Social Computing for Social Good in Low-Resource Environments
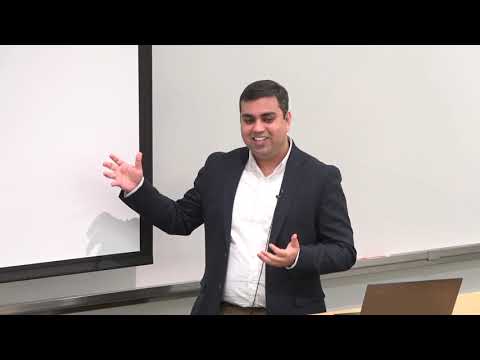
You. I think. A lot of you already know Adygea but he. Is a PhD, candidate, in the, computer, science and engineering, department. At the University, of Washington and he. Spent a lot of time at Microsoft, Research Bangalore. As, one of their associate. Researchers is that what they call it their assistant, researcher assistant, researcher so that's where kind. Of a pre PhD. Program where you spend a couple of years doing. Research at the lab and. So I did choose work in, particular focuses. On computing. In low. Resource, environments. Such. As India. And. His work has been recognized, with Facebook. Fellowship, and with best. Paper Awards, at KY and, assets, and. Of, course it's. Also had a lot of impact through, real deployments. That have reached, thousands. And thousands, of users. In India. And also Africa, I believe so, we're really excited to have Adygea, here speaking today for. People who are joining us online on, teams, we. Have the volume, in the room turned off right now so it doesn't create feedback, so if you have questions, during, the lecture itself on teams. Just type them in the chat window and I'll raise my hand and ask them for you and then at the end we'll also have Q&A and we'll turn the microphone four teams back on for the Q&A at the end. All. Right good morning everyone thank, you so much for coming to my talk today, I'm very excited to share my work on how, social computing can be used for social good in low resource environments. Social. Computing platforms, like these have, revolutionized, how most of us in this room reduce consume and share information, they. Play a pivotal role in connecting us and providing. Us with up-to-date, information. Despite. Their imperfections, these, platforms, are an integral part of our daily lives and have deeply impacted our society. But. These platforms, currently exclude billions, of people worldwide who are old who are low literate who speak, low source languages, who, live in severe poverty or those, who do not have smartphones and connectivity. To. Include these people in this computing, revolution most. Technology, companies, have primarily, focused on bringing connectivity. To load source environments. For. Example companies. Are using drones balloons, and unused TV spectrum, to improve connectivity and, although, these are great first steps just. Improving connectivity, is not enough because, there are several other technical, social and cultural barriers as an. @ CI researcher, who is motivated to solve these barriers I can, speak for hours about how, each of these barriers makes, it very difficult to connect people but. In the interest of time I'll briefly, highlight a few of them. Focusing. On India where have done a lot for my research a high. Majority of people there use feature phones now. Unlike smartphones, it's, very difficult to create a standardized, feature phone application, that, works on all feature phones because. There are hundreds, of proprietary, operating systems, most, without any API documentation or. Developer, support, now. Even if people have smartphones, literacy. And language barriers makes. It difficult for many to use them and just. As an, example. 26%. Of people in India are illiterate, and they find it challenging to use text-based interfaces. Using. Voice assistant, software's like Cortana, Google home Alex and CD are also very challenging because. Most Indian languages do not have recognition models, and training data in fact.
There Are some languages, which, have millions, of speakers but these languages, do not even have fonts, now. As technologists. Even, if we solve technical barriers to provide people's smartphones connectivity. And power, to charge their devices, social. Cultural norms impact, the systems that we build for example a high, majority of women in India are prevented, from using mobile phones and internet and these. Are just three barriers that have spoken about a combination. Of these barriers makes, it very difficult to connect people in low resource environments. And to. Make matters worse these. Environments, have marginal eret ease within marginality, x' which, means that some sections of society are, more, marginalised than others for example. 80%. Of persons with disabilities, live, in low resource environments. In developing. Regions. 90%. Of these children, do, not attend school which, means that they have no knowledge and skills for, employment well, being an overcoming poverty and high. Majority of women undergo. Physical, abuse and sexual abuse and, in. Addition to this social, cultural, physical. Psychological. Marginalization. People. With disabilities, are also technologically. Marginalized, because, they encounter, huge, accessibility. Usability, barriers, in using, smartphones and internet platform. My. Research goal is to build social computing technologies, that, are accessible and inclusive to all people, I am, motivated to bring the benefits of social computing to, people with disabilities, and also to billions, of those who are excluded because, of literacy, language socioeconomic, and, connectivity barriers, in, particular. I am driven to connect them provide. Them information and, bring, social and digital equity to them, towards. These goals I have used a broad spectrum of interdisciplinary. Methods from computer, science HCI. Design, accessibility. Social, Sciences and behavioral psychology, and using. These methods I have built, nine social computing systems, for, people in low resource environments. I have. Systematized, how these new users produce, consume, and share information by. Using quantitative. And qualitative methods and, have. Deployed these social computing systems, in real-world settings by, partnering with governmental, agencies, social enterprises.
And Grassroots. Organizations. And together. These, systems have reached an estimated. 220,000, people in low resource environments. So. Let's find out what are these social computing systems. To. Connect people in low resource environments. I have, designed and built voice, based social computing services, by, using interactive, voice response technology. IVR. Technology, is what you use when you call your bank or insurance service provider and, press, when to access your account information or press - to speak to a customer service representative. The. Voicemail services, that I built allow, users to call a number and record, voice messages, in their local language and listen, to messages that are recorded by others so. Let's see an example of how these services work. My. Sunday. Show kosuna Kalia do, the bye. Says. You saw in this example users. Call a toll-free line and once, the call is connected they, press one on their phone keys to record a voice message and press, two to listen to messages that are recorded by others and all, these conversations, are happening in their local language, the. Design of these services, overcome literacy, barriers by, using speaking, and listening skills language. Barriers by using local language and dialect of users. Socio-economic. Barriers by using toll-free lines so. The different poor people can call these services, and connectivity. Barriers, by using ordinary phone calls from any types of faults the. Inclusive, accessible, and, usable design of these services, has, motivated, not only me but several, other development, researchers, and practitioners who. Have applied these services, and diverse. Domains such as health agriculture, civic, engagement, employment. Education. And many. Others and together. These, services, have received millions of calls and voice messages in local languages from, marginalized, people in fact, these services, are perhaps the only way by which a lot of people are connected, to each other and produce, and consume information. Yes. Correct. That's it not much more than that no starting point absolutely, awesome Wow. Now. Yes. Recorded. Messages which ones do they get yeah. So that and we'll talk a little bit more about it but essentially all the messages with which other people are recording, and they are really interesting cushions, on for. Which ones they are going to listen because when they are listening to these messages it, takes up a lot more time than skilling, is -, absolutely. And I'll talk a little bit more about it in fact, this, question is a great segue so all these services are great but, there are three fundamental challenges.
