Raj Chetty: "Visualizing the American Dream" | Talks at Google
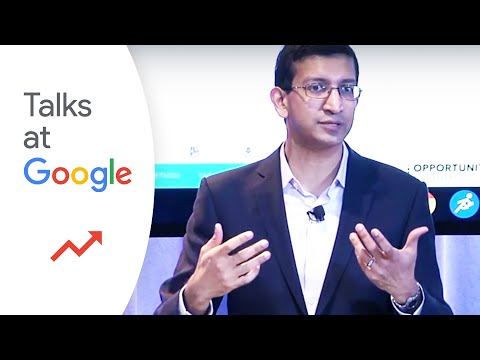
Well. Today I'm delighted to introduce one. Of Google's newest, partners, and. One of the world's leading experts, on opportunity, and mobility and, also. A personal, hero of mine my. Team knows I talk about him all the time as someone, who I think is doing some, of the most fantastic work. Globally around inclusion. Future. Of work inequity. So, we are thrilled to actually, say just, today that Google org is gonna be providing, a $250,000. Grant to help support some of the work that he is doing around opportunity. Insight. We. Don't do this with all of our speakers so just say it just say you know. But. A little background on, Raj, for on, dr. Teddy for those who don't know so he's a professor of economics at Harvard University, and he, was awarded MacArthur. Genius fellowship. And is, the winner of the John Bates Clark medal given, to the best American economist under the age of 40. Raj's, work asks how can we give children from disadvantaged, backgrounds the best, chance. Of economic, prosperity and, one. Thing I find very powerful, about his work is that he and his team has. Have, also figured out how to inject, the data and. More. Importantly the meaning, into, the central, narrative of this, country, and so. His work has been popularized, and been written about and as part of conversations, that probably, all of you are having without realizing, perhaps sometimes his, work is undergirding, it so for example he has found that the generation, born in the 80s is the first in modern history to have less. Than even odds about earning their parents I won't give away all of your good. Stuff but, a. Personal. Favorite of mine as the wife of a public school teacher that high quality elementary. Teachers generate, long-term gains. For their students including higher college attendance, and in, larger earnings and, that. In 99% of neighborhoods, in the United States black, boys earn less in adulthood, than white boys who grew up in families, with comparable income, 99%. So. This. Kind of work is, shaping not only. Our work in policy and academia but, the conversations, around America, and why it's so important, so, we're really pleased, that Google's. Own chief. Economist, dr. Hal Varian is gonna, join us and do. A fireside, chat and so, we're gonna start with dr. Chetty coming up and giving us some. Context. Then, we'll open up to have a chat and there'll be lots of times for questions, from all of you so thank you. Warm. Introduction, and, thank, you all for being here I really appreciate the generous support of google.org, looking, forward to partnering with you so. I'm gonna tell you a little bit today about what our research group is focused on broadly, on restoring, the American Dream and. I'm going to talk about how in our own local communities, and our local institutions, we can make a big difference on issues like inequality. And economic, opportunity. But I want to start at a much bigger picture level by, talking, about the American, dream which of course is a multi-faceted. Complicated. Concept, that means different things to different people but I want to distill it to a statistic, that we can measure systematically. In the, data which is a way that the American Dream has been traditionally conceptualized. The, idea that through hard work any child in America should, have the chance of going. On to have a higher living standard, than their parents of going on to earn more than their parents and so, what we're starting out with in this first chart is just an assessment, of whether that's true, in the United States today and how, the American Dream has, fared over time so what we're doing here is, calculating.
Very Simply the fraction, of kids who go on to earn more than their parents did based, on the year in which they're born and. Then so as you can see here for, kids born in the 1940s. And 1950s, it was a virtual guarantee that you were going to achieve the American dream of moving up relative, to your parents 92. Percent of kids born in 1940, went, on to earn more than their parents did if, you look at what has happened over time you see a very stark fading, of the American Dream such, that as Jacqueline, mentioned four kids born in the 1980s, who are turning 30 today when we're measuring their earnings it's, essentially, a coin flip a 50-50, shot as, to whether you're going to do better your parents now, this broad, trend, I think is not just of economic, interest I think it's a fundamental, social. And political interest, in the United States because I think it underlies, a lot of the frustration that. People around the u.s. are expressing, that the u.s. is no longer a land of opportunity, as it, once was so. Motivated by that trend in our research, group opportunity. Insights based, at Harvard, were, essentially, trying to tackle the big picture question of you know how can we restore, the American Dream what is driving that, trend and our approach has, really, three central, elements, the first which will be very familiar to all of you here so, if we try to harness large, datasets typically, structured, data coming from government. Data sources census, data tax data things like that in. Order to study how to increase upward, mobility and I think this is really a, special, moment in social science where much as you all are using data in your work to improve. The, products that people have access to I think our knowledge of social science is, going to be revolutionized, by, modern, big data in the coming decades. Second. We, focus greatly, as. Jacqueline. Mentioned are not just doing this research but trying to translate, that research to. Policy change, by trying to inform public conversations, or as I'll give you a couple of examples of, increasingly. Partnering. With local government agencies, colleges, other institutions, to really create change, on the, ground the. Third point. Of kind of a departure for what I'm going to show you is that rather than approaching the data at an aggregate, or national, level we, really try to hone in on specific geographic. Areas or specific, subgroups, to try to understand, what the drivers, of inequality. And opportunity, are and so the starting point for, what I'm going to talk about today is that there are very sharp local, differences in, rates, of upward mobility that I think should shape how we think about the broader problem of the, fading American, Dream and so I'm going to start by showing you that data but with this map here, it's, just to give you a bit of background before, I describe what it shows we construct, this map using.
