2024 TechSprint: Generative AI in Housing Finance | Opening Day
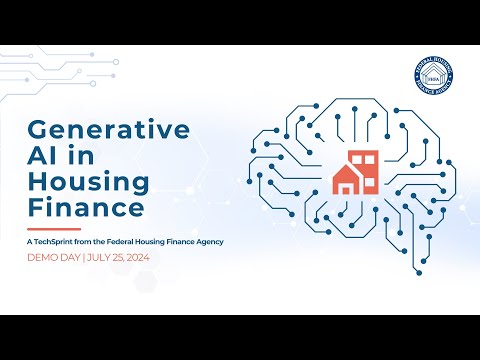
good morning good morning lenders servicers technologists academics entrepreneurs Advocates Regulators all parts of the housing ecosystem welcome thank you thank you for coming and joining us both in DC and on the live stream we're so excited to host you at fhfa for our second Tech Sprint generative Ai and Housing Finance before we begin we' like to remind everyone that today's session is being recorded please make sure your devices are on silent mode and enjoy today's event I'm Tracy stefen fhfa's Chief AI officer and head of the office of financial technology it is my honor to get us started on a 4-day exploration of the potential generative AI may have for solving some of the biggest challenges in housing but we're not just going to identify potential Solutions we're not just looking for use cases our teams all of you are also going to be proposing the control measures that can mitigate the potential risks of using this new technology to achieve recognition teams must not only have the most promising Solutions but also the most thoughtful and appropriate control measures why because we believe mitigating risk is as important as the use cases it's actually not just important it is the key regulation can accelerate the advancement of Technology if we provide the right guard rails to help people avoid dead ends errors and pitfalls if we in partnership with other Regulators develop appropriate policies housing market participants will be able to have the confidence they need to develop and use new tools effectively in a safe and sound manner in other words regulation can help us help the entire ecosystem innovate responsibly before we start I would like to thank everyone in advance for all the work that it takes to put our Tech Sprint together we have team members judges speakers subject matter experts staff volunteers security facilities and Catering to name just a few a lot of people have put in a lot of hours to make this happen and a great big thank you to all of them and thank you to everyone who made it to DC despite challenges so let's have a look at today's agenda first we're going to start with opening remarks from fhfa director Sandra Thompson afterwards I'll get to sit down for a chat with Lisa Rice president and CEO of the National Fair Housing Alliance then Lori Goodman from Urban Institute will talk about the potential AI applications and policies for housing and Housing Finance unfortunately anarie p hippen fhfa's director deputy director of division of conservatorship oversight and Readiness who was originally scheduled to give the closing speech today has been caught up in technology and in the travel delays that hit last week she sends her best and apologies for not being here and asked me to give her final remarks in her place so I'll close us out with those remarks that amarie would have given to frame the events of the next 3 days and Inspire our teams and send them on our way but now it is my pleasure to introduce The Honorable Sandra L Thompson director Thompson has over 40 years of private and public sector Finance experience with expertise in supervision consumer protection risk management and consumer Outreach prior to Leading the agency Thompson served as deputy director of the division of housing mission and goals overseeing fhfa's housing and Regulatory policy Capital policy Fair lending and Mission critical activities for the regulated government sponsored entities which are for those of you who might not know Fanny May Freddy Mack and the federal home loan Banks before joining fhfa Thompson worked at the Federal Deposit Insurance Corporation FDIC for more than 23 years in a variety of leadership positions including as director of the division of risk management and supervision she knows a little bit about risk she is a graduate of the Harvard I'm sorry of Howard Howard University in Washington DC director Thompson [Applause] every time I hear that 40 years it just drives me crazy um we didn't even go back as far as we could have and talk about Goldman Sachs and other places but good morning to everyone and thank you all for joining fhfa's second annual Tech Sprint on generative Ai and Housing Finance I'm so glad we're doing this event again this year we learned so much from last year's event last year we focused on how data digitization could drive transparency and increase access fairness affordability and sustainability in mortgage lending and we saw the power in coming together to inform tangible solutions to ongoing challenges for borrowers our job at fhfa is to to ensure that Fanny May Freddy Mack and the federal home loan Banks fulfill their mission by operating in a safe and sound manner so they can continue to serve as a reliable source of liquidity and funding for Housing Finance and Community investment throughout the economic cycle essential to fulfilling this mission is understanding the opportunities and challenges in housing and Housing Finance as well as the evolving needs of lenders investors homeowners and renters for many years now we've been exploring how technology can help us address those challenges and we want to make sure that new ideas new products and new processes result in positive outcomes for both industry leaders and consumers and we do want to take into consideration both the risks and the benefits in other words our goal is to promote responsible Innovation and Housing Finance despite years of digitization and the ever accelerating evolution of Technology the Housing Finance industry depends largely on paper-driven processes and it's been that way for well over 30 years by harnessing the power of innovation in our industry we can also make progress towards solving longstanding challenges our industry faces such as closing the home ownership Gap and addressing the lack of credit access and credit availability in underserved communities that's why fhfa has been leading in this area particularly within the regulatory Community for example fhfa appointed Tracy Stefan is our chief AI officer earlier this year and we also established an AI steering committee to advise fhfa leadership on AI matters not just for our regulated entities but for our own agency with generative AI already changing the world around us having a chief AI office is critical to helping fhfa understand how emerging Technologies are currently being used how they'll be used in the future and the risks and benefits to the industry and consumers a critical piece to understanding these risks and benefits is working collaboratively with stakeholders as thought Partners to identify best practices Solutions and ideas this is exactly why we host a tech Sprint and I'd like to take a moment to highlight how some of the ideas from last year's event have already made an impact our 2023 Tech Sprint brought together Housing Finance and techn technology leaders to explore Innovative digital solutions to further promote efficiency and cost Savings in mortgage lending the participating teams presented truly compelling ideas for