Putting Data to Work: Data Science for Business
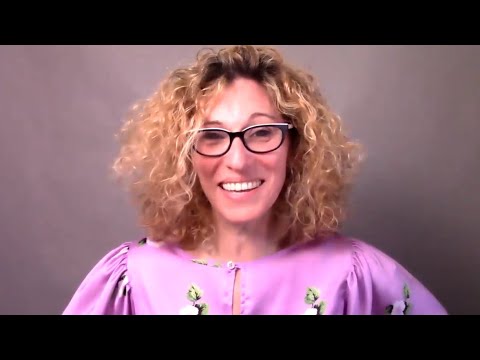
All right I think we're ready to get going. Good afternoon, good morning, good evening, depending on where you're coming from. So welcome to our session today by Harvard online at Harvard Business School Online entitled Putting Data to Work, data science for business. My name is Dustin Tingley.
I am a professor of government, and I'm a data scientist. And I offer a class called Data Science Ready. But we are here today to talk to my old friend Yael Grushka-Cockayne. And Yael, why don't you just introduce yourself for a second and then we'll get started. Sure.
Thank you so much for inviting me. Thank you for arranging this conversation. I can't wait to talk to you. I always like to talk to you about data science in fact, you and I can get lost talking about it.
I am a professor of business administration. I am currently at the Darden School of Business. I'm the Sr Associate Dean for Executive Degree Programs formerly.
And we met when I was visiting Harvard Business School as an associate professor. And I've really been a data scientist, I would say-- I would say that I'm a data scientist, although that was technically never my title. As a professor of business administration, my background is in decision analysis and operations research. And a lot of what I've done, even from the beginning, has been in the areas related to data science. And recently that's been really a passion of mine in the past few years, as it's grown and expanded in the world around us. That's great.
So Yael when I first met you, I'm reminded by that we were at a workshop about how to teach data science, which was really cool because usually data science has just been oh, we're just going to throw stuff up on the board. But people haven't really been thinking about how to teach data science. So that's pretty exciting journey that we've gotten to go on since that point, leading up to you're now about to launch class data science for business. So before we get into the conversation, I just wanted to let all the folks know, data science for business, the first wave starts on March 17. So get that in your calendar.
Applications are due March 2nd, and you need to enroll, pay for the course by March 11th. And just a quick reminder that there are no prerequisites for this course. And the key audiences are folks who are aspiring managers, rising leaders, marketers, product managers, folks who are in financial analysis. But really cutting a big swath across the business landscape. So we're really excited to offer this class Yael and team have worked really hard. It's a fantastic offering.
And so again, starts March 17th and make sure you get your applications in by March 2nd, which is a little bit less than a week from today. So get that in there. So Yael, let's kick it off. So tell me a little bit about how you became involved in data science. As you said before, you didn't come from that per se with that title, with that background.
But like what got you into it? Where did the journey start for you? Well, it's interesting, because I think my background is comprised of the three pillars that I would say data science entail. So I have background, if you go back to my undergrad, engineering background with a heavy emphasis on computer science, information technology. That's what I studied in my-- when I just was starting out.
So I started with that pillar. I got heavy into the field of statistics with a lot of my research that focuses on forecasting and the wisdom of the crowds, and how do you use multiple opinions when forming a forecast about an uncertain future. So I have the statistics as a heavy pillar in my research.
So information technology, computer science, statistics, and then I've been in and out of business for over 20 years now. And I'm a business school professor. I do a lot of work in the fields of decision analysis, decision making, critical thinking. I teach a lot of project management. So the domain expertise that is another part of that data science component has been part of my academic foundation. So you put those things together, computer science, statistics, and a business domain and domain expertise, and you get data science in business.
And so I really have kind of evolved with-- or enjoyed kind of my work evolving with the times. And as I work with decision makers, and I work with a lot of individuals and companies, and as my students are asked to perform various tasks in the workplace, my interests have evolved as well. And so the past seven, 10 years, I've been getting closer and closer and deeper and deeper into it. And it's just an ever-evolving field that fuels with research ideas, cases to write, and new learning and teaching opportunities all the time. So that's a little bit of my background. Maybe more-- maybe more than you wanted to know.
