TRACO 2017: Functional Genomics and HIV
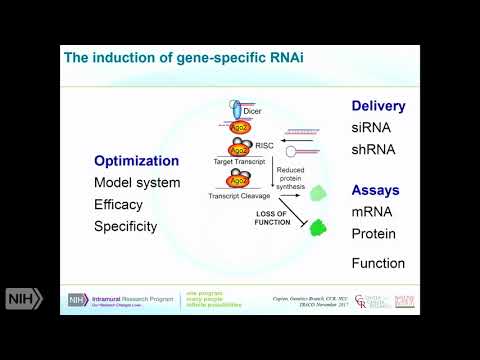
Our first speaker today is Natasha Kaplan, from the genetics, branch she. Got her PhD from the University, of London King's College Hospital, Medical, School. And. Subsequently. She did postdoctoral training, at Saint Mary's Hospital Medical, School, Imperial, College. And. In, 1996. She joined, the National Human Genome Research Institute. At NIH as. A visiting, fellow she. Started working on si. RNA. And. She's. Now a. Investigator. In the Center for cancer research and, she's going to talk to us today about functional. Genomics, Natasha. All. Right good. Evening is I'm. Okay I know I'm okay in here am i okay. Across. The land no they're not not, telling me I'm not so I'm assuming oh okay all right okay. So thank you very much Terry thank you for the introduction so, what. I'm gonna do today is, give. You a sort of an overview, of, my, take on the, functional, genomics field. As a whole I'm, gonna do a relatively. Brief introduction. About some terminology and, some key. Concepts. So I just want to get in place then, I'm going to spend a little bit of time on the technology, but I really want to spend most of the time today, talking, about applications. And predominantly, using work from my lab to, give you some concepts. About how we can, use different. Functional genomics, technologies. I'm going, to really focus predominantly, on RNA I based, technologies, but really a lot of the. Approaches. Are going to be the same irrespective, of, how, you actually. Manipulate. Gene expression. Okay. So. Why. Do we need functional, genomics, so we. Know that the, technological, advances, that we can now use to assess, things like DNA copy number in sequence and I'm really here thinking about in the context of cancer that. We could look at DNA in chromatin, modifications. And we can look at gene expression on. A very very large scale and a very great deal of depth and that, has revolutionized how. We understand. The molecular disequilibrium. That is underlying, cancer and obviously many other diseases but. Our bill, to functionalize. These findings, in a very systematic manner. It still, remains a challenge and so for the last you, know 10 plus years now there's been a lot of emphasis on trying to develop functional, genomic strategies, that we can conduct in a similar, scale and then drill. Down into what these types of changes, that we're seeing either in DNA, copy numbers sequence looking at chromatin modifications, or gene expression what this actually means in the, context, of looking at normal, function, and then obviously in, the, function of genes in a disease setting, so. The. Moral systems, that you can conduct functional, genetics are obviously, as broad as all of the different model organisms, that we have available to us, so. On. A gene. Specific level. You can do a lot or even on a large scale you can do a lot of these experimentation. In bacteria, yeast C elegans zebrafish, the software and a mouse in, the context, of human most. Of the work I'm going to talk about today has been done in transformed, cancer, cell lines and I'm, going to give you a hint, of some of the advantages, and disadvantages of that as we go through. Increasingly. Though particularly. Some of the new technologies, you're seeing it being able to be applied to, primary, cells into, patient-derived, xenograft into. PD X's and then induced pluripotent stem. Cells, as I say what I'm going to talk about predominantly, in cell lines but you can it, can be extrapolated across, okay. So. There, are really sort of two flavors, of functional, genetic analysis that I'm going to talk about you I'm going to predominantly, talk about the second of these but I just wanted to mention this first which is the gain-of-function, says, when you're over, expressing or you're, sort of replacing. A gene to see, what its effect is in. A different context so you can over, express, a wild-type, gene. You. Want to make sure I'm getting them yeah. You. Can over express, a wild-type, protein and mutant background, to, see what the functional, effects are of that you, can use over expression. In epithet. Test, where you can over, express different genes and look at how that effects a. Funky. Q, latino type where. There's actually probably been, most workers in the ovary. Rushon of a gene that, usually this open reading frame the cDNA, in a hat drawer like a system either whole organism, or in the cell lines that I described, earlier. And. These can be used to look at, wild-type. Proteins, or mutant proteins they can be used to create interaction. Networks and they can be used as modifiers.
For. Screens, or. Looking. At drug targets, so. There's really a very large, way, and different ways you can use these types of over expression studies but, there has been a tendency, to, actually not do this on a large scale, this, is hard to manipulate and. It's. Been hard to read the sit this the. Phenotypes. From these so there's been most emphasis, in the functional genomics, field really on a loss-of-function whether, it tends to be a clearer, of phenotype. At the end of it so there, are two types, of loss. Of function in the crudest, type way. That i'm gonna look at you, can have a knockdown. Or. You. Can have a knock out okay. So. I'm gonna talk about both of these but they are different okay, so, when you knock down you've often still, got a residual. Level of protein, expression or suddenly an mRNA, expression there, and that can you, really need to think about that in the context of the phenotypes, that you're investigating, a, knockout. In theory, should take out all of the protein, or all. Of the RNA, molecules, that you're targeting so, you have, a difference, that needs to be boring in there so. The other piece. Of of. This puzzle that I just want to put forward is the difference between for. Genetics. And reverse genetics and, then. I'm going to pull all of these together to discuss the different technologies, that are available to, us so. Forward. Genetics is the mapping, of a phenotype, to emission mutation. With no prior, genetic, information and for a long time this was done in the context, of mutagenesis, studies, using chemical. Radiation. Or or, viral, mutagens, the, other is to do reverse genetics was you using prior to netting information, to generate the read reagents. They induce a phenotype, so, for a long time in particularly mammalian, systems, we. Needed to do for genetics, because we didn't have all of the information we, needed to, do reverse genetics which for a long time was really the purview, of things like yeast or. Drosophila. Models but obviously with the. Identification. Cing, of the human, genome and then with the tools that i'm going to talk about today that we've developed increasingly. We're now able to do reverse, genetic, studies. So. I'm. Now going to sort of move on to the technologies. In the context, now just, of loss of function okay. So. There are really calm in two, types. Those. Whether you're focusing, on gene silencing which, you know is often. As I say is referred to in the literature is sort of a knockdown and is. Predominantly. Using the RNA interference, mechanism. The. Alternative. Which we've now seen increasingly. Now become. Applicable. Is to do gene editing where, actually, this can be both either a knockdown or a knockout depending. On the effector molecules, that you're using and these come in two predominant, flavors, in terms of technologies, that have now developed over the last few years once, using transcription, activator, like effector nucleosis. Or talons, and then, what they want to obviously use clustered, regular, interspaced. Short palindromic, repeats or, CRISPR, and I'm going to go through these and a little bit of detail just to give you some some basics, now, there has been a plethora of different reviews, over, the last few years that have been looking, at these different technologies. I'm, going, to focus on one that was came out actually now a couple of years ago but Whitley is still. Relatively. How. I'm gonna say this it's, very relevant still, and. A lot of the issues haven't changed, that, I really want to you to focus on and the key thing here is and why they've titled, it was what's choosing, the right tool for the job and it's the contest is that actually, what is really great now is we have we have options we can try all of these different things and we can see which is going to work in the bet in the context of a particular experiment, so now walk through these. Three different main areas, I'm going to talk about are and I very briefly then. Go on to talon then. Go on to CRISPR, based techniques. So. I, I is, a post-transcriptional. A. Mechanism. That. Induces. RNA, degradation and, so this is a reversible. Knockdown. And the major ways, of. Inducing. The effect is to use a small, interfering, RNAs, so the RNA RNA, mechanism. Is a naturally, occurring gene, silencing mechanism, it's. Predominantly. Known in the context, of using a micro, RNAs that regulate. Post. Transcriptional, gene silencing. By. Interfering. Or. Interacting. With three, prime sequences. Of a. Transcript. And that alters. The expression, of a, of. A of the protein, from a particular transcript. But, we've been able to exploit. This but, using, sis or shrnas. That. Actually target, specific, transcripts, and induce. A degradation. Of, that transcript, now, one of the issues has been that there is quite a lot of target, space for this effectively. There are because of the way that microarrays work you can have an interaction, with an s RNA with, a lot of different sequences and so that has been one of the major.