example one idea Advanced the concept of a centralized portal to improve access to down payment assistance programs for potential home buyers and there are now several tools like Freddy Max DPA 1 one which is the down payment assistance one that help lenders more ident easily identify programs for home buyers that they might qualify for there's lots of down payment assistance programs across the country and financial institutions and it's hard when you're a borrower to figure out which programs exist and which ones are good fit for you so by bringing all these down payment programs together in one place it allows the lender to help the borrower figure out the criteria and parameters for the programs that they are qualified for another idea that emerged from last year's Tech Sprint focused on using generative AI to educate firsttime home buyers about the mortgage process today's Tech Sprint continues to build on themes of benefiting both industry and consumers and we're already witnessing the positive impact that technological innovation can have when it's done in a safe ethical and fair manner for example the Enterprises are now using advanced technology to identify positive rental payments and gig economy income sources within asset reports in the Enterprises automated underwriting systems the positive rental payment history programs report ontime rental payments to the credit bureaus from renters who live in properties that are financed by Fanny and Freddy and this has been significant in addition uh FHA has also adopted the positive rental payment program but both Enterprises have already helped nearly 900,000 renters establish or improve their credit scores through this positive rental payment history and this will also help them if they choose to pursue home ownership or not because many renters have either thin credit files or no credit files so building their credit in a positive way is very helpful to making sure that they have credit whether it's home ownership or car or whatever they might need credit for but it helps establish their credit worthiness Additionally the Enterprises automated underwriting systems now Leverage The Power of AI to identify income ources from bank statements for Gig economy workers to support cash flow assessments and increase access to credit for borrowers many people don't get paid every two weeks and many people use other forms of have other forms of income like Tick Tock or uh Uber or pick something that wasn't around 30 years ago and so it's important for us to acknowledge and implement processes that take into consideration how we're living today and we're able to use that with the help of generative Ai and the technology that has been beneficial to Fanny and Freddy in terms of helping them understand some of the income Dynamics so we're really excited about it and we're hoping that you all can see that we are trying to remain thoughtful about the role technology can play Within the Housing Finance ecosystem it's our goal that this Tech Sprint will help us better understand how we can unleash generative ai's full potential in a responsible way and capitalize on this historic opportunity I believe that that depends on our ability to answer two critical questions first can generative AI help us improve the consumer experience when potential home buyers are researching or obtaining a mortgage and second could AI help improve industry operations by enhancing efficiencies or strengthening risk management I'm optimistic about this year's Tech Sprint and I know that it'll help us explore answers to some of these questions and more so I really look forward to seeing how far we advaned this conversation throughout the week I'm absolutely going to be at the report outs on Thursday I'm excited about it I specifically asked as to make sure that we not only focused on single family but we also focused on multif family because the Enterprises and the home loan Banks engage in mortgage lending across the Spectrum so I'm really excited about it I really want to thank you all for joining us today and being a part of this Tech Sprint and with that I'll turn it back over to you Tracy thank you so much thank you director Thompson for reminding us exactly why we are here of the Urgent long-standing and historical challenges facing housing and of the unprecedented potential of technology to help solve them let's take a few moments and imagine the possibilities a home is more than just a house it's the center of your life and the found ation for your future a home is often the largest asset a family owns and serves as a primary way to build wealth that can be passed onto children and grandchildren and regardless of whether you rent or own a home provides you with stability and a sense of belonging in community that goes far beyond its monetary value yet many people encounter challenges securing a stable home renters grapple with housing affordability as rapidly increasing rent costs outpace wage growth leaving half of all renters finding it difficult to afford their rent and people trying to buy a home often struggle with a complicated financing process one where the average cost to originate a mortgage has increased by a third in the past 3 years and home prices have surged over 50% in the past five and the racial home ownership Gap is wider today than it was 50 years ago with these challenges many Americans are at risk of losing access to home ownership and people who prefer the community and flexibility of renting or may not be ready to buy a home are finding it harder to find a place to live no matter what their incomes are but what if we could harness technology to solve the biggest challenges in housing like we do in other Industries to create a more Equitable and sustainable Housing Finance system where renters are able to find more affordable housing where the path to home ownership is more accessible for everyone and where both renters and homeowners are provided with the information and resources they need to stay in their homes through financial hardship fhfa believes this future is possible that's why we've brought together experts from across the industry for a tech Sprint to explore ways to improve housing with generative AI while generative AI holds immense promise it also comes with risks we cannot ignore and realizing its potential depends on using the technology responsibly maximizing benefits minimizing risks and ensuring safety and soundness fhfa believes that together we can foster this kind of responsible Innovation to promote a transp arent Fair Equitable and inclusive Housing Finance system one that meets the changing needs of lenders investors homeowners and renters fhfa calls upon all participants to rise to these historic challenges through collaboration and Innovation because we're stronger together and what we create today will shape the communities of tomorrow what we create today will shape the communities of tomorrow that could be the vision or mission statement for the time we are spending together this week at the tech Sprint I want to thank you again director Thompson for reminding us of