No. It's great. It's always interesting to hear how your professors-- the journey, the journey that they went on. I mean mine, quite frankly, it was somewhat similar. As an undergrad, I studied government and mathematics. The thing that I didn't have was the coding background.
That was something that came much later. I studied the math but the coding aspect. But then also the domain knowledge, which I think is just super important. Sometimes when the term data science gets batted around, they forget that the core pillar is like OK, there's got to be some domain that you're studying.
We're talking today about business, but data science genomics, data science of X, Y, and Z. It's really that domain knowledge oftentimes that is able to extract the value of the different analysis of algorithms et cetera. So it sounds like, given your own journey, you might have actually observed it evolution in how business schools themselves, like the curriculum that they're offering.
Describe that evolution. Are business schools now offering more data science type opportunities for students? They definitely are across the board. That is 100% accurate. I mean most business schools that I know offer more courses than ever before in these areas. They have changed.
If you go back 20 years, 25 years ago, which is a whole generation ago, there were maybe some more IT related courses that you would find in business schools curriculum. More like databases or computing. These days, if you go and you look at a typical business school, you'll see Introduction to Decision Making Using R or Python, SQL courses.
You see more advanced-- sometimes experimentation courses on how to run A/B testing and how to conduct experimentation properly. Helping our MBA students really understand in some circumstances, what is causality? Different business schools are offering an array of courses that are preparing this generation for the requirements in the field. And so it's been exciting. And a lot like you and I met in that workshop a while ago to share ideas across the various different disciplines and schools and how to teach data science. One thing that I'm seeing in the business school domain is that the professors who are teaching these courses reach out.
They collaborate. They share cases, they share materials. They point each other to good data sets to study, interesting problems. And so it's a very vibrant, open source rich community, much like the data science space itself that inspires one another to come up with new ideas, new ways to deliver the material and really help us help the future MBA, the future business leader grapple with everything that is thrown in their face. So it's a lot of fun. Our students are soaking it up and really looking for more and more opportunities to learn this stuff.
That's great. And my sense is that that's something that-- it's not just happening within business schools, but it's sort of across the board. That you're just seeing this explosion. And this is a little bit more focused in the business context.
I really like how you brought up the role of cases. Because I think, at least in my experience, I did not learn this material with respect to the real world. I learned this material with respect to a blackboard and chalk and equations. And I just felt like that was such a limiting experience. And when I started to teach more using cases, using things to really draw in learners with actual context, it made all the difference. That's how your course project is built on as well, right? Yeah it's very much applied.
It's very much in the context of a business-- specific business problem, an actual data set from a real company trying to make a real decision. I teach most of my classes using cases wherever that is that I teach. And online is not any different from what I would do face-to-face.
I truly believe that is the best way for learners to grapple with complicated concepts. People are typically-- my sense is that many folks have this, maybe like you, this kind of vague notion of equations and being overwhelmed with things that do not make sense in isolation. And so they have-- they're a little bit intimidated sometimes from statistics or programming or computer science. So you need to make it real. You need to make it relevant and to ask why do I care about this? Or why do I want to look at a summary statistic? Or how is it going to be meaningful for a specific decision? And so by exposing our learners to cases in various industries, the richness of the variety, put yourself in a decision makers position and understand how actual decisions get made, it just makes it much easier to grapple with. It also makes it clear that there isn't always only one way to tackle every problem.
There are many OK solutions or correct solutions. There are typically many ways to go about solving a real problem. And when you have data and a business problem, you need to be creative. And you need to practice and kind of make sense of things, when there isn't always a recipe or a specific equation that you can always apply and you're 100% done. So we want to build that intuition. We want to let people build that judgment, get exposure to how managers in the field use that judgment in practice.
And so that's what the cases do. One last comment on that, my sense is that cases are also easier to remember. Like stories, right? Storytelling, that's how it's always evolved. Knowledge used to be in the form of stories.
And that's why cases are useful because you can tell a story of a company that you tend to remember, more than just module 3.2.6 which is just an equation, right? You know the company, you will remember ultimately the companys names, the protagonists maybe. And that's how you will remember the concepts.
And that's what you want to take away with you. So that's why cases are so useful and I'm excited to offer it in this course. So it sounds like one of the upshots of that is that learners in your course will be able to go through a set of cases along the way learning some data science material which we'll talk about in a little bit.