Which Negatively impact, these services, the. First challenge is that users record, audio content in local languages, and dialects so it's very difficult to search index and moderate these messages. The. Second, challenge is that users, recall. These services by toll-free lines where, the cost of voice calls is paid by these services, and it, makes it difficult to financially, sustain them as the usage grows and third. These, services are technically, challenging, to build and maintain for. Global development organizations. And grassroots agencies, which. Makes, it really difficult to replicate them in new contexts, and, because. Of which the impact is dramatically reduced Bank. Of America pays someone millions of dollars to build their IVR services, and these, millions of dollars are often like beyond the reach of a lot of global. Development organizations. And grassroots agencies. So. These three challenges, result. In poor scalability. Sustainability. And replicability, and. I found it disheartening, to say that, because of these three challenges, most, of the services that I showed in the previous slide are currently. Not running and and this is very frustrating. So. Most. Of my talk today is going to focus, on my efforts on creating scalable, sustainable and, replicable. Voice based social computing services, and although. The talk is going to focus on, work in global South my. Work has interesting, implications, for people in low resource environments. In the US and other. Developing regions as well developed regions as well I have, worked with people with vision impairment, in South Seattle refugees. Communities, in Seattle, and. Those. Those projects. Are the interesting extension of the work which I have done in the global south disability. Is an important part of the work and I'll talk a little bit more about it during the later part of the talk. So. Coming back to these services, let's, first focus on the content moderation, challenge. Now. When you browser edit channel like this it hardly, takes us a few moments to recognize that there are four posts, focusing.
On Canada, Amazon. NRA, and a study in the UK now. Imagine calling a voice based read it like service that, has thousands, of audio messages, in local languages. It's. Clear that you'll have to listen to all these messages sequentially, and if, the first few messages are of poor quality you, may never called the service again it's. Also very difficult to, Kim categorized and searched these messages, and to. Improve the experience, of millions, of users who use these services it's. Important, to categorize, these messages, review, their quality, and decide, their playback order, now. Many services, often hire a dedicated, team of ten to fifteen moderators, who, listen to each voice message, extract. Metadata information, like content type and gender and review. The quality to ensure that only high quality messages. Are available for public consumption, and this. Approach works fantastically, well when, a service, receives 100 or thousands, of audio messages, per day but. If these services grow. By orders, of magnitude to. Match the scale of Facebook, whatsapp or Twitter it. Would be very difficult to compare ibly grow the moderator team. Now. Various internet websites such as reddit and Stack Overflow phase, the exact same challenge, and they, use community, moderation, to manage user generated content and in, this work I explored exactly, that can, community, moderation, be used to manage content on these voice based services in other. Words, can. Marginalize, users of these services, who. Are using a social computing, system, for the first time in their life be, able to search, moderate. And categorize these messages, to. Examine these questions I designed. And built Sangeet shuara a voice, based social media service that enabled. Users to record to, listen to and more, importantly to, vote on these, songs. Jokes, poems, and other cultural content so. When I use these community, words to categorize. And moderate. These messages. To. Use the service users. Called a toll-free number and once, their call was connected, they, could press one to know how many people have liked or disliked their messages, press. Two to record a new message and press, three to, listen to and vote on messages, so. Let's see what happens when users phone call is connected and they press three you. Can see the translations, at the bottom of this screen. Sorry. The. Yeah follow. My, lead and. Guarded. Agar. Aapke has sandesh Poisson daya to, eat the bucket of novelty. Agar. Up we Hasan this person, here yeah thought. About corrupt novelty this. And a scoop up is cynically, eight-team divine is. Sunday, school. So. Get. An idea that while users are listening to these messages they. Are pressing, Keys on their phone keypad. To cast votes and these, words are used to moderate. These messages, categorize, these messages, and review, their quality. Now. It's very difficult to use existing collaborative. Filtering techniques, to moderate audio content, because, of differences in the properties of voice and text features. Of IVR interface, and text interface and challenges. And automatically, extracting, features from, audio files that I recorded in local languages and that's. Why I design new community, moderation, algorithm, to order and rank these messages, based on user sports, to. Order messages, swara, mixed high quality messages and new messages, to, maintain a balance between novelty, and popularity, so, that both listeners, and contributors, have a good experience and. To. Rank. These messages, Sara, gave high score for messages with high ratio of upwards to downwards meaning, that these messages were of high quality and, expressed. High confidence in the judgement when more people voted on these messages, and after. Incorporating, this community. Moderation, algorithm, into, the design of swara I seeded. It with 15, songs and poems and shared, information about it with 73, people in rural India and in. Just 11 weeks the, service received 25,000. Calls from, nearly 1500 users who.
