Workforce Plenary Panel with Erik Brynjolfsson, Roy Bahat, and Susan Athey
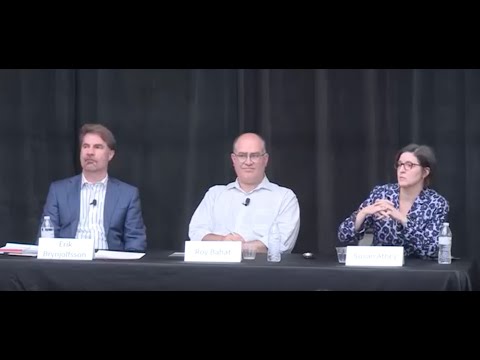
You. We'll, do a little bit of discussion, back and forth before we open, it up so. One. Follow-up. I have for, Roy, is that, you've. Talked about how important, this is and again there's lots of airtime, devoted. To the subject but, yet we don't have you. Know that many household, name success, stories of companies, that totally. Big and then even as you, know we've been searching here, at Stanford for partnerships, from with even medium-sized, companies, that you haven't heard of there's, just not that many, that are surveying a lot of people right now could, you talk about why. That is and, also what, makes you optimistic you. Know to keep investing, well. Some. A lot of things about startups are paradoxes, and so one of them is that in some ways the harder you try to have an impact a social, impact you. May be more difficult to have one and I call this you know the 1% of Amazon or 1% of Microsoft problem which is if you want to have a big impact, shifting. The behavior of some player by a little tiny bit might matter more than all, of the new entrants and so we absolutely do have startups, that, are household names that are active on this issue I mean uber lyft post mates door - you, know just keep going down on the list and so, I think a big part of the question for us in terms of. Creating. A more positive shared. Prosperity is, how, we influence the trajectories. Of those companies one of my views is you might have to get them early and create. An environment, that's more conducive and. Then and. Then but. I also agree with your statement that we have too few examples, of companies that have been explicitly, focused on this and sort of really carried, it as part of their mission I don't. Have any guidance. To offer other than I hope the future will look better than the past and let's find every little green chute that we can and protect it and nurture it so it can grow and. Eric, what do you think and I mean how do you you you know you you put you gave more of a research presentation but. How does, your research inform, well, let me pick up on that last week actually I'm a lot more optimistic in, terms of the, potential I think they haven't been getting as much attention but there are a lot of companies that are using.
Technology. To create shared prosperity inclusive, innovation we. Started something called the inclusive Innovation Challenge at, MIT a few, years ago, and I, was just blown away by the number of companies that entered this million dollar prize. Let, me just describe some of them so you know to make it less abstract, so so, I aura health is a company, that's using technology. Creates a platform. To. Well. Let me describe the problem little bit when. Doctors, prescribe. Treatment. Oftentimes, it's. A good treatment but people don't take the pills they don't change their diet they don't do the exercise they, don't do whatever they're supposed to be doing and most, doctors don't have the time or maybe the skills to. Follow up so our health created a platform to create a layer, of people who have maybe not lots of medical training but they have high skill in other ways particularly, emotional intelligence, they're, good at coaching and motivating, people and then, they follow. Up with the people and they got them to to. Do, the things that were recommended, and they had a huge increase in compliance, and therefore a decrease in costs, the insurance, companies were happier a lot better there's. Another one called 99, degrees custom, that actually is bringing back textiles. To the United States, which. Is an odd thing to do but the reason they do it is they've got a technology, for making much more custom. Unique. Textiles. And they, need some high-skilled workers to, do it so it's not competing, with low-wage people, in Bangladesh but, there's there are literally hundreds of these companies, that are doing little little, things overall, they've raised, over a billion dollars of funding now and affected we counted about 200. Million people being. Affected by I just, don't think they get as much attention in the press as you. Know Amazon or Google or Facebook and some of the the good not-so-good things those companies are doing, and I would love to see more attention, to those smaller, companies. That are using technology, that because because, Minoo. Bring it back to my research a little bit I mean you can use technology, to substitute, you can use it to complement. And to some extent that's a choice and it. It, may be an. Entrepreneur. May be indifferent either way they may make a million or 100 million dollars but, from society's, point of view I think we want to steer them more towards to complement rather than the substitute, with, the substitute, a lot of that wealth. Ends up in the hands of one person or a small group with, the compliment you create a lot more people participating. You. Can do policies, at the government level but to the extent that the government is dysfunctional or, not doing that then, you, also want to encourage entrepreneurs, to do it for free good or just because they realize they can make money doing it and that's, more the direction that we've been pushing I loved your line on. It's. Not gonna be the AI that replaces, the people it's gonna be the people with AI that replaced the people without AI I think, it sort of calls to that yeah absolutely Ann Eric's, point I think is is also a, central. Theme of our new Institute on human centered AI here, at Stanford, with, the idea that just as Rory said that the government.