But when they're out in their own world, they'll be able to reflect and say hey, the situation I'm in here, looks like what I learned in that case in these ways, but it doesn't look like it in these other ways. And that then let's give them something to reflect on, because many of your learners are going to be having to make decisions or advise people to make decisions. So that sounds like it's very valuable.
I also like something that you mentioned, which is there's just oftentimes no-- there's no perfect answer, right? I mean it's sort of makes me think that if there we're always a perfect answer that was available, we should just hire data scientists, like the real people doing all the heavy duty coding and be done with it. Just let them sort of automate our entire operation. But it sounds like the world just doesn't exist like that, in your experience in business.
So that I think that's a great point. There's a lot of judgment in it. There's a lot of judgment, there's a lot of collaboration.
It's a dynamic process, so it's back and forth. So you need-- of course you want the data scientists to provide input and to help you test ideas, but it's an iterative process. And so practicing that process, and recognizing that that's the acceptable way to go about it, is really important. And I like what you said yeah, using the cases, folks will kind of recall. When they're in a position in their business, when they're trying to make a decision or understand how data could be useful to come up with data driven decisions, they will be reminded of maybe similar situations that we covered in the cases. Another thing that I'm hoping folks will walk away with is more of a sense of a process.
So like, even if you don't recall the exact analogous case that we covered, you will know the process to take. What questions do you ask along the way to get you from the beginning, which is very messy and ambiguous situation, to a situation where you're actually running models, looking at predictions, looking at results from some process in order to make decisions. So you know that there are steps.
You know that there are questions. The questions are pretty formulaic, and they could be repeated. And you understand what is the next step. OK, I understand my data. What do I do next? I clean it. I visualize it.
I try to fit some models. I look at the results. Like you get used to the terminology, and then you can practice it with other problems that you face. So it's a dual thing. It's the recall of the exact examples, but it's also the process that you gain along the way.
The process, yeah. I think having that more process orientation probably also helps for folks that aren't going to be doing necessarily like lots and lots of coding, but they want to make sure that those their organization are following that kind of coherent process. And that furthermore, it's always a process that is grounded, and there are concrete decisions that we need to make. And the data is helpful for that, the data is not helpful sort of in on itself. Let me ask you a question. What would you say are some of the biggest misunderstandings that you see that students suffer when it comes to data science? Like when they come in, they just have this opinion about what it is, how it works, or anything else.
What's the biggest-- what's the biggest misunderstanding that you find students oftentimes face? So there's a few. So one is I think a comment that you made actually is that there is this misnomer, some folks are under the impression that unless you are trained from like high school as a computer scientist, and you know every language in the book. And you've kind of been coding for years, you don't stand a chance. Which is not accurate, it is very misleading, as you said. You can kind of get into it in various ways through various avenues.
And it's really important that an intuition for coding is it's never too late. You can develop it by asking questions, by looking at output, by working collaboratively with somebody who knows, by googling and asking the right question on Google, and learning how to read answers. Like there's a lot of resources out there. And so that's one aspect is that if you haven't been doing it for years, you won't stand a chance.
A second misnomer or kind of concept that I would like to demystify is the fact that this is something that is done in closed doors. Like there are specific people that have the label on their door data scientist, and only they do the work, and you don't need to really get into it, or you'll never see code in your life. I think that the audience that we're talking about and the individuals that I hope join the course, and many of us actually in the business world today, including students in the MBA classroom but even in other specialty areas, there is a responsibility-- or we are part of the process. We have this role and this vision that we can be translators. We can help communicate between the people who have more of the technical chops and the rest of the business, how it operates.
And so we can help provide that connectivity. And it's a hugely valuable role. And it's again, you don't have to be deep into the tech to perform that job. For instance, I'll give you a concrete example, is that OK? Great, yeah.
We talk in one of the modules in the course we talk about data wrangling, which also sounds like a pretty technical term. We talk about data imputation, a technical term. But when you stand back, it's really to say OK, we have data. Is the data complete? Do I have all the information in there? Are there missing observations? What is the business impact from having missing observations? How do I deal with it? How do I compensate for data that I don't have in front of me? Those are decisions and those are conversations that every person that touches the business problem can contribute to, because folks understand the nature of the variable. They understand the nature of the data. They understand whether we're talking about credit scores, or we're talking about mileages on a car, or we're talking about prices.
