Panel discussion - Pittsburgh ML Summit ‘19
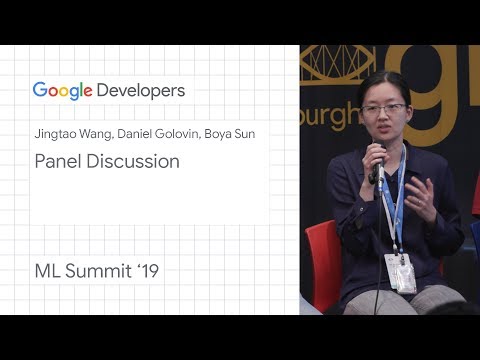
Hi, everyone, this, is Jean hawa I joined, Google last. May before, that I was a professor at the University of Pittsburgh, my, primary interests, include, on device, machine learning and AI in education, I'm also, interested in using, machine, learning to so reward, problems, and this is what I'm working on nowadays. Hi. Everyone my, name is boa I've, been working at Google for more, than seven years before. That I was a PhD, student doing, data. Mining application, for software testing, the, work I'm doing here is also, kind of similar, issue because I'm, not really a ml researcher, but our team is kind. Of utilizing, ml and natural. Language understanding for, solving a lot of problems specifically. Our team is working on, shopping-cart, understanding. So we do use, marshaling. Technology, to help us understand, what people are talking about when. They're when they want to have a shopping tent and, we also use a lot of data mining technologies, to discover, new. Shopping concepts, from from large scale large, amount, of text so in. General very excited, about ml, and we have a lot of very close conversions, with research. Teams at Google. Thanks. Hi everyone I'm Dana Donovan I work. In the brain team here in Pittsburgh I, started. Out so I have a background in theoretical computer science I came to Google I started working in ads learned about very large scale machine learning. Here. Working for a couple years and then started trying to automate automate, away, large. Parts of my job which selectively entually led me to work on things like busier these days I spend a lot of time working on machine, learning driven, optimization. And. I. Enjoy. Working with a broad range of cool, teams really, across across, all the Google, trying. To make their systems, and products better. Cool, thank, you very much and so. Anybody, want to jump, in any. Questions, right off the bat, because. I can kick, one off just to get us started so. This is for anybody on the panel where, do you see. Machine. Learning an AI. In, the next five years, and. Then the next ten years. It's. A kicker right off the bat I know. I'll. Take an overrun answers here no wrong answers. Well. It's it's kind. Of precarious. To predict that far out given how quickly the field is moving. But. I I see a lot of work on. You. Know I think the relationship, between people. And a lot of these systems will change a bit where a. Lot of the computer systems what we call more partners, in creating. Things, I. Noticed. A lot of the work on like, generative, adversarial, networks are kind of interesting in terms of giving machines a, creative, sense there's. Already some interesting work on I.
Mean. You see it in things like video games where you have systems, try to synthesize entire, environments. You. Can try to do that based on various conditioning, you. Can imagine these. Systems, kind of partnering with people in terms of designing. Various. Things and. Kind. Of optimizing, them in various dimensions and, improving. Their performance I think we'll see a lot more of that. Yeah. I think I'll, stop there. I think, so. I this, is you. Know predicting, the future but yeah I I don't think like I'm, reading the position of doing that but like from my work experience I think it's definitely. Martial. Art Ernie is going to. Start. Using more and more and more data so like that scale Billy it's just really important. And. A lot of these new kind of new, state of the art I'm, almost like like Bert models it's really, scaling. To a twist to a degree like a magnet, of order of order, magnitude bigger, than than what we have in the past so I think alongside, of, like utilizing. Much, more, and more data there's, also I, think, a, lot of hardware side development, is going to make. Much more improvements, to be able to have. Much larger, scale. And. A lot. Of compute computing, power to support such kind of such kind of scale. All. Right here are some of my personal opinions, on what's important. Within, the next few years just personal, opinions first, I see, there, should be a shift, from. Benchmark, driven tasks, to requirement, even tap two-and tasks when, I say benchmark, doing, for example object, detection, object. Classification or, blue, scope is the machine translation these. Are well-defined, tasks. After. Certain. The. Technology, improve on those tasks will be shifting, Maude was recorded driven. Tasks, to him by viewer requirement, rather than a will, defund Marable. Metric this, is one scene announcing. Is about making, machine, learning more practical. We have seen the power of big data but. What if small. Data not, every task had big data in. My situation with me to so important, problems. That only have a small amount of data mean, well such. Problem are so important. And people. Will try their, best to. Attack. Your system to. Generate other, words your input, how, should we give, that our model, to be more robust, in a small data set. And be is your bus to the water'll input, it'll be really important, the sourcing I believe that's extremely important, is I believe, nowadays. When. The machine learning algorithm is really good at perception. Tasks, when. I say perception, tasks it was there, was a definition by Andrew, in that's. The task, frizam but our human can complete in Wow two seconds, but. There is no way for example, for the current algorithm to, do something, that's reversible, reasoning.