And The government through universities, are the first venture capitalists, and in some sense we can redirect, the innovation. And AI to be more complimentary, and I came in augmenting, that could, in turn influence, that yuko system that follows so, I also want to come back to something that you that you dwelled on at the end ROI about diversity. Yesterday. On the California. Future of work panel we, had some discussions, about. Challenges. With you, know racism by employers, and the inability, of people with who, except for the people with the perfect credentials, in the top schools and the right race and gender and so on to get those, people had a good time getting jobs but it, was harder for the rest and algorithms, might even if, not, carefully, guided and reinforced, that because they're just going to reflect what the employers. Want and we, talked about yesterday how that's really a two-sided problem, you need to both you, know have, the workers be able to express their. Skills. And also the, employers, trained to understand, them we'll come back to credentialing, I think when we talk in the education, panel but you, went past that pretty quickly in your presentation, could you say a little more about how, we can we can solve that two-sided, problem sure, so. There's. A myth, in the technology, industry that, the product, progress, of technology, has these inevitable, consequences, like algorithms, produce bias for. Example and by, the way I think of it it's more like the weather you can't stop it you can shape it and these. Choices, are the product, of choices, I mean some of them we may be conscious of and some not and so, you know a variation. On what Eric just said about finding complementary. Uses, is let's, find every, possible chance we can to, a elevate. Diversity. Inclusion. Awareness, as much, as we can and be to invent things that actually do something about it I mean I'll give you an example we. Invested. And I'll try to talk about as often as possible about companies we didn't invest in because my intent does not talk about my book but when I believe something and find somebody working on it often end up as an investor in it so there's gonna be you know to your point about conflict, let's be transparent about it but that's the reason we invest in a company called text EO, that. Is to. Microsoft, and Amazon alums, who, took. The notion, of spell checking in a word processor, one step forward spell checking is a, backward-looking. Idea. Right, you've already made an error and here comes squiggly red line there's, is a forward-looking idea, if you, write this job description in this way will, it attract, women, to, apply to the job as the same raise rate as men. And. Turns. Out you can use the data to do that and once you feed that back in and people, discover that there are certain word choices, and some of them are surprising, it's not masculine. Words attract, men you know that the consequences. Can be really surprising and there's only way to get at that and influence, the behavior in real time of the person who's about to hit ctrl, P or whatever, you hit to post it on indeed for. Their job description, is is. To have technology, in that loop well, that's great and it's one of the reasons I actually, more, optimistic, about the. Increased. Inclusion these, technologies, can definitely be used to amplify and. Replicate, the biases, that we already have and that's definitely, a problem but, at the same time I think ultimately they're easier, to fix. And to move forward and to make in a way that is more inclusive I mean the reason that we have a lot of problems in the first place is because humans, are biased. Racist, sexist, have implicit. Issues, and we, work very hard to try to overcome those and get people overcome well and we're. Making some progress but I think ultimately I feel maybe, I'm too much of a technophile.
