Trappes – Big data in behavioural biology – DS² 2021
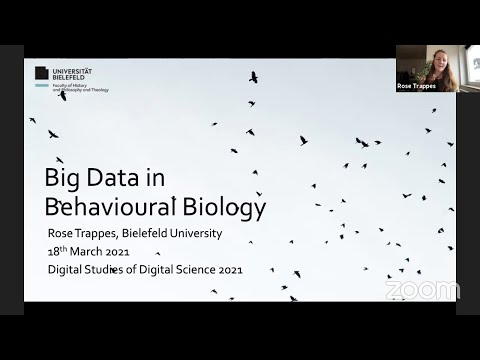
As usual, wait for just a second. Okay, and we are Yes. And everybody seems to have come across from the last session. So Welcome back, everybody to the next talk of the day, the second talk of our final day that I see in our in our crowdcast, scheduled for the 20th session of the conference. So it is my It is my pleasure and honor to introduce Rose traffice, who's also by the way, if you're if you are following the conference on Twitter is doing some amazing live tweeting for us. We're
going to keep going with this theme of of the role of data in science. So I will be talking to us today about big data in behavioral biology. So without further ado, please take it away. Great. Thanks, Charles. And thanks for organizing the conference. But you and to Luca, it's been really fun. And thanks to all the speakers, I've had a really good time and learned a lot about digital studies of science and also digital science. I'm going to be talking more about the digital science
side of things. I haven't done any kind of digital study of this, aside from being in zoom meetings, lots of zoom meetings with the research group that I work with. So it's coming out of an ethnographic study. But at the same time this this project
in particular is kind of right at the beginning, I haven't done any formal work, investigating it. So I'd be really happy to get feedback and ideas on the project in the question time. Yeah, so I'm going to be talking about big data in behavioral biology. We can start off with a black bird. And you might wonder what does the Blackbird have to do with the International Space Station? Probably not much in normal cases, but this Blackbird has a small device attached to its back, that is a radio transmitter, it's sending signals of the bed position coupled with a timestamp to a receive a station in the International Space Station, which is then sending that data back down to earth to researchers in their labs. That Blackbird is part of a really big international research project called Icarus, which has been running since for about 10 years now. It's a international cooperation, looking at animal
movement, and animal movement of lots of different sorts of species, part of the project has involved developing the technology to do this. So they've had teams working on miniaturizing, radio transmitters, and then also lots of teams out there, attaching them to animals and looking at what happens. It's also because there's a receiver station in the International Space Station, it's funded by space agencies, it's funded by national organizations, it's a huge thing. And all of the data gets published on a database called
move thank that's been around since earlier than this project. But it's obviously received a huge input of data from this project. And I encourage you all to go and look at that database. Because for nothing else, there's a beautiful visualization of all of the data that's coming out of these animals. And that's a screenshot from it, so you can see what's going. So if I haven't made any visualizations, at least somebody has, and you can get something out of it. What I find
interesting about this project, apart from pretty graphics, is that there's a lot of interesting claims being made about the use of these, these devices, and how they're changing behavioral biology. This is taken from the Icarus website, from a site that is called the Internet of animals, which raises already some interesting points about digital science and digital nature. But what it's about is the the way that this project is changing the science. And there's some
interesting claims here that I'm going to investigate in a little more detail during the presentation. So they say things like, in the future, these transmitters will be able to tell scientists much of what they previously previously found out only after hours of observation. The researcher in certain cases is sitting 1000s of kilometers away in his Ohio research lab. The process, the progress that they've done in this technology, it facilitates completely new insights into nature, and it allows tracking animal movement in real time, and on a large scale with 1000s of animals. So some of these
points, things about the researchers think 1000s of kilometers away from these animals moving around the ability of these devices to cut out To make things efficient, to deliver this information ever to scientists so that they can, they don't have to work so hard for it, and also to be able to generate new insights that maybe they wouldn't have been able to get with the regular methods. All of these claims, quite interesting and quite powerful claims. Obviously, it's a little bit advertising speak. But there's also it's also
indicative of the way that biologists themselves tend to approach these technologies. So I want to start this presentation by kind of tracking back a bit and looking at why these technologies are so interesting to biologists. And that's because they're kind of they're such a big departure from traditional ways of doing ways of studying behavior. Then I'll briefly introduce these technologies and look a bit into the kinds of benefits they deliver, because they do kind of they are really good for behavioral biologists. But on the other hand, they're not maybe sometimes as good as some biologists would like to think they are. So I'll spend the final part of the presentation, looking into how biologists deal with this movement data together with statisticians. And how that
means that this kind of these kinds of ideas of researchers sitting in labs just receiving movement data is too idealistic. To start with naturalism in ethology. This is Conrad Lawrence and Niko tinbergen. They're the founders of ecology, two of the founders. And they were well known for being great
naturalist, they love to go out into nature and see the way that animals were behaving. And they use this method, they kind of capitalized on this love of exploring natural behavior, and develop that into the method and ethology. So they encourage their students to go out until look at natural behavior. And its natural causes and functions. That's what ecology was about. In contrast to some of the other the American ways of looking into animal psychology. They, they aimed to