Recorded, More than 5,400. Audio. Messages, casted. Nearly hundred and forty thousand votes and listened, to all these files more than 200,000 times the. Average call duration was, five minutes and as you can see in the map the, usage spread all across north in central india. The. User analysis, indicated that more than 50% of the users were living in rural areas almost. All of them were men living, in low-income environments, with, respect to their occupation, more, than 50% for students and the rest were teachers farmers and musicians, and more. Than one-fourth of the users self-reported. Themselves as blind and this number in reality, was actually much higher and. I'll. Talk about these, numbers in a bit but eighty percent of these users, who were using the service were, using a social media system. For the first time in their life, now. For community, moderation, to work the, users need to deeply, value the community and should, ever desire to improve it so the, first thing I've measured is where the users valued their interaction, with the community members, the. Content analysis, indicated that, more than 80 percent of the messages, were generic content songs. Poems, jokes, that, followed the standards of the community, generic. Content included. Compliments. And greetings. To other users and. Discussion. On topics of national, and regional interests. Songs. Included, a much wider variety of content, such as recognizable, hits for, music, solos. Duets, a cappella, and even, pieces with instruments, if, I don't get a job my, backup plan is to mix all these songs in the CDs and then sell them. Now. Though I didn't design the system exclusively to be exclusively. To be used by blind people swear. I found broad and impassioned, usage by them they. Recorded strong positive sentiments, about the service and shared, interesting, anecdotes, about how the service, was impacting, their lives a blowin. Compliant user reported, I. Couldn't. Get educated I want, to thank you because you enabled all blind people to get in touch and share no. Matter how much I praise it won't be enough, I was. Really curious to see if low-income, blind people would derive the same benefits, if they, use mainstream, social media platforms, like Facebook whatsapp, and Twitter and I've found that even if low-income blind people have access to devices, connectivity. As well as, literacy skills they, still face several secondary barriers that, negatively impact their participation, on these services and again, I can speak for the remainder of the talk who why is that so but just to give you a few highlights. Low-income. Blind people had no training on how to use accessibility, tools and how, to navigate these platforms, which have complex user interfaces, their, ex perience on Facebook was as if, it's Amazon a platform, to buy and sell products because they were not expecting, advertisements, and when advertisements, started. Showing up they were like okay this is where I make a post to sell something so their mental models were also very different and those. Who are using these services faced, huge, accessibility. Inclusivity, and, usability barriers, when. They screen reader tool the. Screen. Reader software and accessibility, tool did not work well when messages contained, abbreviations, and code, mixing when more than two languages are mixed with each other when, they were typing messages for others. Did. You have any positive judgment. Objectionable. Content. So. In contrast. The, design of this service put blind people on equal footing with sighted people and made, them feel more included and confident, in. Addition to blind people the, service saw a great adoption by low-income people who perceived it as a platform for rural users and musicians, a musician. And peri-urban area reported who was also blind so. I was trying to get talent from people in villages and towns it, is getting recognition to those who, never got an opportunity to show their talent and the. Users also self-reported. Improving. Their confidence, grammar vocabulary. Communication. Skills through its use and all, these benefits were self-reported, we didn't even actually ask them were, there any improvements, on the. Instrumental, benefits a low-income.
Farmer, Reported some. People record cushions which increases, our knowledge we. Learn new vocabulary and accent I feel, great when people give me feedback, I consciously, think of ways to improve my messages, and collectively. These findings indicate that users indeed valued their interaction, with the community members yes. Community. Members are you saying the. System itself is the community or across. Thanks. Thanks for clarification, sir by community, members I mean people. Who are using that system which, were generally, of the same socioeconomic status. Were. Generally, facing the same kind of challenges but. Of course distributed, geographically. But. Had out of similarities, with them but community of the users who are using the service. Yes. Sorry. Within the system, are there was, their structure, like friends, and Facebook or is also sort of one big open so not in this system so because, we wanted, to design something really. Simple we weren't sure like how people, would react to this this voting mechanisms, because I mean, it's for some of these people or in some of these like. Communities. Like. When people are using an interactive voice response service, for the first time they think that they are talking to someone so, the mental models are really different so we weren't even sure like if this would work out that. Like, that they would understand that they should vote and how these votes are impacting, moderation. And categorization of these messages so in this system there were no like, friends. Or subgroups but, in other systems we have built those and they were fine as well yes, how. Long did you leave this just about and once, the traffic was like over time yeah, and and I'll show you the traffic in a bit but the, traffic was growing it was just so, one, thing to note here is that we least told 73. People about the system and it grew to 1500 just by word of mouth we didn't do any advertisements it was essentially, just like keeping keep, going up and after. Some months like we actually, had to pull the plug because we were out of funding yeah. We. Feel that people gave us the single binary, okay. There's. No. Questions. Like up or down for this topic, yes. So and that's, you, always ask me the questions which is the next life thanks for asking it because.
You're. Right so. Yeah. So in this slide, I won't talk about how we, conducted a, series of evaluations, to examine the extent to which, users, categorized and moderated, these messages, to categorize, content, users, identified. The gender of the recorder, and the type of content all by pressing keys on their phone keypad with, the response rate of 93% and, accuracy of 98%, so, in this case they were asked questions like now, you're going to listen to a message press 1 if it is recorded by a male press, 2 if it is recorded by a female, press 3 if you're confused, in case. Of content. Type categorization, they, were asked press 1 if it is a song joke and some, so we had like different questions which were changed but the, modality, by which they were giving feedback was always up one, or two were press like a port or downward and things like that. Now. As I as you may recall the. Votes from users determined, the quality of the messages so, I examined, whether, low ranked messages and hiring messages, differed from each other and, I found that, most, messages, that were poorly rated by users contained. Inappropriate, and miscellaneous content, and most, messages, that were highly rated by users, contain. High-quality and meaningful content I also, found that messages. From female users received more favorable, response from users again, I'll talk, a little bit more about. The. The. Abusive. Content and appropriate, content. Further, down the line. So. I then examined, how, well did community rank and moderated, these messages, compared, to expert moderators, and I, found that users, made. Meaningful distinction, between top quality messages and bottom quality messages and perform. Judgments, that were in ninety percent agreement with the expert moderators, and finally. I qualitatively. Examined, where the users understood, how their votes impacted. Categorization and, moderation of these messages and, I found that most users understood, how moderation, worked which, was a big surprise for us and were satisfied, with this quality. So. My vocals, were I made two significant, contributions, I built, the first community, moderated, voice based social. Media service that connected, people provided. Them information and gave, them digital equity and I, demonstrated, that, low income low literate people rural. Residents, and blind, people can, moderate themselves, without any outside support. Sarai's. Inspired, several global development, researchers, and practitioners to, use community, moderation, to manage content on these voice based services just. As in as an example researchers. In Pakistan, now use community, moderation, to manage content on a service that, has received quarter million calls messages, and votes.