But I feel like it's probably, easier, to see. The issues in the technology, and then have a touring, box or something that says okay here's the problem let's fix it and iterate, and improve it and the path often can be faster, in that dress yeah I mean I actually don't have a view on whether it's going to be easier or harder I think it's gonna be easier and harder some, ways and harder and others but I think whether it's easier or harder it is controllable, if we pay attention to it and that is the important thing is that we can influence the outcome that's right excellent all right well so let's open it up now to, questions. From the audience we have some microphones that will come around looks, like we have one over here. Hey. There so, nice to see you both I, am, free, to paly I'm the co-founder of a company called, Pye metrics I, just wanted to kind of just I have a question, for you guys but I just wanted to tell you very briefly pi metrics uses behavioral science I used to be a cognitive, neuroscientist, at Harvard and MIT and. Ethically. Designed AI to basically. Match people to roles and I think we are doing a lot of this future for it stuff so we're definitely not Google or Amazon or any of, those big companies but I think we're a. You. Know just. One, solution and I do think that you know we kind of had the premise that you. Can audit artificial, intelligence, but as. A cognitive neuroscientist, I'll tell you you cannot remove bias from humans it's just physically impossible all the literature, will, will tell you that well the question I have for you guys actually is, and. You. Know we work with David autor up at MIT and other academics, I think one of the challenges we've, had two, challenges, I would say one. Is that at least the field of employment we work in is regulated. So there's a lot of regulation, federal. Regulation, by the EEOC that, sometimes, can, hinder, some of the progress and we're actually doing some policy work here. In California, and in New York to try to mitigate that so curious, if you guys have any thoughts on that and. The second thing I think is that you know when it comes to algorithmic bias. It's. Such a hot, button I mean just in the New York Times there was a thing. That came out this, week by ofoma ajuna from Cornell basically, talking about the challenges, and and. I think one of the things that's missing right now I'd love to hear, this audience thought they are really, just some sort of external standards that could say here, is how we can. Create. Ethical, AI or unbiased a or whatever and here's how we don't do it so those, are just two questions I wanted to kind of put to you, guys have you had thoughts. Well. First, off I agree, with your point that in humans. Are also. Biased and that that's something, we shouldn't ignore and, that's why it's sometimes frustrating when I hear people say, let's not. It's. Not not use the Machine. Until it's perfect, we're probably not going to have perfect machines but, they may be better. Well. In fact I'm sure we want a perfect, because. There's. Been research that if. You have a number of ethical. Criteria it's, mathematically, impossible to, satisfy them all at the same time one. Of the interesting, things it does is it forces us to be very explicit, about. What trade-offs, we, want to make and that could be uncomfortable, but. It forces, us to say wait a minute exactly, are we maximizing. Accuracy. Or minimizing. Bias or what which kinds of bias and depending. On what are our values. Are we may make different, choices. When. Humans are doing it it's kind of all very fuzzy, and we, can kind of blur over those moral choices but one of the things that that machine, learning or computers, in general is doing is is making, us be much more explicit, about what trade-offs, we want to make and that's.
Probably In the long run a good thing for us to have that conversation and, do it out in the open and use. Whatever mechanisms, democracy. Experts. Conferences. To, try to hash out what those values are that we care about I mean the the. Broad lesson I take from from the work here is that ours are tools get more and more powerful, that, means we have more power to change, the world sort of almost by definition and, that, means that, our values, matter more, than they did before, and we have to be very explicit about how we want to apply those values. Just. I was gonna jump in on the creation, of standards, of, ethical AI standards, do you have thoughts on standards. Well. I think. One. Of the reasons that we I think we have the human centered AI initiative, at Stanford and other initiatives are growing, elsewhere is that, the technology is rushed so far ahead and that's great I wanted to keep keep rushing head but we are now confronting, all these, questions. We didn't have to deal with before about private it used to be physically impossible for me to look, in your file cabinet right in your office and, now if you have it in the cloud you know we have to have some rules and all. The other seniors so right now there's there's a need, for us through. Multiple, mechanisms through, you, know ethicists. At universities. Public. Conversations. Government. You know candidates. Running for office all of them need to bring up these issues and I, think that's the part of this whole conversation, that's way behind is the. Ethical side the. Riaan, teamwork side all the sort of softer, social, science, side we've, got science fiction but we need to have sort of social, science fiction catching up yeah and I guess my feeling is I think I agree with all of that and I think there's plenty of time to intervene because, for the onrush, of AI I mean you know for, those of those. Of you who work on AI in the room most, of the AI that's actually used in the business world is some form of regression analysis. I mean it's we're not really we're talking about stuff that you know even I failed to do well as an, economist, student so you know you can it's, not really. Happening, so rapidly that, we can't intervene, overnight.