Data Following, 20 million kids from birth to adulthood that's, using, anonymized information, from tax returns. Connected. To census records where, we basically track, these 20 million kids, all kids born in America in the early 1980s. Followed. Them to adulthood and look at how they ended up doing in terms of their earnings. Conditional. On growing up in a low-income family and so what we're doing in this map is taking that data and assigning. Kids to, locations, based, on where they grew up we divide the u.s. into seven hundred and forty different metro, and rural areas and in each of those areas we take the set of kids who grew up there in low-income, families, that is families at the 25th, percentile, the income distribution earning. About $25,000, a year we, follow those kids into adulthood and ask how much do the kids who grow up in each of these places earn on, average and, adulthood so think of this as a way of measuring how upward. Mobility varies. Across areas, in the United States and. So the, map is colored, such that blue areas, like in the center of the country represent. Areas, with higher levels of upward mobility and red colors represent, areas with, lower levels of upward mobility so. Start by just looking at the scale and the lower right you, can see that there's a dramatic range, and kids chances, of moving up across. The United States depending, upon where they grow up in the, darkest red colors you, see that kids who grow up in families earning about $25,000. A year their, own incomes, on average, are about. $27,000. A year and adulthood like in a place like Charlotte, North Carolina for example in contrast. If you look at the center of the country or a place like Salt Lake City for instance some. Areas on the coasts you, see, numbers. Like 45,000. Kids starting, out and families earning $25,000. A year or on, average ending, up earning, about $45,000. A year so. You. Know there's a very broad spectrum, in terms of rates. Of upward mobility within the United States that aggregate, national picture that I started out with really. Is driven by a great heterogeneity, across, areas, now, naturally, the the question of interest to us as academics, and to policymakers, to, ask what is driving this variation, across areas, and what ultimately might we be able to do to, increase upward, mobility in places, where, for subgroups where we currently have less Economic, Opportunity one. Thing I'd. Like to point out on that is that you might you know one of the first explanations, that might come to your mind is maybe differences, and upward mobility across places or, driven by differences in the types of jobs that people have access to or things like you.
Know Developments, and technology, and so forth as. You can see from this map though that. Doesn't, entirely appear to be the case right so if you take a example like, Charlotte, some, of you might know that Charlotte North Carolina is one of the most rapidly growing cities, in the United States it's had one, of the highest rates of job growth over the past 20 or 25 years yet. As you can see here, Charlotte, is one of the places in America with the very lowest levels, of upward mobility among, big cities for, the kids who grow up there so how does that add up how can you both have very high, job and wage growth and have very low upward mobility so, what's happening, in places like Charlotte, or Atlanta, or a number of other high-growth, cities is that they basically import. Talent, lots, of people move to Charlotte, move to Atlanta to get those high-paying jobs but, they don't necessarily grow, up there and it's not always the, case, that high job growth benefits the. Local residents, something we see in many different contexts this is one example, of that now in, this big map your, eye naturally. Gravitates, to the broad regional, variation, places like the Midwest look better for upward mobility than, the southeast, or places. In the industrial, Midwest like, Cleveland, and so forth but, what is striking and what we've been focusing, on more recently, in work that we released a couple months ago is that there's, substantial variation. Not just regionally. Across the country but, even within, cities at very small geographies. So to illustrate that I'm going to turn and flip over to this tool, that. We've developed called the opportunity. Atlas which you can access. And sorry, if we can just there, we go. So. You can access this you know freely by just going to opportunity, outlet org, and. Type in any address very, much like a Google, map and. Zoom. Into any area so I'm starting out with the map that I just showed you at a national, level and now I'm gonna zoom in you know given local interest here to the Bay Area and show. You the same data now, at. A census, tract, level in. In. The, Bay Area okay so census, tracts are. They're. About. There's, 70,000, census tracts in the United States and. Each of them have about 4,000, people so to give you a sense of scale a place like Palo Alto is, itself divided into 17, different neighborhoods. And what, we're showing you here so this is very fine-grained, information, same, statistic, that I started out with take low-income. Kids growing up in each in each place and. Essentially. Calculate their average incomes in adulthood there's some statistical, details in the background but that, that's that's basically what we're doing here and, so the first thing I'd like you to see on this map is if, you look at the Bay Area you see the same spectrum. Of colors that I showed you at the national, level right so you can go from a place that looks like Alabama, or, Charlotte. In terms of rates of upward mobility to a place that looks like Iowa within, a few miles within, the Bay Area, now some of the broad patterns. Here you know will strike you as intuitive so you see for instance that the peninsula, much, of San Francisco, has higher rates of upward mobility than. Parts of the East Bay in particular, Oakland, right. But. Let, me now soom in further to, discuss. This variation a little bit more detail in particular, to the areas where we have large, low-income populations. In Oakland and surrounding, areas to. Show you how you can pick out more granular, variation, that, I think illustrates, some. Of what might be going on here so, if we look here in Oakland. You. See that there's a very sharp you know almost a dividing, line between Oakland. And San Leandro right, where the colors almost shouldn't discreetly, from, red to blue so, part of that is, driven, by something, Jacqueline alluded to which is racial, differences, in economic opportunity, so, what you can do with these data is not just look at the data in aggregate but look at specific, subgroups, so, what I'm going to do here is click on black.
