Cost of a Data Breach 2024 and OpenAI's Project Strawberry
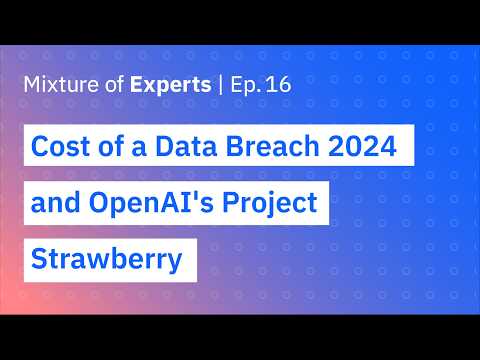
Is AI going to save computer security? I think there's a balance. So while new tools are helping a lot, then on the other side, we are also seeing new risks that arise with AI. There is no evidence that Strawberry is anything at all. OpenAI does need something that is significantly better than where they are right now. So I do believe that they have to release something mega pretty soon.
I'm Tim Hwang, and I'm joined today as I am every Friday by a tremendous panel of researchers, engineers, and others to hash out the week's news in AI. Today, Nathalie Baracaldo, who's a senior research scientist and master inventor, Kate Soule, who's a program director in generative AI research, and Shobhit Varshney, senior partner consulting on AI for US, Canada, and Latin America. So before we get into this segment, I want to do our usual around the horn question.
Um, and I think it's a really simple one, but I think teases up really well to kind of get into this topic. And the question simply is, um, data breaches are very expensive today. Do we think in about five years that the costs of an average data breach will be going up or down? Will it be greater than or lesser than the kind of damage that we see nowadays? Um, Shobhit? More. Uh, Kate, how about you? I think down.
All right, great. Going down. Okay, great. Well, we just got some disagreements, so let's get into this segment.
So we've got a couple of news stories that we really want to focus on today. First one is actually a story that comes right out of IBM. Um, IBM released basically a few weeks back a report called Cost of a Data Breach, which is the latest edition of an annual report they do, estimating the the costs of data breaches. Um, and it has some fascinating implications for AI and cyber security. Um, right now it estimates that the average cost of a data breach is rising, um, 10 percent increase over last year, where the average data breach costs is about 4.
88 million. But I think one of the most interesting things is that it estimates that there's an average 2. 22 million costs savings in the use of security, AI and automation. So that's, that's a huge, crazy, crazy difference. I want to kind of get into the discussion with, uh, Nathalie to bring you in first is that's like a 50 percent difference, right? And I'm kind of curious how you think about sort of the use of AI in the security space and how these kind of two worlds intersect and the world, uh, the implications I think for AI in the security space. Thank you, Tim.
So, um, actually, I read the report and I'm very, very happy to see that Gen AI and like AI in general really reduce the cost of, uh, incidents and help a lot. The teams are really involving the security. I think there's a balance. So while new tools are helping a lot, Then idea. On the other side, we are also see new risk that arise with the eye. Now, uh, the amount of benefits that we have with these new tools.
It's fantastic. So I'm very, very excited that we're heading in the right direction, but we cannot forget that we do need to protect those tools against adversarial attacks and throughout their the pipeline of the system. So overall, I'm very excited to see the entire communities heading in the right direction. Definitely including AI for, uh, auto verification and, and helping humans.
It's really helping out. And uh, so yeah, that's, uh, that's my thoughts. Yeah, for sure.
That's really helpful. And Shobhit, I'm thinking when you talk to clients, you know, you work with clients on a wide range of ai, different implementations and you know, the security space is something we actually really haven't covered very much on this show before. Um, and I'm kind of curious in the market, do you see more and more enterprises wanting this? thinking about this intersection, um, and I guess if there are particular use cases that come to mind where you're like, wow, that's, that's really making the difference, I think, in, in reducing the impact of data breaches, preventing data breaches in the first place.
Um, just curious about what you're seeing out there in the market. Yeah, absolutely. So a very, very hot topic for all of our clients, and it's a two way street. There is. AI that's helping you drive better security.