We Can I, am, skeptical, of, organized. Efforts, to try to articulate, some, clear principles, in part, because the state of the art is changing so much in part, because the sources of resources, for them often. Have enough marketing, bias in there that it turns into a marketing, exercise so what's the alternative, well like no I think it's conversation. And development. Of ideas. Around it I mean it's as opposed, to the goal being an outcome. Of a standard, yeah I know I don't think I'm gonna have some experts. In the US Congress where I just testified that sure wave kind of solution about. Their ability to make decisions there, yeah. Yeah no but but I think that as with a lot of these things there's, a multiple, different things going on simultaneously, and. I'm. Glad there's this initiative at Stanford that it's very. You. Know if you think about though. Before you say there's gonna be a set, of standards, we at least need to articulate the trade-offs yes, a lot of a lot of people don't understand, those trade-offs and then actually, just to plug the academic, industry partnership, angle a little bit if someone from Facebook stands up and says oh it's, actually really hard to have fair content, moderation, because if there's more fake news on the right than the left then my, false positive, and false negative rate, can't both be equalized. But somebody says, you. Know that's. Facebook, they elected Trump I hate them or whatever. Or, that's Facebook they're liberal you, know I hate them and nobody. Actually listens, to what they're saying when. In fact they just stated a scientific, fact. But, it nobody wants to hear it coming from you, know the company themselves yes, so with the, part where you do. The. Possibility. Set, said. Yes actually there is a real trade-off and actually even having, people discuss, the value the, values that go into that trade-off outside, of a company can be much more and hopefully that can focus the conversation, so that you know if people think oh let's just make it perfect, and then as Susan said well we, can just prove that you're not going to be able to maximize all these things simultaneously now, you can have a much more focused conversation, yeah, about what's actually feasible yeah, I mean I think one way that I describe. That and I'm really I mean that the the prep up the academic, industry partnerships, are essential for figuring, this out really, just talking about that the end object of, like this is now the rule, set that we will need to follow I don't know an analogy, for that from, any other place and I'd, say one way I think about it is trust which, is to say when, you hire a new person onto, your team you, don't let them make the team's most critical, decisions the first day and the, ultimate, black box is the black box of inside the human mind we are no more auditable, than, you, know a complex. Machine. Learning model, it and it that analogy, I don't want to overdo it because we don't anthropomorphize the, machine too much but I do think it works in a surprisingly, wide variety, of cases which is to say if you have an algorithm that you think is capable of making an important decision test, it gradually first hit the bumps realize the trade-offs I mean we need to trade craft around this my partner James, Cham calls it we need a Peter Drucker of AI because, his view is that the sort of line managed, goal oriented way, of doing things just doesn't apply in the continuous, feedback loop.
Of Decisions, that data, leads to better decisions leads to more data etc and I think we're developing, that managerial. Practice, as an industry, all. Right so another question from the audience over here, so. You wanted somebody from Facebook to stand up. But. I'm gonna ask a completely different question so Eric. You spoke about which. Tasks, and which jobs can be automated by machine learning right I'm wondering, about the gap between that and technology. In general are. There other technologies, that are behind, the corner around the corner yeah, or, even within the, broader scope of the eye that, would actually change the numbers that you have or are we hitting some, uniquely. Human capability. That is not automatable, and if then. What if we had computers, that could understand. The motion show compassion how. Would that affect we already have those sorry, Tamagotchi you have kids. So. We. Pick machine learning because we'd. Spent a lot of time talking to folks and there was really a kind of a quantum, improvement. In, the past eight years or so but that said we are not taking this methodology in applying to a number of other technologies so, we're doing it right now with robotics, because, machine learning is mostly cognitive. Task perception, and cognitive tasks and there's a lot of physical