that now now it is my pleasure to be joined on the stage by Lisa Rice president and and CEO of the National Fair Housing Alliance to talk about some of those challenges what's being done or might be done to solve them and what we need to do as an industry to ensure the responsible use of technology to enhance equity and uphold privacy the National Fair Housing Alliance is the nation's only National civil rights agency solely dedicated to eliminating all forms of housing discrimination and ensuring Equitable housing opportunities for all people and communities Lisa has led her team in using civil rights principles to bring fairness and Equity into Technologies used in the housing and lending sectors she served on the bipartisan policy Cent's Civil Society advisory Council on artificial intelligence and finreg lab's board of directors Lisa thank you for joining us on opening day of our generative AI in Housing Finance Tech Sprint Lisa [Applause] Lisa thanks again for joining us let's Jump Right In of course my pleasure thank you for having me you've committed your life and work to eliminating discrimination in housing something that the National Fair Housing Alliance counts itself as the only National organization dedicated to doing can you share with us what led you to this area of focus and why are you so passionate about increasing fairness and eliminating discrimination in housing outcomes sure and uh thank you for that question um I grew up in the midwest in Ohio uh and when I was growing up discrimination was a way of life in my community um discrimination was something that we knew was wrong and had a sense that there should be some laws um that made the experiences that my family uh was going through and that so many of our friends were going through um that they should be illegal and that they should not be allowed in our nation when my parents went to purchase their home um uh um in 1963 uh my mother wanted to purchase a home in a suburban community on the outskirts of Toledo Ohio that's where my parents me both of my parents were raised in the gem Crow South so my mother and my father only had a sixth grade education because the schools in Arkansas and Alabama um in the communities where they lived the schools for black children only went up to the sixth grade so my parents uh their educational attainment was restricted because of the Jim Crow laws that existed in those two states so they both migrated actually north so that they could vote because in Arkansas and Alabama they weren't able to vote uh and so uh when they wanted to purchase a home my mom found this house and the real estate agent said to her Mrs I'm sorry I can't even show you the house uh because if I do I will lose my license it's not that I don't want to but I will lose my license and she was not lying to my mother she was telling the truth uh because at that time as you all well know the National Association of Realtors had a an a an a code of ethics that required any real estate agent who wanted to use the multiple listing service and be a member of n they had to engage in residential segregation so my parents couldn't buy the home that they wanted and um ended up living in the community where we lived which was a Redline Community a uh systemically disinvested Community uh so discrimination Hit me hard it hit my family hard uh and I thought this isn't right uh and when I was 15 years old I got an internship at the Toledo Fair housing center and I got bit by the Civil Rights bug and I'm still here today art major undergrad and grad went to school in France I'm supposed to be really in some alternate universe um living on the shores of Ghana painting but here I am well we're glad you're here with us today and and doing such important work so if you think about today what do you see as the biggest challenges right now complicating access to fair housing for both perspective and current home owners there are many challenges unfortunately and I think the biggest challenge that people um are seeing every day are there just exorbitant uh costs of housing both rental housing and housing for purchase in the United States um housing prices have elevated tremendously in part unfortunately sometimes because of AI uh what we're seeing um with um Dynamic pricing systems is that uh they're causing uh an elevation in rental housing rates even when properties have high vacancy rates which does not make sense but it reduces competition right those Dynamic pricing systems I a and so housing costs are are just a major barrier the lack of affordable housing units is a major barrier barrier uh and then we also have other kinds of systemic barriers like appraisal bias we we still have redlining unfortunately uh and other other forms of discrimination uh and systemic barriers that are keeping people uh locking people out of the opportunity to uh secure stable and safe and affordable housing so are you seeing you know anything that has been successful in addressing these challenges in either single family or in the rental space yes we are and and that's part of the work that we do at the National Fair Housing Alliance so we we try to remove uh barriers that are unfair for people and replace them with systems and constructs uh structures that are fair and Equitable for everyone um but because we do have lots of of big barriers that are structural it's hard to break through those barriers I'll give you an example um so for hundreds of years in the United States we have passed race-based race conscious laws that were designed to afford some people in our society access to home ownership and purposefully deliberately foreclose the ability of others to access opportunity uh and so those laws and policies created inequitable Frameworks and systems like residential segregation uh we are still very residentially segregated I explained to you how my family uh was forced into a segregated community that neighborhood is still segregated today right we uh we have in fact we're more residentially segregated to today than we were a hundred years ago because once you build and develop these systems it's hard to deconstruct them right we have a dual credit Market in the United States in which banks are hyper concentrated in predominantly white communities and non-traditional lenders that do not report positive transactional behavior to the credit repositories they're hyper concentrated in communities of color so that dual credit Market is still existing uh in existence today right uh so we have all of these different kind there are plethora of these uh systemic barriers and so we're working to both dismantle them but also build what I call onramps that help people circumvent uh these systemic barriers so that they can onramp into uh mainstream Financial affordable safer uh credit opportunities so one of those things is special purpose credit programs we've been doing a lot of work probably the the leading organization in the nation to make special purpose credit programs possible uh special per credit Pro special purpose excuse me credit programs are available under the Equal Credit Opportunity Act they