So pattern recognition and things of that nature to secure things. But there's also the reverse where the security teams are doing a better job at protecting AI as well. So it's both directions.
We are learning quite a bit. So we've gotten much closer to our security services within, uh, consulting as well. There are a few things that you do in security. There is prevention. There is a making sure that you're being detected fast enough, you're investigating what happened, and you're being able to respond, right? The whole life cycle of it. So across the whole platform, if you look at what, from a tooling perspective, you're doing things like what's the attack surface, how do you manage that? How do you do red teaming around it? How do you do the do posture management, things of that nature, right? So there's quite a few areas where Gen AI has been, or AI has been able to make a meaningful difference to it.
The report that we're talking about, that's a, that's a massive study. I'm just to give you the scale at which we did this, there are about 600 plus organizations that had data breaches in the last year. 17 industries.
We interviewed, this team interviewed about, um, Close to 4, 000, uh, people, senior security officials who dealt with the security breaches and stuff. And we looked at the entire spectrum of where AI is getting involved, is being applied, right? So when you start to look for patterns or looking at how do I do training, so the number one reason, number one was human error or human training that's needed to prevent these from happening. So small things like social engineering.
I can use generative AI model to create a very, very plausible email that will be very tempted to click. So that click baitedness of how we generate content has been applied to social engineering attacks. Right, like using it for red teaming is kind of what you're talking about now, right? It's like, yeah, right. So red teaming, great use case. The second one, I'm working with a large Latin American bank. We're working on cybersecurity, uh, uh, pattern detection.
So we're saying, here's a set of things that happen. Can you, can you create an early alert? based on the pattern that you're seeing. And then the same information needs to be assimilated at different levels and being able to send out as alerts, right? So we're being able to automate parts of what a human would have otherwise done in managing the whole life cycle from detection, education to detection, to managing the thing, right? On these SWAT calls, you join a SWAT call and it's been running for the last six hours.
And executives will jump in and say, Hey, can somebody recap? Right? That's a very easy one for us. So now we've started to generate recaps of what has happened so far. Actions that people have committed to taking. So those things show up on the right side. Anybody who joins the SWOT call knows exactly where we are with trying to Get a sense of.
That's really cool. Yeah. I never really thought about that. Yeah.
I think that's kind of the funny thing is like when you think about like AI and security or like, Oh, there's a, you know, hyper intelligent machine, you know, uh, system that will just defend against hackers. But I think what's really interesting is like a show, but a lot of what you're talking about is just like, how do we optimize like the human team that's doing a lot of this, which I think is really, really important. Um, okay. Maybe a final question for you to kind of bring you into, and I'd love to kind of get the, the researchers sort of view on some of this is.
You know, Shobhit talked about a big piece of this is defending AI systems, uh, against kind of subversion or manipulation or attack, which is a huge issue, right? I mean, you know, I was joking with a friend recently. I was like, there's probably a whole product you could build that's just around kind of manipulating open, you know, chatbots that people have on people's websites and that kind of thing. Um, and I guess, I don't know if you want to give our listeners a sense of like the kind of like, sort of like state of affairs there. Um, because it feels like, I mean, there's certain things that just seem like very hard to defend, right? Like it's like within a few minutes of any model coming out, people have already extracted the prompt and the system prompt out. Like that's like just something that's like hard to control.
Um, and so, yeah, I guess on the technical side from this kind of perspective of defending AI systems, curious if you have any thoughts or hot takes on sort of like where we are there and if the kind of state of the art is getting to the point where we feel like, yeah, we can actually kind of handle some of these attacks when we these systems to the wild. Yeah, well, I want to make sure we give Nathalie a chance to jump in there because Nathalie, I know you're doing some really exciting work specifically in that space, so it'd be great to to get your perspective as well. You know, I think my where I've seen some really interesting research that we haven't. Quite touched on yet is actually on the data itself. So not that necessarily the life cycle, but imbuing the data itself with different protection.
