A skeptical look at AI investment
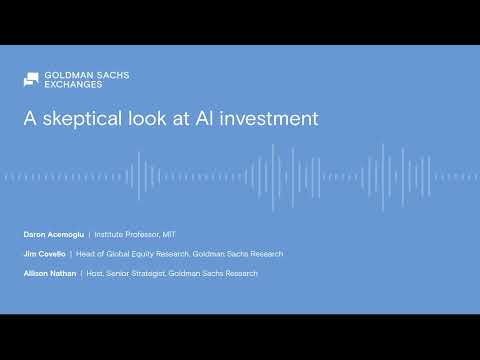
Generative AI is being heralded as one of the most transformative innovations in human history, and AI optimism has become one of the market's biggest drivers. Companies are estimated to devote over $1 trillion to AI-related spending in the coming years, so will the benefits and returns of the technology justify the cost? Jim Covello: We're a couple years into this, and there's not a single thing that this is being used for that's cost effective at this point. Allison Nathan: I'm Allison Nathan, and this is Goldman Sachs Exchanges. Every month, I speak with investors, policymakers, and academics about the most pressing market-moving issues for our Top of Mind report from Goldman Sachs Research. This month, I looked at generative AI. 2
I think we're all familiar with the bull case by now. My colleague Joseph Briggs from our Global Economics Research team estimates that generative AI could ultimately automate a quarter of all work tasks and boost US productivity by 9% and US GDP growth by 6.1% cumulatively over the next decade. And investors have certainly bought into the theme with the big tech firms at the center of it all, accounting for over 60% of the S&P 500 Index's year-to-date return. But given the enormous cost to develop and run the technology with no so-called application for it yet found, questions about whether the technology will ever sufficiently deliver on this investment have grown. I spoke to two people who are skeptical. Daron Acemoglu, an Institute professors at MIT, the author of several books, including Power and Progress: Our Thousand-Year Struggle Over Technology Prosperity. In a recent paper, he estimated
that only a quarter of AI-exposed tasks will be cost effective to automate over the next ten years, implying that AI will impact less than 5% of all work tasks and boost US productivity by only 0.5% and US GDP about 1% cumulatively over the next decade. I asked him to explain. 3 Daron Acemoglu: Part of the reason why I wrote the paper is because I did see a lot of enthusiasm, some quantitative, some qualitative, from commentators, some experts, others observers of the tech industry about the transformative effects that AI is going to have very quickly on the economy. And I think economic theory actually
puts a lot of discipline on how some of these effects can work once we leave out those things like amazing new products coming online, something much better than silicon coming, for example, in five years. Of course, if that happens, all right, that's big. But once you leave those out, the way that you're going to get productivity effects is you look at what fraction of the things that we do in the production process are impacted and how that impact is going to change our productivity or reduce our costs. So those are the two ingredients. And my prior, even before looking at the data, was that the number of tasks that are going to be impacted by gen AI is not going to be so huge in the short run because a lot of the things that humans do are very multifaceted. So almost all of the things that we do in transport, manufacturing, mining, utilities has a very central 4 component of interacting with the real world. And AI
ultimately can help with that as well, but I can't imagine that being a big thing within the next few years. So therefore, my intuition was that, from the beginning, it's going to be pure mental tasks that are going to be affected. And those are not trivial, but they're not huge either. So the way I go about doing that is I rely on one of the most comprehensive studies by Eloundou, Mishkin, and Rock that codes what the current AI technology -- generative AI technology -- combined with other AI technologies and computer vision could ultimately do. What they did seemed fairly, fairly solid, so I decided to start from what they did. So if you take their numbers,
it suggests that something like 20% in terms of value-added share in terms of the economic importance of the tasks that we do in the production process could be ultimately transformed or heavily impacted by AI. But that's a timeless prediction ultimately. But when will that ultimately be realized? And in that, there is another paper by Neil Thompson and Martin Fleming and coauthors which looks at a subset of these technologies, the computer vision technologies, where 5 we understand how they work and the cost is a little bit better. And for the computer
vision technologies, they come up with some numbers as well. But more importantly, they make an effort in thinking about how quickly these things are going to be cost effective. Because something being ultimately done by generative AI with sufficient improvements doesn't mean it's going to be a big deal within five, six, seven years. They come up with a
number for computer vision technologies, again, which looking into the details, seemed reasonable, which is at about 20-25% of what is ultimately doable can be cost effectively automated by computer vision technologies within ten years. So then I combined these two estimates, and I forecast that about 4.5-4.6% of those 23% is going to be done in the short run and within the 10-year horizon. And that's the