It matters, and we need to think about the data in context. And so these conversations bring together the tech, people they bring together the business people. And the data scientists and the folks that are learning this material can really help bridge those gaps and make very critical decisions that have long term impact on the result of the whole process. And so that's why I encourage folks to get their hands dirty, to ask those questions.
To get more exposure, to understand that these terms are not something beyond them, but they can really understand and internalize them. That's great. I'm really glad that you two of the things that you brought up really resonate with me and my experience, and I'm super excited that you talk about them in class. The first is about the context of the data, and I would extend that to be the context on understanding why you don't have certain data. That in some sense can be just as revealing. Like oh jeez, I don't have that data? Why don't I have that data? Do my competitors have that data? It's like it's like the notion of dark data.
Like what can you learn from the data you don't have. The other thing that I like bringing up data wrangling. I'll take it. One of the misunderstandings that I often see is that people think that their data is clean. It's ready. It's formatted.
You just have to like load it into R or Python or Excel, and you're like off to the races. And I have a couple of friends who've been at a large internet company for many years and now are directing a lot of their data science operations, and they'll say that 80% of their time is around the sort of processing and preparing of data to make it useful. And 10% of their time is running the algorithms and so on and so forth. So that's really great that you get into the sort of data wrangling and all the things that go into that. And it's also about gaining appreciation for what people around you are doing. Like if you're working in a company that has folks that do this kind of work, which to be honest I have a list of companies and industries here, there's hardly an industry today that doesn't have some kind of data function in the organization.
And so if you're working around individuals who are doing this work, getting an understanding and an appreciation for how they spend their time and really kind of being able to understand the effort that goes into it, the type of time that they have to spend on it helps us be better leaders and better managers of those teams, right? So it's important to understand where that is coming from. And another very important topic that relates to data wrangling, but then expands to most phases of the data science process, relates to this notion of bias and ethical machine learning or ethical data science. If we don't spend time thinking carefully about our data and where we collected it, what do we have, what don't we have, as you mentioned. We will struggle to identify opportunities to debias our data or opportunities to note that there are certain directions that are consistently going in only one direction.
Or maybe our data was collected from only one subgroup of our population, certain customers but not others. It is very important that we are careful during this process and are thoughtful, because that's our chance to make a difference. To make a difference in the world, to make a difference to how companies conduct themselves. And to improve the decision making that we are all responsible for, such that it is much more ethical and unbiased. Yeah, I completely agree with you. And it's something that in the Data Science Ready course that I teach on the Harvard Business School Online, Harvard Online platform, that role of ethics is crucial as well.
And part of it, too, is just if you're someone in that kind of managerial role, you're overseeing the data science team, and you get some of those things wrong, right? You get a lawsuit coming in? You're on the hook. And so you don't want to be the person who is not thinking about those things, just from a legal perspective, or just from the perspective of not wanting your company to be on the front page of the New York Times, or getting that bad publicity, because you weren't thinking about those things. So that's great.
And of course, there's always the and, and I'll say yes, and I've been saying that a lot recently. But I agree completely with what you just said. And beyond that, it's an opportunity to develop better products. That's right.
I spoke recently with a colleague who you might know, her company does a lot in AI and machine learning in terms of interpreting emotions, and she works with a lot of car manufacturers and companies in the auto industry, to think about how we can design cars in which the cars can help us detect when people are getting tired, or when they need to be woken up a little. Or they shouldn't be driving. It's a hugely valuable kind of set of products. And for that, in order to develop the best product you want all kinds of drivers. You want short people, tall people, you want women, men.
You want different hairstyles. You want different colors. You want different eye sizes. You want all the different-- glasses, no glasses. You want all the diversity. And as somebody that comes in touch with the data, that's part of the questions that we can ask.
How can we ensure that we're getting the right data in to do the right modeling to come up with better products to ultimately do good for our company and of course to serve to serve the population and society in a better way. That's great just to let folks in the audience know, we are going to take some questions. So I invite people in the Q&A, let's put things into the Q&A function if you don't mind. And we're going to get to that in a couple of minutes.