Problems. With this I'll mention how this can be overcome in a few minutes, but, it's still a very robust, and it's very quick in comparison, with crispy you can do an S RNA experiment, in a couple of days we're, still doing CRISPR, experiments, it's going to take you a long time so you've sometimes. It'll a lot of the question, is simply you know what is the question I want to know and how quickly do I want to know it as to why it would make sense to use an RNA I based. Experience. So, just to cover what I just mentioned. In terms, of how this works so. Predominantly. We're. Using si RNAs or si Charlie so srna's, are synthetic, molecules, that can now be purchased from many different commercial, sources. Using. Fairly. Robust, algorithms. We in, typically. One will test at least three to four different are srna's, per gene to, be sure of successful. Knockdown. Shrnas. Are going to be expressed from a, plasmid. And they can be. Expressed also. From an expression, vector this. Is targeting. Luna said the RNA I pathway, I'm showing a highly, simplified, version. Of this where. I'm just focusing, on the. Signer plasmic, part of the pathway where, it's going to interact, with the RNA induced silencing, complex, are, predominantly. With the protein, I a go-to which. Will locate, will take one strand it will take the guide strand, from. The s RNA or from, the shrna that's been processed by Dicer it, will align that on a sequence, based and it will cleave in the middle of this this, will reduce protein, synthesized, as a consequence, of the fact that you have degradation. Of, the transcript, and therefore then a loss, of function so the assays, that can, conduct. You downstream, of this are fairly obviously, going to be mRNA, protein or, function, and what really has to be optimized, to apply this technique, this, technology, is making, sure you've chosen the correct model system, to be able to look at your particular question. Related to loss of function the efficacy of the, particular reagents. Here you are using and then as I mentioned specificity. Now. The talons. Are, sort. Of. In-between. Stage. If you like between RNA I and, and, and CRISPR, and what, they are using is, a way. Of inducing, a, frameshift DNA. Mutation, or as I'll talk about in a different way you can actually repress, transcription which, sort of becomes a little bit more like RNA I. The. When. You're inducing the DNA mutation, obviously this is a permanent knockout. Whereas. If you're using an effective molecule, that induces. A repression, of transcription this. Can be reversible. So. The required information for this is a transcriptome. Which, is the same as with RNA i/o it's key for talent, a tail and repression, is you actually need to know exactly where the, transcriptional, start site is for your gene and that's not always, very clearance, not always very amenable. So. The. Off target. Space for this is thought to be a little particularly. For gene editing is thought to be a little less because as you'll see you need to use you, need a lot of sea, once to define where you're going to edit and that should reduce the. Off-target space but. You can also in the context of regression, you can see that that may not be as. Specific as you would like. When. They talk I should, have stressed this here in Hindman echo so many. Genes, have, transcript. Variants, and that's one of the things you really have to consider when you're designing these experiments, that if you're going to be editing a gene or silencing, the gene are you going to want to take out all of the variants, one subset, of variants because these variants may have different. Functions. And so that might be come, into your thinking all right so they took a little bit more about tails. Or transcriptional, activator like effectors, that can be used for programmable. DNA binding, proteins, so these are talents, and they use synthetic, proteins, that are based on the protein screw secreted. By the exam for mice -, which. Is a gram-negative, bacteria. That can infect a wide variety, of plant species. Okay. And so these have a. DNA, binding domains, and that's what's absolutely key about these so they contain, seven, to 34 homologous, direct, direct, repeats, of these, 33, to 35 amino acids, and these come recognize, a specific DNA, motif that. Is also, still present in a mammalian. So, the specificity. Of each of these, arm. Tails, each of these repeat, sequences, contained, within the two amino acid positions that are 12 and 13, of each repeat I'm going to show you a picture in a minute they'll make more of this clear, and these, are these, repeat, variable. Duress. Would use so. That's what the specificity, comes in now, the key, thing is is each target.