basis of my sort of fairly uncertain -- of course, we cannot be certain about any of these things -- but baseline estimate of what can be achieved with gen AI within a 10- year horizon. Allison Nathan: When we think about applying AI technology to various tasks to improve productivity and 6 increase cost savings, we have seen in the past that over time, as technology evolves, you end up being able to do harder things and you end up being able to do them in a less costly way. Do you expect that to be the case for AI? Daron Acemoglu: Absolutely. Absolutely, I expect that, but I am less convinced that we're going to get there very quickly by just throwing more GPU capacity. So in particular, I think there is one view -- and this is, again, another open area. That's why any estimate of what can be achieved within any time horizon is going to be very uncertain. But there's a view among some people in the
industry that there's, like, a scaling law. You double the amount of data, you double the amount of compute capacity, say, the number of GPU units or their processing power, and you're going to double the capacities of the AI model. Now, the difficulty there is at least three-fold. One is what does it mean to double AI capabilities? Because what we're talking about here -- for example, open-ended things like customer service or understanding and summarizing text -- there isn't a very clear metric that I get twice as good. So that's one complication. 7 The other one is "doubling data," what does that mean? If we throw in more data from reddit into the next version of GPT, that will be useful in improving prediction of the next word when you are engaged in some sort of informal conversation, but it won't necessarily make you much better at helping customers that are having problems with their telephone or with their video service. So you need
higher and higher quality data, and it's not clear where that data is going to come from. And wherever it comes, it's going to be easily and cheaply available to generative AI. So the doubling data part is also not very clear. And then the final is I think there is the possibility that there could be very severe limits to where we can go with the current architecture. Human
cognition doesn't just rely on a single mode; it involves many different types of cognitive processes, different types of sensory inputs, different types of reasoning. So the current architecture of the large language models has proven to be more impressive than many people would have predicted, but I think it still takes a big leap of faith to say that, just on this architecture of predicting the next word, we're going to get something that's as smart as Hal in 2001 Odyssey. 8 So those are all the sort of uncertainties which, to me, says if you're thinking about the next few years, we know already the models. So anything that's invented or big breakthroughs, it's not going to have a huge effect within the next few years. Allison Nathan: You've said many
times it's really about the horizon. Daron Acemoglu: Right. Allison Nathan: But ultimately, there are people out there arguing that this technology is paving the way for superintelligence that can really accelerate innovation broadly in the economy. Are you questioning that at all? Daron Acemoglu: Well, again, for the current paper we're talking about, all I need to say is that it's a time horizon issue. So I don't think anybody seriously is arguing or can make a serious argument that, within five to ten years, we're going to have superintelligence. Now, going beyond the 10-year horizon, I would also 9 question the premise that we are on a path towards some sort of superintelligence, precisely because of the reasons that I tried to articulate a second ago, that I think this one particular way of understanding and summarizing information is only a small part of what human cognition does. It's going to be very
difficult to imagine that a large language model is going to have the kinds of capabilities to pose the questions for itself, develop the solutions, then test those solutions, find new analogies, and so on and so forth. Like, for example, I am completely open to the idea that, within a 20-, 30-year horizon, the process of science could be revolutionized by AI tools. But the way that I would see that is that humans have to be at the driving seat. They
decide where there is great social value for additional information and how AI can be used. Then AI provides some input. Then humans have to come in and start bringing other types of information and other real-world interactions for testing those. And then once they are tested, some of those reliable models have to be taken into other things like drugs or new products and another round of testing and so on and so forth have to be developed. 10
So if you're really talking about superintelligence then you must think that all of these different things can be done by an AI model within a 20-, 30-year horizon. Again, I find that not very likely. Allison Nathan: Your colleague David Autor and coauthors have shown that technological innovations tend to drive the creation of new occupations. Their stat is 60%