allow lenders to design credit programs that meet the special needs of underserved uh consumers and people um and you just heard director Thompson talk about uh the increased ability to use rental housing payment information to qualify people well we we really don't have uh an infrastructure to allow people to use rental housing payment information uh and so one of the things that we do is help lenders design programs so that folks can more readily use rental housing payment information to access affordable and Safe Credit opportunities yes as as director Thompson mentioned um we are there are programs we are making small as a as an industry making small progress and uh you know we're here today and and this week to work uh and to see what generative AI right and I know you've spoken a lot about artificial intelligence in the past and I I had the pleasure of hearing you speak on it and I would love for you to talk tell our audience here in the room and and watch watching on the live stream you know when Ai and gen AI you know this ramp up in the past two years what were your initial thoughts and then you know have have have those initial thoughts evolved um over time oh that's an interesting question uh my initial thoughts were one uh ones of fear and trepidation to be honest with you uh these systems are highly powerful and and I was afraid that entities would release systems that were unconstrained which has happened as we well know uh unconstrained models are wildly dangerous and we have no laws we do not have a sort of um Omnibus or comprehensive statute to govern the area of AI in the United States which is frightening in and of itself so it's sort of like the Wild Wild West and we have to rely on entities that are developing and using these systems to um use responsible AI principles when they're doing it not all entities are doing that as you know so yeah I was very afraid uh and um maybe this is just a part of my nature I don't know but when when I see a problem I want to try and fix it rather than bury my head in the sand I and I think that's what civil rights experts do we don't bury our head in the sand so we got to work to try and uh develop principles that responsible entities could follow if they wanted to do the right thing and we've also been working to try and put in place legislation to address these issues as well as try and help uh people understand how EX existing laws can be used to protect consumers and to ensure these products are safe did you want to talk a little bit about the framework and kind of the Partnerships that you're developing to advance the responsible use of AI sure uh so we developed a framework a couple of years ago uh for it's the state-of-the-art uh framework for auditing uh algorithmic based systems it's called purpose process and monitoring I've been to told by a number of marketing professionals that we need to change the name so working on that you'll see 2.0 sometime soon uh but it is a it is a comprehensive framework uh that helps uh entities audit their systems responsibly from Soup To Nuts so so from the pre-development stage the development stage and post uh deployment stage you can use this framework to make sure that you're accurately and responsibly monitoring your systems so that they are not perpetuating harm in our society uh we also are doing a number of things we I I serve on the advisory Council for the leadership conference on civil and human rights uh uh Center of Technology uh and so we're working on policy Frameworks there um we also are working with Federal uh uh regulators and federal enforcement agencies to help train this and also to help them understand how uh you they can use existing laws and policies to help sort of police and provide oversight in this area um and I I we held our first responsible AI Symposium earlier this year and we had the privilege of talking to uh the leaders of the the four major federal Regulatory Agencies uh and if anybody missed that you can look on our webs site and and and access uh that panel discussion and one of the things that the regular uh Regulators said was that they do acknowledge that we have existing uh Frameworks existing policies and they do expect anyone who is using any form of Technology AI or otherwise to um to develop and Implement those Technologies within the constraints of existing guidelines uh and they also said that you're not going to be able to say well I the the tech is so complex I don't I couldn't understand it nobody can understand it you're not going to be able to use that as sort of a get out of jail free cart like like you know a pass um or a safe harbor to to um um avoid um responsibility in this area so policies Frameworks and I imagine a world where we do get our act together as an industry so that we can responsibly innovate so I would just love to you know know what you see as opportunities for generative AI specifically to help reduce bias or discrimination or bring Improvement as we've been talking about to the Housing Industry what are your wish lists um well you know it an interesting question so I I I I did say before that um when when generative AI uh models like chat GPT Etc um were introduced into our society it did cause us some fear and um and and a bit of angst um but we soon began to see that we could use these tool tools to actually help people and we could use these tools to help us Advance uh civil rights and fair housing and fair lending uh principles so we're using the tools and what I believe is a very positive way to advance the ball uh we're even using the tools in our enforcement work so we're using the tools to help us uh conduct investigations to identify uh discriminatory practices and to measure the extent of discrimination for example last year we held a uh hackathon in which we looked at automated valuation models the hack hackathon was designed around identifying and measuring bias within uh avms and developing ways uh to uh uh mitigate the bias in those systems so we did find that that AVM models hold uh uh can perpetuate bias and discrimination largely because the models are built on a data set that is uh infused and uh unfortunately with uh a long history of of biased transactions uh and so we're also looking at uh using AI to mitigate bias within systems and and I I can talk about that um um more but we just released research uh around a new Innovative approach for uh uh reducing bias in Legacy systems and for Building Systems in a fair way going forward so we're using uh AI in many many different ways to help Advance the ball and make the landscape fairer for consumers that's fantastic um before I open it up for questions I just want you guys to start thinking about your questions for Lisa because she has been so generous with her time and we do have mic Runners I'll I'll give you guys a few moments to think about your questions but I would like to know for those in the room we have quite a few um lenders uh single family and multif family lender Representatives here and so what advice would you have for them what should they be considering in in terms of their use for