To Subset, the data to, black, Americans, and, you can see that some of that divide is driven by race but, even conditional. On race there, are sharp differences, in Oakland, relative, to San Leandro so, one, thing I should note just as a side note as, you pointed out even, within a given area there are really sharp differences, in rates of upward mobility between, blacks and whites and in particular, between black men and. White men if, you look at black women versus. White women you see very similar outcomes, but. In particular for men race plays an extremely important. Role okay, and so, it's, important, to see, that even, when you focus on the. Black population there's still the substantial, variation across very nearby neighborhoods, now, if I look specifically, at black men where you see some, of the most striking patterns, as I was just saying and I'm gonna look at black, men growing up in the very lowest income, families, you, see that there are certain places here, for instance let me click this tract which, is a neighborhood, called Fruitvale, in Oakland and you, see some really striking I think disturbing. Statistics, about the United States so here. You see that for. Low black men growing up in low-income families, and Fruitvale their, average, income in adulthood is five thousand, six hundred dollars, right, and importantly here one thing I should note is we're tracking these. Men no matter where they live in adulthood right so these are people who grew up in Fruitvale they might not be living there now might. Be somewhere. Completely different but among, the set of people grew, up there you have an average income of just five thousand six hundred so how is that possible, the way you get such a low average income is by having lots of people who are not working, at all and in, particular, what, you can do with these types of data there's, a lot not just at income but look at a variety of outcomes so if I click over here now to, look at incarceration. Rates you, see a really, striking, statistic which, is that 43%, of, the, black men who grew up in. This particular neighborhood are incarcerated. On, a single, day on the date of the 2010, census. So. If you think about lifetime, odds of being incarcerated growing, up in that neighborhood it's gonna look like 60. 70 % something like that which is how you end up with, an average earnings, of five thousand six hundred dollars right so, the point here is that there's this incredibly. You, know granular. Variation, at some level you might react to that by saying I'm shocked. By the magnitudes. That we see such you, know adverse outcomes but, maybe I had some sense that there were parts of Oakland that a really tough place is high poverty high crime, so, qualitatively, maybe I'm not so surprised by that picture but, you know what I think is perhaps. Much more surprise. You can go to, places that are just you, know a few miles south. Like. Down here in San Leandro where. You have rates of incarceration that, are, dramatically. Lower 5%. Or 1% compared. To what the 40% that we were seeing in Oakland right and so the point I'm trying to make here is that when we think about, economic. Opportunity. In America, really. We should be thinking at a much more local scale it's, not even about the bay area versus, you know other cities it's actually within the bay area and within a few neighborhoods that are within a couple of miles of each other that, you see extremely, different outcomes, for kids and so, understanding. What's driving that variation, in our view can potentially. Provide a path to, figuring out how you increase opportunity. More broadly so. Let me now with that example. Come. Back here. And. Walk. You through some further, analysis, we've done to try to understand, what's driving that, very local variation, and ultimately, what we might be able to do from a policy perspective, to. Change outcomes and the red colored parts of the map so the. Variation, that I was showing you in Oakland occurs. Not just there but in many other cities and I'm going to focus on a different example here in Seattle, where, we're doing a bunch of work, where. This is showing you the same kind of map of upward mobility in Seattle, you see a similar pattern of a very broad spectrum of colors going, from red in the center of the city to, much greener colors in places like Normandy, Park south. Of the city alright, so. You. Know what is driving this variation, across, places, why does upward mobility differ, so much across, areas, so. I'm gonna show you a couple of results on that the, first, point I want to make is that most of the variation and upward mobility that we're seeing across these areas is caused, by, differences, in childhood. Environment, so they're really two key words there the, first is the word caused as many of you might know in social science traditionally, it's very difficult to, distinguish.
Correlation, From causation, but, my view is with these the. Would availability, of these large data sets as I'll show you we. Can make great strides and distinguishing, causation, from correlation, I'll illustrate that, in a second we think we're actually able, to show that this is about causal, effect of where you're growing up second. It's about childhood, environment, and not about, labor, markets or conditions, in adulthood, so, the way we established those findings, is by. Studying. 7 million families, that move across areas. And these data, that we're working with, rather. Than getting into the statistical, details of that study I'm gonna summarize, what we find with a very simple example let's. Imagine a set of families that move from, the Central District which as we saw in the map that I just showed you of Seattle kids, who grow up there from birth they have average, earnings and adulthood of $26,000. A year and take. A set of families that move from the Central District to Normandy, Park which was in a much bluer color on the map higher rates of upward mobility for kids who grew up there from birth so imagine, now set, of families that move between these two places with kids of different ages. Starting. With families, who move when their child is exactly, 2 years old so, what we do is take those kids and move when they're exactly to track. Them forward, 30 years using the tax data look. At how much they're earning in adulthood, and, we see that on average these kids who move at exactly, age - or earning 39 thousand dollars when. There are adults in their mid-30s all right so. That's for the kids who move when they're exactly, 2 now, let's repeat that analysis, for, kids who move when they're 3 4 5 and so on they make the exact same moves what, you can see is a very clear declining, pattern, the, later you make that move from. The Central District to Normandy Park the.