of workers today are employed in occupations that didn't exist 80 years ago. How does that dynamic factor into your AI productivity and growth calculation? Daron Acemoglu: I think that dynamic is very important if you are going to think historically about say, for example, how digital technologies and the Internet have impacted things. But that is not a law of nature. So it's about what types of technologies we have invented and how we have used them. So again, my hope is that, in fact, we can use AI for creating these sorts of new tasks, new occupations, new competencies, but there is no guarantee. So we talked about the scientific discovery process, for instance. So my scenario for doing that right would be
exactly by creating 11 new tasks for scientists rather than scientists using intuition for coming up, say, new materials which then they test in various different ways. If AI models can be trained to do part of that process then humans can then be trained to become more specialized and provide better interactions, better inputs into the AI models. And that's the kind of thing that I think would ultimately lead to much better possibilities for human discovery. Allison Nathan: Right. Well, the investment in AI is absolutely surging. Daron Acemoglu: Yes. Allison Nathan: We have equity analysts forecasting a trillion-dollar spend. Is that
money going to go to waste? Daron Acemoglu: That's a great question, and I don't know. So my paper and basic economic analysis suggests that there should be investment because many of the things that we are using AI for is some sort of automation. That means that we are substituting algorithms and capital for human labor, and that should lead to an investment boom. And that's the reason why my numbers for GDP
12 increases are twice as large as the productivity increases because economic theory suggests there should be an investment boom. But then reality supervenes on that and says, when you have an investment boom, some of it is going to get wasted because some of it is going to be driven by things that you cannot do yet but people attempted. Some of it may be driven by hype. Some of it may be driven by being too optimistic about how quickly you can integrate AI into your existing organization. On the other hand, some of it is going to be super useful because it's going to lay the seeds of that next phase where much better things can be done.
So I think the devil is in the detail. So I don't have a very strong prior [?] as to how much of that big investment boom is going to get wasted and how much of it is going to lay the seeds for something much better, but I expect both will happen. Allison Nathan: I then spoke to Jim Covello, the head of Global Equity Research at Goldman Sachs, and a longtime watcher of technology trends as a former well-known semiconductor analyst. He drew some pretty surprising
13 insights from past tech spending cycles when I asked him whether tech companies are currently overspending on AI. Jim Covello:: The biggest challenge is that, over the next several years alone, we're going to spend over a trillion dollars developing AI, you know, around the infrastructure, whether it's the data center infrastructure, whether it's utilities infrastructure, whether it's the applications. A trillion dollars. And that is the issue in my mind. What trillion-dollar problem is AI going to solve? This is different from every other technology transition that I've been a part of over the last 30 years that I've closely followed the technology industry. Historically, we've always had
a very cheap solution replacing a very expensive solution. Here, you have a very expensive solution that's meant to replace low-cost labor. And that doesn't even make any sense from the jump, right? And that's my biggest concern on AI at this point. Allison Nathan: But isn't technology always expensive in its nascent stage, and then you improve, you evolve, you iterate, and the cost comes down dramatically? 14 Jim Covello:: Yeah, not always. Let's take ecommerce and the Internet as the best example of this. From the get-go, right, you had a very cheap technology, ecommerce, replacing a very expensive brick-and-mortar retail solution. Amazon was able to sell books from the
first day that they started selling books on the Internet because it was cheaper to sell over the Internet than it was for Barnes & Noble to have retail stores. That was cheaper from the beginning. Like, so there's a real-life example of arguably the most important technology development of our generation, ecommerce. That was cheaper from day one. Fast forward 30 years,
right? And it's still cheaper. We still have a cheaper solution replacing a more expensive solution. Take, you know, Uber replacing limousine services, right? So you started cheaper, and 30 years later the Internet is still enabling things to be cheaper than what the incumbent solution is. There's nothing about AI that's cheap today, right? And you're starting from a very high cost base. So that part I think there's a lot of revisionist history on about how things always start expensive and get cheaper. Nobody 15
started with a trillion dollars. And there's examples of when there's a monopoly on the bottleneck of the technology, the technology costs don't always come down. I'll give you an example. You know, the main bottleneck in making a semiconductor is lithography, and there's only one company in the world, ASM Lithography, that can make advanced lithography tools. Lithography systems, when I covered semis 20 years ago, were in the tens of millions of dollars. Now, a single lithography system can cost in the hundreds of millions of dollars because there's only one person that can do it.