But this gives you an opportunity to throw some questions in there, into the Q&A so we can get at them. Yael, I want to turn to getting a little bit more specific for folks around exactly what types of business decisions could benefit from data science, right? So just give me just kind of a landscape. We talked a lot about we want to use data help make decisions, but what are examples of some of those types of decisions that coming out of taking your class you'll be able-- people will be on a firmer footing about how data science can have an impact on? How long do you have? Because I can go. I can talk about that for quite a while, Dustin.
It's really endless to be honest. If you can think of a business decision that gets made, I can tell you how data science might contribute. I'll give you just a few examples for the rich-- of the richness of it. And then we can see how far we want to go with it. But agriculture, companies like Cargill, or large companies that do a lot with farmers or with products that are agricultural products, or even Anheuser-Busch and beer manufacturers. Crop yield, typical example of things that you can do better prediction of that are fundamental to those organizations.
You can help with sophisticated censoring and all the technology that you have out there. You can develop models to help predict the life expectancy of some animals, their health. You can detect issues that need to be taken care of in terms of the machinery that you have in the field.
It's endless. From there, you can talk about supply-chain. You can talk about inventory levels and tracking the right level of inventory. Predicting what kind of inventory you're going to need based on fluctuating demand and uncertain demand, coming up with improved predictions at the skew level, when you have multiple products and a huge variety that you have to forecast on a regular basis and control your inventory. That can help you control your supply chain. Then once you have the products, you have to think about the retailer front.
How can we better engage with our customer? So data science can help with image recognition at the shelf level of the products that we have in stock versus the products that we're going to need to replenish. Data science can help predict customer demand and customer personal preferences. We can predict various tastes, changing tastes, seasonality. That is all part of the gambit and pretty natural for data scientists to be thinking of. Is that good enough? I can take you in a different direction.
No that's great. You know you've covered a lot of things, questions of supply, stocking. I read an article the other day that was about a group of bakeries that are using data science to sort of make projections about what they need to be putting in to inventory, because somethings spoil, right? And some things don't spoil. And prices change more for some things versus others, and so on and so forth. So it sounds like people have always been making some of those decisions, some of those actual things for a long time.
I mean like predicting crop yield, people have been doing that for millennia. But it sounds like part of what has changed, part of the landscape that has changed is that we have richer streams of data. We also have the ability to process that data in more analytical ways rather than anecdotal, although there is such a thing as anec-data, right? It sounds like those have been some of the big drivers of change about why these tools now are unlocking value for companies in ways that other approaches of just sort of gazing at a spreadsheet maybe might not provide. That's exactly right. So the change in computing, so the fact that we're computing in the cloud.
We all have less limitations on our CPUs and our computational powers. We have more storage space, and we collect more data. We're all connected, and so there's again this open source and the knowledge gets diffused much faster. And those are all some of the properties. Interestingly, as you point out, the business problems, some have-- some are new.
Some are new that have evolved from this new phenomenon. So customization I think has ramped up. Personalization has ramped up, because we have more opportunity. I don't know that people were thinking about it exactly the same way 20 or 30 years ago, but they always thought about customizing products. And the business products and the business questions are not necessarily all that new. And even some of the statistical processes or the statistical tools that get used in data science have been around for a long time, 150 years sometimes.
These are not all new ideas, but they're coming together with that added storage, and the added data, and the added computational power, to form what we now as data science. And that's the same thing for AI and machine learning. A lot of the concepts were introduced a while back, but they've now gotten more of a popular exposure because it's been made available to more people for more people to use. And so that's part of the innovation, and in a way I hope that makes it less intimidating, because it means that some of this is very familiar stuff.
It's just you have to find it. You have to recognize it. You have to look for it and know that it's there. Great, I want to flip this in some senses on its head. And it's inspired by a fantastic question from, I believe I'm going to say this right, Cassiely.
Which is super interesting question, get ready Yael. How does one utilize data science knowledge and skills in a region, like Africa, where data collection is very limited and constrained? That's a great question. It's a great question, and the potential there is huge, right? So first, data is limited in some traditional senses, but data is also available in other ways. In the sense that, for instance, from Africa there's been some fundamental work.