Site For these tails in the, genome requires a different. DNA. Binding, domain of 500, to 7,000. Amino, acids, so, this is made it not, as flexible as, many of the other technologies, available because, you require to. Build it like this and so here, is that. Model that I was showing and you need an awful. Lot of. Space. Of, DNA space to be able to build these different, domains and finding, exactly the sequence that you're going to be able to cut with this. So. The. Nucleus effectors, are these are fuck one domain. The. Acting as a dimer to introduce the cut the DNA double strand a bright and so, you actually should have minimal off target effects because you've got to have both of these sequences, aligned. Correctly to get a cut but the problem is is that you have to design each, of these and these are very expensive so. This has not been very widely, used, but, it does have a lot of specificity. So. The way it will work then is through either DNA repair, through homologous, recombination. Repair where, if you include, a donor, or. It. Will you can integrate. In, a sequence, or it will introduce non random point mutations, targeted, deletions, or as I say if you put in a donor you can get in a DNA fragment. Equally. You can have error-prone. Non-homologous, end joining, and. This will induce deletions. Insertions or, substitutions. And that's where you're, going to get your loss of functional. Phenotype, because, it's going to have a frameshift mutation. Or it'll truncate, your protein. Now. The way it could has been adapted by several, groups to try and not, induce. Repression. Rather than a complete DNA editing. Is to. Use a. Couple. Associated. Box a crab domain, which, is then fused, to. This. One. Of the DNA binding domains, in this case. That. Will target a, transcriptional. Start site, so effectively, we'll just sort of sit there and it will block the ability of, the, transcription, to, occur at that site now the different, disadvantage, do you have to often a test different sites within the transcriptional, start site to, avoid the occupancy, of where the core promoter is up because you're sort of you've got a you've, got to be able to actually interact at, the site to block, where. The, transcription factors, are going to be fine or whether it's high nuclear, zone so actually, being able to design, these can be quite difficult as a, monomer, these can induce or off target effects that was the advantage of here where you have the double this. This is less. Less nonspecific. Than here, where you tend to have a risk, of off-target, effects, because trying, to find these sequences. Can be quite difficult, well. A lot of groups have used them they've, been less used in the context of screening. So. Now I want to turn to what I'm sure, everybody, in this room is now heard of and actually just out of curiosity, so, how many people have actually done CRISPR, experiments. Okay. I'm trying to get a scent wheel across, the campus we're trying to get a sense actually of how many people so how many people have done it s RNA experiments, or RNA AI experiments. Okay, all right and how, many people have done telling experiments, no. One okay all right would you and I know I can't see. What everyone, looking. On the web is do it but we're just trying to get a sense across campus, how much people are using these types of technologies the moment so obviously you can't at the moment open your newspaper not find any you know somebody talking about what people are doing with CRISPR, at the moment I'm, not going to go into, I, could, do a two hour lecture on Chris but I am NOT going to do that so I'm just gonna give you some basics. Just. To make sure everybody's, up to speed so there. Are really comes in three major flavors so this is as I say changing, by the day at the moment one, where you're predominantly. Using, a, highly. Active cast 9 nucleus, that, will induce a faint shift DNA mutation, and then is therefore a permanent, knockout so this is more like that you know you're making, and a single.
You're. Going to make a change that is going to completely knock out a particular gene, if you've, designed. The. Targeting. The guide molecule, is a sink, is, a guide RNA. Correctly. So, this information you're, going to need ideally. You're going to need to the whole transcriptome. That you're actually going to need to the genomic, information to. Because. It's going to cut the genome, okay. There, are isn't, off target space across the genome and I'm going to talk about that in a few minutes in this context, and you, have the potential to be able to target lots of variants if you know exactly where those are all mapping, to now. There are now, increasingly. A couple, of other flavors of, CRISPR, where, you are trying to do repression, of transcription or, activation, of transcription. In a way that's very analogous, to in, the case of I to, RNA I or where you're using the. What. I've just shown for the talons where you again putting on a crab domain to block, expression. At a transcriptional. Start site so. This is really the same as you'll see I just described for the talons, and then CRISPR, a is where you're activating. Where. You're using a VP 64, to actually activate, expression and I'll show a picture for that in a minute okay. So. Past. Nine, is. The CRISPR associated. Protein. Nine a nucleus, that was identified. In the streptococcus. Pyogenes as, the and this is the most major source of Kass nine there, are now increasingly. Lots of other cast. Molecules. That are being found in different bacteria. That have slightly different. Properties. Most. Recent one of which is cast thirteen and that, appears to be able to act out. To, edit. RNA. So. Cassadine, has innate in nucleus, activity. And the targeting. Is achieved, using the, crispr, RNA which. Is shown here and, the trans activating. RNA. Which can be formed as a single, guide. RNA. Here, okay, and I'm going to go into this a little tiny bit more detail, alright. So the constant, region of any, guide RNA, is going to interact, with the cast nine and then the variable. Region is going to interact with your genome target, of about 17, to 20 nucleotides, and the way that that is recognized, is by what's known as the pam sequence, so, this is the proto spacer. Adjacent. Motif or pam that is a short region immediately, three-pound, of the sequence that you want to target so, if your here's the it. Represented. Here okay so you need to know exactly where the pam sequences, and then you can predict, where the, cast nine will will, cut. So. The pam, sequence, for cast 9 from. Streptococcus, pyogenes is, ng g so as you can imagine there's, a lot of those having, said that there are still some parts of the genome where, actually there isn't one that's convenient, and so in those cases actually what individuals, are doing is that you're going back to talents, because, you suddenly, as I say it's about having the right option, for your, particular question. So. Here. It's showing the different types of gene editing strategies, that exist so, a dry page is said for four talents if you have you can have non-homologous, end joining which. Will result in an, insertion, or a deletion which, if it's induces. An outer frame mutation, will mean that now this this, gene will no longer be expressed, if you, add in a donor DNA, you can then get homology, directed repair which, you can use to insert, a gene and this can be very useful as a way of tagging. Genes, either at the three prime end or the five prime end to. Maybe put on an epitope, tag or to put on a fluorescent. Tag that you can use for imaging, and this is now increasingly, being looked. At on a larger, scale, so. You can also and, this is becoming very important, is you can actually now get, a much more precise. Homology. Directed repair by. Actually using two different, molecules to, allow you to insert, and so one of the approaches, that this is being used for is even to try and model translocations. So, you're actually trying to if people are trying to take transportations. That have been seen in. In. Different tumors, they're using, a multiple. Recognition. Sites to actually try and induce that, transportation. In. Our model, systems. So. I mentioned ammonia, go that we can also use, a nuclease deficient.