As Simple. As counting, for. Example tell me how many dogs are there in. This picture this. Is a trivial task for. Human, but we still cannot do that reliably. With, today's algorithm. Similarly learn. An algorithm, to be able to. Answer. Questions that a plus B equals to C again. This, is more than, perception. It'll require some level of. Logical. Reasoning and I believe that would be extremely, important, to push today's, algorithm. To the next level to, address some, of the really important, tasks we are facing. To. Teach. I wasn't, how to count. So. It looks like we have a question over there. Yeah raise. Your hand and we'll run a mic to you anybody. It's. Basically the same question but fall off if you would put you, know number, two integer. Number how many years, till we get human, level of intelligence, your personal opinion. Got. To think about that one. Yeah. Uh so I don't know exactly how I would answer. This because well. It's. It's not such a well-defined, problem right like we already have think. It depends on what aspects like what human capabilities, you consider, to be important, right so, in many aspects, mm-hmm. To. Do anything a five-year-old can do. Yeah. I I mean it's the uncertainty is so great right. I don't. Know. Fifty, years. Can. Really just get back to this like in 50 years and see what's right. I. Think. It's gonna be faster than we think. I. Think if you go back to ten years if you think about autonomous. Cars it's not something, that people would imagine but. Like the, robot. Industry has been improving, in a much faster speed than anyone could imagine I think it's, gonna be really, faster. Than anyone. Else would expect, so I would from. That logic I would guess, for. Eight years. My. Estimation. Is to. Achieve their goal we, need another huge, bricks roll just, like what we had in terms of deep neural network, if, neural network itself is not enough we need something, as this level another, one that. Can complement, what we have in. Deep, neural network, and it's, hard to predict, what, that pricks. Through is and how long it would take it. Can be as. Soon. As five years ten years it can take longer but. Personally, I think I'm a little bit optimistic maybe. Twenty. Years not, fifty years sometimes, if we are lucky it. Can be five. Years ten years we. Just need another bricks. Roll. It's really, hard to time such a brief snow and named such a brief break through. So. I'm, excited, about autonomous. Cars and my little. Robot lawnmower, has saved me a lot of time but. Not everyone, is, so excited and I want, to ask about one thing I'm a little bit concerned about is, automated. You know stock, trading and how, we can put some policy, or regulatory guidelines and, from, a security aspect both policy, and and cybersecurity. What, kind of thoughts do you guys have on the kind of cutting edge of both. Things I'm excited about and things, that I worry about myself. So. I can talk about one thing that I am, you, know slightly. Worried about you know development. Of AI in particular I just. Feel like one thing that distinguish. Human. And machine is the creativity so. I think we can definitely utilize machines. To help do a lot, of things that need. Large scale like computations a, comma things that kind of exceeds human capability, but they are they are largely repetitive, in my mind but. One. Thing worries me is is the you know you teach machine to be creative teaching them to, you. Know there's lot of research. Going on for teaching machine to create, artwork and creates music right, there are even things that create, create. Fictional. Actors. That can play in movie, so, those, type of things kind, of is something. That then I'm worried about like if if machine is starting to get at the same level creative creativity. As its humans I, you. Know that's kind of a word that that scares me. So, I'm, not sure I quite understood the question, it's you. Wanted like you asked one question about what. Policy. Guardrails. You'd put around algorithmic, trading. For. Example. Well. I, mean. For that I guess, it. Depends on, in. Some sense that's a political. Question right. You. Know the the algorithm that create trading, it just enables people to make these trades trades much faster.