Less Of a gain you get and if, you move after, you're in your early 20s, the, relationship, is completely flat and there's no impact at all so, what do you see from this chart I think they're really Keith's three, key takeaways, the. First is that apparently where you grow up really, matters places. Have a causal effect on kids long-term, outcomes it's not just that different people live, in different places apparently, if you move a given child to, a place that's a few miles away you can see very different life. Outcomes for that child that I think is a very encouraging result, for, trying to tackle problems like, poverty, and inequality which, we've all been concerned about for decades because. It shows that the answer you know doesn't necessarily, lie and switching, to a complete, different economic, structure, like Scandinavian. Countries or something like that often. The answer can be five miles down the street where you see completely. Different prospects. Second. You see that what really seems to matter here is childhood, environment, rather, than conditions, and adulthood so things like jobs and labor markets you know obviously they matter but. You. Know where you're growing up childhood conditions seemed really central and third, you see that every, extra year of exposure, to a better childhood environment, improves. Kids outcomes, it's not just the very earliest, years that matter which is often emphasized, especially. Recently in policy circles but. Also you know if you move to a better place when you're ten instead of you're twenty there's still a substantial game, to be had so, environment, matters throughout childhood not just in the very earliest years so. How can you use these. Types of data to, actually create, policy change to increase upward, mobility so given what I've just been showing you here one, thought that might come to your mind is what if we help low-income families, move to better neighborhoods that, we see you know will potentially generate better outcomes for their kids now that's not going to be a scaleable, solution you can move everyone. But, it is actually something practical to think about on a small, scale. So. Just, to illustrate how we're, translating some of this research to policy change this. Map and we're, now showing it's a map but we're overlaying, where. Families. That receive housing vouchers, in Seattle, most. Commonly, live so we spend about forty, five billion dollars a year in the US on various, affordable, housing programs and, what. We see here is that most of those vouchers, that people get from the government are used to rent housing, in pretty, low opportunity, areas they're clustered, in the red colored parts of the map so in, light of this evidence we've. Been working, with the Seattle Housing Authority, and the Housing and Urban Development Agency in the u.s. to develop, a pilot program which we're testing using a RCT, and randomized control trial where. We're, helping, families with housing vouchers move to high opportunity areas by. Providing them information by. Recruiting, landlords, to make them more willing to rent their apartments, to these tenants and providing. Housing, broker, search services, to help you find, units. In these IRA opportunity, areas. And you, know while I don't have results. To show yet the preliminary, signs are this is working incredibly well at, essentially no incremental cost, to taxpayers you, can help families access, much, higher opportunity areas, for, their kids will do much better so this is a concrete, illustration. Of how you can take this type of research and data and translate. It to change on the ground now, I want to take a couple more minutes to discuss a. Different, direction so. You, know this is I think a solution, that's relevant for some families, but as I was saying earlier it's. Not scalable, you can do this maybe for a couple million families, but you can't move everyone so, the deeper question, is, how. Can you improve, upward mobility in the places that currently have low levels of upward mobility and so that's what, our research agenda is, centrally, focused on now and in, the coming years as a first step one, useful way to think about it is what are the characteristics, of, areas.
Where We see high levels of mobility are there certain common, features of those places and the answer is yes, they, tend to have for systemic. Characteristics, they, tend to have lower poverty, rates more. Stable, family, structures, so a larger, share of two-parent. Families. They. Tend to have higher levels of social. Capital so. Think of the old adage, that it takes a village to raise a child places. With a lot of social capital for instance Salt Lake City with the Mormon Church those, types of places tend to have high levels of mobility and then, finally, as you might expect intuitively, places, with better schools both, at the k-12 level and access to high quality higher, education tend. To have higher levels of upward mobility so this gives you some sense of the. Types of areas I. Think, we should be focusing, on it, doesn't necessarily, tell you though what, exact, interventions. You should implement in order to increase economic opportunity. And so, the direction that we're headed in is partnering. With many, cities across, America, in this case you know I'm giving you an example from Charlotte, where. We're using this opportunity Atlas, data to ask what. Types of programs what's, going on in neighborhoods, in your city where, we're seeing much better outcomes, can, we then learn something, about interventions. That might work by, you know testing, new things or looking at historical, data about inter engines that have been attempted and looking. At the, outcomes that have emerged okay. So let me wrap. Up since. I want, to make sure we have time to get to your questions by, just making one one. Final, point. Here which, is usually. We think about issues of equality of opportunity, from the perspective, of principles, of justice everyone. Should. Have a shot at the American Dream no matter what, their background is but, improving, opportunities for upward mobility I think it's important to keep in mind can also be. Important, simply for increasing, economic, growth and so to illustrate that I want to show you one final piece of data, which. Focuses. On a specific. Pathway to upward mobility it's very relevant here in Silicon Valley which is innovation and so to do that we're gonna study the lives of, 750,000. Patent, holders in the US by linking the universe of patent records to, the tax data that we used in the previous studies, that, I described, so. We'll start out with this chart here which shows you in some sense your probability, of becoming an inventor, using, patents, as a proxy I recognize, that as some limitations, but. Plotting, your probability, of having a patent, in your mid-30s by the time you're 35. Or so versus. Your parents income and you, can see that if you happen to be born to parents in the top 1% of the income distribution you're, about 10 times as likely to go on to become an inventor as if. You happen to be more into parents below the median of the income distribution, now, one, potential. Explanation for, why you see this big gap is that this is about differences in environment, and resources and schools the types of things I've been talking about here a different, explanation, is that this is about differences, in ability perhaps, the kids born to parents at the top 1% of the income distribution your. Parents had to be pretty talented in order to get there there's, genetic transmission, of ability across generations, maybe that's why you're more likely to become an inventor one. Way to distinguish, between those two explanations, is to use data on test. Scores early. In childhood as. A way to get. A rough sense of whether aptitude. Seems to matter here and so, this chart same. Y-axis. We're plotting patent. Rates but now versus, your third grade math test scores as opposed. To your, parents income and so you can see here that if you happen, to be at the top of your third grade math class you're, much more likely to go on to have a patent then, if you're below something like the 90th percentile, now, more interesting, for what I'm talking about here is if, you then split, that out looking. At kids from high-income, families, in, the green families, in the top 20% versus. Low and middle-income families, and the gray you, see a striking, pattern, which. Is that high scoring children, are much more likely to become inventors, if, they're, from high-income families if you're from a low-income family and you're at the top of your third grade math class doesn't. Do a whole lot in terms of your, odds of becoming an inventor so. To put it differently apparently. In America, in order to become an inventor you seem to need two things you need to have high quantitative, aptitude as, measured. By your 3rd grade math test and you. Need to be from a high-income family, and so you can see why from that perspective. Increasing. Equality, of opportunity, could potentially, be of benefit to all of us bringing.