So if it is leaked, maybe it's not as big a deal, right? So there's some interesting work going on that we've done, for example, with some different financial institutions looking at, can we create versions of the data? That are privacy protected where we actually create a synthetic version of a, you know, a customer bank transaction records. We extract and remove all PII. We try and make it, you know, so that you could never identify the individual and we use that data set.
to now go out into the business and drive decisions and, you know, have a much broader reach across organizations. And that way, if that information is leaked, sure, there's, you know, maybe some business knowledge that's leaked, but there's not actual customer information that's leaked to the same degree. So there's a whole area of research around kind of synthetic data and making that decision. data, um, private that I think is going to be really powerful as a tool.
But Nathalie, you know, what are, what are your thoughts? You're, you're so ingrained in this space, really eager to get your perspective. Yeah. Uh, this, this question, I really like it because it really touches upon the entire life cycle of the model.
In my perspective, risk is throughout the system. And right now I'm working on something that it's really, really, uh, interesting. And it's the concept of unlearning.
And, uh, a lot of people find it interesting that it's not learning. Uh, but actually we're removing knowledge from a model. So let me, it's like, we're all about machine learning. You're like doing the opposite.
it basically. Yeah. And if you watch a Star Trek, there's this, uh, Yoda saying, you always need to unlearn or something like that.
It's because actually sometimes we touch upon certain topics that later on we'd really want to get rid of. And the reality is that when we have a machine learning model, the way that we arrive to these very large models is by feeding lots and lots of data. So one of the things as Kate was mentioning is really trying to mitigate what data goes into the model. However, because the data is so huge, it is really, really difficult to make sure that you filter everything.
So at some points in time, even after we apply defenses like we're doing here at IBM, we filter, then we try to align the model and everything. At some point, we may realize that the model is spilling out data that's bad. And this is going to happen just like in any security, uh, kind of, uh, area, we are going to see things that happen way after. Now, what do we do? We have two options. Option number one is cry. No, I'm kidding.
Option number one is actually retrain the model, uh, which is not going to break the problem because Think about how long it takes to, to train these models and how costly it is. So the idea of unlearning is rather than retraining, can we create a way so that we manipulate the model and forget all the information? in retrospective. And that is one of the things that really, uh, has got me really excited to work on, uh, because it's a new angle towards security and it's not only security, it's also life cycle management of the model. And that is a very, very, very, I think it's going to be the future. And, uh, Tim, you were asking the first question about how do I see the future? I'd see having not only guardrails and not only filtering, but also having this way of going back to the model, modifying the model, and then make it better for everybody. And we don't need to foresee every single thing that will go wrong if we can do this.
So that's, uh, uh, one of the things that I think it's, uh, very trendy. Nobody knows how to fully solve it, but we're there. And, uh, It's getting me really excited.
That's so cool, yeah. I mean, you hear it here first, listeners. Uh, unlearning is the new hotness in machine learning, so. I call it the new black.
So this week, and late last week, rumors are swirling around a thing called Strawberry. Uh, and if you are too terminally online like me, um, there's a large amount of discourse, uh, about this potential model that OpenAI is going to release, which is going to be, uh, promises a substantial increase in capabilities and reasoning ability. Uh, everybody's saying that it might be the model that fits.
finally brings the company into level two in their internal technology tiering, which is models that have much more powerful reasoning capabilities. Um, this is a really bizarre story in some ways because open AI has not disclosed anything publicly. Um, and in fact, most of the discussion online is being led by this completely weird anonymous account that showed up a few weeks ago, um, that goes by the handle, I rule the world Moe, um, which is this weird account that the Twitter algorithm just appears to love. right? Basically, it's just promoted into everybody's feeds all the time. And it promises that today, actually the day of recording is going to be the day where we're going to see this godlike model emerge. And now this, this account has promised a lot.
A lot of people have called it out for basically just not actually providing any real detail and just kind of adding to the AI hype. Um, and so I think there's two questions I want to cover here, but maybe let's just do the first one, which is, this is just hype, right? We have like no reason to believe that open AI is going to release. anything at all, um, and I guess I don't know which of you have kind of been watching this, this story.