things that there, are some folks especially here in Silicon Valley who are very, optimistic about, the improvements. That may happen in robotics in the near term there others that are more pessimistic but, we're looking at at those, and then we, should have done this first probably we're, also doing a set a rubric, for, traditional. You call a good, old fashioned of AI or procedural. Knowledge the kind of coding that that really, dominated, into created a multitrillion-dollar industry, over the past 30 or 40 years that, has not played out entirely, anywhere, nearly, either. So, we will eventually have these three different rubrics that we we run in parallel, you. Know as, to whether or not we'll be able to the full spectrum of human things I mean we're getting. It's. Worth just briefly, especially on my, take, is that there's. Nothing there's no law of physics that prevents that from happening, someday. And I think that's why a lot of people are very focused. On that but, more importantly, I don't. See it happening any time soon, I don't see it happening in, the next five 10 20 30 years you know what Pollock used to Ray Kurzweil, and others and. So the problem I think we need to focus on right now is, what are the set of tasks, that are likely to be affected, currently. And I. Don't think, that we're going to have mass joblessness, or, most jobs, or most tasks, being, eliminated it's, much more a matter of major, restructuring, and then how do we make sure that the income is there for, those people whose jobs are affected. And that kind of refocus is the types of solutions you might want to focus on the kinds of problems you want to focus on rather, than this, scenario. Where where there's nothing left for humans to do. My quick. Question for Roy this is my name is moses i work at orange. Which is a, telecom. Company in europe sort, of like a verizon, in the US and my, question, for roy and you and i we kind of talked in our group here a little bit about impact investing you. Had a really interesting slide that said capital, of all patients, is and i'm, wondering if you could just comment a little bit about kind. Of the state of where we are in venture, capital broadly. And we're definitely at sort of a certain. Point in our cycle i think but. At the same time how can fund, managers, who have fiduciary, responsibility. To limited, partners, really. Drive towards. Thinking about, unintended. Consequences. Bias, and how can, an investor, begin. To do diligence on companies, to help sort of safeguard. Some. Of these risks, and think about those if you could just comment a little bit on that'd be great wow, there's, a lot of you thank you for raising moses is an essential, set of questions and there's a lot in there. Well. First just a general comment on the state of VC, there.
Is So. Much activity that, if we can't fix these issues now it's. Hard to imagine better conditions, under which to address them you, know, so. That's one thing I'd say that's why, I have a lot of focus and attention on trying to carve this right now second. I. Think. There's a trade craft emerging. Around how to understand. The consequences, to the world of what you invest in, that. Is really powerful, and important and I'll give you examples of things that I don't think work I think, there's this sort of guns and butter Pareto, frontier. Analysis. Of like well maybe I'll trade down a little bit on impact on return. But I'll have more impact I'm not quite sure how that works because. I don't think that it measuring. Impact is not a single number you, know as we were discussing earlier and, I, just can't quite imagine that trade off and it seems like you more often than not with all due respect to any impact investors, in the room you, can end up with organizations, that are neither fish nor fowl like, I'll tell you when we invest in the company that we believe is gonna have a lot of impact and an impact, investor, shows up sometimes depending on who the investor is that's bad news because. We're like they're not gonna behave rationally. You know in an economically, rational way they'll be unpredictable they'll, have motives that are harder to discern like, we invest to make money not because making money is the most important thing but because then at least somebody can discern what, I'm solving for in, any given situation now what I do think works is limiting. The scope of companies you'll invest into things that you are good with so for sure lots of people avoid obviously, immoral. Things but. Avoiding. More things you. Know we passed on a company because we didn't feel comfortable with what that founders, approach. To privacy was and one, of my I'm I'm. A believer in the stakeholder, capitalism. Idea. But. One of the places where I think shareholder.