These Talented, kids through the pipeline wouldn't. Just benefit them but, would potentially, benefit, all of us by increasing, the amount of innovation and so let me end by just quantifying. The the magnitude of that if. Women, minorities, and children from low-income kids were to invent as the same rate as high-income white men the. Data that I've been showing you imply that the, patent rate or the innovation rate in America would quadruple so, there's a tremendous amount of talent. And some sense being left on the table and I, think figuring, out a way to harness that talent would, be of great benefit, to those, kids and more broadly help, us restore the American dream so let me stop there thanks. Very much. We'll. Have a few questions for, me and then I'll turn to Dory and turn to the the, audience here in the in the room. So. We've got a lot, of Engineers here at Google and everybody, wants to know what, technology. Did. You use to build the. Atlas briefly. And, what. Was the most difficult part of it well what would you like to see improved. So. We. Were most focused on the statistical, analysis, right so we're doing a bunch of work internally, at government agencies Census, Bureau tax data just. Constructing, these big tables that underlie, what was visualized, in those maps we then worked with a firm, called darkhorse analytics, that does data visualization. That created. The actual, interface building, off of the mat box. Tool. And. You know I think the. Limit of how much I'll be able to talk about how, that data visualization, happened is is very, low compared, to what. All of you what, all people know about this but I think what we. What. Would we like to see improved. I mean I think we've been encouraged, by the fact that half. A million people have visited that site and various, policy makers are using it and so forth my, sense is using, that that data could be used in lots of other applications. So having it pulled, into you. Know situations, where people are searching for housing or other types of things kind of day-to-day practice, would be really, useful. The, other thing I think that we've struggled with a bit is there's a tremendous, amount of information there, lots. Of different variables lots of different ways to cut the data and so forth so how do you balance presenting. That information in, a way that. Is comprehensive. Yet. Is not overwhelming, for, the typical user and you know this is where of course Google excels, and clear. Presentation, and things like that I think thinking, about ways to do that would be really valuable, one. Thing we have, announced. A few months ago is this Google public patent database, which, actually joins together all the patent offices, in the world do you can look at the US China Japan and, and. Europe. In a unified, way and all sorts of nice cross-country. Comparisons on. The innovation. Yet mentioned but, as I looked at this as a data person myself how. Do you get kids, third, grade math scores, from, 40. Years ago yeah that's, naive, didn't, go into the deep and and what was mine but, uh. So. That specific, data, was for all kids who went to New York City Public Schools so. For a separate, study which is actually what Jacqueline was mentioning, you know showing the long-term impacts of teachers the, way we did that study it was by linking, data. For two-and-a-half million kids who went to New York City public schools between 1989, and 2009. To the tax data New, York City happened, to maintain, on tapes etc. Information. On kids test scores going way back got. That digitized, so whole process, to link everything as you can imagine. But. You know that shows you the potential of what you can do I think especially when you combine these different data sets I, just. Read a paper, a few days ago by Stephen.
Rose Who's at the Urban Institute and, it's a meta, study of looking at different measures of inequality, so. For example Paquette ian sayers who i know you know, well founded. Between 79, and 2000, to medium income growth declined. By, 8%. But. Then they, came back and visited, it this. Year piketty's, sales and Luckman showed a gain in real, median income of 33, percent when. You account for. Taxes. Transfers, price, index choices, and household size and. Mice's. Similar to many other findings. Of course the Congressional, Budget Office, and, and. Other. Groups. Have studying this and so much depends, on what exactly goes, into this, estimate. Of household. Income so. I think the figures you were showing us we're all numbers, of without, including transfers, yes just three earnings what's, your view on this how should you, account. For the existing, transfer, programs how should you count for changes. In real income household, deep the composition, as another big. Anniversary. Here we had this yeah to say I meant so my general view on questions like that how you know issues of measurement is it really depends upon what question, you're seeking to answer right so there's no globally. Correct, measure it depends upon what question you're seeking to answer so in this context, you know the fading. American dream we chose to measure earnings in, our baseline, analysis, at, a pre-tax, earnings, level, rather than including taxes. And transfers, the. Reason we did that is we felt, the notion, of the American dream at some level was the idea of. Being. Able to do better than your parents in terms of your own earnings perhaps rather than getting. Transfers, from the government, of course you could define it in different ways and then the appropriate, statistic. Would differ. I think, one thing that's clear, regardless. Of the 8% decline, or 33% increase is, that there's been much. Much larger gains, at, the top of the distribution than. In the middle of the distribution right, no doubt even. 33%. You know pales in comparison relative. To the gains that, people, in the top 1% of the top 5% of the distribution of experienced, and that. I think is very important, and connects to what I was showing you at the, beginning. On the on the fading American Dream the. Fundamental, driver of why you only have 50 percent of kids doing better than their parents today as opposed to 90 percent for, kids born in the 40s and 50s is, that. The, way in which economic growth is now distributed, is extremely. Different from the way Kannamma growth was distributed, in the past so, growth rates as you know are a little bit lower today than they were in the 1950s, and 1960s but. We show in that paper that if you had the. Same. Growth, rates that you had in the past high growth rates like we had in the 50s and 60s but divided. It, divided. That growth in the way that it's shared today across, the income distribution you. Would stem only, 1/3, of the decline, in the fraction. Of kids doing better than their parents most of the decline is, due to the fact the fact that median, wages however, you measure it have largely stagnated.