And right now, Nvidia is the only person that can provide GPUs that power AI, and that's why AI is so expensive. It's really the GPU costs, the number that you have to use in order to run the data centers and then how much the chips cost. I think a big determination in whether AI costs ever become affordable is going to be whether there are other players that come in that can provide chips alongside of Nvidia. If one wants to argue that we're going to see costs come down significantly, it's going to be because other providers like 16 Intel and AMD come alongside Nvidia and are able to make GPUs that can be used in data centers and/or the data center, the hyperscale providers themselves like Google and Microsoft and Amazon, are going to make their own chips. I think that's a big leap from where we are today. There's certain pockets of semiconductors that those companies can compete with Nvidia in, but they haven't been able to take over the dominant GPU position that would enable a more competitive cost environment where Nvidia would have to be more accommodative on pricing. And so I think that's a big question
mark. I think there's a lot of complacency on the part of the tech world that costs are going to come down, and I don't think that's a foregone conclusion. Even it does come down, the starting point of how expensive this technology is means costs have to come down an unbelievable amount to get to the point where this is actually affordable to automate some of these technologies. Allison Nathan: Right. And ultimately, you don't really have a lot of expectation that it will be able to perform in terms of cognitive ability close to humans. Do you see real limits to the technology relative to the promise that some 17 people are purporting? Jim Covello:: Many people want to say this is the biggest technology invention of their lifetime. I just think that's, to me, almost a silly starting point for all of this, right? How can someone say this is bigger than when we first put a cell phone in someone's hand or when we first put the Internet in front of someone or, frankly, when we first put a laptop in front of someone, right? Like, those were transformative technologies that were fundamentally enabling you to do something different that you had ever done before. You couldn't make a phone call from
wherever you were. You couldn't compute from wherever you were. And, you know, relative to the Internet, you could buy something over the Internet that you used to have to go to a brick-and-mortar store for. Allison Nathan: Got it. But when we first came up with cell phones, I don't think anyone understood how transformative it could be. So why are you so confident
that this won't be just as or more transformative? Jim Covello:: I think that's revisionist history, too. Like, I covered semiconductors when the smartphone 18 was invented, and I sat through hundreds of semiconductor presentations where they showed the road map right away, like, from day one of the smartphone. "Here's everything that this is eventually going to be able to do." And it was out in the future that we were going to be
able to do them, but we had identified the things that we were going to be able to do. Like, immediately upon the advent of the smartphone, people said, "Well, we're going to have our GPS in the smartphone," right? Because at the time, you would have your Hertz rental cars that had those old clunky GPS systems, and they would show that and they would show your iPhone and they would say, "Here's the road map of what this is eventually going to be able to do." Same with health applications. Same with Internet. Same with a lot of these things. But AI is pie-in-the-sky, big picture, "if you build it, they will come," just you got to trust this because technology always evolves. And we're a couple years into this, and there's not a single thing that this is being used for that's cost effective at this point. I think there's an unbelievable misunderstanding of what the technology can do today. The problems
that it can 19 solve aren't big problems. There is no cognitive reasoning in this. Like, people act like if we just tweak it a little bit, it's somehow going to -- we're not even in the same zip code of where this needs to be. Allison Nathan: Even if
the benefits and maybe even the returns don't justify the costs, do the big tech companies that are spending this money today have any choice but to engage in the AI arms race, given the competitive pressures? Jim Covello:: Yeah, great question. I really think that's an important one. And I think the answer right now is, no, they don't have a choice, right? Which is why we're going to see the build-out continue for now. That's sort of what the technology industry does. Like, look at virtual
reality. This would not be the first technology that didn't meet the hype, right? That's the other part of historical context that I think is so important. People act like those of us who think this might not be as big as some other people think it is or naysayers on technology. No. There's
just historical context. There was a period where nobody was ever going to go need to see a 20 house again in person if they were buying it because they were going to use virtual reality glasses to look at that. Blockchain was supposed to be a big technology. A metaverse was supposed to be -- all
the money that got spent on metaverse. Those things are nonexistent today from a technology use case standpoint. And just because the tech industry hypes something up doesn't really mean a lot. But to your exact point, we're going to keep building this for the time being. In the eyes of the tech industry and, frankly, in the eyes of a lot of enterprises, if it does work and they haven't positioned themselves for it, they're going to be way behind. So there's a huge FOMO element to this which is powering all the hype. And I don't think the hype
