Transforming Healthcare with AI: The New Era of Medical Technology
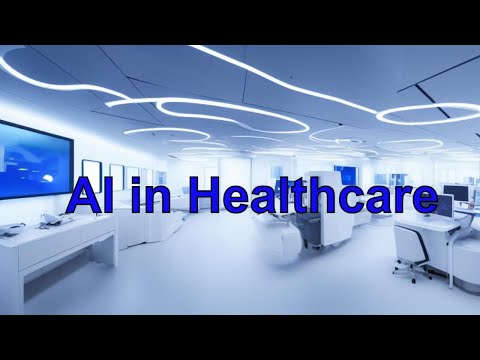
Artificial intelligence (AI) is revolutionizing the healthcare industry by providing powerful tools for diagnosing diseases, analyzing medical data, and improving patient outcomes. With the ability to process vast amounts of data quickly and accurately, AI has the potential to transform the way we approach healthcare, from personalized medicine to population health management. By leveraging AI, healthcare providers can make more informed decisions, improve patient safety, and ultimately save lives.
As AI technology continues to advance, it is clear that it will play an increasingly important role in the future of healthcare. WHAT IS AI AI (Artificial Intelligence) and Machine Learning are closely related fields that are revolutionizing the healthcare industry. While they share some similarities, there are also key differences between them. Machine learning is a subset of AI that focuses on the development of algorithms and statistical models that enable machines to learn from data without being explicitly programmed. In healthcare, machine learning can be used to identify patterns in medical images or data sets, to make predictions about patient outcomes, or to assist with diagnostic decision-making.
AI, on the other hand, refers to the broader field of developing intelligent systems that can perform tasks that would typically require human-level intelligence. In healthcare, AI can include not only machine learning, but also natural language processing, robotics, and expert systems. One of the primary benefits of machine learning in healthcare is its ability to analyze large amounts of data quickly and accurately.
This can help healthcare providers to identify trends and patterns that might not be apparent to human analysts, and to make more informed decisions about patient care. AI, on the other hand, has the potential to revolutionize healthcare by automating routine tasks, providing personalized care to patients, and improving the accuracy of diagnoses. For example, AI-powered virtual assistants can help patients to manage their health by answering questions, providing reminders, and offering personalized recommendations based on the patient's health data. Overall, both AI and machine learning have enormous potential to improve the quality of healthcare by making it more efficient, accurate, and personalized.
However, it's important to note that there are also challenges associated with these technologies, including concerns about privacy and data security, as well as ethical issues related to the use of AI and machine learning in healthcare decision-making. HISTORY OF AI AND MACHINE LEARNING IN HEALTHCARE The history of artificial intelligence (AI) and machine learning in healthcare can be traced back to the early days of computing. In the 1960s and 1970s, researchers began to explore the potential of computers to analyze medical data and support clinical decision-making.
However, it was not until the 1980s and 1990s that these technologies began to be used in earnest in healthcare. In the early days of AI and machine learning in healthcare, researchers focused on developing expert systems. Expert systems are computer programs that use a knowledge base and inference rules to make decisions or solve problems that would normally require human expertise. These systems were used in healthcare to support diagnosis and treatment planning but were limited by the fact that they relied on a static set of rules and did not learn from new data.
In the 1990s, machine learning began to emerge as a more promising approach to AI in healthcare. Machine learning algorithms are designed to learn from data, enabling them to identify patterns and make predictions based on new information. This approach was particularly well-suited to healthcare, where large amounts of data are generated every day. One of the earliest applications of machine learning in healthcare was in medical imaging.
In the early 1990s, researchers began to develop algorithms that could analyze medical images to detect abnormalities and diagnose diseases. One of the first successful applications of these algorithms was in the field of mammography, where machine learning algorithms were developed to analyze breast X-rays and detect early signs of breast cancer. Another early application of machine learning in healthcare was in the field of natural language processing (NLP). NLP is the process of teaching computers to understand and interpret human language. In healthcare, NLP was used to analyze clinical notes and other free-text data, enabling healthcare providers to extract valuable information from unstructured data sources. In the early 2000s, machine learning began to be used more widely in healthcare.
