Technology Radar — preview webinar, Vol. 29 Western
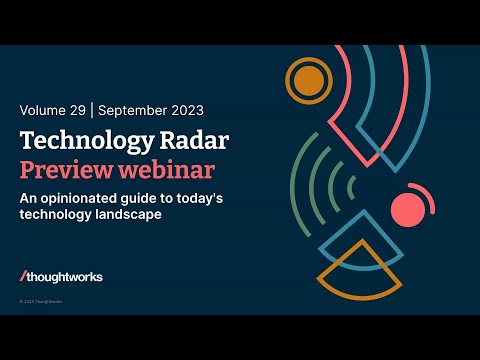
- Welcome, everyone to the Thoughtworks Technology Radar, Volume 29. As you would know from joining us today, the latest version of the Radar is coming out really soon. And what we like to do each time is share a sneak peek with you all by going over some blips and themes from the upcoming Radar. Got a lot of content. Very excited to share that with you. To start with,
let me introduce myself. My name is Vanessa Towers. I'm a principal technologist and co head of tech for Thoughtworks here in North America, and I'll be your moderator for today.
And I'm joined by two of my lovely colleagues who are today's speakers. So we have Camilla Crispin, if you'd like to quickly introduce yourself. - Yeah sure. Thanks, Vanessa. Hello, everyone. I'm Camilla. I'm based in Sao Paulo, Brazil.
I've been at Thoughtworks for over 10 years now. I have a technical background, and my current role is technical director. And I look after the Thoughtworks Brazil's international clients. It is very much a pleasure to be here today.
- Wonderful. Thank you, Camilla. We're very lucky to have you. We also have Neil Ford, who perhaps needs no introduction, but just in case, Neil, can you say a quick hello? - Sure. Hello, everyone. My name is Neil Ford. I've been with Thoughtworks a little over 18 years shockingly. And I've been involved with every Radar session, save one.
I ended up missing one in Chennai in the early days, but I've been involved in every single one of them. And I'd love to talk about it, so I'm super happy to be here today to talk about the latest edition of our Radar. - Thank you, Neil. OK. Let's do a quick introduction to the decorator before we jump into sharing blips.
I'll go over what the Radar is just for anyone who might be joining us for the first time. So the Radar is actually a publication that Thoughtworks produces twice a year. The way we pull it together is, it's actually crowdsourced by more than around 12,000 Thoughtworkers who are out working with clients every day, globally, across our client portfolio. And the thing about Thoughtworkers is we like to share our opinions on the technology landscape we're working in. And so in terms of what's working well, and sometimes more interestingly, what's not working so well on our projects. So that's what we'll be doing today.
And what's really special about our Tech Radar is the fact that we are a technology agnostic consulting company. So importantly, you can't pay Thoughtworks to get something on the Radar. And in fact, you might notice that we have a lot of open source software on it. And that's a reflection of the fact that we use a lot of open source technologies at our clients. So what you'll see on the Radar truly is a reflection of Thoughtworker real world experience in the industry building software alongside our clients. OK,
so let's really quickly talk about the Radar model that we use, which you can see on the right hand side of this slide. So some terminology-- when something lands on the Radar, we will call that a blip. So you'll hear that word, blip, quite a lot today. And then another important one is the rings of the Radar, right? So if we look at the Radar model, and starting at the bottom right corner, that's the center.
And moving outwards, there's actually four rings there. And so the first ring of the Radar is what we call the a dot ring. And so if a blip falls into a dot, what this really means for us is that we think about it as a very proven technology.
And it's quite likely something that we're using quite widely across our client portfolio, and of course, in production. If we move out a little bit to trial, if a blip is in trial, that means it's a little bit less mature than the adopt ring, but we still consider it ready for use with some disclaimers in the right setting. And then for assess, we really think of these blips as something promising or perhaps an emerging technology. It's usually a little bit too early for us to make a definitive call on, but something we think is really interesting and something to keep your eye on, right? And then that last ring, which is hold-- we use this one to communicate technologies and blips that we've seen perhaps use problematically in the wild.
And so it might be that we're seeing a trend that the industry is starting to move away from it. There's a lot of potential for misuse. Or maybe it's just really early, and we don't think it's quite ready for prime time.
So in any case, when we say hold, we really think proceed with caution. OK. So there are four rings. And then the last piece of the Radar that's also very important is that each of these blips or instances that land on the Radar-- we categorize them into quadrants.
And there's four main quadrants that we use. And so at a high level, those are techniques, languages or frameworks, tools, and platforms. And you'll see that today as we move through and share themes and blips with you. All right.