Gen and managing risks well one of the things that lenders should be considering uh is what are the policies the existing policies that they have to ensure that they're building Technologies or using Technologies developed by Third parties in a fair way particularly if they are using uh um uh technologies that are developed by a third party they have to be able to examine those system systems uh we brought a lawsuit against um a major financial institution uh a number of years ago in fact in the 1990s that that uh financial services provider it was an insurance company they were using an insurance a third party off-the-shelf uh Insurance scoring system and they had not properly vetted it in fact at the time they didn't even have staff within the organization that could understand that tool so they basically were listening to the sales pitch and the sales pitch said oh no it's fair it's based on data right it's it's uh it's a computer that's generating the decision computers don't see race computers don't see gender and the insurance company bought it and they started using this uh Insurance scoring system that was perpetuating discrimination and bias against uh stakeholders uh and in that case we had to prove that the Discrimination was uh being perpetuated but we also because of the way that civil rights laws are uh the Civil Rights jurist Prudence uh um um in the US is is is sort of constructed we had to also prove that there was a less discriminatory alternative to that model which we did we we could build a fairer model uh and so uh lenders have to make sure like they're not going to get a pass right so they've got to make sure they understand that technology and they have to make sure that they can explain the technology the the the decisions emanating from the technology um they also have to make sure that they have civil rights experts within the organization that are looking at systems and analyzing them through a civil rights a fairness and Equity lens great advice great advice I'm going to turn it over to the audience and I know you must have some great questions out there we have two mic Runners happy to we'll just there we go right in the middle we'll start um just wait for the mic as we do want the folks on the live stream hey there um to uh be able to hear us hi thank you uh I'm sigy Johnson from better markets um everything you've been saying resonates so much with us because once you remove the people uh and you kind of stick to the data that's where kind of you don't see who the person is you see who the borrower is uh but it's very interesting when you say you can have models that actually going to create implicit bias because of how the data is used so my biggest question here is when you look at modeling you have traditional models that have that can be explained like here are the factors that go in here's the relevance etc etc but then now you're having more of neural network models which are totally different because in a neural network you train the model but you can't explain why it comes to a certain conclusion or not as with the traditional models now they do have some benefits because they're able to catch a bunch of correlations with an data that traditional modeling would not catch but at the same time the only way to debate them is you run them again again in a various scenarios and you try to make some conjectures about what's going on so the question here is do you think we can ever get to a position where we are trusting neural networks with actual scoring and decisioning and lending decisions or do you think that's an area that like we we always need the explainability so we will probably not end up there yeah that's a great question I do think that we will ultimately get there and uh here's the punchline it may take AI uh to enable us to get there right uh it may be the case that very sophisticated AI models are what help us understand and explain uh how those neural network systems work uh having said that as we're BU as entities are building these models you have to have civil rights experts on your teams who can understand who analyze and can understand the underlying data used to build that neural network so that you're very clear about whether or not you're having biased inputs going into the system as you're developing it and I can tell you this now if you are using any data from the housing or financial services markets in the United States of America you're going to be using bias data so now what do you do you have to constrain that model that neural network for fairness I and um I I'll talk a little bit about research that we've just released using a an Innovative approach called distribution matching that allows you to constrain optimally uh constrain systems for both fairness and profitability which is what to be let's just be honest that's what uh uh most businesses are looking for so they want to make sure that the systems they are using can can can be optimized uh based on profit but if you optimize based on profit alone you're going to be building a discriminatory model we know that you have to simultaneously optimize your system for fairness I and because the data that we're using to build these systems is already embedded with bias you have to force and compel you have to design it from the outset in a way that is forcing and compelling the model toward fairer outcomes will that system be completely non-discriminatory no it will not we know that but if you're constraining it for fairness you're going You're that is the way that you're going to ensure that you're using a less discriminatory alternative I was talking to one of the executive leaders at Freddy Mack about this this problem that we have it's an inherent problem we can't get away from it I and I asked him I said just based on what you know about the industry when do you think we'll get to the place where we'll be building models Based on data that is not embedded with bias and he said at least a hundred years so that lets you know that if you're not constraining your system for fairness you're probably in trouble which is why we're we're buil we're doing the research that we're doing at the National Fair Housing Alliance at NAFA it's why we're doing that research it's why we're trying to bring more tools on board to help entities constrain their MO models especially Legacy models and third-party uh models for fairness it is a lot of work but it's really necessary that we do that because if we don't do it we'll never get to where we we want to be thank you for that question I think we have time for at least one more question it's all here and then here thank you hi Melissa Cornwell with North Point Bank um fellow ohioan Go Buck um my career has been in single family housing for you know the entirety of it uh however I've been placed on a team meant to discuss multif family Lending um we've got a great team with a lot of subject matter experts but I would love to hear from your perspective where do you see opportunity for fair lending within a multif family transaction especially when the borrower on the other end is typically an