kind of do this really detailed observation of animals in order to develop a film familiarity with the habits of the species, the one that usually tends to do, and only then they argued, could you start to figure out what the functions of different behaviors were, what their causes were, how they fit into the animal's way of life as a whole. There's a lot more I could say about this. But this is enough to get the picture of kind of where behavioral biology has come from at least one of the places it's come from. And if you'd like to see some fun
pictures, I do encourage you to look up current Conrad Lawrence on Google Images, because he loved geese. That's, that's the one thing. Okay, so the ecological approach sounds painstaking, because it is painstaking going and watching geese for hours on end. It's hard. It's hard work. And in particular, it's not easy to get kind of a more objective method, when you're just going and looking at animals. As a result, there's been a lot of ways of a lot of tools that have been developed to, to study animal behavior. in a way that's kind of objective in the sense of comparable across different individuals, different humans. One of the most important tools
is ether grams, they're kind of lists of behaviors that have been selected for being kind of relevant to the species with a code. And that means you've been you're observing the animal, you sit there with this sheet of paper, and you write down the codes with a time for whatever behavior the animal is doing. So this allows into researchers, individual researchers to quickly record what animals are doing. But it also ensures that there's intercooler compatibility. And they do tests
with that. So you sit there with somebody else, and you both look at the same animal and you check that you're all caught coding the same stuff. There's also bird ringing, which is kind of it's not really an ecological tool, but it's a way to track animal behavior, and how they're going out into the world. So you attach an identifiable little plastic ring around the foot. And then people out there in the world who like watching birds, they'll tell you when they see it. And this helps you keep
track of where animals have gone, whether it's maybe settled in a territory where they're migrating these sorts of things. That's again, another way to to kind of keep track of animals in a way that's a bit more easily easy than just looking with the Bear eyes. Finally, more recently, people have developed software and our codes and things like this for processing videos. And this can help you to get, like computer automated measurements for certain sorts of behaviors that are fairly simple things like jumping distance or jumping angle of a grasshopper, in this case. So these are all pretty powerful tools, and some of them have more new, some of them are quite old. The tool that I'm most interested in, obviously, is
these technologies used to tracking animals. There's various sorts of technologies that are used for this, this tracking movement, there's radio transmitters, accelerometers, GPS tracking devices, and there are others the basis, probably the three most dominant things that people use. They've been used since the 1950s, and 1960s, actually, to track animals. There's a really good history of this by Etienne Benson. But it's only in the last 20 years that they've started to be used on a broad scale. And that's because they've become because of other technological developments that become much smaller, much more inexpensive. And it's been much
more easy to get the data out of them remotely, rather than having to find the animal again, and kind of extract whatever information is recorded in an internal program processor. So because of these massive changes in technology, suddenly, you've got 1000s and 1000s of studies being done using these these devices. And you can see there, some of them are really, really tiny, like the one that was on the blackboard at the start of the presentation, the size of your finger, some of them are a little bit bigger, like this one that's on the first day, I'll pop there you can see it's about the size of the palm of your hand. But both of these are designed for like a particular organism so that it will fit a particular species and won't obstruct its behavior as it's moving around in the environment. There are a lot of benefits that you get from using these devices. Obviously, one of the biggest things is that you
can have a larger sample size. Because they're cheap, you can buy lots of these devices. And all you need to have the manpower to attach them to the animals. But sounds simple, it's not always that simple. Obviously, manpower in
Antarctica is not quite as cheap as manpower in your backyard. But you can get much larger sample sizes. Regardless, you can go from say dozens of animals in a normal behavioral study where you're sitting there and watching animals to 1000s. quite easily. You also tend not to lose the devices as easily as you would with say bird rings they you can have with bird rings, you can ring a lot of birds, but you often lose them, you never see them again, you don't know what's happened to them. With radio transmitters say you attach it to the animal, and it's constantly relaying information back to you. Even if
a human doesn't see it again. What I think is more interesting benefit of these technologies is that you can get much more variety. And that's not just kind of within a population, because you've got a larger sample size, we can also then capture more of the variation in a population. But you can also get, you can study behavior under different sorts of conditions. Say, it sounds trivial, but you can't watch what an animal's doing when it's underground, when it's underwater, or in a storm or in inclement weather. Unless you
have kind of special expensive technology like not night vision cameras and things like this. So these technologies, once you attach them to the animals, then you're able to track them in places and under conditions that aren't easy for humans to observe. Part of this then means that you can get different sorts of species. So a lot of in the past, a lot of the behavioral
studies were done on animals that were active during the day, that were kind of easy to keep track of maybe they had a fairly small territory. And these days, you can do this kind of large scale tracking of animals of all sorts. So you can get studies of the first 30 seals, for instance, the moms go out for days on end, and in the past, nobody knew where they went. Now you attach trackers, and you can find out where they're going to forage. And this is an I think, quite an interesting benefit because we were so used to as philosophers thinking about lab science where you're dealing with these tractable Animals.