Capitalism Deserves. You, know a sympathetic. Look is it, all depends on what time horizon you're solving because eventually all of those stakeholder, threats become. Root threats to returns too so. There's that and, then the last thing I'd say is as investors, in early companies we do have a lot of influence. You. Know we don't have as much control as people think because, the founders, have lots of choices about where to get money from but. We certainly have a seat at the table and we. Certainly have the ability to set norms and expectations as, a community, and so I think it might be those levers as opposed to some magic model of like well if you only set up your fun this way everything. Follows from the structure, I don't, know those solutions but I do know the solutions, of an emerging trade craft. All. Right so we're, closing. To the end maybe we have do we have what somebody else have a microphone, did, we I'm sorry did was there somebody else waiting for a question, so somebody have a final question. All. Right over here. Thank. You, Patrick. Brothers and I know, it takes the next panel but how do you guys think about workforce, as, it relates to education because, it's, getting blurry, yeah we had this discussion over email and preparing, for this that we couldn't figure out what the line was ourselves, so we basically said well we'll just talk to each other about what we're doing now me oh I mean we had something around like well if you call yourself education, then you're ed tech I'm the, answer is it's a it's a set of continua that all overlap, and I don't know I'm not sure what hangs in the balance of answering, that question is part of why I kind, of struggle with it so I'm not sure why the answer matters. Well. I have, the same view but I think it matters tremendously and, the reason is because there's they are if they are they'll fit together, so, well and as, you, learn about which kinds of workforce changes, there are I, think one, of the most important things on my list maybe because I'm an educator is you know so let's think about educating, people to have the right set, of skills in fact that machine learning rubric that we developed, I mean some. Of the companies wanted to know where machine, learning was going to be effective but the interesting was most of the companies we talked to we're more interested let's, look at the inverse, of that what are the things that humans will still be doing that's what they really cared about because they want to focus their training in education and, so there are some areas that. That's going to be very important, one. Is in creative, work you know figuring out large scale problem solving asking, the right question, because machine learning systems can't do they were great once you have it structured but, humans can arts. For now needed, to figure out how circular the other huge category, is even bigger I think is and. Roy alluded to this is you know EQ, or interpersonal, skills, it's not like there's just one dimension of intellectual. Skills that are important. And on for the, ones that the machines are the weakest in are in, coaching. Leadership. Persuasion. Selling. Negotiation. Caring, these. Are things that you don't want a machine learning system to, be trying to do but there's a lot of work in society that needs to be done and many of those also can, be tried to taught or brought out so, I think there's a whole set of things we're gonna have to reinvent education we're. Trying to at different universities to focus more on formulating. The problem working. In teams coordinating. An inch and we're. Motivating, other people. Those are the kinds of skills, that would be increasingly, - before although I do want to say I just very briefly when when Roy. Dissed, teaching, coding I usually don't have that on my list but I want to put it back on the list just because usually I'm on pan with a bunch of technologists. Who are you. Know Danielle, or roost might my dear friend who's the head of the AI lab at MIT, talked. About turning coal miners into data miners first. I think that's going a little too far that's definitely going too far I think that not everyone was going to become a a coder, but I do think that there there's a reason that that's so much in demand and it's not just because tech, people want to make more people like themselves it's, because they're paid incredibly, well because the market is saying we, do have a shortage it's, just that I don't think that that's, ninety, percent of the workforce most of it ninety, ten is the other two things I talked about but I would still reserve a really valuable.
10%. For the coders, I accept, all the caveats to the disc but if I can am I gonna just add one more second. Which. Is these. Questions. About what's left for humans I call it the last job question, they. Tend to bring out all these emotions in us because it's scary, to think about it and there, is a bedtime story that, like empathy and, and and. The, the the the human, skills or will be left for us first of all I think that's nonsense the machines can totally, be empathetic and I'm. Happy if you want to talk. About you want a robot, taken care of. Yes. I mean, yes in many ways but but we already expressed, empathy toward machine cliff Nasser at Stanford wrote a great book on it the man who lied to his laptop we anthropomorphize, the machine but back, on our language when, we talk about those higher order skills especially, in a university, context, the implicit assumption there is really higher order skills of working for companies like ours which, is to say CEO. Information. Industry jobs I was, just talking with one of my fellow Commission members Tom, Kalil got the call out earlier, from. Schmidt futures, yesterday, if you want to see another version of that skills, remaining, for humans Fresno, has the first public high school for entrepreneurship, in the United States it's a vocational, school 50, like America, half, and half go to college don't go to college and their, take on it feels, much more like how do you manage risk in your life how do you persuade, somebody. You, know allow you to do something, how do you not. Just starting tech companies but so. I agree with all of that with a twist. On the valence, of how it's done so that it's for everybody not just for folks like us. You.
2019-11-27 03:16