investor for profit yeah that's a great question and so um one of the things that we strongly Advocate is that when you are um uh uh lending in a multif family environment that you're including protocol or requirements within those loan documents that will um push us toward fairer outcomes so for example um you know you're going to include things like making sure that the collateral being used to underwrite this multifam uh loan that that collateral is built within uh code and and that it is uh compliant with different codes but you also want to include things like making sure that anyone um who um um that the multifam um housing developer is not going to be discriminating against people who use housing Choice vouchers and that the underlying collateral is going to be built in accordance with our nation's civil rights laws the fair housing act the Americans with Disabilities Act so that there are no accessibility violations and ultimately that that developer will not be uh subject to liability under a civil rights laws uh for building a complex that is not compliant so things like that that you can use you can also if you want follow Fanny May and Freddy Max uh lead and um require or encourage or incentivize that multifam uh housing developer to report rental payments to the credit repositories so there are lots of positive things that you can do to push us toward uh fairer outcomes thank you so much for the question we have a speed round if we have one time for one last quick question and response we I can hear you I'll repeat it uh thank you so much um for sharing your insides my name is sudhir and I have been in the IT industry tech industry for now 20 years working a lot on the AI and ml models one thing that I would like to ask today is about the people part of this entire conversation we have the AI we have the models we have the new tech hitting the markets people need to be upskilled people need to be reskilled we need to have people ready to govern the models we need to have people ready to monitor what is going on we don't need another incident like what happened last week especially with AI hitting the market so vigorously and so fast any thoughts on this ma yeah so our uh purpose process and monitoring frame framework remember that framework I told you the marketers told us we re need to rename uh it includes a people component in it so I encourage you to read that framework people have to be as you said as you suggested we need to include people who are highly trained and highly skilled uh when include those people in the development uh and the deployment of the model that's that's really important because when you have people who are train trained on civil rights fair housing and fair lending laws they can help ensure that those models are going to be built or those systems are going to be designed in a fairer way um people are surprised when I talk about our lawsuit against Facebook they cannot believe that Facebook did some of the things that they did uh so for example if you were advertising for a if you were trying to place an ad for a lending a housing or employment opportunity on Facebook's old system you could literally say I don't want black people to see this ad I don't want Latinos to see this ad I don't want asian-americans to see this ad now that sounds crazy doesn't it but they built and designed the system because the people who were in that designing room were not trained on civil rights they didn't understand our us-based civil and human rights laws uh so those kinds of absurdities can exist if you don't ensure that people are highly skilled um and trained on these these critical issues in as a part of the process of building the system the last thing that I'll say because you mentioned it uh and and I don't know if you meant the outages uh uh that happened this year where you know Banks were shut down and uh 911 systems were shut down and Air Airline systems were shut down um but it's I think it is an example of why we really have to ensure that we're always including a human alternative and if you look at the AI Bill of Rights that human alternative is that fifth component because sometimes the technology won't work and even if the technology is working it may not be the best solution for a particular group of people and so you need to make sure there there is Al always a human live or manual alternative so that people aren't being uh unfairly discriminated against absolutely thanks for the question I want to thank you again Lisa for sharing your time and insights with us I do recommend you all go to the National Fair Housing Alliance website and and check out the resources they are quite comprehensive and there's always more um and give us a lot of things to think about for the tech Sprint teams to think about as they ideate and for us all to think about so please join me in uh thanking Lisa thank you so much for having me thank thank [Applause] you thanks again I would like now to introduce our next speaker Lori Goodman a fellow at the urban Institute where she founded the Housing Finance policy center before Jing Urban she spent 30 years as an analyst and research department manager at several firms on Wall Street Goodman serves on the board of directors of MFA financial and Arch Capital group and is consultant to the amher group she has published more than 200 Journal articles co-authored and co-edited five books has a ba in mathematics from the University of Pennsylvania and an AM and PhD in economics from Stanford University Dr Goodman [Applause] thank you so much for having me here today this is very exciting and I'm going to actually pick up many of the themes that both director Thompson and Lisa Rice talked about the um outline of my talk is as follows I'm very excited about the applications for generative Ai and Housing Finance it has implications both for home ownership and for rentals and we're going to talk briefly about both of them I'm then going to make the point that efficiency isn't always beneficial for consumers I'm going to pick up pick up many of Lisa's themes in AI bias and then talk briefly about what what has been done so far in the regulatory Arena and then conclude with some policy recommendations first to St set the stage AI has the potential to both enhance efficiency and equity in efficiency AI brings the promise of effic of efficiency gains in processing time underwriting accuracy and could improve original ation volumes it can improve efficiency and accuracy while reducing labor costs and by the way improving the consumer experience on the equity Side by incorporating alternative data a point director Thompson made converting unstructured data and using newer techniques for finding less discriminatory Alternatives AI could greatly expand access to mortgage credit so let's talk about AI throughout the single family mortgage life cycle in terms of pre- morgage preparation you can think in terms of chat boxes to answer consumer questions you can think about FHA putting up a series of questions and then giving people a personalized Todo and information list including things like would