So. You. Might say well okay we want to make sure that that people are in the loop so they can make sane decisions. That's. Fine but like collectives, of human beings can also make rather. Irrational. Decisions right like markets, can be irrational even, if individual, people are very, like you. Know the, famous tulip crash of. You. Know that. Centuries. Ago in the, Netherlands right. So. I guess, I would probably recommend similar, policies, that, prevent, those sort of crashes, in, an algorithmic age we just have to maybe. Automate, the policy enforcement in some way so if they can ruin so that the policy can respond the same time scales as the algorithms. Right. But I'm not sure that that these present kind of fundamentally, new challenges. Hi. What, new sorts of jobs do you see coming out of any. Sort, of AI revolution. In the future. I see, this like, as, a huge, opportunity, its. Opportunity. As big as the invention, of auto mobile, or, airplane. All her, mobile made us much, easier, to go from point A to point B. Aircraft. Made us even, faster, to make a lot of international business travel, possible. The, current revolution, in AI made. It possible to so, many of the perception, tasks, that, was not, possible to so eighty, years ago something. As simple, as a, real. Human that can so in two second but, the computers, just couldn't solve it eighty years ago now. Those things become possible I can. Imagine that a lot of things for, example that, couldn't. Be automated. Will be automated, that. There. Are a lot of things that for example computers. Has placed. Zeros all in. The past the, primary contribution about, computers, is mostly, about storage. About. Automation. It's about. Connectivity. Now. We can so some of the perception, based at us I can, imagine that a lot of for example opportunities. Will be created, in. Manufacturing. In farming, in. Animal. Rising, etc, and. We. Can significant, increase, the scale, can. Wear something that. Was. Not scalable, to things that are, scalable with the help of post algorithm, and some, of the administrators, so. I see this more like a great opportunity, it's. More about asking, us with. The power, of human. One, second human perception, what. Can we do with computers, now we. Just opened a new door and, we haven't explored, sufficiently. Enough it may, take us maybe five, to ten years to figure, the additional, power of one, second perception, what. It can contribute to our community. Yeah. So it's, an interesting question you know. It's kind of again it's hard to predict exactly how the economy, will evolve, over time. You. Know if you study, things, like the Industrial Revolution or. Electric thing like, when electricity was introduced it. Actually took a fair amount of time for people to, kind. Of reorganize, society in ways that actually like, utilized, the surplus appropriately. Right, the, Industrial Revolution kind. Of made everybody wealthier. But. It took a while like, about, 40 years in that case in the case of electricity, usage, it. Took essentially, a new generation to realize that they could redesign, factories. In order to leverage, electricity. Effectively. So. I think we've gotten better at these things we are our society, adapts much more rapidly than than, the Society, of you, know 150, years ago say. But. It's it's still kind of difficult to predict in. The short-term obviously. It helps to have you. Know there are there are jobs actually building these systems and making them more efficient and kind of just increasing, productivity in general, so.