Maybe I'll start with Shobhit, but like, Shobhit, like, this is, this is just hype, right? Like, we have no reason to believe that anything is about to happen today. Yeah, so there's, there are, he, he earlier said it was coming out Tuesday at 10 p.t., right? So he's been, you know, like moving it around as well.
All kinds of conspiracy theories, whether this particular Twitter account is just a shadow account for Sam Altman to just build some excitement and whatnot. There's just so much fan fiction in the space. I can't deal with it. I'm just like, I'm just trying to do machine learning here.
So I think just, uh, overall the arch of the reasoning capabilities, uh, is improving. It's not anywhere close to human, but it is starting. The models are starting to get better. I'm very encouraged by how enterprise friendly features are being added. Uh, things like function calling or structured outputs, things around, uh, observability and so forth. Right. So I think we're all moving towards the right direction.
OpenAI does need Uh, something that is significantly better than where they are right now. They have enough competitors that nibbling, uh, on the, on all the benchmarks and so on and so forth. So I do believe that they, they have to release something mega pretty soon.
Uh, Strawberry, all the rumors that I've heard so far, it's very encouraging. Uh, we've never seen any benchmarks around it yet. The models that were showing up on LIMPSYS and others in shadow mode and stuff, those are revealed to be the new 4.0 model and so forth. But you've still not seen any actual validation that these models are going to be any better. Seeing that iPhone is going to, Apple is going to come up with the next best iPhone, of course that's going to happen. It's just a very obvious thing.
I like that, yeah, like a prediction is like OpenAI is going to release something big at some point. Yeah. It's like, yeah, I guess that makes sense. And Tim, our clients, at least from an enterprise perspective, we're no longer jumping up and down with the latest releases of models and stuff, right? Now you're at a point where, From an enterprise value perspective, right? There's so much to be done before and after the LLM call, there's so many other things that non functional in nature.
If my data is on a particular cloud, the security IP, what's the licensing agreement I have on? Can I actually commercially use this model? How? How have I adapted that model to my own data? So on so forth and there's just so many millions of things that happened before and after earlier that has been my team's focus on creating the end to end workflows with the right evaluations and so on so forth for the business value unlock and the model itself we keep swapping that out on a fairly regular basis so our clients are not at a point where, oh my god, this beat the benchmark by 0. 1. They're not like texting you being like, what's up with Strawberry? Can I, can I get Strawberry? I actually, I do want to also kind of like, so that's very interesting on the business side, right? Because there's so much hype about on social media, sort of interesting on like the really day to day, like getting the business done kind of angle, like clients are not asking about it. Um, Kate, Nathalie, I would love to kind of bring you into this kind of on the research side as well, right? Like having worked with a lot of researchers in my time, what's kind of interesting is that a lot of this kind of Twitter hype doesn't really impact the day to day. Like a lot of people are like, Oh yeah, I know about it, but I'm not really paying attention to it. Is that your sense of it? Like there's kind of this like weird universe of discourse, which is about AI, but it's like not people who are actually doing the research.
I curious about how you, if you're a Strawberry believer, a, but just how you view this whole weird new cycle, I guess that we're in this week. Okay. Thanks. I mean, I haven't been paying too much attention to it. You know, it's a waste of time.
Yeah, we got more interesting problems to solve than figuring out the meaning behind Strawberry. But I don't know, Nathalie, what are your thoughts? Yeah, uh, the first thing that I thought I was very, very curious about Project Q, which seems to be same as Project Strawberry, uh, but being really day to day working with these models. The thing that I first thought is like, okay, now they are saying we are moving to the next level of AI when we cannot really fully measure the performance of the current chat based model, a level where we are. So I meet it with a skepticism in that, uh, it may be. great answer certain questions and in certain scenarios.