Relative. To growth at the top. So. There's this other side your, colleague Chad Jones has written a very interesting, paper about, why, we saw such increases. In productivity during. The 60s, and 70s and, this, was. Potentially. He doesn't establish this, but bye-bye simulation, it's potentially, the fact that lots of barriers broke down for women and for, african-americans. And they were able to enter the labor force dramatically. Increasing, productivity, but. At the same time they were pulled away from the, jobs as teachers and librarians and. Other low-income service, jobs so what was an opportunity, for. One group of people turned out to be a problem in terms of the community, they serve so you have to balance these two, interests. To really understand. What. Kinds, of, behaviors. Should be, improved. And I think it's very interesting I mean there might even be dynamic. Effects right so a few men imagine. You. Know people. Have argued that in the past the quality of the teaching workforce was, greater because you had many high-skilled, women who, would choose to go into teaching whereas, now they don't and, that as you noted could have potentially, contributed. To greater productivity in the 50s and 60s but. When you now think about the current generation, and you, think about the long-term impacts of teachers that may actually have adverse, effects on productivity, now. Yes, yeah one. Of the one. Of the interesting findings, that, you said when it when, a company, or when tech not high. Income people move into an area that. Displaces. Or potentially, displaces, people who are already there, on, the other hand there have been other studies from Moretti and so on that show that when you see a, high-tech. High-paying firms, move into an area five. New, jobs are created over, the course of a decade so. There's a lot of I. Suppose. I shouldn't call it trickle down but trickle across whether, you bring. Economic. Resources, in the area it tends, to impact. Most of the participants, so. I think that's absolutely right this is something we're studying. In more detail at the moment basically what are the effects, of new, company, coming in you know Amazon of course the new headquarters, things. Like that, what.
Impact Would that have on. The people who were already there, so the fundamental, advance, were able to make with the types of data I've been showing you today is that we're able to look at things longitudinally. You're able to track a given, person over time why, is that important. You might see that when a company moves in lots. Of new jobs are created etc, the places start the place starts to look much richer, in terms of the average incomes of the people who are there and so forth a lot of that could be driven by migration, right lots of new people come in lower-income, people get displaced the place looks much better but have you actually improved, the outcomes of the people who are actually there we actually I think you do not really know the answer to that what. I've been showing you here, is that least. For the kids growing up in those places, it's. Not a one-to-one thing that they capture those benefits and that's because there's so much mobility in the u.s. you, know of course lots of people are gonna move to, Silicon Valley to get the, great jobs that are here it doesn't necessarily, mean but the kids growing, up in San Jose are, gonna directly, benefit and I think we have to think hard about how you make, that, connection I think companies like Google can potentially play, a role in that thinking beyond attracting. The best town how do you cultivate the, talent in, the areas where the company is yeah and I think this, mobility. Which. You refer to Geographic, mobility has, really slowed down significantly in, the last few years it's much harder much, more expensive much rarer to, see lower-income people moving into high-income. Areas, because of course they can't afford them. On. Their social, responsibility. When Google. Was of course opening. A lot of offices, in areas. Around the US what do you think what would you recommend, or, suggest that, we could do to. Help. Be. A positive force, in the local communities, in those areas you know I think one thing we find, so, let's go back to the inventors data for a minute so one of the interesting, patterns which I didn't discuss is if, you ask you, know what is driving these sharp differences, and innovation, rates across low and high-income families a lot. Of it seems to be about exposure, so. Let, me give you the following example, if we take two kids who currently live in Boston, and let's say they're at MIT and, we. Take one of those kids grew up in Silicon Valley one, of those kids, grew up let's say in Minneapolis, which has a lot of medical device manufacturers. We. See that the kid who grew up in Minneapolis is much more likely to go on to have a patent, in medical, devices and the kid who grew up in Silicon Valley is much more like to patent in computers, more, generally, the. Chances. That you become an inventor in the field in which you innovate are greatly, influenced. By the area, in which you grow up then the environment in which you grow up and that, operates in very specific ways so when women grow, up in areas where there a lot of female and mentors in a, given field they're, much more likely to go on to innovate in that field but if they're more male and mentors in that field it has no impact at all on women's.
Innovation, Rates and so, all of that to me suggests that, what matters, is exposure. And so if you think about a company like Google going. Into a new area you. Know of course there's gonna be a lot of attraction, of top talent from other places but. Thinking about how you develop programs. So, that you connect with the kids in, local schools. You. Know you might have mentoring, programs, or other things that provide. That sort of exposure in a scalable way I actually think you know design properly, and tested carefully but these types of data could, have quite a substantial impact, and. We have done some programs like that I was. Talking actually at my bank, and the teller said, oh my gosh my kids taking, your computer, course, from Google I never even knew there was one but we've been doing it for, less, for for several years valuating, the in fact those things figuring out how to scale them would be quite entry how that can have a really big impact I think. From Saturday, morning programs after school programs, and so on one. Thing that we've been looking at lately is, future, of work issues, and, as you know there's a lot, of issues about, changing, demographics. At composition of work what kind of job opportunities, are going to be available has. Any of your work shed light on some of those issues or is that something that's for. The future I mean. So we, say two things on that one that relates to our work and just a broader reaction. So, one. Of the things we find in our work is what, seems to predict, a longer-term success, especially in the current economy is. Not just. Technical skills, I gave you an example with math test scores for instance but, more broadly if you look at so-called non-cognitive, skills so, measures of social skills how well do you get along with other discipline. And so forth those, are actually more predictive, in the general population of, longer, term success, than. Technical. Skills and I think that's going to be especially relevant, going forward as man. Is in some sense competing, with the machine it's. In the more social, kind of nebulous. Dimension. Interacting. With people and so forth where, I, think there are there big returns, to be had