If You have the skills to like, be a developer, that certainly helps, longer, term it kind, of depends on what people value right, so a, few. Like, you, know back this past summer I went an art fair and there. Are a bunch of people who were making, handcrafted. You. Know handcrafted, sculptures or paintings, or other things and selling, them to other people right, and you might ask well you know why do you want a handmade. Wooden, chair right. Like is it really better, quality, than what you can buy and like, some. Masked-man manufactured. You know furniture store and, like. Well in objectively. Like, you, know you can sit on both of them but people still sort of value with finely, handcrafted. Chair or the you know hand-painted, painting. So, maybe, the future looks like that where people there's there's, a large, artisanal, class. That's, just churning. Out kind of interesting, art and music, and so on. But it, is kind of hard to tell exactly how, things will develop. Okay. So this question is for Daniel so as a member of Google. Brain Pittsburgh, so, it's two sub questions how, is the interaction, collaboration with, like top, ml. People in the world like Jeff Dean and Jeffery Hilton and, also. How, is, the interaction. With Google, brain and deep mind. Yes, so the brain team is fairly large now. You. Know I certainly, enjoy, interacting. With. Jeff. And Jeff. Sieh. But. I mean there is a it's a large group there are lots of projects going on. I, think that there's there's a wide range of people that do fundamental, research and, work, on kind. Of the, underlying technology, right, so some people are very focused on kind. Of foundational, research and, well. All aspects of deep, learning and machine learning and others, are working on tensorflow, other. People are working on my. Group is working on some of these core, technologies, around black box optimization, and contextual, bandits and reinforcement, learning both. On the research side and on the infrastructure side, so. I think it's it's it's a great I mean, it's a great group to be and I. Feel. Very privileged, to be part of that particular group in. Terms of the interactions with deep mind you. Know we do like my group actually does work with or collaborate with some folks in deep mind. There, are various, connections it. Is true, that geography. It's. Easier, to collaborate with people that, are in your time zone or at least a similar time zone so. The fact certainly. Between California. And London there's quite a bit of a difference in time zones and I think that that that does make. It a little bit more difficult to collaborate. That's. Less, of an issue for us here, on the you know in Pittsburgh on the in Eastern Time. But, yeah the the D mine does retain its own kind of identity they have their own research agenda. But, I think that both groups work together nicely. So. A, question, from here. This. Question is kind of follow-up from dr., Wong's just. Mentioned a one sec, perception. So. I, think a big issue in, human-computer, interaction. Is the perception. How people. Perceive. These AI technologies. And, and a, big issue was the trust, issue, people. People. Usually doesn't, trust those, system, part, of is because, the, system was not that reliable. And, sometimes it broken, up or it, performs. And, unpredictably. And, also. Is that there, as you, said there needs a revolution of. Changing, people's. Perception. Towards, these machines. And, they. For. Example a, chat, bar a conversational. Agent so, people. When, they are using. The conversational, agent do they really, trust the results the, the agent. Back, to them so. So. I guess my question is. We. Have the technologies. Here, and a can. Words. It. Works and in some, logic, but, the. Humans perception.
Might, Comes, later and, how, do. You connect. The humans perception, into our, building. Of this technology, and, how do you influence humans. Life in, this way. This. Is a excellent, question I would try to answer it, from multiple, aspect, the first one is. In. Order to make the algorithms, more trustworthy, we have a lot of things to do for example most, of the people who. Are treated as a black. Box with. Hundreds. Of millions of parameters. Y-l, generate, prediction. A versus, prediction. B, without. A clear, and reasonable. Explanation. It's hard for human, to trust, the output. We. Also believe this is extremely important. See that. May. Prevent, the adoption of machine. Learning algorithms, and in the research community we call that interpretable. Machine, learning. Interpreter. Given and this is a emerging. And extremely, hot direction, and we. Are making progress in, terms of understanding the, output. In. Deep. Neural networks and in, many situations such as finance. Healthcare. There, law regulations. Requiring, for, example we, have a, explanation. About. Certain, predictions, for example if we predict, the. Patient has a 90 percent chance, to. Have a lung, cancer. You. Cannot just, output. Lung cancer, 90. Percent you, need to give specific reasons why. You. Make such a new prediction without that. The. Patient won't believe you their. Family members won't