Researchers began to develop algorithms that could analyze large data sets and identify patterns that would be difficult or impossible for humans to detect. These algorithms were used in a wide range of applications, including predicting patient outcomes, identifying patients at risk of developing certain diseases and supporting clinical decision-making. One of the most significant applications of machine learning in healthcare is in the field of precision medicine. Precision medicine is an approach to healthcare that uses genetic and other molecular data to tailor treatments to individual patients. Machine learning algorithms are used to analyze patient data and identify patterns that can be used to predict which treatments are likely to be effective for a given patient. Machine learning is also being used in healthcare to improve the accuracy of diagnoses.
For example, machine learning algorithms can analyze medical images and other data to identify patterns that might not be apparent to human doctors. This can help healthcare providers to make more accurate diagnoses and improve patient outcomes. More recently, deep learning has emerged as a powerful tool for AI in healthcare.
Deep learning is a subset of machine learning that uses neural networks – algorithms that are modeled on the structure of the human brain – to analyze data. Deep learning algorithms are particularly well-suited to healthcare because they can analyze complex data sets and identify subtle patterns that might be missed by other algorithms. One of the most exciting applications of deep learning in healthcare is in the field of medical imaging. Deep learning algorithms are being used to analyze medical images and identify patterns that can be used to diagnose diseases such as cancer, heart disease, and neurological disorders.
Deep learning is also being used in healthcare to support drug discovery and development, by identifying new drug targets and predicting the effectiveness of new drugs. Despite the many successes of AI and machine learning in healthcare, there are also challenges and limitations associated with these technologies. One of the biggest challenges is the need for large, high-quality data sets to train machine learning algorithms.
MACHINE LEARNING Machine learning and artificial intelligence are changing the healthcare industry in profound ways. By enabling healthcare providers to process and analyze large amounts of data quickly and accurately, these technologies are helping to improve patient outcomes, reduce costs, and increase efficiency across the entire healthcare system. In this part, we will discuss the various ways in which machine learning and AI are being used in healthcare, as well as some of the challenges associated with these technologies. Machine learning is a subset of artificial intelligence that involves the development of algorithms and statistical models that enable machines to learn from data without being explicitly programmed. In healthcare, machine learning is being used to analyze medical images, identify patterns in patient data sets, and make predictions about medical outcomes with greater accuracy than ever before.
One of the most significant applications of machine learning in healthcare is precision medicine. By analyzing patient data, machine learning algorithms can predict which treatments are likely to be successful for individual patients based on their unique genetic makeup and medical history. This enables healthcare providers to tailor treatments to individual patients, improving outcomes and reducing costs.
Machine learning is also being used in medical imaging, enabling healthcare providers to identify patterns and abnormalities that might not be apparent to the human eye. By analyzing large amounts of patient data, machine learning algorithms can identify patterns that might indicate the likelihood of certain medical outcomes. For example, machine learning algorithms can predict which patients are at risk of developing certain diseases, allowing healthcare providers to take preventative measures to reduce the risk of illness. AI is a broader field that includes machine learning as well as natural language processing, robotics, and expert systems.
In healthcare, AI is being used to automate routine tasks, provide personalized care to patients, and improve the accuracy of diagnoses. One example of AI in healthcare is virtual assistants, which can help patients to manage their health by answering questions, providing reminders, and offering personalized recommendations based on the patient's health data. Virtual assistants can also help healthcare providers to automate routine tasks such as scheduling appointments and ordering tests, freeing up time for more important tasks. AI is also being used in healthcare to improve the accuracy of diagnoses.
For example, AI-powered diagnostic tools can analyze medical images and other data to identify patterns that might not be apparent to human doctors. This can help healthcare providers to make more accurate diagnoses and improve patient outcomes. One of the biggest challenges associated with AI and machine learning in healthcare is data privacy and security. Healthcare data is highly sensitive and must be protected from unauthorized access or misuse. As AI and machine learning technologies become more sophisticated, it is essential that healthcare providers take steps to protect patient data and ensure that it is used ethically and responsibly. Another challenge associated with AI and machine learning in healthcare is the potential for bias.