Cast Iron so these are known as dead cast nines or D cast nines that, you can then put on with effector molecules, to also, manipulate. You in expression, so in the same way as I mentioned for talents earlier you can put in a crab domain, that will act to repress. Gene expression. If you, can target exactly, at the. Transcriptionstar. Site, this is done typically, about 500, base pairs downstream for the tran for the same reason again you need to find a low occupancy. Site. For, the molecule. For the cast nine to interact, with the. In expression, activation. You usually is fused to a transcriptional, activators, such as VP 64. Which, will then actually induce, expression and. Finally, one, of the other options that people have been using these types of technology is actually you can actually add on, a first and protein just to the caste 9 and you can use that for visualization, purposes. And. So that can be has, been used by a lot of groups. So. I just want to do stress, about the off target, effects on this because this, is going to be relevant as we go through this so. In the context. Of, caste. 9 or, in in. The context of CRISPR there's a couple of pieces of information so. The proximal, 8 to 14 nucleotides, that are immediately up straight strand, upstream, of the cam are the, most important, for targets, like cleavage and so you've really got a look. At a lot of different options when. Trying to design where. You're going to be targeting, and in this case you can have the, cassadine combined to a large number of off target, sites and the levels, of the guide RNA may also be contributing, to the off target, effects and I'm going to talk about how groups. Have been looking at addressing this in a moment so one of the ways to do this is, by. Making. Mutations. In to Cassadine, nucleus, Mane's and turn, the cosmos from a nucleus into a neck ace but. Then you can use the. Two to. Target, adjacent, sites and so, this is one attempt, that groups, have been making to, try and get around this, problem. So. There. Are lots and lots of different options for trying to make, guide, selection. And. There. Are increasingly. Commercial. Sources, but, almost all of those will tell you to test at least three, or more guide. Sequences. Just in the same way as I said you need to test multiple si, rn8, because. We hear it's not just about specificity, and it's about efficacy as well in both of those cases but. I I want to stress I am not advocating. For, either of these algorithms I just pulled a couple that are currently, available that. You might want to look at for, doing guide selection, one is from George, church's lab at Harvard and, the other is from Gregg Hannon's lab. Who's. Now in at. This in, Cambridge, in the UK. So, these, allow, you to try and predict. What might be the best. Guide. RNA, to talk to. A target, your particular gene. Of interest and, one of the things as a feature actually of Gregg. Hammonds is that they have really focused on these dual guide systems, so, they have two guides to try and improve this specificity. Now. One of the other features to consider is, where you're gonna target, within a gene using these guides and I sort of just wanted to point, out here and I apologize I realized that this didn't this figure wasn't in the printout. But. What. These are two studies that came out in, the last year, or. So one. Here from Chris bojack's group at Cold Spring Harbor where. And then this, I think, was from I apologize. A continent, where this one was from I think it was from the Harvard, groups.
Where. It they showed in both in two independent, studies that actually, guide RNAs. Where you're targeting a, specific, change. Trying, get injuries and knock out a complete, knockout phenotype, that if the guides were. Targeting. The functional. Domain so what you're seeing here is this is the functional, domains for, one particular gene, and these, are the key domains that we know are. Involved. In the function of this protein that the guide RNAs. That targeted. These regions. Are more, likely to induce a loss of function, phenotype. Than, those that are targeting. Domains. That have are not, important, to the function of the protein and you've seen this here for a second, gene that you see in these ETA that, where you're targeting the ATP, a site, you, see more effect and this. Was actually seen in exactly the same in. This study also looking at. The. Beta-catenin. Gene, where these proteins. That are involved these. Particular. Guide, RNAs, that were taking were. Targeting. The armadillo. Repeats, with the beta-catenin. Gene, were, much more, effective. Inducing, a phenotype, than those at the five prime three prime to end and. You. See this again, also, in for example in the oral kinase, B so. While other thoughts is that if we induce, these. Mutations. In highly. Functional, domains within proteins, that you're going to get a much more robust, phenotype. So. In the last you, know part, of the. Half sort. Of twenty. Twenty-five minutes what I really want to do now is take some of the. Things. That I've talked about and now apply, it to. In the way that we've done a Meyer in my lab and then throwing a few pieces of information from, other people's lab I said. I'm gonna focus predominantly on. RNA based, studies but the types, of ways you can use these. Effector. Molecules, in is basically the same in terms of thinking of the the downstream, work from that and, I'm going to talk about it in the context, of where, we've gone from a screen so we're actually a genome-wide, a, scale. Study then, down to the specific, gene function analysis and then I'm going to finish with looking at how we've looked at it use these types of techniques to look at the interaction, of genes and and drugs okay, so. I said earlier that my labs predominantly, uses s RNA, mediated, RNA I so we're using small, synthetic. Si RNAs and what, we're in a position to do is actually scale this up through, the. Work of the trans-nih are earning eye screening, facility, that's actually administrative. Lee how the MCAT. Is we can scale this all the way up to. Genome-wide, scale, and, what I'm showing here is where we've actually conducted. A screen. Looking. At the activity. Of a fusion transcription. Factor EWS, fly1 and, we've done it by doing looking. At a reporter. I say that involves two different, cell. Lines same. So on but they were what we call isogenic. One, expression, Oporto. That, will be a readout, for ews by one transcriptional. Activity because. You have a luciferase, reporter that, is fused. To a promoter, that is recognized, by the EWS, by one, transcription. Factor and then as control, is a solo amour we have a reporter, with sem via constitutively. Expressed. Luciferase. And what, we were asking, was the question if we silence. A gene so if we silence gene a or we silenced gene B what is the difference, between that effect on this. Reporter. I save versus this reporter, I say on the basis that if we saw effects just or predominantly, in this cell line where. We are looking, at the effect of the EWS, on the EWS fly one target, gene would we find genes, that were involved, in the expression.