believe you doctors, wouldn't believe you either, this. Is one thing announcing. In terms of trust I would, say. It's. Also it's. About the weakness. About, our human, being in, many situation is not about not, enough, trust, in, many. Solution is about, too, much trust. Especially. If. Something. Is the phrased, in. A way that. Could. Listen. It your fear, uncertainty and, doubt, II. If that specific, output. Or message makes, no sense a human. Being have the nature to trust, that because, just, because such a message elicited. Fear, uncertainty and, a doubt, it's. Build into our DNA and. How. Can, we make for example. Information. Sauce, more, reliable, how, can, we for example. Make. Sure that people, are thoughtful, about certain, messages. Fake. News is another. Thing we really need to pay attention to within the next couple years again. Each of them is a big problem and require a huge amount of efforts, too so. I'll. Add a little bit of color there there. Are a number of efforts to try to make. The results, of models more trustworthy. You. Know that there's an enormous amount of work on model interpretability and various approaches there in making. Models, kind, of forcing them to explain why they reach certain conclusions, they. Adding sort of certain, constraints, to how. They make predictions, or what, their outputs look like. And. Just. In general like tools to kind of deeply inspect what the models are actually learning and. So. I think that this is an important line of work and will continue. It's. Kind of funny in some areas, of AI there's. Kind of a natural tendency for, certain people the hype things and then some people buy into the hype and actually like trust too much and other, scenarios, people really trust too little and actually prevents, like. Systems. That could actually do a lot of good from being deployed. But. Like. Certainly, so, for example in Google there's a large, organization. Focused on, like. AI for medical, applications and they, are painfully. Aware of the, need to kind. Of make these predictions in a safe and reliable way and ground it in like good medicine, in a ways that the physicians can understand, so there's a lot of interesting work on ok. Like are you going if you diagnose, like a lung, tumor or something from some image can, you actually highlight. Exactly which pixels you think are the evidence for, a, tumor right, and then you can get like these super - high-resolution. Scans, in. Some cases and instead. Of having some doctor, kind of carefully. Scan through the whole thing, you can just sort of highlight in red like these are the problematic pixels, and then they can very easily spot check the machines predictions, so. It's. Definitely a very active area. Okay. As. Ml. Is being applied to problems, and all sorts of you know new and novel domains, what, problem, do you think it has the best chance, at addressing. In, like the, next five years. I. Mean. So. Some. People have compared like AI, or machine learning the state these days to something like electricity, all. Right when you introduce electricity, in society.
It's. Difficult to say like if someone asked okay you know what is the one thing electricity. Will solve the next five years it's. Kind of difficult to come up with a single answer I think. A lot of these things are there's a gradual evolution alright there's an evolution of the technology, there's an evolution, of the industries, and how they use the technology and. And. So it's, and. And and this is happening in a lot of different areas of society simultaneously. So. It's it's difficult and also when there are solutions it's. Not as simple as you, know sprinkle, some ml dust. And the problem goes away right, it's usually a concerted effort that involves. Careful. Engineering, careful. Policy, planning, various. Other aspects, and kind. Of AI and machine learning are part, of the solution they're not the entirety of the solution. So. In that sense I, don't. Know if I can give an answer about a particular, problem also. These systems often make things better, as. Opposed to just going from no. Solution, to solution. But. You. Know we see a lot of work on. AI, e solutions. Are getting better and better at modeling. You. Know you do better models for climate science you better models, for, materials. Design you. Can build various things maybe you can make better. Batteries. Using. These things so I'm interested in like using, machine learning for design so one, of my answers are skewing, in this direction but, if you think about things like climate change or making move. To more sustainable energy sources, there, are a number of areas where ml and I can, help there in terms of accept the climate modeling, designing. Better batteries, designing, better solar panels all of this other work and. I and I imagine that there are a lot of other kind of areas where you can make a similar kind of story about these. Technologies, kind of making. Gradual. Improvements, in, various, industries. Personally, I'm extremely optimistic about. What. AI can do within the next five years on one hand there, are some low-hanging, fruits. Such. As leveraging.