Some of the first pieces of work that I've seen that have used texting and data from phones in groundbreaking ways. It's not the typical way that you would use it perhaps in maybe in developed countries or in the US, but they use it in ways because there's more individuals using their phone on a more active basis in a more intense way, and so they can take that data and just build around that-- whatever information comes in through those that usage, they can build models that fit that need. And in a way, the ubiquitous use of it is just so much more prevalent than it is in other places that it's richer. And it gives-- it provides opportunities to get to every little small town or community that is far out reaching what you could get with a browser system for instance in those locations.
And so it's just finding other sources of data, finding different sources of data, and building different models. That would be one way. I don't know Dustin if you're familiar with those kinds of usages, but it's super exciting. Yeah. And you know I think that's exactly the right way to look at it, which is know your environment, right? And how is your environment different from other environments, which means that the types of data might be very different that you can utilize. So I think that's exactly the right way to think of it.
In a way-- in a way it's a lot more entrepreneurial I would describe it. Many more entrepreneurs, agents with data, collecting data, and acting upon that data. And so in a way, I find it in some ways more powerful and more useful data than you might find in other locations. Yeah the other phenomenon I would point to there given Cassielys great question is that you're starting to see hubs of people in Africa, in South America, et cetera, who are sort of poking their heads up in whatever kind of social networks et cetera say hey, I'm a data scientist.
I'm interested in this. Is anyone else doing this work? And you're seeing things like the data science language R, which is something that people will learn something about in your course, there are what we call affinity groups in Africa of people doing that type of work. And so plugging into those, I mean that's one of the things I really like about these courses is that there are-- we really like learners to be learning from each other. But the reason we're doing that peer learning within your course or with my course is that we want to get people to think hey, I can go out into the real world and learn from other people who are in my region, and hey what are you doing? And it might be that what they're doing is different than your business case. But being plugged into that community, being plugged into that ecosystem is just super valuable.
And you're seeing that pop up in these affinity groups throughout Africa. And one of the nice things there too I should say, is that many of the tools that data scientists use are open source and free, right? Whereas when you and I were first learning some of these things back in the day, we were having to purchase $150, $200 software programs. And things have changed in that respect. So I think that's another exciting reason for why people throughout the world could be getting involved in the data science space. I totally agree, and the access to cloud computing. So little start-ups. So a few individuals who want
to start a company, they don't have to invest a huge amount in IT and infrastructure. They can use data science. They can use sophisticated tools like TensorFlow and open source tools for nothing. It's made available for all to use, and so it's really at our fingertips. And that is an exciting opportunity.
And that's part of why there's such a draw. First, it's a community that embraces online. So you can learn a lot of the mechanics online.
It's an open community that engages with each other as you pointed out, which we're trying to foster the conversation, always ask and discuss and share. And it's a community that the boundaries on entry are low, right? The ability for anybody to join in and try to spin up their own server, or get more cloud access, or find ways to dig in is fairly easy. And that's really exciting. Yeah, that's great. I want to turn to another question, which is just because we have talked a lot about, which is around visualizations.
And the question from Maria is a little bit more specific about what specific visualization platforms and options. I should just say that we're not going to go deep into that now, but other-- there'll be some Tableau. You can visualize things in R, so on and so forth. But tell us a little bit about why visualization was important for you to hit on in your course.
I love-- first I think that visualization is fascinating because it draws into data science, different individuals. Some people like the coding aspects and are drawn to that, and some individuals really sparkle and shine when you start to show them visuals. And they get creative in how to form new visuals. It's almost like it's a subdiscipline I would say, but it's like-- some people get it. They have that like journalistic kind of sense, and they can think about what is the best way to tell a story with the data? And that's an incredibly powerful skill.
I've seen folks get hired for jobs just because they knew how to walk in and kind of play around with the data and show it in a compelling way and in a dynamic way. So in a meeting situation or while you're brainstorming, kind of having the ability to take data and to create on the fly new visuals that give insight. One of the reasons it's so important in today's world, and it's rising and its prominence, is because as we've talked about data is larger and larger. You can't always open things in Excel.
You can't always scroll up and down to see the data. And so you see to some degree, we have to get comfortable with losing that first-- the ability to touch it, I would say. We used to be able to see it on the screen.