and so, figuring out how you provide, those skills, in, addition to technical skills or some, work showing that it's really the combination, of those two things that's. Critical for success in the long run so I think thinking about how we, education. Is not typically, structured with that in mind you traditional, systems of Education are about teaching, a certain set of technical knowledge I think figuring out how we adapt to that is is very important, my. Broader reaction. Is people, I think are rightly concerned about, the future of work but I would note that you, know a hundred years ago people, were, also extremely, concerned about the future of work and thought that you know once we had cars and all these other machines, there would be nothing to do and. I think humans are inventive, people and we will figure, out other things to do as the, economy of wolves another. Important. Point is, that the. Economic. Dinah Dinah, sysm we saw in the 60s and 70s from the entry of men and. Women into the labor force baby, boomers, and women. Who were. Being. Able to take up opportunities outside the traditional. Jobs. That's. All running in Reverse now the baby boomers are all retiring, and women's, participation in, the labor force has, leveled, out or even gone down a little bit so. What. We're seeing is a society. In most of all of countries that's really aging very rapidly, mmm-hmm and you'd expect to see maybe less, time dynamic. Behavior. Might see less support, for education, for example from, that political. Political, oscillate possibly, because it and, their big big, changes, geographically. Between, what's happening let's, say in Utah and Nevada and what in Wisconsin America. White, quite interesting areas thanks. So much for sharing beautiful, insights on missing, the data you talked about red. Pockets, in blue, areas, like the Central District in Seattle, or yeah Oakland, in California. What. Does the picture look like in. In, the. Carolinas, Georgia Alabama, that. Are largely red, what's. The notion of moving, to a better. Neighborhood, five miles yeah. Yeah, what does it look like good question, so I didn't you know I could show you a map or you could look yourself by going to this website at a place like Charlotte, or Atlanta, and you would see very much the same picture actually that I showed you in Seattle which is what I think is the encouraging, thing so when, we we've, done this work kind of zooming, in overtime, to narrower, geographies, as we've had better data so. We put out study. Comparing. Basically, cities, of the metro area level but, four years ago and Charlotte. Was ranked 50th out of 50 in that list and they got you, know they were both unhappy about that but also motivated, to try to do something about it but, at that point you, know all we could say is Salt Lake City looks much better than Charlotte was like kind of interesting, but what do you do about that right what's more interesting I think now and what we've put out a couple months ago is that, you see that even within Charlotte, there are lots of places that actually look, like you.
Know The best places in Seattle, or. Iowa. Etc, even within Charlotte, itself so it's not like entirely, a sea of red and I think that's extremely important, because it shows people in Charlotte you can look within your community, to, figure out how you potentially, expand those opportunities, elsewhere what. About the. Dory question here on who becomes. An inventor, in America. This. Company's demographic. Disparity, what would you recommend to bridge this gap between, the, socio-economic. Issues. That impact innovation. And what. We're trying to do here I mean. I think it comes back some, of it can be done at the level of a company I think some of it comes back to social issues that the government the broader public has to tackle right so, what. I will say, you know looking at this question on disparities in, demographics, one thing that comes to mind is if you don't do anything these. Things are changing but an extremely, slow rate so we see for instance if you look at gender disparities, in innovation. 14, percent of patents, in the u.s. currently go to women and that's, changing, at a rate of a quarter of a percentage, point per year, it's. Increasing, at a rate of a quarter of a percentage point four years if you just do the math that means it's going to take another 140, years to, reach gender, parity at the current rate of progress right, so. The. Question is how, do you accelerate, that I you, know at some level I feel like it's kind of a chicken and the egg problem where, if. There, were more women in. Innovation. And companies, like Google I think more girls would be inspired to pursue those. Careers, so, the question is how you get that cycle, started, I think, some of it comes back to some of the broader factors, that I was talking about on other dimensions thinking, about racial, disparities, socio-economic. Disparities, schools. The, segregation. In our neighborhoods, the lack of contact, between low and high income people things, like that I'll, be very interesting. To see what happens to schools in, New, York where when, am aware amazon is moving in yeah, there'll be a proliferation, of, private, schools of course and, be a proliferation, of, charter, schools and, of course the New, York educational, schools which are pretty good, compared. To the. National. Average, question. Here hi, I'm thanks, so much for coming to speak with us um. One. Thing that I thought was really compelling about your talk was the focus, on from Big Data to hyper local data, and how we can begin exploring those kinds. Of interesting, topics to figure out ways to change our. Economic. Ability, at. Google I'm working strategy and analytics in the human capital side so it's also interesting from there but outside of work I run, a nonprofit called, consult your community that helps college students work with small business owners to kind of focus on this, space so. For, both of you is what's, the role in local nonprofits, and community builders and helping accelerate this change from it from a different angle hmm, so. I think local, nonprofits, as you can see with these data I think they can play a huge role given that the scale of the problem appears to be fairly local. What. I think, would be really useful to do and what we're trying to do with our group opportunity, insights with these data is connect, with lots of nonprofits, like, Big Brothers Big Sisters other, groups like that that are doing things and mentoring or racial. Issues criminal, justice etc, to. Try to evaluate more, systematically. What, has the, most significant, impacts in the long run right I feel like there's. A bit of a disconnect between lots, of people who are doing a really good work on the ground, where. They have a sense that this might be working but you know how do we scale that how do we think about in a cost-effective, way which aspects, of different programs, are successful. I think these sorts of data can be useful and figuring that out and, there's a disconnect, between those people on the ground and researchers. Who, may not be as aware of the various programs that people are crying and so forth so one of the things we're trying to do is is bring. Those things together and hopefully I have more to say about it in, the coming years. What. About this, question on the correlation. Causation issue. I know. Positiveness. Might be parents. Who reduce and the kids might move earlier, yep and by the way as you know there are studies of immigration, where immigrants, tend to be highly motivated people who make, decisions that, move, and.