Those Kind of one second, perception, power there, will be a lot of opportunities. In those factories. To, automate. For example, inspection. Assembly, etc, nowadays many of those tasks require. Workers. It, can be automated, those, are some of the low-hanging fruit. In addition to low-hanging fruit I like to point announcing, that's not relevant to technology, it's. Relevant, to, people's. Belief, I, believe. For example to. Make any. Change, in the future it's, not just, as simple, as inventing. The technology it's. The combination of, Technology. Policy, and. Experiments. In. For, example 30 years ago, even. If you believe something is possible especially, in, the enterprise environment. A lot. Of freedom, real, problems. In, the large, enterprise environment, can. Be sold, with. Today's technology, but. We didn't give them a chance because, we didn't believe, it, it was so low but. Nowadays with the technology, rickshaw, I think there, were a lot of men style change. Give. Us additional. Opportunities. To try, to sow some of the easy, real-world. Problems. Those. Problem, was so low in the past but we, may not have the confidence to use, a machine, learning based approach to so that and. With. Increased. Confidence we, think that a lot, of those, problem. May be solved, especially, in the. Enterprise. Computing, environment. I think those are great answers I learned a lot so. I. Think, this is like as Daniel said this is extremely hard to predict but I do think there there's a large chance of low-hanging fruits for places, where first. Of all the the task, is not super hard to define that, you can concretely define, this task as a machine learning task and the, second thing is that the. Place where we have huge amount of data already, existing, so. You, know large amount of text webpages books, historical. Data. Of any sorts this. Book places where I have a huge. Amount of data. Like. It, seems. Like those are the that place that we can make easy wings. Cool. And in the interest, of time we'll have three more questions, the. Panelist will be available, after, at. The happy, hour but, kicking. Off with the next questioner here. Set. A question I'm, not an, expert on quantum computing. But I'll just gonna, ask it seems like those. Types of circuits are really good at solving you, know optimization. Problems, and minimization, problems and I. Believe, that's sort, of the underlying logic, or, math behind all, machine, learning is gradient. Descent in some, form so. Do you know of any like efforts, or what the progress is on maybe. Building, an analogous. Algorithm. To SGD. Or. Yeah. And for, like a quantum circuit. So, Google does have a quantum. AI team, and. There, are efforts to build a quantum computer. Trying. To think, think. They're they're trying to build like a 53. Qubit machine, so it's still pretty early days. In. Principle. Like. So. Nobody's actually built like a pragmatic, quantum, computer yet one that can actually solve practical, problems better, than a real like. A classical, computer. In. Theory. If. You can get the quantum error correction good, enough I mean you need some more breakthroughs as, at, least to. My understanding before you can build these things practically. But. Yes in it, with certain, breakthroughs, in theory, it should be possible to build machines that can solve certain optimization, problems very efficiently, and then. There's a question of can you leverage that for, various. AI applications there. Are definitely people that are looking closely at that but, I would say it's far. Enough out that. I. Haven't. Been personally, following the developments, there. Personally. I know it's really little about quantum, computing but, I like to add some original. Points, from a different perspective, essentially.
Speaking Our computing, power is, bounded. By online, physical, laws, there. Is no way to believe, that our current, computer, architecture, the, twin machine the von Neumann, architecture is, already, the optimal. Solution, for any problem we are facing there, must be unlived. Solutions, for, certain tasks we are facing I do, not know what it is maybe condemned computing, is why there, could be other alternative, computers, for. Example a, bird. With a small, brain, can, already. Consume. Little power when compared, with a large, GPU, or Takeo generated. A slack object, recognition. Image. Recognition pretty. Well with. A print of three gram this, is something, we definitely couldn't do especially. When compared with weight, when compared with power. It means. That there, exists, some alternative, solution, for example. By. Using DNA. Programming protein, programming, that, can achieve the same tasks more, efficiently so, I. Do not, believe the. Twin version is the optimal, solution for all. The problems there. Must be more, efficient, solutions, and we, shouldn't, stop. Looking, at those alternative. Solutions at any time. Thank. You. With. With. Our. Personal. Profiles becoming, more, available to any buyer and. Considering. The volatility, of the social structure across the world, what, is Google doing to. Actually. Maintain. SiC. Our data the user security and. I. All person, in favor of regulations, for when it comes to our data. This. Is again a pretty, important. Big question, how, to see that. I'm. Not an expert on that but I know Google. Treat, those security. Privacy, problem. Extremely. Seriously, we. Just, created. Something. Called air, principles. We. Have a, designated, committee, in, terms of reviewing each. Upcoming. And I project wiser, for example. It conforms, to the AI principles, in addition to that who, has dedicated, privacy. And security review. Teams for, every, new project in Google we. Need to drive a privacy. Design talk security. Review talk and get healed, and the, approved. In, order to proceed, and, who. Will really take, those kind of security, privacy. Extremely. Seriously. So, I'm adding, a bit to that so um as. A workspace, Google. Is trying to really. Build this very inclusive culture, that. In inclusive. And diversity among. The work workspace.