Of This fusion protein, or the activity, of this fusion protein. So. Why do we care about EWS. Fly one and why am I even showing, this to you so II WI, one it is, involved. In a. A. Relatively. Rare but, pediatric. Tumor called Ewing's sarcoma and, Ewing, sarcoma that. What you see is that this - these typically, have a, chromosomal. Translocation, involving chromosome, 11 and 22 that, makes this fusion gene that I mentioned just a moment ago, ews. Fly one and so. The key thing about Ewing. Sarcoma is, that the moment there is very we'd, have an overall survival, rate of about 60 to 70 percent in these individuals, but it's associated, with very intensive, chemotherapy and, radiation and, surgery, in a very young population and, in, fact if there's metastatic, disease or, there are refractory, to this chemotherapy. Our overall. Survival rate is less than 25% so. We're, trying to find new ways of targeting this and this is what the basis of the. Study that we set out to do using. An RNAi ice cream. So. What I'm now summarizing, if you remember is that reporter, I said they'd have talked about just a moment and I'm now summarizing. Here about. Two. Years work in one, graph, okay. So, what I'm showing you here is actually a way where we can look at the entire, genome, so this is where we've looked at every single one of these dots represents, a gene, in the genome and where we've silenced, it with three. Different s RNAs and we've compared, the effect of silencing, those in. The CMV, that, constitutively. Expressed. Reporte, versus, our. Experimental. Which. Is the EWS, fly one read out and, what I'm going to just going to point you here is these were the control, srna's, that Kuras will take out the. Fusion. Gene and then, what we're looking for is anything else that has a similar effect genes, that are going to affect transcription globally. Are going to be a down this axis, here okay. So. What, we can do is score, the effects, of this so I'm showing here for the controls, what we have the srna's, that we're looking for is the difference between these two different screens. As I say on a genome-wide basis, I'm showing you the controls here so we're looking for these values, that are are very negative, in, this case a z-score where, we know that these srna's, are affecting, selectively. The. NRO. B1, assay, and not the CMV this is how we can then rank these so, this is looking at the top genes when we've gone through 21, 21 thousand, genes this, is where we're now looking, at, the the. Rankings, of all the genes across, this, genome-wide. Screen, so. I'm just going to walk you through one. Of the genes that came from this to give you a hint of some of the techniques, that we use and how we use srna's. To then probe, down our individual. Gene function, so, here we're looking at H&R, mph, one so, we found one of our our lead hits shown here, so, this is this these are the genes we're really interested, in because his fly won his EWS l1 and here, was one of our hits h and r and p h1 so. Here, we've now validated. This we've used now gone up to using six, different SI rnas and we, see here that when we silence h9, p h1 we, see if get effects in this assay, that are comparable, to whiff we silenced the EWS, fly one gene. Directly. We. See that when we actually look at the mRNA, levels, and then this is you know a very typical, type of experiment, you're going to conduct if you're gonna use srna's. To look at gene expression if. We silence, H and on pH once I'm showing qrt-pcr, data. Showing the silencing, of Asian are in pH one we see that EWS fly one expression, in these cells is reduced. So we've now got a chance to say that well 89 pH, looks like it's required, for the expression of ews play 1 we. Can see that the same occurs, at the protein, level and, that we lose EWS, weigh one protein again. I want to stress in terms of this particular presentation we're, going through and we're doing this with three different si RNAs. To confirm the specificity. Of this effect. Now. Asia and pH one is an RNA binding, protein, and that allowed us to come up with a hypothesis. That this is actually required for the processing, of ews, by one pre mRNAs, in. A. Subset. Of EWS, flowy WS. Fly one expressing. Sarcomas. We. Now know because if they don't, process. This correctly they end up with a premature, stop codon, and this is related to the fact that we know and goes, back well we've been able to integrate our RNA, I results.
To, All of the data that's now available looking. At the transcript. The transcriptome. Of Ewing sarcoma. It's tumors, and cell, lines and, what we know from looking at the transcriptome, of again, tumors, and Ewing's, sarcoma signs is that there are a subset. Of these, tumors, that have, a breakpoint, that, means that exon, eight from these cells, must, be excised, to make the in frame fusion, and so what we discovered by qrt-pcr, are. Shown as analysis here is that asian r and p h1 is required, for, this processing. Event to remove this exon, eight here, but, it's the. Other cell lines that have a different, breakpoint do not need. H. NR m p h1, and. This, is shown here at a protein, level where if we look at these cells that. Have this. Breakpoint. That includes exon, eight at a genomic level if, we take away asian RM p h1 they no longer make EWS flow one protein, but the other cell lines that have this breakpoint here, or here, they, have, no dependency, on h9 p h1 further. Down from this we can then look to see well I told you that AWS play one is a transfusion. Transcription. Factor and we can see using, this that we can change the mRNA. Expression, of. Target, genes of EWS, by one we silence HR and ph1 in one of these cell lines again. We're using multiple fi, RNA so I'm showing you this to really illustrate how, we can use, srna's, now going, from a genome level now down to looking at an individual, gene and seeing, what he effector, is on a genes. Downstream, of EWS who I want and in fact EWS flow one can actually repress, the, expression of, some genes and we see that here, that when we take away H norm pH one that actually allows, the activation. Of some of these genes. Now. There's an alternative strategy, that we could have used it would, have protecting us a lot longer I think which was to say well what is this actually, the effects, on a very, broad endpoint which is to look at cell viability and, this is what a lot of people have done and they're showing you here is a segue then into some of the studies that have been done like that which is where actually if we had enough so lines and we screened, with hnr mph one and what we're measuring here, is cell viability six. Different s RNAs, and we looked at two different cell, lines we, have the TC 32, cell lines which is the one that we've done all of most of the studies in then we know a dependent, at a nation on pH one expression they are sensitive, to the loss of 89mph, one but the other cell lines are not so, if we sort of could. Have used this as a way to get to this information it, would have been I think a lot harder because, the only way you can actually get to this information is to screen, hundreds. And hundreds, of so lights but, there's actually watch some groups have done and so I'm showing you that because if. You're working on a particular tumor.
Type Of interest. To. You you may want to go into these different datasets. They were both of these were published, earlier this year in July and so, this is a group the, group from Harvard. Where, they screened. That's. Right they screamed, I, think in this case. 500. Cancer cell lines. Looking. For. Specific. Effects, on their viability. So. This was an SH RNAi screen this was also another shRNA, screen in this case is this project drive out of. Novartis. And they, screened. 8,000. Genes in 4,000. Cell line so, one. Of the onsets is you can take these really, really big data sets and then integrate, these with other data sets to find out the types of information I just described, to you but. These, are very. Noisy, and. Can. Be really difficult to interpret so I want. You to be aware of them please go look at them in your particular cancer, type you may have may have interest to you but just, be be wary of, some. Of the information it's quite noisy. Now. Because. We've spent the last decade using, RNA AI screens. On a very large scale if of course it was really quite quick though people turn to doing, CRISPR. Based screens, again here using, cell viability as, an endpoint and there, were two studies that came out last. Year both published in in cancer, discovery, where, in this case they took this, study took 33, a, cancer. Cell lines looked. At basically. The whole genome, I'm, looking at 19,000. Genes looking. At a six guys per, per year per. Gene and. They, were doing these screens where they use about 500. Guides per, cell, then. The other study took just, five cell lines and and, just. Under 3,000, genes but they had many more. Guides. Per gene so these were the first sort of really really large-scale screens, that were done in, multiple. Cancer cell lines now, they got a lot of information. Out of these that was very was, gene specific and, I'd urge you to go look at these but, there was a problem, that came from these that's very relevant to thinking about the off target, of faith in that what they saw, was, in regions. Where there was significant. Amplification. Which is obviously something you've seen a lot of different cancers, so. In these incidents where there was a region, that was amplified, what. You saw them was there any guide, RNA. That was targeting, that amplified, region, was, reading out as a false positive. So. One was that why was that the case well. So here is an analysis. Here looking at two different, regions. Around. The Met gene or the Yap gene in. Either, a gastric, cell line or in a brain cell line and this.