is really going to end anytime soon. Allison Nathan: Right. And then companies outside the tech sector have also started spending a lot of money on AI capabilities. Well, what do the early results from that investment show? Jim Covello:: I think that almost universally it's showing that there's not a lot that AI can do today. And 21
again, there are people on different parts of the spectrum of anywhere from, "We shouldn't expect it to do anything today; it's such early stages. And technology evolves and finds a way" to the other side of the spectrum is, "We're several years into this and by this time it was supposed to be doing something." And everybody's on a different part of that continuum. Right now, there's very
limited applications for how this can be used effectively. Very few companies are actually saving any money at all doing this. And that's where I think you get into the how long do we have to go before people start to really question? Allison Nathan: So what does all that we've discussed mean for investors that are focused on AI over the near, medium, and long term? Jim Covello:: Yeah. I think it's all on the infrastructure side. It gets back to the point that we were just talking about where we're still going to keep building AI. I don't think we're anywhere near done building it, right? The world's so convinced that this is going to be something significant that there's nobody that's even 22 remotely close, in my opinion, to scaling back on the build. And so what I've been saying for
two years is what I continue to say. Keep buying the infrastructure providers. Is it really expensive? Absolutely. But I've never seen a that stock goes down only because it's expensive. It got expensive for a reason. People
believe in the fundamental growth outlook. If the stock collapses, it's going to be because there's a problem with the fundamental growth, not because of the valuation. Allison Nathan: And ultimately, if you are right, we are building all this infrastructure and capacity that at some point won't really be in demand. Jim Covello:: Right. The very nuanced view, right? Allison Nathan: What's that look like? Jim Covello:: Yeah, it looks bad. It looks exactly like 2001, '02, and '03 for the Internet build-out. Like people, again, it's a relevant discussion, right, when they want to talk about that "if you build it, they will come," and when we built the Internet and then 30 years later we 23 developed Uber and all those things are true, right? It ends badly when you build things that the world's not ready for, right? And I don't know that it's as problematic simply because a lot of the companies spending money today are better capitalized than some of the companies that were spending money then. But when you wind up with a whole
bunch of excess capacity because you built something that isn't going to get utilized, it takes a while. The world has to then grow back into that supply-demand balance. So it ends badly if we're right that this isn't going to have the adoption that everybody thinks. But I would say one of the biggest lessons I've learned over 25 years here is bubbles take a long time to burst. So the build of this could go on a long time before we see any kind of manifestation of the problem.
Allison Nathan: What should investors be focused on to see a changing of the tea leaves here that you expect to come eventually? Jim Covello:: I think it'll be fascinating to see how long people can go with the "if you build it, they will come" approach, right? At some point in the next 12 to 18 24 months, you would think there has to be a bunch of applications that show up that people can see and touch and feel that they feel like, "Okay, I get it now; here's how we're going to use AI." Because again, investors are trying to use this in their everyday life, and there was a period a year ago where everybody was pretty excited about how asset managers could utilize AI. And I think if you interviewed an asset manager for this, most of them are going to tell you the same thing, which is, "We're struggling on how to figure out how to use it. We can't really find applications that make a ton of sense."
Again, there's isolated examples, models, and things in that nature but nothing significant. And so I think the longer it goes without any applications that are obvious to people or significant applications that are obvious to people, the more challenge. The bigger thing to me is the corporate profit issue, right? That's what I would watch if I were investors -- corporate profits. And as long as corporate profits are great, companies have money to try experiments. But negative
ROI experiments are the first things to go when corporate profits slow down, so that's 25 what I would really have my eye on. Allison Nathan: So some really provocative thoughts from Jim. I should mention that I also spoke to my Goldman Sachs Research colleagues Kash Rangan and Eric Sheridan, who see it very differently. Eric, our US Internet equity research analyst, says that current CapEx spend as a share of revenues doesn't look markedly different from prior tech investment cycles. And Kash adds that the potential for returns from this CapEx cycle seems more promising than even previous cycles, given that incumbents with low costs of capital and massive distribution networks and customer bases are leading it. But Eric does warn that, if AI's killer application fails to emerge in the next 6 to 18 months, he'll become more concerned about the ultimate payoff of all the investment we're currently seeing. We'll leave it there for now.
Thank you for listening to this episode of Goldman Sachs Exchanges. I'm Allison Nathan. If you enjoyed this show, we hope you follow us wherever you listen to your podcasts and leave us a rating and comment. If you'd like to learn more, visit GS.com where you can find a copy of this report
26 and also sign up for Briefings, a weekly newsletter from Goldman Sachs about trends spanning markets, industries, and the global economy.
2024-07-20 16:25