Now. After this work I tried, several approaches, to address the financial sustainability challenge. To. Remind you users. Call these services by toll-free lines where, the cost of voice calls is paid by these services. And this, makes it difficult to financially, sustain them as the usage grows. So. A first examined would users pay for calling a service the deeply value and passionately, use to. Investigate this I converted. The toll-free lines to regular lines at the peak of surahs usage, but. Soon after the conversion the, usage dropped to zero indicating. That users cannot pay for voice calls even, to use the services they deeply value I. Then. Examined, can, incentives, prompt people from a slightly higher income group to pay for voice call costs, I hope. That if this new service grows, to a larger scale then, profits, from paying users could be used to cross subsidize participation. Of low-income users, to. Investigate, this I relaunched. The exact same service in an urban environment and, gave, users financial. Incentives, in the form of chances, to win a smartphone each week with the hope that these incentives, are going, to see long-term participation. But. Soon after the last smartphone, was awarded the usage again dropped to zero indicating. That this approach also cannot be used to address financial sustainability challenge. I then. Asked is, there a way to reduce cost at least for those users of these services, who, own a smartphone and use the Internet, I found. That building a smartphone application that. Works exactly like an IVR application, and uses. Voice channel instead of dates give the chooses data channel instead of voice channel to upload and download voice messages, can. Reduce the cost of participation, by a factor of 25 and you. Can imagine that I was pretty happy when Mark Zuckerberg mentioned, this application, in his internet hog speech but. This approach alone cannot address the financial sustainability challenge. Because, only a few users of these services, own a smartphone and use the internet I, then. Investigate, it can, users, complete, some useful work on their mobile phones to, get free airtime to use these services and, all. Of us know about crowdsourcing, marketplaces. That have enabled low-income, millions, of low-income people to earn money by, performing micro, tasks such as image tagging keyword tagging transcription, and translation. But. These platforms, are inappropriate, for typical users of these services, who, often do not have connectivity, devices, and literacy and even. If these people. Or have, like access to devices, connectivity. And literacy. Skills prior, researchers, including, some of those who are in the room have shown that. Low-income students. Or low-income people and blind people face huge, usability, accessibility and, inclusivity barriers, and using, these crowdsourcing marketplaces. So. Although I started, exploring digital, work ecosystem, in the context, of financially, sustaining, voice forums I quickly. Realized that there is a much bigger opportunity to. Enable illiterate, people and basic, mobile phone users to earn money I hoped. That, if. Illiterate. People basic, mobile phone users can do something useful by using their existing devices. And skillsets then, profits, from that work could be used to provide them earnings as well as, a small portion of those profits, could be used, to provide them free, airtime to use these services. The. Most common thing around here will be just seek advertising. Yeah so. Is, that something you skipped or is this seems more ambitious and nobler. So. Advertising, was something. Which was definitely on the mind but. These most of these services are driven, bottom-up, are by. Are. Provided, by generally. Organizations. Which don't have high operating budget and advertising. Is really lucrative, when it reaches a large scale for, advertisers to come and showcase their product, so generally Facebook. Is fantastic, for advertising because there are 2 billion people who are using it in order. To reach this scale it, requires a huge initial investment, because you are paying for cost of voice calls to, reach the scale at which the advent advertising, is going to be useful and a. Lot, of these organizations do. Not have that initial investment having. Said that there is an existential proof that, there is a. Company. In India which, has 5.4 billion dollars of revenue so, they created a service which is exactly similar to the one I showed earlier, and. People, use it for entertainment and, they use it to advertise, their products, to them so, there is an existing shell proof but that, model doesn't work for most of the services that I deploy it all across the world yes. Especially problem for these services are specifically, targeted, at very, low income, users that they probably aren't a great market.
For Advertising, so. For that company, the reason so did they make everything, like shampoo soaps and. And, in, developing, regions generally like you get a shampoo for like. A CSI, SEM shampoo, which. Cost like, one Indian rupee which is nothing and a lot of people like can, afford it because they can't buy a big bottle which would cause them three dollars but. They can definitely spend one cent in buying a shampoo which is just this little so, this, model of, providing. Services, in very, small cheap, packaging, is really. Popular in. A lot of low resource environments, and that's why that company is, using that service to market, those sachets and, like. Really small soaps which, could be used like this maybe one or two two, times and that's it but, in general yes like advertising. It's a it's a good and interesting problem because most of the things for which people want to advertise it's. Not. Available. Or is not usable for these communities, because of the price so, that too. So. Towards this ambitious, goal I needed. To create a new crowdsourcing. Marketplace that. Works on basic mobile phones and use. Voice as the modality, to do tasks. But. With these design constraints, the key question is what, is a compelling problem that can be divided into y space micro costs and generate. Revenues and many. Of you may have used speech transcription services, for your work but, speech transcription is the process of converting an audio content, of a file into, the equivalent text, and speech transcriptions, are really, popular huge, industry and therefore, there are several solutions such as manual transcription using. Online services as well, as submitting the files directly to speech recognition engines, but. These current solutions, yield, transcription, either with poor accuracy or high, cost for audio files containing local languages and excels and just. To give you an estimate the, average market, rate for Hindi and Indian English transcription, is five dollars per minute. So. Collectively, in this work my goal was to design a voice based phone based crowdsourcing, marketplace that. Facilitate, transcription, of load source languages, and accents, so as to generate enough, profits to provide earnings as well as free airtime to users. To. This end I designed, and built Reis Peak a voice, based crowd powered speech transcription platform. That, combines the benefits of both human, intelligence and speech recognition systems. Instead. Of transcribing audio files by typing, and reduce, tedious, process in which you have to listen to a segment remember, it type it by the time you are typing it you forgot what you heard so, you go back and forth and if you have to punish someone and just ask them to transcribe an audio file for you for one hour, but. Instead of transcribing, audio files by typing briefs, Peak enabled users to vocally, transcribe, audio files to produce transcript, free speak users repeat content, into an off-the-shelf speech recognition, engine which, automatically, types a broken, transcript for them and, I'll. Come back to how how we make use of that broken transcript. Now, the respec system has two main components the engine and the user application, now, initially I was unsure whether the goal of designing a, crowdsourcing, marketplace that. Enables users to vocally, transcribe, audio files is have been possible, so. I first build a smartphone application and deployed, it to low income students, one of the main target demographics, of voice based services I. Then. Built an accessible, version of the same application and deployed, it to low-income blind people another, target, demographic, of these voice based services and after. Carefully, investigating, these deployments I converted. The smartphone, application into, an IVR, application, which works on basic mobile phones our actual goal and deployed. It to low-income rural residents, the third target demographic, for these voice based services all, these. Three applications works. In the exact same manner and uses the same underline engine. Now. To enable users to vocally, transcribe, audio files briefs peak uses a five-step process in the. First step the engine segments a large audio file for transcription, into short recurrences that are easier for users to remember in the.