Is The data where they looked at the shrnas. And this is data compared. For the CRISPR so this was from Monsanto, in cancer. Discovery, and, what, are you showing here as you can see here, that this, is. The shrnas. And this was really basically a straight line and really, what it was focusing. On in these if you see the orange dots was around, the, met gene where, you really in this case the met gene or in this case around. The. West, Yap as yeah this was around the Yap gene. This. Was what this was working. The. Green. That's. The green the greens hidden I'm sorry it should be the green but. Is what this had work exactly. The way it was expected, to what, was strange on the CRISPR, was you saw this this line is now no longer focused. Just around the, genes of interest and, what, it was discovered, was that when the caste 9 is interacting, with these amplified, regions you end up setting up a DNA damage response and, this is actually, resulting, in a false positive response. So. It's. Again just being careful that if you're using a cell line for your types of studies using CRISPR and caste 9 you may need to be very aware that if you're targeting and I amplified region, this may not be the best strategy for you. Okay. So, in the last few. Minutes I'm now going to just move to another way that we've been applying RNAi. Eye screening. And I've been bringing, this in really because of the translational. Focus. Of this particular course, which, is where we've been using our RNAi, eye and, a lot of others are now moving on into using this in different contexts, to. Look at how to. Understand. Drug mechanism, of action or combining. Knockdown to find ways of chemists sensitizing. Different. Drugs. And I'm going to talk about one study that we've done in the context of breast cancer but we've published many over the last few years in this context, so, what is the rationale, for doing this and I've obviously, done this for RNAi eye base but it would be the same in whatever the, approach, you're using was, the RNA based analysis, for drug activity why are we doing this well, one of the reasons is for looking at identifying synergistic.
Like Euler targets, that can exploit complementary. Vulnerabilities. So, you want it in a lot of cases increasingly. You want to be able to do combination studies. Those. Are very very expensive so almost no. Drug. That's coming in for the cancer field today. Is going to be used alone it needs to be done in combination but. Those are expensive, to do if we can find out off the bat what would be the right combinations. To do that, would be more efficient, and so that's one of the things that we're trying to do it should allow you to use some drugs at lower concentrations. Which will have to be highly effective you, have the potential, to overcome drug, resistance, and you can actually broaden, the clinical application, to many other cancer, types. So. I'm going to just talk about one of the studies that we published now a couple of years ago to. Illustrate, some of the points that I've, raised and how we've gone about doing, these, studies so, I'm going to talk about trail. Which. Is the tumor necrosis factor related. Apoptosis inducing, ligand. Which, is in part of the apoptosis, extrinsic. Pathway and. What, we did and this was work done with Stanley, pivots in the women's malignancy. Branch, here at CCR, and what, he was interested in looking is finding, predictive, bligh of biomarkers, for the responsive, trial for, a trail because. They wanted to do, trials. With, trail and they. Were interesting, also with all you could predict what would be the right combination. Strategies. For using trail so trails become a very interesting potential, target. Particularly. In in breast cancer so, we devised, for, this what we wanted to look at was the different stages in the way that trail, is. Triggering. Apoptosis, and, conducts. A whole series of different screens, that would allow it to assay, what was occurring if we manipulated. Different genes to. Alter, this response, looking. At different points. In the pathway looking, at the activation, of caspase, 8 one of the first effector, molecules, then. Looking at activated, caspase-3 7, and then looking eventually, at loss of viability. So. What this involved, was doing with a whole series of parallel, or no I screens we focused, on the.
Chi Know and phosphor tompas that are about another 300 50 additional genes, and we were going to do these in the context, of the absence, and the presence of trials we're adding trial and seeing what the responses, are this was all done initially in the screening format, in M D and B 231, cells and so we had three different endpoints. So. One. Of the absolute, critical things if you're going to look at in the context of a screen, whether. It was the screen, I discussed earlier or in this one is you have to have very robust. Controls. To be able to analyze, your. Screening, data and for this what we had to do was actually obviously was fine a try. Controls. That would work in all three assays, in the end what we were looking for with one that would actually, suppress. Our signal, and ones that would activate our signal, so if we can actually use srna's, to caspase-8. Here. This will actually block, this, response. It's. Not working. And, then if you use srna's. To flip this will actually activate, the. Caspase-8. Response, so, these were the controls here, they're actually much more robust in the caspase-3 seven, I say yeah. But you actually go also have to remember that in there we, see the wreath there you. End up with the different. Set of controls for the cell viability assay. Caspase-8. Should have no effect. It's. Right that's where we're going to actually block the response, in the tonk context of cell viability and it, will be, enhanced in the context of flip. Okay. So. Again, what I talked about when I showed you these big graphs when you're going during this scale this is again two years work in six, graphs this time rather than one, but. Where we were trying to get here was actually then show the interaction between these, different, that. The datasets that we generated, the key thing first is in the absence of trial we really see no noise and this was really important your baseline needs to be really. Nothing. And that was really good we saw, no then, once we had trial we see a very, broad, range of responses. But what we were looking for was seeing where was the correlation, between responses. Between the different assays in this case well we're looking at caspase-3, versus. Caspase-8, here, caspase-8, versus cell viability. Here what we saw really nice correlations, are shared between caspase-3, seven and cell viability now, these may not look the best our values, in the world and the concepts of G of very large screening, these are actually pretty good for what we're trying to get out of this okay, what. We can then do is drill down into this data and there are multiple, ways of drilling down into this data so I'm just going to show you one of the ways that we're able to do this which is particularly to use pathway, analysis, or network analysis, tools to see where there's an you know there's a lot of noise here but. Can we pull out the signal from this noise and so, what we were able to do here was sort of show where there were nodes that where we saw hits where, there were three or more srna's that were associated, with the phenotypes, we sort of saw nodes around sark we saw nodes around bcl-2. L1 which should be expected, and we were really pleased to see that we. Also saw some nodes, around. Fgfr4. But, yes up we, thought oh that was because that was a link to start so, we saw these these links, and we were able to then go back and start drilling down into these looking. At these in India at the individual, assays. Importantly. We were able to take some of the lead. Hits we were had from this and then, now go back and do a sort of a secondary, screen but now increasing. The different. To, number of cells, types. Including. Those that are now quite trail resistant, and we're able to now take a, silver, is actually quite trail resistant, we silenced with, an F RNA we can now trigger, the. In. This, case so. I think a change in cell viability. We. Know caspase-3, seven, activation, we now see activation. In. A trail resistance, cell line so, you can actually then take this now.
