragmatic approach since because we decouple the system to two independent systems we can validate each of these systems independently to a much lower validation criteria. If one system fails, each system fails every one
thousand hours, both systems will fail every one million hours. And that's a very strong, strong indicator of robustness and validation method. Okay, well thank you very much Erez, I really appreciate your time. Unfortunately, we've now run our time and I wish we had a bit more to carry on and now we're gonna go to a quick five minute break and we'll see to just to our last panel Boosting Safety, Integrating Sensors and Technology. Thank you very much Erez
2021-07-08 12:48