Second Step each, segment is send to multiple application, users. These. Examples, are English just for the demo the actual UI and, the, audio prompts were in local languages, now. In the third step the application, users listen to a segment. Repeat the same words back into the application. Sorry, remembered the content and repeat. The same words back into the application in a quiet environment the. Application, uses an off-the-shelf speech recognition engine to producer transcript, that is read aloud to the users, the. User then verifies if the transcript, matches, with the original audio segment they heard and if, it does this, submits it in order to receive a new task. So. Let's see a demo of how users vocally, transcribe, audio files yes. Something. So I, see. That you've got humans verifying, the speech recognition, Jetta, correct no. Understanding. Of the so, I mean that's the verify oh, yeah, the, verified part is so. They listen with the verification, could be against just, doing ASR. Against the original yes can't speak speech. No. No so in this, step they repeat, the content right and they get a transcript, the, transcript, is read aloud because most of them can't read because. Of literacy issues and then, they see whether the transcript, matches with the original, audio segment they heard like in terms of coin I think what my asking, is why don't you just run the. Because. The sorry the, original audio files have a lot of noises ambient. Noises unclear. Speeches, so if you submit. Yes. Sorry. Yeah. Yeah. So let's see a demo of how users vocally transcribe audio files on the, left is a blind person using the smartphone application on the, right a group of women are using the IVR application, and all of them are doing the same task. One. Rupee please. Button. I. Made. Her. Press. The record button and repeat what you just heard this, will open a Google app for recognizing, speech break. It button. Sati. Sati, okey but something, is missing. We. Recognize this soir p.m. he bought something he does. This closely match with the audio file you heard. No. Button, yes. Button. So. There transcript yes I mean. There was verify, the transcription. Thank you they a lot of these are literate so normally. You would read that out loud yes so even in this case it actually read it aloud and it. Wasn't clear it was the screen reader which read it aloud in this, case like we build a text-to-speech system. Which. Was like getting the text transcript from the ASR and that read aloud to the users and there was a slight delay that's why they were like zooming, a little bit later than this guy. So. The transcript this produced is expected, to have some errors since users may not fully recognize, the content or, the speech recognition engine may make some mistakes and recognizing, some words and to. Reduce these errors for, each segment the. Engine combines multiple users, output transcripts, into one best estimation, transcript, by using string alignment, and majority voting process if the. Errors in the output from speech recognition engine are randomly distributed merging. The transcript from multiple, users improves. The overall accuracy, if the. Correct word is recognized for majority of users the. Transcript sent by user is then compared, with the best estimation transcript, generated by the engine to determine users reward. Now. This figure depicts the estimated, improvements. In accuracy from majority, voting and string alignment process now. X-axis, has the speech recognition accuracy for individual, users and y-axis. Is the accuracy of transcript. Obtained after string alignment, and assuming. That these errors are randomly distributed, the. Accuracy of aligned transcript, increases, as the number of speakers increases, but, the comparative, gain decreases between, 3 5 & 7 users.
Now. In the final step the reach speak engine combines. Best estimation transcript, for each segment into, one large file to yield the final transcription. Now while building a new system a large, number of design parameters, needs to be investigated in a systematic, manner I first. Conducted a series of cognitive experiments, usability. Studies and experimental, evaluations, with, 67, low-income participants. To, identify, key design insights into, each activities, users due to vocally transcribe, audio files like listening remembering, Ries, peeking and verifying transcript, and I apologize, that this slide is dense but. Just to give you an example to, make it easier for users to remember the segments examined. How these audio files should be partitioned, what, should be their length and how, these files should be presented to the users and I found that, audio file should be partitioned, by detecting, natural, pauses to, yield segments, of less than 6 seconds in length and these, segments, should be presented sequentially. To reduce cognitive load and improve. Content, retention, and after. Incorporating, these design. Insights and to reece peak, the. Design of free speak, I then, ceded the engine with, nearly 5 hours of in the content, such, as interview songs news speeches, telephone, calls and the. Engine segmented, these files to yield over 4,000, micro tasks I then. Deployed the race peak application, for, one month with lowing 25, low-income students, be, speak application, to 24 low-income blind people for another two weeks and the, recall IVR application, to 24 rural residents, for for another two weeks and, together. These users completed, more than 50,000, micro tasks and on 31,000. Indian rupees by vocally transcribing, audio files. The. Speech recognition, accuracy was, 71%. The. Accuracy of transcript, obtained after. String alignment, and majority voting was 92%. The. Transcription, cost was one dollars and 30 cents per minute nearly 1/4 of the average market rate and users. And 50 Indian rupees per hour nearly 1.5, times the average hourly wage rate in India. Now. The deployment, also indicated, clear benefits, of the approach, of asking users to repeat content, and using string alignment and majority vote and this, goes back to the question of mic like why we shouldn't submit the audio files directly, so. In this graph x-axis, is the number of transcripts, used in multiple strong alignment and y-axis. Accuracy of speech transcription, now. The green line plots the accuracy, for regional files are submitted directly to a speech recognition engine and in, this case the accuracy was pretty low because of MBA noises and unfair speeches, the. Yellow line plots the accuracy, if users repeat content, into a speech recognition engine and in, this case the accuracy, is much higher because users, are speaking high-quality content in a quiet environment directly, into a speech recognition engine. The. Blue line plots the improvements, in accuracy by string. Alignment, and majority voting and. The. But. You. Can ask is accuracy here. And. So. Accuracy. Is one - 100, - word I read in percentages, and the, white line plots the expected, improvements, in accuracy using the model I showed in, the, couple. Of slides back which. Expose you, mning that all errors are randomly distributed, the. Gap between white and blue line is because there. Were some errors that were systemic, in nature for. Example there, were certain portions of audio files which, were difficult for a majority of users to remember and three speak or the, speech recognition, engine had some biases because of which it couldn't recognized, the extent of majority of users. Now. Comparing, the, different deployments, compared. To sighted users blind. Users completed, three times more tasks, and on, 2.5 times more money in just, half the deployment, duration blind. People were much more enthusiastic to, vocally transcribe, audio files because, most of them earned an earning opportunity, for the first time in their life, one. Of them reported, I am grateful. To you for creating, the app I owned, the money for the first time and learned, the value of each grouping and again. They also self-reported. Improving their knowledge and pronunciation without. Us asking anything about it another. Blind person reported the, app improved my pronunciation. As I was speaking words more carefully to, get them recognized, they, also valued listening to extent, from a wide variety of people while they were transcribing. The audio files because, that's a skill which they really care about that they they want to be able to understand, extends.