Into A drug setting, so, there are bcl-2. L1. This. Is actually a bcl-xl. Bcl2, bcl-xl. And. Shown, that this actually combination, with trail is actually, able to, sensitize. Cells, so you have an, synergistic. Interaction that. Was predicted, by our RNA ice cream. So. From. Our study, which. Will allow me to a segue to something else is that we actually found about 150. GS who are identified, as putative, negative, regulators, of trial induced apoptosis oh and. We were able to use small compounds, so I should confirm, this both. Compounds. To sulk and bcl-2, old one came from this but. Actually understanding this. Was route became really complicated. And what we realize is the ash we needed more data from this and I mentioned, earlier about the, trans-nih our inner eye screening facility, and I'm going to just mention that at the very end of this so this was one of the project that was actually expanded, to go into, this so. A. Now. Over ten years ago I helped. Found and until. Earlier. This year I oversaw. At the, 60,000. Foot feet level type of thing this trans-nih, RNA I screen the facility this basically, allows us to interrogate the genome, in the same ways people have been doing drug screens for the last. You. Know 20 or 30 years it's, a fully automated, system where, we can look at a rare e range of different, endpoints simple phenotypes, some of the ones I've described here we, can look at pathways using reporter, assays in now increasingly, we can do high, content, imaging, that's one of the things that we're working towards, is being able to do that in. The lab so. This, is. Actually, available to. The. Entire NIH, intramural, program. But. Your be careful, because your PI's, need, to put in major major, work to make these to. Be able to access these, these, are very very expensive screens, and so there's a full review process, for it but every year we. Request. Projects, for, this facility today. They've looked at lots of different endpoints. In the context, of cancer I just described one of these which was the Ewing sarcoma 1, babli, as to say expanded, to some of the others, studies, that have been started, in my lab and and gone on to this and these are all the cancer targets, but they've also done a lot in infectious, diseases you're about to hear about HIV. So. I wanted a plug in there we've even done an in, bola based, RNAi, I screen that was interesting, and. Then we looked at lots of different, disease, phenotypes, I'm just gonna finish there and I think got time for just a couple of questions. I. Think. It's both but immune to purified, protein. Was. The scale up yeah, so it's all done in 384. Well plates, so. It will vary from cell, type to soul type how many cells you're going to need from that so typically. We'll usually, if it's a if it's an epithelial, so on it's about 500, cells per Wow okay. So, then you're gonna is gonna scale, that up to. Hundreds. Of plates, okay it's usually done out so I always, joke I see the screen is the simplest part of it it's usually bout done over about a two-week period so it takes us usually about a year, to get to the point where you can do a screen the, screen is it's two weeks work and then it's another three years trying to figure out what it told you if. It's done well. So. I'd. Have to do the math on that I've been a long time so that I actually had to worry about doing the screen I. Think, you a bit you know to scale up accordingly. But. It's all done in 384, well plates. Sometimes. You've done a tour to n hats in the past yeah with, this group. You've. Done a tour to and cats with this group in the past right maybe yes so I don't know if you'll get a chance to see it. Okay. Thank you. Well. I'd like to wish everyone a, Happy Thanksgiving, and, our, last. Speaker is Frank. Mulder LA and. He's. The, investigator, and the host virus, interaction.
Branch. HIV. Dynamics, and replications, program. You. Know does PhD, from City University. New York an. MD from Mount Sinai School of Medicine, did. His residency in, internal medicine at the Columbia, Presbyterian Hospital, New, York and. He. Joined. NIAID. Initially. As a staff fellow and. Subsequently. He joined the HIV, drug resistance, program I'm, sure. You'll enjoy his lecture on retroviruses Frank. XOR. Erik. It's. Nice to see you all I don't, think I have anything as extensive, as 21,000. Different. Screens. Or drug screens but. I do have a few drugs and. It. And. I'd, like to talk about how we got to there and. Also although it may seem a. Little unusual to have a lecture, on retroviruses or. On HIV, I should say in an. NCI, I, think. You'll, see that, retroviruses. Probably, had a very have a very, deep history in our Institute, and so I'm. Very grateful for the opportunity under, Terry to be able to talk about that this afternoon. So. What. I'd like to discuss, really is, the. Molecular biology and replication, of the virus retroviruses. In human, populations. How, they emerged and they spread how, we treat them and end some lessons, and I. Would, just encourage you to interrupt. Me at any point in case especially in case I go ranting. In one direction or another. So. First of all to. Start out with with, one particular retrovirus. HIV, and this is HIV, one to. Give you a sense of the. Problem. About. 36. To 37 million, people are infected with HIV worldwide. And I, think what you can see is that the. Majority of them are here. In sub-saharan. Africa, in fact. 2/3, of the problem but also place. Like Southeast. Asia and. The. Caribbean. Have. A significant. Disease. Burden, and finally. There are areas where the disease is expanding. And I would add, Central. Europe where it's. It. Hasn't exploded but it's certainly. Increased. Rather quickly, and remained, endemic, and I, think the thing I wanted to point out is that depending upon where you look. The. Risk for HIV, is different, in those places so for, instance in in. Central. Asia, former. Soviet Union the. Epidemic, was initiated, through IV drug use and perpetuated. That way with. Some. Commercial. Sex work at csw, men. Who have sex with men hardly. Any and then this other group other which. I'll come back to in, Southeast. Asia, it's. A largely, driven, released. Initiated. And propagated, through commercial, sex work with. Some, intravenous. Drug use and some. Men who have sex with men and. Then. Here, in. The Caribbean now the Western, Hemisphere a significant. Epidemic, but. As you can see not. As much commercial sex work or IV, drug use but. Reported, MSM, and in, each case this. Transmission. Risk category. Known, as other is either, more or less predominant. And I think the reasons for that is that, this is really. Proportional. To, the stigma, of HIV. Plus. The stigma, of. Of. Being, male, who has sex with men so. You'll notice that this. Group other here, probably. Includes. All of these individuals. Who can't disclose, their status, here. In Southeast, Asia where things, are a little bit more. I. Guess. Freer where you can say you're a commercial sex worker but, still other twenty, four percent and here in Latin. America 38 percent so, I guess, my point is I'm not so sure what. All. The driving risks, are for, HIV, infection in, today's. World, because. We can't get a handle, on all. Of the demographics. But, it certainly suggests that. That. There's a substantial. Problem. To. Understand, you, know what. To do about it I think, we need to know a little bit more about the molecular biology and replication, and so, that's what I want to talk about next and as sort of curious like. You. Guys are all in the NCI. Who's. NCI. Who's. Non NCI, who. Doesn't have hands alright nevermind. Are. You working in labs are you working in office it's labs. So. Pretty much our offices. Any. Familiarity. With, things. Like, cDNA. Does, that word mean anything, to anybody, okay. It. Helps. So. If we were going to define, retroviruses. It's, not viruses, that came from the, 1970s. With plaid. And, polyester. But. Instead they're a group of RNA, but this is these are the jokes it's Monday before Thanksgiving I'm. Sorry teri this is the best I'm going to be able to do there. They're a group of RNA, viruses, that replicate, via DNA, intermediate. Using, reverse transcriptase. And so, they're developed, in the nineteen that, there's they're really identified, in the, mid to late 1960s. And if you think about it that's. About 15. Years 16, years after. The. Structure, of DNA was identified. And maybe about 20, after, it was relatively. Clear that DNA was the genetic material. And in, those those, first 20 years I think one of the predominant. Paradigms. Was, that you start with DNA you, made RNA and then you made protein, and along. Comes a virus like retroviruses.