I be eligible for down payment assistance when you're first thinking about applying for the mortgage rather than when you're actually in the process I am most excited about AI in terms of assessing borrower creditworthiness um generative AI could greatly expand the use of bank statements um as director Thompson mentioned Freddy and Fanny have taken some really major steps in this direction by using bank statement income by using bank statements to detect ontime rental payments essentially the way this works is someone submits a mortgage application if they're not an accept but they're reasonably close the program says will the would the borrower qualify if they had 12 months of ontime rental payments if the answer is yes Fanny and Freddy go back to the originator and say your borrower would qualify with 12 months of rental payments the originator contacts the consumer the consumer agrees to provide the bank statement information they then get a link from the lender's asset verification um provider and they provide the asset verification um provider with the information which is then passed on to Fanny and Freddy this um it would be this is actually a big Improvement o in in the sense that you're using ontime rental payments however it would be even better if it were incorporated into the process from the very beginning so for example one can Envision better propensity to pay measures based on both credit scores and bank statements form free and Guild had a pilot along this this line but it's very very easy to think that bank statements could help augment one's credit score to produce better information on your willing on your ability and willingness to pay as director Thompson mentioned income could be more accurately captured from bank statements than from tax returns this is particularly true for self-employed borrowers it could be used to capture the consistency of income for those with a second and third job it could be used for multi-generational households where some of the income uh where some of the where the rental payments have been made by Borrowers who are not on the mortgage so you can actually think of great advancements in terms of AI and assessing borrower credit worthiness um in terms of home appraisals automated valuation models obviously rely on avms as these automated valuation models continue to improve we can think more about not completely um um eliminating the human appraiser but sort of giving them the AVM data to begin with and saying is the data on the home itself correct is the square footage right Etc um and then is the condition approximately what the AVM model has has assumed um and basically sort of use the AVM model as a base rather than use the human as a base you can think of the use of AVM models in terms of fraud detection borrower um borrow sort of stolen identities and things like that there's been a lot of talk about using avms and servicing you can think um um sort of talking to a chat box when you're in financial difficulty isn't anyone's um idea of fund um however what you can think of is avms what you can think of is AI augmenting the experience when you're on the phone with a human being and we're not very far along with this at all but if I'm on the phone with a human being the AVM could be running I'm sorry the um AI could be running in the background summarizing the information about me it could then B sort of prompt the human being to say have you asked about this have you asked about that so it does have potential but then the question is what training data would you use so I think sort of servicing um while it holds tremendous potential ultimately down the line it's hard to see AI being used extensively in the near term although it could be used as a helper to human beings now let's think about AI in terms of rental opportunities you can think about chat boxes to set up appointments to show Apartments make sure the lock box has the correct code you can think of um lease creation and then you can think of asking AI to just hey can you summarize that lease into one page to improve the consumer experience tenant screening is something where AI could actually be very useful for landlords often screen based on eviction history criminal records credit scores and income but we actually don't know which of the these factors is actually predictive of future pay history so for example I had an EV I have might have had an eviction um an eviction filing 10 years ago is that at all predictive uh um given that I given that I've improved my per given that I've been a very good tenant ever since um so I so here's one suggestion that we need reporting on tenant performance for gsse borrowers and I would really love to see this because you know as we talk about use of data the lack of data really hinders AI in multi in the multifamily space so this would allow for the provision of better information on best practices ensure fair housing and build more predictive systems it should include information on rent security deposits screening done and the results unit size and location as well as monthly rental payments it should include landlord actions such as whether the tenant has receiv received received a 30-day notice on an upcoming eviction filing whether the landlord has filed for an eviction the primary reason for the eviction and was the eviction completed because a huge there's because for many landlords eviction filings are basically a rent collection technique and very few of those evictions are actually completed um this would allow us to do to basically do predictive models for tenant performance it would allow us to identify outlier landlords who evict two much given census track and tenant information so it would be very very useful um back to Ai and and other rental opportunities making maintenance more efficient so when you put in a maintenance call or a maintenance ticket as getting enough information so that the maintenance uh so the person providing the maintenance only has to make one trip out there where there's enough information to predict the issue so your air so what exactly is the problem with your air with your air conditioner is it making a noise what is it leaking what exactly is the problem where do you think the pro where do you think the problem could be coming from so making sure the parts are available there's also a lot of talk about using AI in terms of preventative maintenance that is looking at the average life expectancy of the equipment having monitors in the in the home where it gives some idea of what the of of equipment that's nearing the end of its life and then incorporate and then looking at for example weather saying oh that air conditioner it's on its last leg and it's expected to be a really hot summer maybe we want to replace it now rather than replace it when it breaks in the middle of July um it could be used for fraud detection in mortgage applications in multifam you have situations where properties uh where there's where there's fraud and properties sell for ridiculous prices um given where given other comparisons in the area it could allow you to look at the