Here, you're looking at a different kind of broader set of species that are trackable, as Benson puts it. The third advantage that I'll just cover quite quick quickly is that you can get longitudinal data. So in the past, it was quite difficult to track the same animal over time, unless you were able to ethically attach a label to it, or a tag these days, with the trackers, you put the tracker on, and the tracker does the re identification for you. And this longitudinal data is obviously very interesting for biologists, who want to find out about long term migration or developmental patterns in movement, these sorts of things. The final
benefit that biologists also like to talk about is that you get reduced bias. And there definitely is an element from these technologies of reducing human bias. I mentioned before that behavior, biologists tend to tended to work with ether grams. And even though these are you do have inter inter coder compatibility tests before you go out and use them, to a large extent, they're still biased in the sense that it's humans working with them. So it's humans trying to identify
behavior and humans notoriously limited in terms of what they can see and what they pick out as a behavior to code. But also, the the code itself is biased in the sense that it's the, it's humans who have designed this code and picked up these behaviors. And as interesting and not added other behaviors that they don't find interesting or functionally relevant, even though they might be in the end. Quite interesting, quite important for this particular species, or this particular individual. tracking devices, then overcome these sorts of
sources of bias, because you're just getting whatever data that's being transmitted from this individual moving around in the world, you're getting, you're not having a human, they're deciding which data points are the most relevant. These are sort of the benefits that biologists themselves talk about, you hear them talking about them pin talks, at least I've heard them kind of getting really excited about all this new technology, they've gotten all the huge sample sizes and all this stuff that they can they can get with this technology. As philosophers, or scholars of science, we can kind of translate these direct benefits into benefits in terms of scientific values, or virtues. So scientists or behavioral biologists with these tools, they're getting greater significance and greater representativeness in the samples. They're also potentially going to be able to get more novelty in objectivity. And it's these two at the bottom that I'm more interested in. Is it really true that these
devices help behavioral biologists to get more novel results in the sense of results that aren't pre determined by theoretical assumptions or human biases? And then as a correlate of that, are these technologies more objective? Or do they give us a more objective science? To answer those questions, we have to look a bit closer at how what movement data looks like and how biologists deal with it. I mentioned at the beginning that the sorts of data that these kinds of trackers and transmitters are producing its position or acceleration data point coupled with a timestamp. So certain time intervals, this device sends information about either where the animal is or how fast it's accelerating, depending on what device it is plus the time. The way the data sets look is something like
this. This is data provided to me by Roland Lang rock is a statistician at Bielefeld University in the group that I work with. And he deals with animal movement behavior. He's a statistician, but he deals with junk primarily just with this data. The first example is of a shot, and it's being tracked with an accelerometer. So that tracks how fast it's accelerating. on the x axis is time like several many I think
it's weeks. And on the y axis, that's its acceleration, then you can see that it's quite complex. There's very low acceleration, there's very high acceleration and there seems to be some sort of fluctuation. The generally just it looks kind of messy, to me at least. The second example is an elephant track. And this is a
location track, I think it was a GPS track. And you can see, so this would be like a 2d projection onto a map. So you can put a map underneath it. Now you can see where the elephant
is going in that territory. The things that you can identify kind of as a naive person from this is that there's parts where it's kind of the elephant is just moving around, and a little squiggle there. And then there's parts where it's taking long, straight lines, or kind of is traveling further distances.
