THE INVESTMENT BLUEPRINT Podcast by RAIN Technologies, Episode 1: Sonam Srivastava
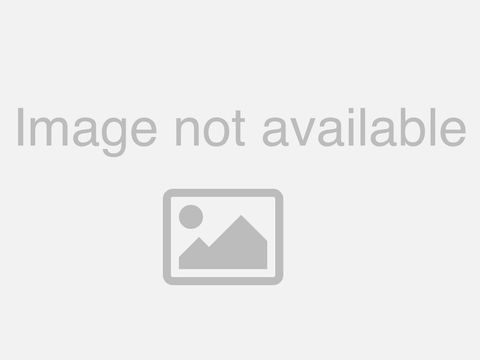
[Music] hi welcome to the investment blueprint i'm your host raghu kumar from rain technologies rain is a provider of fully automated trading solutions to learn more visit rainfund.org the investment blueprint is a weekly podcast where you can find me interviewing real-world quantitative traders and investors hedge fund managers and industry experts in the field of quantitative data driven trading and investing my goal is simple i want to help you make more consistent profitable trading decisions in a data driven and meaningful manner the general layout for this podcast will be a bit different from the one we had launched a few months ago titled the rain podcast with the investment blueprint each episode will feature a highly focused topic and each episode will be more bite-sized in nature the idea is for you to walk away from each episode with actionable insights on the topic discussed today's topic is on how to do algorithmic trading in india with the focus on the ideation phase as all quants know coming up with a good idea is perhaps just as important if not more important than back testing and deploying the strategy in the first place but how does one actually ideate a good trading idea luckily for us we have an expert to discuss this topic with my friend sonam srivastava from write research sonam is an iit kanpur graduate with a master's in financial engineering from world quant university she has worked in portfolio management roles at hsbc and q plum and as an algorithmic trader at edelweiss and forefront capital wright research is a new age quantitative active investment firm dedicated to helping investors reach their investment goals and navigate the risks of investing through strong technologies and investment acumen so so and welcome to the podcast hi uh hi ragu thank you for the introduction awesome so i thought we can kind of start today's conversation by talking about your background um and specifically how you got into algorithmic trading right so yeah algorithm my journey was again very similar to other coins was not uh you know very well defined i think in india algo trading sort of started in early 2010 9 10 only and that was the time when i graduated from college it was an interesting field that everybody was looking at but i didn't get my first job in i'll go trading i started in chemical engineering and because i knew that i had a lot of interest in you know algorithms and technology i moved moved out of chemical engineering to and i started studying for cfa and where i you know when i studied about quant i thought that this was really interesting because it is an application of data and numbers which is something that is really interesting for me and that's how i made my switch i joined forefront capital which was a boutique hedge fund in india back in 2000 2008 to 12 and i joined them and since then i moved to hsbc and that's how my journey started got it got it yeah and um and specifically with algorithmic trading when did you first kind of make that switch from discretionary trading to algorithmic trading so i never started doing discretionary trading i started with the algorithmic trading i mean i sort of started like when i when i joined that firm i was given you know things like uh analyzing the data and you know creating sheets for other portfolio managers and stuff like that and eventually i moved on to you know actual trading got it got it so obviously today's topic is more on the ideation phase you know i think that's a a part of the process where um it gets easily overlooked by a lot of people but before we specifically talk about that i wanted to kind of ask you you know from a bird's eye perspective or high-level perspective what is the entire process for building an algorithmic trading strategy yeah so the whole process i think it is a long process it is not a quick process it starts with an ideation phase where you you know you start with an idea and you don't have a lot of you know specifics on that idea and then then you go into the research phase where you might uh re go through journals by other researchers on the topic you might do a google search and find a lot of stuff now on various blogs or you can talk to other people who have implemented these strategies and once you have your research in place i and then like for me because you're data driven it's always a good idea for us to you know actually take the data and start playing with it uh right see if the thing that you're trying to model is actually there or not and once you have looked at the data a little bit then that's when we go to the back testing phase which is implement the thesis that you have found during your research and see what is the performance of it and then you iterate on it quite a bit right it is always a good idea to have in sample out sample demarcation between your data even when when you are back testing and after after a few iterations um i mean if you have a good good thing that works well both in in sample and out sample then we do the pilot and in the life right so that is generally a general view of how to sure yeah yeah so and in terms of you know the strategies which which end up being good ideas right or are kind of based upon good ideas what are some kind of common elements of good trading ideas um the common elements of good trading ideas is first of all they should be backed by you know a fundamental reason fundamental not as in based on fundamental data but something you know that is very clearly there uh anomaly that you're trying