Of Different people so that they can have more fruitful, and faster conversations, with others. Now. Blind users produce transcripts, with 14%, less individual, accuracy, and more, cause than sighted users and the, accuracy was low not because of disability, but. Because they were non-native, speakers of Hindi so during our selection process they, could have conversations with us in Hindi but, they find it really challenging, to, repeat, words in, a timed environment into. A speech recognition engine because, they were non-native speakers and, because. The accuracy was low the, engine sent, tasks to more people because of which the engine had to pay more people which increase the cost of transcription. Now. Compared, to users who use the smartphone application, rural. Residents, who used the IVR application. Completed. Five times more tasks and on, seven times more money and just have the deployment duration. Rural. Residents, were immensely, excited, at the prospects of earning, money by digital, work and they found it this will work much easier than manual labour one. Of them reported, labourers. Work nine hours a day to 120 500 rupees per month they. Can use the application for just two hours daily to earn the same amount. Just. Towards daily for, the entire month. Now. Compared, to the, users. Of smartphone. Application, IVR. Application, users produce transcripts, roughly with the same accuracy which was a surprise for us because we were thinking that using. Speech recognition engine on basic phones would reduce, the accuracy. Dramatically. But it dint but. At more cost almost, double the cost and the, cost was higher because, the engine was paying for two things earnings, to the user as well, as their cost of calls to use the recall IVR application, itself. Great. Question so, to, revisit the, goal of this work was. To. Revisit the goal of this work was to enable users to earn money and to provide them free airtime and, recall. Generated, enough profits to do both an hour, of crowd work on recall provided. Users eight hours of free air time while, supplementing, their income at a rate higher than the average hourly wage rate in India and if, all profits are used just to provide them earnings and hour, of crowd work could enable users to get more than three three. Times the average hourly wage rate and. Of crowd work could, give them 12 hours of free time free air time if all of it is used to provide them this, so. In the final evaluation I integrated, recall, with sang kids were at the system that I showed earlier and, I found that users were successfully. Doing tasks on recall to, get free air time to use the service, moreover, the switching between two applications, to do tasks, and to use the air time did, not affect their user experience. So. In this work I made, three significant, contributions, I built, the first voice based crowdsourcing, marketplace for, illiterate people and basic mobile phone users I demonstrated. That low-income students, blind people and rural residents, can, vocally, transcribe, audio files with high accuracy and, I showed that the, profits from crowd work could provide them earnings as well, as free airtime to users thereby. Giving us a pretty solid approach to address the financial sustainability challenge. The. Potential, of this work to increase the income of blind people is so strong that several, social enterprises, in the US and India have reached out to me to scale this technology, and to commercialize, it. Now. Let's focus on the final challenge, the setup and connectivity challenge, and to, remind you these, services, are technically, challenging to build and maintain for, global development organizations. And grassroots agencies, because. Of which it's really hard to replicate them in new contexts, even when, these services, work they operate in silos impairing. Information, exchange between local, community of people who are using the service and global. Community of people like us who use mainstream, social media platforms, like Facebook Twitter whatsapp, etc and, to, overcome these challenges, I built. IVR Junction a toolkit. That makes it easier for anyone to build and maintain voice based services, all they. Need is a community computer and off-the-shelf, modem costing less than hundred dollars and Internet, connectivity. Also. The services built using IVR, Junction are connected to Facebook, and YouTube give. Thereby giving a global platform to local voices and, facilitating. Information, exchange between local, community of users and global. Community of audiences, if desirable. IVR. Junction also has a distributed, architecture thanks, to SkyDrive, and table crop box which. Makes it cost-effective as well as resilient to network outages in the, cases these services are deployed in areas, where there is reproduce. And because. Of these advantages in, the last few years several. Organizations, have used IVR, Junction to create the, kind of services that have been describing since the beginning in several.
Countries And these, services have received more than hundred and ten thousand phone calls from nearly 25,000. Users and just to give you some examples, the. Office of the president of Somaliland, used, IBR junction to connect, government. Officials with rural tribal people, to, improve trust and transparency in, the political processes the. Government officials record, a message by using an, IVR service and citizens. Respond, to these messages the public policy opinions, etc, by, making the simple phone call and all, these conversations, are also available on a youtube channel for the, interest of people in diaspora Andorra, and are also indexed, on the official website of the Parliament of Somaliland. Similarly. Voice of America used, IVR junction to provide reliable and up-to-date news, in the, local language in Mali and. Women. Right activists, in India used, IBO Junction to create a voice petition, platform, after, a gang rape incident, that sparked national and international, outrage. IVR. Junction has received recognition from global development, organizations, like US aid Human. Rights agencies, like humanity United in excess now as well, as mainstream social media platforms, like Facebook and. Overall. My, thesis research has addressed three fundamental, challenges, of voice based social computing services, to make them more scalable sustainable and, replicable and my. Work has enabled illiterate people basic. Mobile phone users blind people to connect, with each other produce. And consume information and, get, a voice agency, and equity but. One of the questions that still bothers me is, how can I bring benefits, of social computing to, people who do not even have mobile phones and this is not a hypothetical question during. My fieldwork in low resource environments. In India, Africa, even, in the US I found. A lot of people who do not who, do not have mobile devices and according. To an estimate there. Are more than 1.7. Billion such women in low, and middle income countries and unrelated. To the fact that they don't have mobile phones a high, majority of them have poor access to health information resources, because. Of which there is an unusual high, number of maternal, deaths in these regions and that's, just one use case of how. There, are environments, where there are no computing. Solutions, at all and how, there are like huge social, challenges. In these areas. So. My thesis research has outgrown into a new significant, direction where, I examine, ways to bring benefits, of social computing to, women who do not have mobile, phones and who do not have health information, I'll give. You a very high-level summary of these investigations, but happy to follow up in more detail after the talk now. To enable women without mobile phones to access report, and share health information, I extended. My work beyond, voice to, include other modalities, like video, I've. Worked with path on projecting, health a video-based social, computing intervention.