You could kind of like bound yourself to those number of rows. Now, the data is too big. When you have-- in the course, we have spreadsheets with a million rows. Like you have a lot of data, OK? And so when you're working with that data, the best way to really get to know what's in there and to familiarize yourself is to start throwing visualizations together, OK? Histograms, scatter plots, line plots, throw it on the map. Get a sense for the geographical phenomenon. Word clouds, if you're talking about text.
Like there's so many reasons to start with a visual to get a sense for what it is that your data looks like. And it helps you to spot things that you're surprised by, or maybe even going back to wrangle the data, because you notice in the visuals that something doesn't look quite right. It builds an intuition. And you can come back to visuals later in the process to continue to improve your modeling capabilities. So it's just, it's endless. And it's such a powerful phenomenon.
And it's also something where you mentioned, seeing a map. Like what's the geography of the data? But there's also how does the data evolve over time? That's another way to structure-- to structure that data. And with the tools out there today like Tableau, you can put together a quick-- like with a drag and drop of a couple of buttons, you can put together like a little movie that takes you through time. It's incredibly powerful.
Yeah, no, it's the super rich. We've got another question from Oladapo, who's asking what's the difference between data analytics and data science? And so I thought what we would do is use that question to jump into a brief discussion about how Data Science for Business as a course that folks can take, your course Yael, how it's different from some of the other data driven courses that are offered by Harvard Business School Online and Harvard Online? And one of those courses is called Business Analytics, which I think is really a class that is kind of in that more data analytics tradition. And it is, I've got to say, I don't know how much of it you've taken Yael. It is a fantastic class taught by Jan Hammond, who's just this like The guru? The guru, yeah exactly. So that business analytics class, so that's teaching you a little bit more in the way of what the kind of fundamental statistics are that are used in business problems using Excel, which is a powerful tool. And it's covering descriptive statistics, some hypothesis testing, some regression analysis.
And it's a fantastic class that really I think is gearing people a little bit more for that data analytics course. Whereas I see your class, Data Science as Business as being different in the sense that it is still covering some of the same statistics concepts, but you're much more invested in the sort of data science process writ large that you laid out earlier in the conversation. Where there's all this stuff about different sources of data, how do you wrangle with the data, how do you clean it, how do you build a model that might not be a regression. You do things that go beyond a standard linear regression in the course. How do you-- how do you visualize in ways that go a little bit beyond kind of the standard descriptive type setting? And so it's a really-- your class does this definitely does not have a prerequisite requirement, and I think that's great. And so I see Business Analytics as the nice kind of compliment to that.
So that gives a sense Oladapo about that distinction between kind of data analytics and data science. And then we have Data Science Ready which is the course that I teach. And so people oftentimes ask OK, like how would-- Dustin, how is your course different than Yaels? And so I like to think about Data Science Ready as equipping people for the entire landscape of data science in a way that has no coding and no math to it. And so it's a little bit different in orientation in that sense.
So I for example, spend a lot of time on things like privacy and ethics. I spend a lot of time and things like causality. I also spend a lot of time on non-numeric data.
So Yael, you mentioned some examples of this, but we have a whole section about text as data, sound as data, images as data. And then, when you have those types of things, what's your data pipeline? What's the process? You can't just load things up into an Excel or some spreadsheet the way you used to. So it's really trying to give people what's the entire landscape of what data science is in a non-technical way.
And what you're doing Yael, which I think is great, is saying OK, let's start to focus on some of the particular tools without doing like a super, super big deep dive, right? What are some of the tools? What is that data science process itself? And how do you connect those things to data driven decisions that businesses have to make? And so that's how I kind of characterize those three different classes in the portfolio. But it's a common question that we get asked, so I just wanted to take a chance to talk about that for a second. Yeah I mean I agree with your description. It's almost like they're like layers in this whole kind of space, with Jans course, being very, very much about the basic statistic concept. The inference, the basic get your head around the basic statistical terms and tools. And then Data Science in Business, the course that we're talking about, my course, goes bigger.
Bigger data sets, more tools, much more coding, and the rationale for why do we need to make that shift. Why do we need to move beyond Excel? What is the reason for it? Why do we see it around us? And then really spend more time being thoughtful about the different points in the process. And then yours takes that those processes and assumes where are they in the world around us, and what are the bigger concepts that have to be considered in that regard? So I think that's a great way to think about that sequence of courses. Yeah, yeah, that's great.