That's at least what it looks like. As well as the movement that you've often got other sorts of data, environmental climate data, as well as often information on the track individuals like the age, sex, condition, phenotype, and sometimes if you've taken a blood sample, you've got that genotype as well. What do you do with this kind of messy data, you look for patterns. And because it's so complex, this
sort of data, you often then go and ask statisticians to help. One of the most common ways to analyze movement data is using hidden Markov models. There are other ways but this is quite a dominant way to analyze this data. And it's also the way that this data that I showed in the previous slide was analyzed. So I'll look at this. Take the example of the shark. This is
the same data now the colors as shown. And you can also see the model that's being produced from the data, I won't go into too much too much detail, because it does get quite technical. But what we can see is that the model has split up the data into three states, there's a blue state, which is low acceleration, the yellow state, which is medium acceleration, and then a high green state, which is high acceleration. So the model has kind of decided that this is the best way to represent this data based on these that those three states the shark can be in. And then what you can do is you can look at the likelihood that that shark will be in one of these states, depending on the time of day. So seeing if there's a circadian rhythm in, in the sharks movement. And then you
can see that it looks like there is a bit of a rhythm, this high energetic movement seems to only occur in the early morning and in the evening. Whereas the, the medium acceleration kind of also seems to happen most often. And you can sort of see that from the data as well. Now, what's interesting about this sort of model is that it delivers these three states, and you can see on the graph that they're cold, cruising, active swimming and high energetic movement. But what what it's actually delivering is low acceleration, medium acceleration and high acceleration states. So it's up to the researchers to decide what these states mean. And here
they've decided it's cruising, active swimming, and some sort of high energetic movement is yet to be decided what exactly that is. So this is one of the major challenges with dealing with these dealing with this data and dealing with these sorts of models is interpreting what the models are telling you. What are the states is high energetic movement, kind of foraging, or like hunting is perhaps meeting or traveling.
This is not clear to a statistician dealing with the data. For that you need a biologist who knows what sharks do. I'm not a biologist, I don't know what sharks do. But I imagine it's something like hunting, I think in this case. But what's interesting is that you need this kind of biological background of knowledge of what's typical for that, for shark behavior. You see this also, in the case of the elephants, where they're similarly three states. One is a low movement state, which they've they've labeled resting.
There's a medium movement state, which is they've called area restricted search. So that's kind of moving but not not. So it's not doing more than resting, but it's not traveling and traveling is the sad state. This is a classic way to label these three states for terrestrial animals, actually, it's up to biologists then to say, Well, you know, in this area restricted search state, for an elephant. What does that mean? Maybe it means it's kind of going through a farmer's field and destroying all the crops and picking out the column that it likes. What's then interesting in particular about this model is that demonstrates the second challenge for dealing with movement data. And that's selecting variables. a
statistician probably wouldn't choose to plot these kinds of state probabilities against temperature. But the biologists were interested in how these increasing temperatures affect elephant herds. And what they found was that high temperatures, quite surprisingly, elephants were more likely to travel to engage in these kinds of long distance walks. And what the biologists then inferred is that they're looking for water. And probably the biologists were out there, also looking for water on a hot day, and observing how water got scarcer as the temperatures increased. What I think these
sorts of examples show us is that analyzing movement data, it's more than statistics, you can get a, you can download the data of the internet, you can run Hmm. But you're gonna have to have some sort of background behavioral knowledge about the species or about a similar like a closely related species, in order to interpret what that model is telling you. As well as to then kind of investigate it in more detail in terms of causal explanations or the function of the behavior. You also often need theoretical assumptions. And that wasn't
super clear in the examples that I showed. But you can see it in some other examples where there's an assumption that what the animals are doing there is going to be adaptive. Maybe you've got optimal foraging theory in mind. And that shapes them the way that you interpret the data that you're seeing, and the way you explain it, as well. And both of these mean that we