Where, Local villagers and community health workers come together to, create, hyperlocal. Infotainment, videos, discussing, key health issues in the local dialect and once. These videos are produced health. Workers use a handheld projector, to show these videos and facilitated, group sessions with pregnant women and new mother to, provide them information and, this, work is in the domain, of health but it borrows from the work which was done at Microsoft, Research India almost 10 years back and which. Is now a successful. Organization perhaps. Working in several countries with hundreds. Of thousands of farmers in the, agriculture domain but. The challenges, in this intervention and that large-scale product, is exactly, similar. So. I conducted, several investigations. To improve the design and implementation of project in health for, example a limitation. Of this intervention is that there is no they by which women can watch these videos against again. Once they step out of the group session with pregnant women and new mothers I have. Examined how, different, community members like, shop owners students. And community, health workers can extend, the reach and Ja graphic spread of these videos also. These, videos are designed locally, to improve, information, absorption, but. What is the definition of local is, it, their neighborhood, is it their village is it, their city their, County their state so. I've investigated how different video attributes. Like content-type. Accent. Quality. Effectors production, quality should. Be localized, and how these localizations. Effect information, absorption, and finally. It is extremely difficult to get constructive, feedback from women to improve these videos I have, used techniques. From behavioral psychology, to reduce response, bias and I would like to call out this work because, this work has really interesting implications for accessibility researchers, as well because. Accessibility, researchers. Design technology, for people who are absolutely excluded. And they, feel so nice that someone is making an effort and as a result they give really, strong positive feedback. Which. Might not be the honest and critical feedback which you would need as researchers, and Mary. And I were talking almost a year back about, how this work could be extended in the domain of accessibility. So. Far in this intervention hundred, and ten locally produced videos, have been shown in hundred and eighty Indian villages impacting. Lives of an estimated hundred and ninety thousand whole residents. To. Reiterate my research, goal is to bring benefits, of social computing to billions, of people who. Face literacy, language socioeconomic. Accessibility. And connectivity barriers, in particular. I am driven to connect them provide, them information and, bring, social and digital equity to them, more. Broadly much, of the work that I've done and that a plan to do follows. The framework of belt systematize. And deploy, I built. Social computing systems, for all people, particularly. To empower those who, are excluded because of their disability, or because of other barriers i systematize. How, these new users produce consume and share information and offline and online social, spaces and I. Deploy these social computing systems, to achieve social good by addressing information and instrumental, needs of all people in today's, talk I showed how, I used interdisciplinary. Methods and how I partner with several organizations to. See my research goals solution. Although. Today I shared only a slice of my research I have, built several social computing systems, including, social media platforms, discussion, forums crowdsourcing. Marketplaces, and information. Systems and these, systems target, different populations, such as low-income blind people rural. Residents, indigenious, communities, women, living in societies, with prude patriarchal, values and, these. Systems attempt, to improve their access to education, health, employment. And entertainment. Looking. Forward I plan, to explore multiple focused, directions, at the intersection, of social computing and accessibility, but, in the interest of time I will briefly mention only two of them and this. Goes back to Steve's question about, missing about, harassment on these services so.
Although The services, that I built worked exceedingly well for low-income people blind. People rural, residents, I found. It disheartening, to discover that, women face systemic, marginalization. And encountered, flirts threats abuses, and blackmail on these services and it's. Not just on the services that I have built and deployed, in different continents, but also the services, which are built by other researchers, and that's, the recent CAI paper which you should which. You should read to understand. Like what are these kind of harassing. Behavior but. Just to give you a context. Almost. 98%. Of flirty messages. 62%. Of threatening messages, and 46%. Of abusive messages were targeted, at women who were, only 6% of all users I, also. Found several instances of disinformation and, misinformation, on, these services and these, challenges are, not. Unique to these services in fact mainstream social, media organizations, also, face these grand challenges, so. Going forward I plan, to use techniques from collaborative filtering machine, learning public, policy, to reduce harassment, and misinformation on these, services as well as other systems which we which, are all around us but, just to give you some really interesting research, questions, like. What. Features could identify an appropriate content, in local languages audio files or, how. To identify interconnected. Network and interrelated, activities of, DOE spreading disinformation because, it's always a disorganized. Effort. And. What to do in situations where. Collective, intelligence of the community, is eclipsed. By their collective ignorance as in, the case of community, condoning bullying of women on the platforms that I have read another. Area of future work that I plan to do is to bring benefits of social computing to people with disabilities. During. My PhD I successfully. Built several social computing systems, for blind people but. Vision impairment, is just one the many types of disabilities, as. I speak more, than 1 billion people in the world struggle. With some form of the disability, and most, of them live in low-income settings, even. In countries like us I am. Eager, to build inclusive technologies. To make this world more equitable, for, people with disabilities, and just to give you an example I have. Access to a large amount of audio files containing, speaker diversity. Content. Diversity, and natural, speech elicitation. Using. Techniques from NLP, I'm really, excited to, build. New local language recognition models. And generate, labeled speech data and once, I have these models using, methods from HCI and accessibility first design I want, to create local language conversational, agents that, improve access of health education and employment for people with vision impairment, and people, with motor disabilities I, have, already started collaborating, with NLP and machine learning researchers. And these directions, and I look forward to collaborating with people here. So. Far the social computing systems, that I have built have reached an estimated, 220,000. People in, low resource environments. Looking. Forward I am driven, to expand the impact of my research to orders of magnitude more people, by, solving complex problems at the intersection of computing, and society I would, like to thank users, who have used the systems that I have built and have allowed me to be part of their lives I would, also like to thank organizations.