to model right so it should have a solid reason for that strategy to work and once you found it you should found a mathematical model that fits into it which has a certain statistical accuracy and then the consistency of that idea should be there over time right it shouldn't be like it works well in some conditions and it doesn't well after that and yeah and then risk management like you said you should be able to understand the fundamental reason of why that idea is working or not working which is very important right right right right and i think that's the tricky part right where you think you have a good trading idea to work with but maybe the idea itself is flawed and yet the results of your back test might look great you know so then you're kind of stuck in a bad situation got it and in terms of you know the the kind of trading ideas you come up with for your investment and trading strategies what is you know like you know what does your process look like for coming up with a with a good trading idea so you start to start off with an idea as in usually i mean there now there are so many you know public channels people are discussing strategies and what works or doesn't work in the in the market right so you get an idea that you think would be interesting could we could have a lot of quotation and that's sort of the starting point and then what i like to do is i try to research on it a little bit i i like to go into ssrn and other you know industry journals where you you find a lot of good resources about the topics that you're trying to research and then now because we have a very budding and a blog system in algo training you'll find a lot of good articles on medium and various blogs about a lot of things that you're trying to do quantum and yeah it's all always a good idea like if you have an expert that you can talk to somebody who has worked in the field that always helps out a lot sure sure and that kind of gets me to my next question i mean you know usually quants are known to build systems on their own and you know trading is already a very kind of lonely profession and i always feel like algorithmic traders take that to a whole new level um but you know with you specifically do you work with others when you build your strategies uh and do you even kind of seek the approval or you know some sort of communication with others before you look to roll something out live yeah so uh i mean so i have been working and building algo strategies for more than nine years and actually i've had the most fun building them and i think the best experience when you have some sort of a feedback right um so when i was in englewise uh we had a team where of you know we had a certain senior senior people we can talk to but also we had like all these traders who would run that that algorithm once it's been and they would look at the market and they would give you all these feedbacks which really uh you know getting a feedback from people really helps and even hsbc which was one of my best experience building albus i had i had a team of traders in london who would you know review everything that i was doing and you who would you know if not implemented they would like to know you know every specifics of what we were implementing and validated which really helped the chances of anything going wrong goes down a lot and you also validate a lot of yeah so yeah it's always a good idea to have somebody give you yeah and i think i think there has to be both a willingness you know from the quants perspective to to go out and and seek that feedback uh because you know chances are the other person might not agree with everything you say or so you have to be probably a little open to getting critical feedback as well right right yeah that is true yeah so now in terms of you know your your recent strategies uh because i obviously you know uh follow you on social media and we do kind of talk about the different strategies that you work on um can you kind of walk me through like one of your recent strategies um kind of how you came up with the idea um and maybe kind of walk me through that whole process so over the past years i've been working very uh actively on a statistical arbitrage strategy which is like a long shot strategy between futures on the market and that's a very large scale strategy you know it runs it requires a large capital to run i i started talking about it on the retail platforms and you know i got a lot of requests from people that they want something like that but with a smaller capital so with that idea we started our space dating strategy which is which is just a pair trade between nifty and bank nifty and it was really interesting uh strategy uh i had a very good intern working with me on the strategy and we had a and we very quickly implemented this and you know tested it out the idea was to find a patent between nifty and bank nifty so we started off with some mathematical uh formulation as in as the stationarity of the spread and if there's no stationarity then find that stationarity and once we found it we were very very easy to implement it using contra blue shift platform which is a very good uh practicing based platform that they have put up um yeah and once we validated it on a couple of platforms and we were the two of us working on it who had validated it so we were fairly confident and very quickly we went ahead and launched it in a live session got it got it so how long did that whole process take in terms of going from the initial idea to uh you know rolling it out live yeah so we actually did it within a couple of weeks but i mean i had like a basic structure of the strategy