Machine learning algorithms are only as good as the data they are trained on, and if that data is biased or incomplete, the algorithm may produce biased results. Healthcare providers must take steps to ensure that their data is representative of the patient population they serve, and that algorithms are tested and validated to ensure that they are producing accurate and unbiased results. RULE-BASED EXPERT SYSTEMS Rule-based expert systems are a type of artificial intelligence technology that has been used in healthcare for many years. These systems use a set of rules and knowledge-based reasoning to make decisions about a patient’s diagnosis and treatment plan. In healthcare, rule-based expert systems are used to support medical decision-making and improve patient outcomes.
The development of rule-based expert systems in healthcare began in the 1970s and 1980s. At that time, the systems were primarily used to diagnose and treat infectious diseases. As the technology evolved, rule-based expert systems were used for a variety of medical applications, including the diagnosis and treatment of cancer, heart disease, and other chronic illnesses. The rule-based expert systems in healthcare consist of a knowledge base, inference engine, and user interface. The knowledge base contains all the relevant medical knowledge and data that the system uses to make decisions.
The inference engine is the component that applies the rules and reasoning to the data and generates a diagnosis or treatment plan. The user interface is part of the system that allows the healthcare provider to input the patient’s data and interact with the system. NLP IN HEALTHCARE. Natural Language Processing (NLP) is a subfield of artificial intelligence (AI) that focuses on teaching computers to understand and interpret human language. NLP has a wide range of applications, from chatbots and virtual assistants to text mining and sentiment analysis. One of the most promising applications of NLP is in healthcare, where it is being used to extract valuable information from unstructured data sources such as clinical notes, electronic health records, and medical literature.
One of the key challenges of NLP in healthcare is the complexity and variability of medical language. Medical language is highly specialized and includes many technical terms, acronyms, and abbreviations. Furthermore, medical language is highly context-dependent, meaning that the same term can have different meanings depending on the context in which it is used.
For example, the term "heart attack" can refer to a myocardial infarction, a cardiac arrest, or a heart failure, depending on the context. To overcome these challenges, researchers are developing NLP algorithms that are specifically tailored to the unique characteristics of medical language. These algorithms use a combination of rule-based and machine-learning approaches to identify and extract relevant information from medical texts.
One of the most common applications of NLP in healthcare is in the analysis of clinical notes. Clinical notes are the primary means by which healthcare providers record information about patient visits, including symptoms, diagnoses, and treatments. However, clinical notes are often unstructured and contain a large amount of irrelevant information, making it difficult for healthcare providers to extract valuable insights. NLP algorithms can be used to analyze clinical notes and extract relevant information such as diagnoses, medications, and procedures. For example, an NLP algorithm might be used to identify all IMAGE PROCESSING IN HEALTHCARE Image processing is a subfield of computer science and electrical engineering that focuses on developing algorithms and techniques for manipulating and analyzing images.
In healthcare, image processing has become an integral part of medical diagnosis and treatment. By analyzing medical images such as X-rays, CT scans, MRI scans, and ultrasound images, doctors can make more accurate diagnoses and develop better treatment plans for their patients. One of the primary applications of image processing in healthcare is in medical imaging. Medical imaging involves capturing images of the internal structures of the human body using specialized imaging techniques. These images can be used to diagnose and monitor a wide range of medical conditions, including cancer, heart disease, and neurological disorders. Image processing algorithms can be used to enhance and analyze medical images, making it easier for doctors to identify and diagnose medical conditions.
For example, image processing algorithms can be used to remove noise and artifacts from medical images, improve contrast, and sharpen edges, making it easier for doctors to see and interpret the images. Image processing algorithms can also be used to segment and classify the different structures and tissues in the image, making it easier for doctors to identify abnormalities and diagnose medical conditions. Another important application of image processing in healthcare is telemedicine.