That Have funded my research or partnered with me and thank. You all for giving me this fantastic, opportunity to present my work thank, you. Yes. They. Are you're talking at, the beginning of the talk you mentioned in one of the childless the challenges, resulted, in many, of the services no longer good, but, then you demonstrated. That at least one of them is financially. Viable to, the pastures and I'm wondering it is that once more I mean and if not what. Were the challenges that resulted, in that line yes. That's, a fantastic question that's, not an example of a service which is running because I mean it was more or less on the lines of showing. That these services could, run and the plans right now is to integrate the, crowdsourcing marketplace that, I showed into, the existing, services that happening and I'll give you thanks, for asking because I wanted, to bring this in the talk and I couldn't that. Inspired. From the first project I presented where people are just talking to each other using basic mobile phones an organization. In India called enable India have, created, a really large social network, of I. Don't. Know how many users they have perhaps like. Definitely. Tens of thousands. Could be like reaching hundreds of thousands of users who. Are using the exact same system to, connect with each other to share information with each other about jobs, and opportunities, but that. That, service given. That it reach has, reached a larger scale sustainability's. Is, really important so we are actually working and talking, exactly with, that organization to, increase to include this crowdsourcing, marketplace into. Their into. Their system so that that. Service could, does. Not need to be dependent on funding from other organizations, now. I would also like to say that there are some examples of services which are running one, of them is CG. Netsu era which is again, a project from Microsoft, Research India and it, requires tremendous effort, to, run these services, like I as a graduate student count, on them because. I have to write papers I have to do PSD pick a new research project but. It requires some champion. Like who is and you. Know devoting their entire life to that service, or to, that organization it. Requires people who are constantly generating, funds it, requires a team of moderators.
Who Are listening to these messages so. This works makes it really not. Really easy, definitely, is in the right direction to make easier, for those people, to. Have. A sustainable. Well. The people. But. That is soon there is actually a market for, these prescriptions. Indicates. Can you find a big customer yes, definitely. And I I can't. Tell, you the name of the organization because this is recorded but there is a major. Player, who. Does transcription, and they, want to use this technique for transcribing, insurance, calls, and. So. Given call centers are really really popular I mean of course in the US but, even in developing regions and there is a lot of data which requires to be transcribed in these, local languages, so that's. The other organization. I was talking about so we are thinking how we can transcribe, in. That. Particular. Specific. Yes. Yes. And for, the next one I think that was. Every. Speak recording, yes so did, you see more females, participating, there or. So. Like in case of Reese peak and recall like they were pretty, much like a relatively. Long term I mean, I hesitate, to say long term because my definition of law is like really long term like two years when, you're kind of deployment but it was definitely running for one, month in two weeks so like it was a set I mean we asked them like hey would you like to participate right and we. Could like control the, I mean we could send a balance and we tried doing it in our selection process of participants, who are using the, in, case of the first system it was a system out in the wild and we had no control on how who, wants to use it how they use it. So. I spoke, about how I want to make. These services, more inclusive, accessible safe, for women and there. Are like bunch of ideas I would like to try for. Example giving. Them in more incentives, to join these services, using, gender recognition filters. To. Create only women, only services, like, creating some form of a constitution. On these platforms appointing. Sheriffs like who are like kicking people out, who. Are like reducing who are recording, abusive. Content on these services so a bunch of techniques we would, like I would like to try to ensure that even these services. Son, gets what I like services, have, Ardmore gender balanced but in case of Reese peak like it was we, were recruiting people, and, it was pretty much balanced. Yes. Great. Cushion so in, general, like at least to the people we recruited, like they had a mobile device. Their. Personalized, smartphone, device because they were generally young like students, or even blind people like, they were the ones like who used a smartphone because we deployed a smartphone application. But. You're right like we know that there was a shared device in at least two homes, or two participants, and we, know that the older family. Members were doing the tasks as well so, that was pleasantly, surprising, that okay like people who are like, old. Can. Also do these tasks, especially because they have much lower literacy skills. They. Haven't interacted much with the smartphone. Or the baby in that case it was a basic phone actually and, it. Goes on to speaking of hurt the values of universal and inclusive design. Yes. So. Two, questions one. Of which. Is. About. Repeat. Users so, first system like your, you kind of show this lot about traffic which is great that shows a big kiss, how often did people come back to the system versus like just seeing it using it because of novelty the.
Fantastic, Was honestly, I have forgotten the numbers but I mean I can give you some some number in the. Second. Some range so, I know that. The. Repeated users were like 30. To 40 percent and, I would have to look into the paper there were a lot of people who were just listening the content, not. Recording, it I. Know. I. Forgot what I wanted to say I've had, some interesting statistics to, this answer. So. Repeated users yeah, so, for women right, so I said, that there were only six percent of women users so, our analysis, indicated, that initially they were much more and they, were like. Excluded. From the community by people who were recording, abusive and harassment so. They were harassing, content, so they were like some users which were not repeat users because of what was going on in the content but in general I would remember I remember that they were like 20% or 30% of, the users were repeatedly calling the service and then, they were maybe. 10 or 20 percent of users who were only one time callers and the, remaining were somewhere in between that they would use it once in two days or once in three days my. Definition of regular users whoa who, are calling at least once a day. No. Yes. And it depends, on how how do you define an organization but. If. I was one of the users when I was in the message how likely would it be that I heard a message from somebody I've actually ever heard. Way. High. Pretty. High and anonymization. So no one knew like what is the. Number of a person who recorded the message so, that way it was anony
2019-10-20 04:26
there's no resource at the bottom of the population, and it sounded like a public services, so it should be funded by the population at the top or by the government.
Thx for video very interesting !