So I want to have this answer one last question that's really interesting. And then we're going to wrap up. So this is coming from Jessica. Super interesting question, I'm so thankful that we have folks like this on the call today.
So can you talk a little bit about data science ways to avoid confirmation bias. So you're a decision scientist. You have this background.
So how do we avoid confirmation bias when we're presenting things to decision makers? Like my boss he just wants to hear the answer, and it's the answer that he wants to hear. (LAUGHS) How do we-- what are things in the data science tool kit or the data science process that help us avoid confirmation bias? It's a fantastic-- I agree with you, it's a fantastic question. And I agree with you that's one of the beauties of doing these types of webinars, because it's great to get an engaged audience and to get other participants to recognize what a wonderful community they're buying into and they're joining, because they'll get these types of questions. Confirmation bias is really a tricky one. It's an important one to know that when you are in a situation where you're making a decision or when you're trying to look at a phenomenon, we as individuals, as humans, are more inclined to convince ourselves that we're right as opposed to look for contrary information. So we're going to confirm what we hope to see more often than not.
And this is a phenomenon that's been studied by psychologists, it's been studied by economists. It's out there around us in many different ways. And it's one that awareness helps. But more importantly, and this relates to data science, it's also about asking ourselves constantly what would we have to see that would make us change our mind? And holding ourselves to that.
Meaning, what would the data have to tell us, or what questions do we need to ask that the answer would make us decide differently? All too often, I ask individuals OK, what more data do you want to in order to make a decision. Because the first thing that people say when they're trying to make a tough business decision is I need more data. OK, so then you say what kind of data would you like? And they describe the data that they're thinking of, and then you ask them, what would you see in the data that would make you change your mind, decide otherwise. If it's a project, and you're inclined to say yes, what would make you say no? If it's a certain decision to serve a certain ad, when would you not serve that certain ad? Stuff like that. And it's very hard for folks to articulate that.
You'll see that the natural tendency is to say oh, nothing I'll see you in the data will make me change my mind. It's like wait a minute, you just said that you wanted more data, and then you're not describing when would you act upon it. So you have to challenge that to some degree, because once you've identified certain observations or the way that the data would help inform your decision to the point where you're willing to walk away or you willing to change your mind, then you can avoid confirmation bias.
Because then you're actively seeking the contrary opinion. Does that make sense? I hope that makes sense to you. No, no, no. I think that's-- I think that's super interesting. At times when I've done consulting and whatnot for businesses, and they do the same thing. We just need more data.
I oftentimes sometimes think that I want to charge them for the data, such that there's a constraint. But I will charge them a lower rate for data that is contradictory to their priors. Just like something like that. Yeah I have an exercise in the classroom that I actually do that. I have an exercise in the classroom that we actually like have students, and they get a business question and there's a bunch of data potential data out there, and they have to decide-- they have a certain amount of budget, and they have to decide which data they're going to purchase. It's a great exercise for folks to tackle with.
That's excellent. Well Yael, it as always is a pleasure to talk with you. Again, for folks I'm Dustin Tingley. I'm a professor at Harvard.
I'm a data scientist. I offer a course called Data Science Ready, but we've been very lucky to talk with Yael Grushka-Cockayne who's also a professor of Business School at Darden, a colleague of mine at Harvard for a while. And we are really excited to get your course launched. Just a reminder to folks the first wave of Data Science for Business starts on March 17th. And the applications are due by March 2nd.
So if you want to get in on this, it could be a great community of learners. A really exciting case, practical driven course for all of you. So get your applications in. You have to formally enroll or pay by March 11th, so start to put things on your calendar. And get excited.
There's no prerequisites for Yaels course. And again, some of the audiences are aspiring managers, rising leaders, marketers, product managers, financial analysts, et cetera. So it's a very rich course it's been fantastic to see it emerge over time. And just really thankful for your spending some time with us today. We put it in a link that you can-- into the chat that you can use to get more information.
But again Yael, thanks so much for spending time with us today. Thank you so much for inviting me. Thank you all for joining and for the great questions. I can't wait to have you on the platform and to engage with you through the platform. I look forward to meeting you in other avenues. So thank you so much.
Excellent. All right thanks very much everyone. Have a nice day. Thank you, everybody.
2021-03-01 14:50