Telemedicine involves the use of technology to provide medical care and consultations over long distances. By transmitting medical images over the internet, doctors can provide remote consultations and diagnoses, allowing patients in remote or underserved areas to receive medical care that would otherwise be unavailable. Image processing algorithms can be used to compress and transmit medical images over low-bandwidth networks, making it possible to transmit images over the Internet quickly and efficiently. This has enabled telemedicine to become an important tool for providing medical care to patients in remote or underserved areas, where access to medical specialists may be limited. TODAY’S AI IN HEALTHCARE AI in Radiology.
AI has revolutionized the field of radiology, providing fast and accurate analysis of medical images such as X-rays, CT scans, and MRI scans. AI algorithms can detect and identify abnormalities in medical images, such as tumors or fractures, that might be missed by human radiologists. This technology can help reduce the time and cost of medical imaging, improve the accuracy of diagnoses, and increase the efficiency of healthcare providers.
AI IN Emergency Department. AI is used in emergency departments to improve patient outcomes and reduce the time required for medical diagnosis and treatment. AI algorithms can analyze patient data such as vital signs, medical history, and lab results to identify patients who require immediate attention.
This helps healthcare providers prioritize patients based on their needs, allowing them to provide more timely and effective care. AI in Drug Discovery. AI is used in drug discovery to develop new and more effective treatments for a wide range of medical conditions. By analyzing large amounts of data, including genetic information, medical records, and scientific research, AI algorithms can identify potential drug targets and develop new drugs that are more effective and have fewer side effects.
AI in Patient Risk Identification. AI is used to identify patients who are at risk of developing certain medical conditions, such as diabetes or heart disease. By analyzing patient data, such as medical history, lifestyle factors, and genetic information, AI algorithms can identify patients who are at higher risk of developing these conditions. This allows healthcare providers to develop preventive measures and interventions to reduce the risk of these conditions. AI in Primary Care/Triage. AI is used in primary care and triage to provide quick and accurate medical diagnoses and treatment recommendations.
AI algorithms can analyze patient data such as symptoms, medical history, and lab results to provide healthcare providers with a diagnosis and treatment plan. This helps healthcare providers provide more timely and effective care to their patients. AI in Oncology. AI is used in oncology to improve cancer diagnosis and treatment. AI algorithms can analyze medical images such as X-rays, CT scans, and MRI scans to detect tumors and identify the best treatment options for individual patients.
AI is also used to develop personalized treatment plans based on a patient’s genetic profile and medical history, which can help improve treatment outcomes and reduce the risk of side effects. AI algorithms can help improve the accuracy and efficiency of medical diagnoses and treatments, reduce the time and cost of medical imaging, and identify patients who are at risk of developing certain medical conditions. As technology continues to evolve, it has the potential to revolutionize the way healthcare is delivered, making it more personalized, accessible, and effective for patients around the world. CHALLENGES OF AI IN HEALTHCARE The integration of AI technology into healthcare has the potential to revolutionize the industry, but there are significant challenges that must be overcome for AI solutions to be successful.
One of the biggest challenges is the need for vast amounts of patient data to train and optimize algorithms. However, accessing personal medical records is strictly protected, and data sharing between hospitals and AI companies has generated controversy, raising ethical questions about data ownership and patient privacy. Who owns and controls the patient data needed to develop new AI solutions? Should hospitals be allowed to provide or sell vast quantities of their patient data, even if de-identified, to third-party AI companies? How can patients' rights to privacy be protected, and what are the consequences of a security breach? Furthermore, new regulations such as the General Data Protection Regulation (GDPR) in Europe, which includes the right to have personal data deleted, add complexity and potential penalties. Another challenge is the quality and usability of healthcare data. Unlike in other industries, where vast amounts of data are generally reliable and accurately measured, healthcare data can be subjective and often inaccurate.
Clinician notes in electronic medical records are unstructured and can be difficult to interpret and process. Additionally, data inaccuracies can occur when patients provide misleading information. Data sources are often siloed across different healthcare providers, making it difficult to capture a full profile and range of determinants for a patient's health. Regulating cloud-based and constantly evolving AI technology poses additional challenges.