sales price history very easily and compare it to the area and sort of and help detect fraud in mortgage applications um again it could be used for um un servicing both in terms of rental payments and in terms of mortgage uh in ter terms of mortgage servicing but I'm less again I'm less optimistic in terms of servicing in the near term than many other areas now I'd like to turn to the point that efficiency isn't always great for consumers one party's efficiency can be another party's Misery the case of rate rate set rate setting um those so in terms of setting rents initially those with better information tend to be more responsive to the market in both directions on average rent increases tend to be slightly larger but the difference but the really big difference is the responsiveness you've got better information so you can better gauge the market with AI this better information will be more widely shared and used by a growing number of landlords so let's just look at the case of um large single family operators so the um who tend to have better information because this is what they do they scrape websites they get information from all sorts of sources so um this is this is dbrs data um on securitized sfr rent changes um which is the Blue Line rent range is three bedroom and four bedroom estimates which are basic which are basically the market as a whole so you can see that blue line is much more responsive so when rent R when when rents are going up a lot the um institutional landlords tend to be quicker to raise when rents are softening they tend to be quicker to soften their their rents as compared to um the market as a whole on average the differences are slightly larger on the order of about a half a percent of the year but the real different five about 5.1% for um the institutional landlords about um 4.4% for the rent range landlords but the real but the real difference is in terms of the responsiveness now let's think of other um uh let's take these examples a little bit further we know that in some cases renewal increases can be larger than vacancy increases particularly in soft markets in a hard in a in a hard Market where rents are going up a lot um you want you basically usually give a break to the guy who you want to stay who's been paying their rent um so if rents are going up 12% for the market as a whole maybe you give your existing tenant an 8% increase and you do the 12% increase on the new guy that's moving in however in a softer Market landlords tend to go the other way they say well gee the market increase is 3% but I can do 5% for existing tenants because because they won't move um and you can see that very clearly on this next slide um you can see that the ren that the renewal rent changes tend to be much much more static than the vacant to occupied rent changes that is over this entire period the renewal rent changes were averaged about 5% the vacant to occupied rent changes averaged about 5.6% and this is for um this happens to
be for institutional landlords however what if your landlord could combine this with information about you to further optimize so they observe that Lori Goodman has had the same credit card for the past 10 years she's passed up all sorts of lucrative offers because she's just too lazy to fill out the damn application so they say okay well and this is information that's very easily obtained from Lorie's credit history um so they say well GE Lori is we can you know we were gonna it's a rents are going up on average 3% we're offering most renewals 5% but we can offer Lori 7% because she can't even fill out the damn mortgage the damn credit card application so she's apt not to actually want to move so just so um you know just bear in mind that there is some opportunities um so so you know efficiency and better information isn't always a good thing the you know the question is can AI help with some of the bigger issues in particular housing Supply um and I think you know I think there's potential down the road we're beginning to just see the be S the early signs of it so AI could allow a developer to maximize the square footage or maximize any objective function on a property consistent with local zoning regulations which could help increase density so you're actually seeing this in California there are programs available where they ingest the zoning rules for a particular location they've ingest the building codes and they allow then they come up with a hey here's the maximum square footage um accessory dwelling unit that you can afford to put that you can put on this property that would can be consistent with both the zoning rules and the building codes so I you know I think we're beginning to just see the very early emergence of that um Can AI help affordable hous deals pencil out so affordable housing deals are often quite complex with layers and layers of financing AI could potentially help optimize the capital structure but again you'd need very very good information on who the in on who the investors are and what that and what and what training set to you to use for that um to pick up Le's theme it's very important to monitor bias in the results and the bi and you know and you can have biases in the training data so um you know as Lisa pointed out um AVM um real estate prices which go into AVM models have um contain a lot of data from areas that have been historically redlined a um you know if you if you were doing if you were looking at mortgage credit and you said Okay I want to train based on what was accepted in the past um to the extent it reflected loan officers rejecting black applicants more um then AI would do a great job perpetuating this so it's very important to be aware of the training data you're using um machine learning algorithms lack transparency and interpretability the tradeoffs between fairness and efficiency are extremely unclear and you can have a model that is based that you can there are wide variety of models that fit almost equally well most of the time where the differences in efficiency can be very very minor but the differences in equity could be hu could be huge and the models and the result of the either biases in the training data or biases in the algorithms means that the models could potentially produce disperate impact to neighborhoods and individuals of color so you know just so just to pick up the point on the tradeoff between equity and efficiency if you don't do anything to debias the the the data is you know if you look at model one it has a forecast standard deviation um that's very small on the other hand the circles um the circles have a much higher forecast standard deviation than the square so the model is more accurate but it's more unfair than model two where the circles and squares have a standard deviation that is much more equal between the two of them so essentially you can think of a Paro Frontier that is the tradeoff between accuracy and efficiency um so you um so you have your most accurate model for each degree of unfairness and we're we're measuring un we're measuring um accuracy as the overall forecast standard devia
2024-07-28 05:57