But when you dig deeper and try to change a little bit the context, it may be possible that it's not working. And the reason is that right now we really are not very good at measuring the performance of the models. There's tons of benchmarks out there. Uh, but if you throw the model to the wild, then you'll see stuff that is slightly different. So I meet it with a skepticism, really, I'm pretty sure it's going to be great. Uh, the other thing that I was thinking is that how do you know what is behind and the fact that it's closed doors makes me wonder, what is it? Is it really intelligence or are there like rules on top of a model? And, and maybe it is really, really tailored to this solution and the benchmarks that they are trying to beat.
So we'll, we'll see. But that's, uh, my, my take on that. That's right. And it's a very interesting outcome, which is like, you know, OpenAI drops like the new big model. Um, but like because our evals are kind of so crude for evaluating model capability, it's actually kind of unclear how much of an improvement it is. Like I think that's actually also really kind of potentially funny and interesting outcome.
Yeah. I push back a bit on that, Tim. Okay.
You think it'll be obvious? Like when they take action, it's going to be. Yeah. And it's very transparent. Uh, like we do this every day with our clients, right? So we'll go in and say, Hey.
Everybody has some sort of a knowledge search use case and rack patterns and so forth, right? So we have our own, our entire benchmarks. We create golden records, truth, grounding of truth and stuff. And we compare against those. We'll do a human evaluation. We will do an LLM as an, as a judge, whatnot, right? So we'll do this whole entire rubrics.
for clients. We see a meaningful difference when you're applying an OpenAI GPT 4. 0 model versus a smaller model. We do see a better response. It's crisper.
We do see quality improvements over the last, uh, 18 months to two years, right? So like I'm generally I'm very impressed with how well the models work, as long as you do the before and after ridiculously well, right? If you form the question in the right way, and you're asking it, and you're getting the data, the answers are getting better with these model upgrades. I still don't think that the smallest model can come close to what the OpenAI models are doing. There are some bespoke use cases like Cobalt to Java, right? Of course, IBM's model has to outperform a general model because we have all of this first party data, we have a ridiculously good set of talent around it, research, IBM tech can create that model and fine tune it really well.
So those use cases, obviously it's not even a competition. But if you're looking at knowledge article use cases, can I understand the nuances of what happened on this IT ticket? The ticket itself is 15 people have touched it. And each one had different updates.
What's the root cause of what happened? The bigger, nicer models have better reasoning capabilities, do an exceptionally good job at picking out the needle in the haystack, which smaller models cannot, can't get to. But Shobhit, do you think we're at the point where like, I can translate a 0.01 increase in MMLU or like the degrees of which, you know, we're starting to see these model incremental changes are so small. into like, this will improve my accuracy and then reduce my cost by x. So I do see, uh, different weight classes, right? If you're just still in the Olympics frame of mind right now, different weight classes. If you're in the, in the, in the top league of frontier models, you will not see that much of a difference because there are other techniques that you're using that have a higher impact on it, whereas just swapping out the model itself.
But the same use cases, if I go from Gemini to OpenAI to Claude, I do see meaningful changes in the way they're interpreting the data and how they're responding to it, right? But then once you pick a model, then the way you're asking the question, the way you've created embeddings and things of that nature, you have to tie it a little bit to the model. You can't just swap out that, that model for the new one and expect it to behave better. So it's, it's just not a very plug and play right now.
But if you find a model. You adapt the rest of the before and after to it. You see a fairly decent quality bump, but again, different weight classes will give you different results. Yeah, yeah. So I think, uh, hearing show it, one of the things I thought is totally agree with you in that large language models have improved substantially the performance of smaller models. Uh, the comment was really towards more how do we measure those big models, those large language models, and I think, uh, we still some to have some more research to measure a nicely what's their performance.
And I agree with Kate, uh, definitely higher MMLU does not guarantee that the model is going to perform, uh, great in certain use cases. So yeah, lots of interesting challenges to, to address there. We are unfortunately at time. Um, so Nathalie, uh, Kate, Shobhit, thank you for joining us as always. Um, and for all you listeners, if you enjoyed what you heard, you can get us on Apple Podcasts, Spotify, and better podcast platforms everywhere. Uh, we'll see you next week.
2024-08-18 13:14