Or, What would become retroviruses. What we're known as RNA tumor, viruses, before that, and. There, you, have a DNA. Molecule that's, integrated, into the host right. It. Produces. RNA, any infected cell it puts RNA, in the B Rhian and then. That RNA, in the virion gets transcribed, into, a DNA, mall kill before, it's integrated, in the host in other words this. Is going against. The, current of the time in the 1960s. And for, those reasons not. DNA, to RNA to, protein, but RNA, to DNA to. RNA to protein, it was, it. Was named. As a retrovirus. Because. It was going in the opposite direction. So. It's not, only a different paradigm, for replication, but, it as, it turns out, it's. Quite it has been or at least the theory is it's been quite a benefit. Not. Just for you molecular, biologists, who could raise your hands when they heard a cDNA, because the only way to make that is with reverse, transcriptase. But. Perhaps for. The other organisms. On the, planet who, don't work in molecular biology labs. And so, if you look at all of, the current phyla. Every. Last one of them has in its. Host. Genomic. DNA a copy. Of reverse transcriptase. And as. You know because, I see, these folks every year thanks to Terry I know how smart you guys are and. I, know that you already know, that life. On Earth probably, did not start, with. DNA as its genetic, material but, as RNA, is its genetic, material is that a surprise to any of you it was, what they call an RNA, world. The, earliest enzymes. Are, our. RNA. Enzymes, and so, it has, been thought for a number, of years now that, life on earth started out as an RNA molecule and as, you can imagine you, know just, like us the, first organisms, grew. Developed. Divided, and. Created. You. Know, poop. Side. Effects things, that it made in the process of replicating, those things in the, case of RNA molecules, was, molecular, oxygen and, as, you know if you've made cDNA, that. Molecular, ology. Molecular. Oxygen, is, the. Enemy, of. RNA. And, so. Imagine. Living in a planet, this, planet. Where. The. Only organisms. Are making, molecular, oxygen there, isn't a lot at, the time but, the more of it accumulates, the more vulnerable, your, genetic, material is and so, the.
Theory, And it's a theory I'm, almost old, enough to remember and, Terry, is not what, it was like 15, billion years ago but, the theory is that things started out as RNA and the organisms. That were able to persist, were, those that could convert, their RNA. Their. RNA genome into something a little bit more stable in the presence of molecular oxygen and, that was a, DNA. Molecule and so getting from that RNA, to DNA. You. Know they all got a reverse transcriptase. Maybe that's basically. A remnant of the. Of. The of. The process Jedi, Knights as somebody would call them who, saved life, on Earth by. Converting, our making, sure that the organisms, could convert RNA, into DNA so if, you ever get out of the NIH this is a lovely park Seneca, Creek Park up on copper, road. Anywhere. You look in the. Plants, or any of the phyla, you'll find the reverse transcriptase. They're all around us and. So. If we look at the families, now of viruses, that use reverse, transcriptase. They're, divided up into this seven, in. In, the form of this phylogenetic. Tree I like. To show this around this time of year because some of you may be going to, I. Don't, know a family. Event on Thursday. And they'll be you, know you can, look, around the table and maybe draw a family, tree well there's the family tree for retroviruses. And as you can see there are about seven of them the point I'd like to make is that there there, are all kinds. Of, organisms. And the, ones that infect humans, are down here, one called htlv. For human t-cell leukemia. Virus. Discovered in part here, in the NCI and, the other set is lentiviruses. And you can see there are a couple of variants, and one. That is probably very familiar all, to all of you HIV, one and. There's a bunch, of others here and that these spuma, viruses, which, don't typically, infect, humans, can but, don't cause any disease, they're typically in primates, and, there's a bunch of other ones here's mice. Here's. One that are is in monkeys, this is mouse mammary tumor, virus here's, ones in chickens, and the. Closest. Relative to htlv. Is actually, bovine, leukemia virus. Is a, as. An infection, of cows and so, the point I'm really trying to make here is that the, the. Phylogeny, of the virus, doesn't. Recapitulate. The phylogeny, of, the, host so. If it did then we'd expect that, htlv, would be up here with the lengthy viruses, they all infect, humans, but, they are very, different, from each other and so, just because you infect the same host doesn't. Mean you, you. Are closely related to each other if we. Cone down now just, on the HIV, viruses. Or the human immunodeficiency virus, we. See that a second, time so, here's just the, all, of the viruses that are closely related to HIV one and those, of you know a family, trees are gonna create one on Thursday. You. Know that the, the closest, relatives, are. The ones that have the least. Horizontal. Distance, in a phylogenetic, tree the, vertical, is just display, all the organisms, out and the, horizontal is really here so to get from. HIV, one to this virus you only have to go here. Down. Doesn't count to here so, these two horizontal. Lines is essentially, the genetic distance between HIV, one and this, other thing the other thing is actually a virus. That infects, chimpanzees. So, the closest, relative to HIV, one is a, chimp, virus, and as, you can see that, there's another HIV, on this one HIV, to a totally, distinct, virus, but, look at the genetic distance to get there there's this it's, this there's, this forget about the vertical and then, here and here but. This virus, has a very close relative, also. In the, the, non-human, primate, community, and it's, an a sooty mangabey, so this, is a chimp, this, is a city mangabey. That's. A monkey. This, virus, is. 98%. Identical. To. This virus so, they're very closely related this, probably.
Around 70%. So. Once again just, because you infect the same host doesn't. Mean you're that. Closely. Related this. Kind of analysis, gives us some real insight as to where, the viruses originally, came from and and a little bit how they got there, the. Other viruses, in this group in. Fact again monkeys. This, is mandrill, and then, you get a whole wing of the, family, that infects, these. Are goats, horses. Cows. And, cats. Feline, immunodeficiency virus. Some. Of you may have had. Some experience, with that with pets. This. For referen
2017-11-24 01:44