Is, It really, the immigration, per se or is it those characteristics. Of being highly positive, so this is a great question gets it kind of the heart of the statistical, issues and in the paper. This. Is this is referring to the graph I showed where the earlier you move to you know Normandy, Park and that example versus the Central District if you appear to have better outcomes and adulthood so natural, concern you might have maybe. The people who move when they're kids or younger are more, motivated, they're more educated maybe they're different in various ways that's, why their kids are more in adulthood not because they're growing up at Normandy Park that would be a natural confound. We would worry about give, you a sense of how we can deal with that kind of issue so. With the amount of data you have you, compared, siblings, within the same family the, way we would talk about that now regression. Context, is put in family, fixed effects so, you're only comparing, kids within the same family and so, effectively, what you're doing there is asking suppose I moved to Normandy Park with. A six, year old and a 12 year old I now, compared their outcomes, what, is remarkable is you get exactly, the same chart back if you, only rely on within family comparisons, so in other words your, younger kid does better than your older kid exactly, in proportion to the age gap between the two kids so, if you think about you know could, it be a confound, that eliminates, a lot of possibilities, that it's about different families, who are moving when their kids are young versus. Old etc. And so if we do a bunch of other things along those lines including. Actually study a pure randomized, experiment, called the moving, to opportunity experiment. Where housing, vouchers were randomly assigned and we showed. That you get this pattern so I think it's for, quite convinced, that this really seems to be a causal effect excellent. Jackie. Yeah. I'm, wondering what you think the role is of government, policy, in creating some of these low opportunity, neighborhoods, so, for example a lot of I think high concentrated. Poverty communities are, housing projects, where government, policy requires you, not to have two parents, and. It requires there to be you know these high percentage, of exactly. The opposite of all the things you said are important, low social. Capital and all of that so. The. Role of government, policy and/or. Market. Policy, for example redlining, districts, and not allowing people to move the opportunity, yeah yeah. I think government. Policies. Inadvertently. Perhaps and, historically. Perhaps. By design have. Led to tremendous segregation. That have basically made it hard for people to access opportunities. Let me give you two concrete. Examples of that that we are thinking about so. As I, was saying earlier we spent forty five billion dollars a year on affordable housing in the u.s. you. Would think the intention, of that is to try to provide people access to housing that will give them better opportunities. In practice. I think unfortunately. Much. Of that expenditure is working in the opposite direction so I gave you one example with, the housing vouchers where, for whatever reason, families are using these vouchers to live in pretty low opportunity, areas there by basically exacerbating. The problem of a. Lack, of intergenerational, mobility a different, example of that which, gets to government policy design something. Called the low-income housing, tax credit. Where we spend about 6 billion a year giving. Developers tax, credits, to, develop affordable housing but the way that program is structured is we give you bigger incentives, if you develop housing and lower in, high poverty, would, end up being relatively. Low opportunity, areas so, we're basically, concentrating. Poverty, further if, you overlay, where these light tech developments, are on our maps they're, all in very low opportunity, places and so, I think that is you, know unless you think those developments, are gonna change the level of opportunity in that area which is something.
One Can look into you. Might actually be exacerbating. The problem through. The design that policy another example, which will be familiar for. Folks here in Silicon Valley is, zoning. And you, know the ability to build housing, right so, ultimately, you can move people around but supply, is a fundamental, constraint, like if you can't build any housing, in Silicon. Valley area. That's. Going to prevent people from being able to live in places where their kids would do very well. There. They're serious, political, economy, problems there where if you already own a house in Silicon, Valley you might not want to allow vote. For more building and so forth but I think figuring, out how, you get around the zoning restrictions so, you have more density of development more. Mixed income communities, in another area where one can have huge impact, I. Mean. Actually. We talked about this first question here, already. Before, the meeting but why. Don't they this is Suzann popkins, research, suggests, adverse effects. For. Children due to the disruption, that's caused by moving. Yep, so that's a how. Do you view that and, turns out this prescription. Yeah. So what. The way I would think about the moving idea, is not that we're gonna uproot, families. That. Are happily, living in a place and ask, them to move somewhere else the way this actually works for instance the Seattle, experiment. I was describing to you is, we take a set, of, families. That have come into the Housing. Authority they're looking to move 20, percent of low-income families, move anyway, every year and I think about it as kind of redirecting. The flows you've decided to move you're gonna move somewhere let's. Give you the information and support to potentially move to a place where your kids will thrive not. Let's go find somebody who doesn't want to move and encourage, them to move so that's the way I think about this issue kind, of in a narrow sense but the broader question, is how. Can you revitalize, communities which, of course is, the big, issue. And, some of it you might think about the factors that I was describing.
Related. To changing. The quality of schools trying, to have better teachers you. Think about things like social capital and family structure those things might be very important but how do we change them that's. A really difficult question so the best I can say there is we, are trying to now use these data to study, various, community, revitalization efforts. That, have been conducted over the past 20 years or so by, the government, by local nonprofits, by developers, but, we're building a huge database of all such efforts around the United States and then, evaluate. With this longitudinal data what. Are the characteristics, of the things that seem to have worked and things that haven't, and that's our hope of getting a scientific, answer that's. Excellent. Well our time, is up but thank you so much for coming. You. You.
2019-01-31 15:00
Someone is using a microchip to control my girlfriend. It's been a month and the controller seems to be relentless in using her to affect my actions and nothing is changing. Somebody please help!
If your parents don't have children, chances are you won't have any, either. - j q t -
Inspiring Story.
Given the span of time shouldn't income gets adjusted based on tax, inflation, purchasing power, subsidies, family size etc. For example, there are more single parent household now, who's on government support, whom gets tax benefits, and could theoretically buy more products using the same amount of money.
this is correlation he doesn't know this
Income
"If women, minorities, and children from low-income families invent at the same rate as high-income white men, the innovation rate in America would quadruple" And if trees, street lights and trash bins invent at the same rate, the innovation would quindecuple.
What happened to segregation at 23:10 compared to his previous points almost 2 years earlier (https://www.youtube.com/watch?v=m05NeaG3d2A&t=340s)?
"Family" it is a father a mother and one or more kids. Conform with the facts...