can think better this, these these claims about the revolutionary nature of these technologies, it's not quite clear that we are going to be getting entirely novel results if we're still relying on theoretical assumptions to interpret these data. And it's also we're not entirely departing from some of the biases that we already had, because we're starting, we're feeding in behavioral observations, our own behavioral observations back into our interpretation of the models, then this then feeds our own biases back in. So what do we see is the important things that they're doing that it's foraging, it's meeting, things like this? Who knows? Maybe they're doing something completely different that we haven't noticed? What can Icarus learn from what I've just been talking about? I think that it's pretty clear that radio transmitters can't tell scientists much on their own, the data that it delivers, must be interpreted and that interpretation that requires not just researchers at their desks doing stats, but also researchers in the field, and in the lab, looking at animals dealing with animals in their day to day life. In both of these, and in theory, of course, as well as the consequences, then it's not entirely likely that we're going to get completely new insights that overturn theory or overturn the kind of initial beliefs that we had about what animals were doing, of course, you do get you do get some new insights, especially into the different sorts of variation that there are, but it's, you have to be cautious about these sorts of claims, I think. So, apart from the irony that this project is called Icarus, I think there's something else we can take away from this, this philosophers. And that's that
these sorts of claims about big data, I mean, that we've investigated them in lab context, a lot of the time things, or human biology or things like immunology, or large scale projects, in epigenetics, so what's less, been done less is looking at big data in field sciences, like tracking animal movement. And it's interesting that he, you see the same sorts of things coming up, where you need this practical, maybe embedded knowledge of the organisms that you're dealing with in order to interpret the data and use the data for anything. And with that, I would like to finish up. This is the research team that I've been working with the NCQA group. And that's where I got a lot of the insights from the talk today. So I'd like to thank them and also obviously the DFT for funding me and to you all for listening. Very cool example. Thanks so much. Let me let me do my usual
and ask ask a question once Now, while the while we wait for everybody to catch up with the end of the with the end of the broadcast. So I wonder. I wanted to ask about, you mentioned that I mean, obviously you're you're you You know, some of these biologists, well, you're engaging with them, you're engaging with them regularly. So I wonder what what's their attitude toward these theoretical presuppositions, I guess toward their relationship towards theory, I guess is a very is probably the best brief way to put it, because this is a classic thing that we sometimes hear about that there's a kind of distrust, that perhaps the reason that they're presenting their work this way is that they want to show themselves is not being engaged in the field, we're just reading off the data, right, not being engaged in a theory laden enterprise. So I wonder what that's been like in your, in your discussions with them? Yeah, that's a good question. I haven't like explicitly gone
into these questions, say about adaptation ism with them. That's kind of future work that I'll be doing, hopefully. But I am fortunate to be in a team where there is quite a lot of interest in theory, and in philosophy, actually. So they're quite, quite happy to engage with me about discussion in discussions about kind of more, much more theoretical pie in the sky sort of topics. So my thesis is on individuality. And now we had a
lot of discussions about these sorts of theoretical ideas to do with individuality. And, you know, I would ask critical questions, and sometimes they do get a bit uncomfortable with it, you know, but there was less of this sort of, and potentially, so that I am in a German context, there was less, you know, hesitancy to to rely on theory? And do you think it does have something to do with the German context? Actually? That's an interesting, yeah, yeah. The the impact of of, of broader scientific cultures here is that that's it. That's a cool, that's a cool angle that I that I hadn't thought about it. Of course, it's relevant for a project like Icarus, that's completely obviously, I assume totally International. Right.
Yeah. Although it is hosted by the Max Planck Institute for theology in Germany. So, but the, like, the main research are there, he was working in the US for a long time as well. So, yeah, sure, wide, wide network. Um, let me uh, let me pick up on another element here. So So I tried to think about how to how
to phrase this as well. So So I wanted to pick So you mentioned that there's all this data is publicly available. And so I wonder, is there a significant environment of reuse around this data? What have you been able to pick up on that? And I'm picking up on I'm sort of, I'm sort of riffing on sabinas. Talk from
from earlier? Is there a, is there a community built around the utilization of this of this kind of data? Can you can you tell from from working with the biologists that you've talked to? And that's, that's another good question. I haven't seen that with the biologists I've been working like they're much more engaged with producing the data. And I think also, because of some of the stuff I talked about in the talk, reuse is going to be quite challenging for a lot of these researchers, they're producing not not only because you need this kind of background knowledge of what the species normally does, because, you know, because there's such variety, it's, you have to know something about these these species. And often you don't as a behavior biologist, you know about some sort of species, but not about these other things. But apart from that, there's also the issue that a lot of this data is being generated in very specific kinds of situations. That said, I think