And, I think Google is trying to apply the similar principles, to our ml. And AI solutions, so, trying, to basically build AI solutions, that's benefit. Everyone and not bias to certain, group of users. Yeah, so I, guess, I'll add a little, bit more. There. Are pretty. Significant, security and privacy reviews, for all products, there, are significant. Efforts. That protect user. Data. In. I mean there's. Also all, kinds of additional rules for various countries. Like GDP our compliance. Is a big issue where. They're, like. There's large infrastructure, in place for auditing and tracking exactly, internally, what, data is being used where, and, making sure that it's not, retained longer than it should be, are not retained you. Know in places where it shouldn't be and so on. You. Know Google takes this enormously. Seriously. There's. I. Mean. Is, something, she was like Google+, was shut down, and. I'm. Not like privy exactly to their reasons there but I imagine. That some part, of that was to make some of these compliance issues easier. And. In. Terms of kind. Of what's Google doing and in terms of trying. To protect the social fabric I think, you. Know. We. Have a vested interest in making sure that that everyone behaves responsibly, in sort of the the ecosystem, we don't want people to feel. That they're, being. Taken advantage of. It's. A it's a challenging. Project problem. In general, in. Terms of like the the people who are aggregating, profiles of users and buy and buy and selling them are, typically companies you've never heard of right, it's not the bait. Companies. That are kind of interacting. Directly with consumers. Yeah. I mean I personally I, would be in favor of regulations. I would protect people's privacy in a lot of these circumstances I, think it is problematic. But. It's I don't think it's actually, a lot of it's, not Google that's doing these things Google is not selling, anybody's, information. To. Third, parties to build profiles. Thank. You okay, so it's kind of fun question okay, so we all engineers, here most of us I engineers, and be thinking about things exact science right like how precise, is it how good is it you know how much how big you know new. Materials, how good is this materials this is how we looking at this new system this autonomous. Karlie is it able to drive me certain distance and this is towards questions of IQ, right but there is also EQ, emotional. Intelligence. Right, and there. Is a sci-fi. There. Is some stories in sci-fi like my favorite one is her, right, there, is the agent, Samantha, it's, a you know, artificial. Intelligent agent and person. Is able to develop feelings towards. This agent, right, so you feel, something, towards it and it's possible to do there, was another project actually your project, guys it was Google, duplex, right that, people actually believe, that they're talking to human being and at, the moment then they felt that you know it's a artificial, intelligence, they felt tricked and you know they have a certain push back because. They felt like they've been relayed. To something that's not real they feel like they've been lie upon to but, maybe, it's not supposed to be like that because there is social robotics. Right there was Robert, GE but there is a robot Kiki which is still active there. Is huge movement, and you know Japan Japan. What is it wife oh I think it's called why for the, hologram, that you can talk to and develop certain feelings towards it so first. Of all how do you feel about that, right and second, if you would want to solve this problem how, would you frame this problem is it supervised, learning is, that reinforcement, learning how, would you do. I like. To answer this question first because I did, have some experiences, with, your question the, problem you guys computers. Folks more about IQ. Rather, than EQ is true but, it doesn't mean that researchers. Haven't thought about the, EQ problem, in the past there, is a dedicated field. Called, affective, computing, it's. All about trying to understand. What's. The feeling of a, user, because.