How can patients be protected, and how can regulatory oversight be provided for a solution that is constantly learning and evolving, rather than a distinct, version-controlled medical device? For AI solutions that involve direct patient interactions without clinician oversight, such as chat-based primary care tools, questions arise regarding whether the technology is a 'practitioner of medicine' rather than just a device. In this instance, will it need some form of medical license to operate, and would a national medical board agree to grant this license? Another significant challenge is determining liability should anything go wrong. If diagnosis or treatment is controlled by AI technology, does the AI company assume liability for the patient's well-being? Will insurance companies ever underwrite an AI tool? Finally, user adoption is a barrier to the utilization of AI technology in healthcare. Patients may be hesitant to trust a diagnosis from a software algorithm rather than a human doctor, and clinicians may be slow to embrace new solutions. In an industry that still widely uses the fax machine, it may be unrealistic to expect rapid adoption rates beyond proof-of-concept studies.
These challenges include data ownership and patient privacy, quality and usability of healthcare data, regulatory oversight, liability, and user adoption. It will require collaboration between healthcare providers, AI companies, and regulatory bodies to address these challenges and ensure the safe and effective integration of AI technology into healthcare. AI IN THE FUTURE OF HEALTHCARE AI IN HEALTHCARE in 5 years. In the next five years, we can expect AI to become even more integrated into the healthcare industry. AI will be used to improve patient outcomes, streamline administrative tasks, and reduce costs. We will see more AI-powered tools for personalized medicine, medical diagnosis, and drug development.
AI will also be used to automate medical image analysis, and we will see more virtual health assistants that use natural language processing (NLP) to communicate with patients. AI HEALTHCARE in 10 Years In the next ten years, AI will play an even larger role in healthcare. We can expect to see more AI-powered robots that can perform surgeries, assist in physical therapy, and provide companionship for elderly patients. AI will be used to predict and prevent diseases before they occur.
The healthcare industry will rely heavily on AI-powered predictive analytics to make more informed decisions about patient care. AI IN HEALTHCARE in 20 years. In the next twenty years, AI will have revolutionized the healthcare industry. AI-powered virtual doctors will be able to diagnose and treat patients remotely. Medical records will be completely digital, and AI will be used to analyze this data to improve patient outcomes. Nanobots powered by AI will be used to deliver drugs directly to cancer cells, and we will see more AI-powered prosthetics that can respond to the user's thoughts.
AI IN HEALTHCARE in 30 years. In the next thirty years, AI will have transformed healthcare beyond recognition. We can expect to see AI-powered implants that monitor our health in real-time and can deliver treatments automatically. We will also see AI-powered exoskeletons that allow people with disabilities to walk again. AI will be used to predict and prevent pandemics, and we will see more personalized medicine that is tailored to an individual's genetics and lifestyle.
AI IN HEALTHCARE in 40 years. In the next forty years, AI will have become an integral part of our daily lives, and healthcare will be no exception. We can expect to see AI-powered medical devices that can detect and diagnose diseases before symptoms occur.
We will also see AI-powered healthcare robots that can provide 24/7 care for patients in their own homes. AI will be used to create customized diets based on an individual's health data, and we will see more AI-powered mental health treatments. AI IN HEALTHCARE in 50 years. In the next fifty years, AI will have transformed healthcare to the point where we no longer must worry about diseases that once plagued humanity.
We can expect to see AI-powered gene editing that can eliminate genetic diseases from our DNA. We will also see AI-powered organ replacement that eliminates the need for donors. AI-powered nanobots will be able to repair damaged tissues, and we will see more AI-powered brain-machine interfaces that allow people to control machines with their thoughts.
AI IN HEALTHCARE in 100 years. In the next one hundred years, AI will have enabled us to achieve the goal of healthcare. immortality. We can expect to see AI-powered implants that allow us to live forever, free from disease and aging. AI will be able to create synthetic organs that are better than our natural ones, and we will see more AI-powered exoskeletons that enhance our physical capabilities. AI will also allow us to communicate with other beings, including animals and possibly even extraterrestrial life forms, about their health and well-being.
2023-05-06 02:43