that there must be people were using this data or they wouldn't bother to put it up. And I especially I think, with the the migration data, that's where things start to get interesting, because people look at them in terms of the spreading of diseases, or kind of changing land use with changing agricultural patterns, these sorts of things. So yeah, it's it's something actually that I should look into in future researchers like how this how this data is traveling into other contexts. of question coming in from from Sarah Davies, who asks, he says, What a fantastic case, I really liked the idea of looking at data creation and management in different kinds of spaces. So also also reminded of the work on metadata management that we heard about the other day. So how did your informants deal
with this? How much data cleaning data care data curation, do these data need? And also, where's this done? Is this how does that does that kind of work happen out in the field? Or is that is that happening back in the lab? Yeah, that's good. Good question. Thanks. I don't know too much about how much like the extent to which they have to clean this data. I imagine there's a fair bit of cleaning that goes on although it is often quite simple data, location, plus timestamp. It will usually have in a lab, so when you go out into the field, often you don't have a good internet connection. So you're not actually receiving the data
as it's being produced unless you kind of go attach the transmitters, then go straight back to your lab and watch what happens. So these people in Antarctica, they spend six months down there. And then only when they get back to they find they find get all the results that they had, you know, been accumulating, and start to look into that data. So yeah, but
obviously depends on your on your study system as well, where you are and what you're doing. Serious delayed gratification for the for us for Antarctic work. That's impressive. Let's see on a bill of rights, I like what you say about theory and forms to the statistics and the models that you use. Can you elaborate on your experience
working with a statistician? So is there a back and forth process between the questions that you want to ask the data you have available, the statistics that are available to be used? So I haven't really been working with Roland, and in to the extent I've had conversations with Roland, and he has, so this is kind of actually where I got the idea for most of the stuff of this talk. He's told me a lot about how like he, as a statistician, has no idea about biology. He really like he had his no training, he was asking me at the start of the research group, what's the phenotype? Like? Can you explain to me What's a phenotype? I don't understand? So what's what's been interesting is looking at these challenges and communication between statisticians and biologists.
And this this difficulty, because obviously, biologists then they don't know what Hmm, is they have to kind of, like, figure out what it is that this model is doing. And what are these states? And what is that telling me? So you see it in really interesting, like learning process between these different communities? And obviously, I've learned a whole lot because I didn't know what Hmm, was either. Not Well, not really. So that's been I think that's one of the most interesting things about these interdisciplinary communications is the learning that takes place, and then also some of the challenges that they have to deal with in the process. Just to follow up on that this is this is for my for my edification. So
the group that you're working with it, it is it's all of these people mixed together in a in a big research cluster. That's really cool. Yeah. That's very cool. Okay. Next question coming in, from Christoph mountaire, who writes a fascinating area of research. So you mentioned two limitations concerning interpreting the data, and selecting the variables. Because I'm wondering also about about the role of choice of model types. So for example, hmm. Versus versus other kinds of models, or choice of params, like hyper parameters for fitting the models? So you talked at all about your thoughts on that for this particular case? study? Or, or more generally? Yeah, that's a good question. I don't know too much about what
sorts of four processes go into choosing the models, I have a feeling it's like, at least in the group that I'm dealing with. The statistician is quite familiar with dealing with Hmm, and it is one of the most common ways to deal with movement, movement data, because it's a way of kind of modeling steps. And that's often what you've got is you've got step data. But obviously, they can do things like linear regression. And but
biologists are more familiar with doing linear regression. So maybe they'll try that out first. And if it's proving too difficult, they'll go to the statistician and the statistician will say, here's this tool that I've got, let's try it out. So I have a feeling it's much more in terms of tools. And once the tools are available, then in terms of
like, what would be the best for this data set in terms of like, very considered processes? I mean, people have talked about this, this kind of tool based use of stats in other cases as well. So yes, you say that that that fits with a sort of toolbox conception of biological modeling that you hear about fairly frequently in our literature's at least Yeah, yeah, for sure. Let me see. with that. I think we're, we're running out of questions and I don't have anything else to add. So let me just let me wait for again, just to give people some a little bit more chance to type in type in something into the box if you're interested. We are almost out of time. So that's
that's not a problem. But if anybody has anything they want to add, but I think Well, I think we can go ahead and wrap it up there then thank you so much. Really, really cool example. I'm interested to see where where the where the work keeps going and I I'm going to go I'm going to go look at all the all the fun movement data on Long soon, too. That's really cool. Thanks. Fantastic.
Thanks so much. And we'll see everybody back here in a few minutes. It's one of our slightly longer breaks. So we'll see you in a few minutes for for the next talk. Thanks so much. See you. Whoo hoo hoo hoo things
2021-03-25 15:52