Unlined, Assumption, is we, spend. Maybe six. Hours eight hours some people even 10 hours with, different computing device, devices. Is totally, wrong that. The. Community wise we, are using has no. Information, about our feeling, do. Not know whether we are happy, we are angry etc, this, is totally wrong, we. Do not take those things into account twenty, years ago for a reason, but, now it's a right time to, take. Those things, into account either, as a feedback mechanism or, as implicit, input. Also. I would say this is the right time to, introduce. Effective, computing, because. In the past how. Could we know users, feeling we. Can tell you those feeling from facial, expressions. Body. Gestures. And maybe. Physiological. Signals such, as, skin. Conductance, such. As blood, pressure such, as heart. Rate etc, in. The past maybe more than ten years ago such, kind of physiological. Signals are extremely, hard to Merril we chimera that but only in lab environment, but, with more. Recent technology. Advances. Such as wearable, computing, wises, small. Low-power. Sensors. And. Better. Own, device, machine learning algorithms, we, are. Reaching. A new era that we can collect, and monitor, and make inferences from, those physiological signals. And to, help us to know a little better about. Our feeling, the, EQ, part of users. I already. Did some research, in, this area in the past my approach was to use a sensorless, approach, just. Use a unmodified. Smartphone, what, kind of signals we can collect from, user, interactions. The. Answer is we can click a lot for, example we can collect. Users. Muscle, stiffness signal, from, their schooling, screens coding activities, we can collect for example harvest signal from, the skin, transparency. Change we, can also collect. With sample emotional. Feelings. From the facial. Expressions, we can do a lot of things what, I did in my previous research, project, was to use those as signals. To understand, the learning process, to make the learning process, more efficient, but. I believe, that there. Are a lot of other opportunities and, there, are also a lot of other researchers, working, on that, we. Are reaching. The tipping point of, making. The. Computers. More emotion, aware improving. The EQ of computers, with today's, technology. With more recent, technology. Pricks row especially, breakthrough. Single, candle portable, low-power, sensors. So. Your, first question was how do we personally, how do we kind of personal feel about you. Thank. You the, first one I think I I, think in general I feel like it's a positive thing that computers, can can have emotions like for example if you have there's. Possibly, going to be more and more agent, type of things that you're gonna have actual, dialogues with people helping out with, solving, any issues they have in life it's, actually in general good, if you feel like you're talking with a person rather than a machine like, my, daughter I should really enjoy talking, to Siri because Siri tail tells a lot of drugs. For. Second thing I don't, think it's a matter of that computers. Are good with IQ type a problem but not with EQ type of problem, I think this is more kind of a new area that we haven't been dive. Into yet I do think there's a lot of potential for that like for example we do have research. Areas, such like sentiment. Analysis, like basically giving, you a picture, forecast, is this cat cute or not right that's kind of really, predicting, your how you feel about that picture right there, also kind of medical research for example like why, are some people more, agile. More easier educated than the others there are actually medical things so you can actually trace back to their, structure.
In Their brain that different from from you, can find traces on at. The medical level um you, know trying to refine find out that the relationship between, feelings. And also, you. Know how that actually physically, works in your body right so, I do think these are tractable. Problems, but, it is a new. New, coupling, new area of research that I think, is gonna have, a huge potentials in the future. So, yeah, affective computing has been going on for quite some time I actually, I'll, take a slightly different stance I think that. There. Are some concerns about machines. Trying to influence the emotional state of their users I think that this kind of thing has been going on for a long time you, just see it in different forms like advertising, is is, one where, you. Know advertising you. Basically had a bunch of people in a room in the 1960s trying to predict what an ad campaign, would get you to like buy their product right and they. Were trying really really hard to like manipulate, emotional, state of people, who would see the ad in order to get them to buy some product. You. Know and like people have been trying to persuade each other since the beginning of time and. Now. We just use like better and better tooling for it so, now we have. Kind. Of algorithmic, advertising. Where people try to figure out what videos are, most. Appealing to get people to watch and and what, news stories, will kind of get them to, read. And click. On and so on and, I think it's sort of a gradual thing so, these systems, are actually, getting better at kind, of sucking people into this negative news cycle and we see it with like fake news where it's actually it is a serious problem. That like, I think people are aware. Of it and are working on it now but, I see this as it's, it's an extension I mean these technologies, can be used to, cause problems with like the. Fake news cycles, a lot of them are driven by advertiser, revenue right, and you, have various bad actors that are basically just trying to inflame sentiment, in order to get views. So. Yeah. It's it's very different than the world imagined, in them in the movie her, but. It. Is it is definitely an issue we need to think, about ways to address this in, terms of like identifying. Whether. Or not some, some, news article is fake or identifying, if some particular, website is trying to manipulate users, in some way that's, kind of, unacceptable. Right. Yeah. And. I think I'll skip the second part about how to do this. Awesome. And with, that I want to thank the panel again.
2019-11-28 13:43