ready even before you know we we started this product project right so it was very i mean i had a fair idea because i had looked at the data and implemented something so it was a good starting point and i quickly got the intern up to speed on that and so it didn't take us a lot of time it's a simple strategy in construction so it did not take yeah and in that process where you kind of go from that basic contouring idea to testing it out and eventually deploying it what are some common mistakes people make in that specific step uh i think uh i think um the first thing is that you should uh you should do in sample out sample testing because you could be overfitting something and you have to be careful that you test it out on some data that you haven't looked at before before you take it live people sometimes miss that and you know always staying true to the you know mathematics of the phenomena that you are trying to implement is always a good idea uh so if we are doing fair trading and we make it uh very complicated in terms of technical signals and stuff like that instead of looking at the mean divergent phenomena sure yeah so basically complicating it past the point of what the strategy is based on kind of thing right yeah okay got it um and in terms of you know which asset classes and which data you know you work with are there certain asset classes you prefer over others and if so uh why is that the case yeah so i have always logged in the equity and equity link derivatives asset class like for the nine past nine years uh i work on fx also a little bit but mostly with equity liquidity derivatives in india and you know in the global markets that's the classes that i haven't worked with are our commodities and bonds i think uh looking at it from algo trading perspective commodities could be a very interesting space yeah even bonds is a very interesting place but maybe not in india but in the global u.s market sure and since you you know have so much experience trading the equities market in india because even with me you know when i came to india in 2009 i basically started trading the equities market as well um and that's when the bsc had opened up its uh equities market for algo trading so um so i have a pretty long history with the with the equities market in india as well um but you know in your case what changes have you noticed in the equities market over the years in india have you noticed you know any any trends or changes in the markets algorithmically uh algorithmically see the markets have been you know relatively uh liquid like i mean i actually started doing this in 2012 right resources then the markets were quite liquid and um especially in the switches in auction state space and i was working on a big broking desk which was user wise institutional desk back then for the four years from 2012 to 16 and obviously we we had like i mean the deaths grew by a lot the percentages that we were trying trading the volume that you're feeling grew a lot the spread sort of widened when we're doing arbitrage so i think that should that was the trend across the industry on you know the markets becoming more active more penetrated by algorithms i mean we we started the dma desk at italwice and we didn't have you know trades on it for the first few years and i think that has now become a very significant part of indian trading industry dma now i think sure yeah and generally speaking has it become more difficult to come up with uh consistent alpha generating strategies over the years uh i don't i mean i don't think so i mean i mean i used i work with the global markets from for a couple of years in this process and so it is much difficult to find alpha generating ideas in the global market than in india because india is much more illiquid and you know it has a lot of volatility sure so yeah so india might be easier than global market here yeah i i i would probably agree with you there because i think there's probably less general competition but although that is kind of changing um yeah yeah and so obviously you know a lot of our listeners are going to be just uh aspiring quads and you know um i think there's a lot of mistakes that quants make um but apart from you know mistakes that they make what are some resources that a quant could uh kind of go to to kind of get better at building their own strategies so i think see if you want to do the research you have a lot of good resources like i said you'll find excellent blogs and excellent papers on ssi and mostly free and stuff like that uh quantz pdi you'll find a lot of resources and if you're a aspiring quad i think it's always a good idea for you to implement an experiment on your own i think that is the place where i learned the most by experimenting on my own what really gave me confidence or again was that i did a course with the word corn university which was a master international engineering and it was a very practice driven course where you know the types of things that i like to do experiment on my own we were they were teaching it in a very structured fashion so i think that gave me a lot of confidence and of course is like these those i think i would really recommend and if you're not doing a course then try to do you know learning on your own try to build algos on your own and experiment with data because you'll always need that skill set well thanks so now i think that was a really nice uh you know uh episode for the podcast um yeah and we will include all those you know notes and the uh the show notes of the episode right um so yeah so thank you so much and we'll talk again soon hi thank you [Music] you
2021-04-05 17:32