So what you can expect in today's sneak peek is a little bit of a reveal of some of the blips that made it onto the latest Radar and also some of the emerging themes that actually bubbled up from the many conversations that happened in the room during our internal Radar collection process. And we have Neil and Camilla on the call who are members of Doppler, and of course, are in the room for that and would love to share those stories with us. So throughout today's webinar, we want you to be involved. Please ask questions using the Zoom Q&A function. I'll keep an eye on those, and we'll do our best to answer them throughout. So all right. With that, let's get started with some of the content for Volume 29.
I'd love to hand over to Neil to kick off with our first theme for today. - All right. Thank you so much, Vanessa. There's a quick question from Alex in the chat.
I'll go ahead and answer. Does a blip move towards the arc or away? That really depends. This is actually a change we made to our Radar, which reflects how fast the technology ecosystem is changing. Because we used to-- once a blip had made it on the Radar, it automatically stayed in that spot for the next Radar unless we wanted to move it. So it stayed there for a year. But we realized a few years ago that the technology-- the pace of change has picked up, and so now each blip has to fight for its survival on every Radar. So sometimes it moves in, sometimes it moves out.
It's not unusual that sometimes something is promising in a sense and goes to trial, and we realize, Oh, there are big problems. And it moves back to hold. So that's all contingent upon-- and very much-- as Vanessa was mentioning, this is a very reflective process. So the people on the Radar panel-- we don't nominate any of these things. These are reflected from Thoughtworks projects, and we are just the filtering mechanism.
And one of the things that we do for every Radar, and probably the most organic thing that we as a group create, are themes. So when we start sifting through all of these blips, and it seems like every time we put one of these things together, we have the most number of blips ever. And guess what? This time, we had the most number of blips ever. We had 300 and something blips to sort through, and our goal is to get it down to 100 or 110, or something like that. So that makes for a really long week and a lot of very intense conversations. But one of the things that we do during those conversations during the week is look for overarching themes.
So one of the things that we will talk about late in the webinar today is something that we call too complex to blip. It's because to put something on our Radar, we have to be able to summarize it in about a paragraph. And that's not a lot of text, particularly when you get to really nuanced things. And sometimes things happen that we notice a preponderance of things happening on our Radar. Lots of connected
things, and that's where themes come in. So at the very end of our meeting, at the end of the week, we sit down as a group and nominate what themes have we seen emerge from our conversations and from the blips that either made it or didn't make it onto our Radar. And we typically have three to five themes for every Radar. And this is our really only opportunity to address across blip connectivity of things.
Because everything in our Radar is very discrete because it's a single blip, a snapshot, about that particular technology. So we're going to highlight a couple of the themes today, too, as part of our highlight journey. And the first one is going to shock no one. That we ended up with an AI assisted software development theme on our Radar. It's common when we do our Radar that there will be an overwhelming number of blips around a particular technology or family. I remember a few years ago, we drowned in JavaScript blips because when JavaScript was really entering the ecosystem in a big way, there was just an ocean of JavaScript related material that we had to sift through.
In fact, one of our themes for that radar was-- I believe one of our titles was the key to the JavaScript ecosystem comes down to merely chaotic or something like that because there was so much of it. But even in the heyday of all of that complexity, we never had to resort to an extra visual realization to understand all of the stuff until this one. We had so many related things happening on our Radar that we ended up having to create an additional visualization to categorize it and sort it and understand it. And we're going to show you a few pieces of that visualization today. It will find its way into some
publications that we will do through our insights channel or something like that within Thoughtworks because it's a really great way of categorizing the ecosystem right now. But that was how many of these AI assisted software development themes we ended up with this time. We ended up with-- I think the final count was 23 or 25 different blips on our Radar around this subject. We went through probably
50 or 60 related blips because AI assisted software development is very purposefully worded this way. Because it's not just AI assisted coding. It's AI-assisted software development. So one of the things that we found really interesting were the number of non-coding blips that came up around AI, but were really useful uses for AI. And I think that's a really rich place where we're going to see a lot of growth of clever uses for some of the new capabilities that have come out in the AI world. And so it's not surprising we ended up with a huge amount of material on AI assisted software deployment. In fact, the first three blips that we're going to talk about are directly related to that. And so is one of the other ones,
so I will turn it over now to Camilla to talk about some of the blips that led us to that theme. Thanks, Neil. Yeah. I think it comes without need to say what GitHub Copilot is. But
I'm going to try. So Copilot is a coding assistant. That's at least how we call it. Created from a collaboration between GitHub and Microsoft.
And it calls itself your AI pair programmer. And as very enthusiastic of pairing that we are at Alteryx, we think that is a very simplistic and even wrong idea of pair programming, right? Writing code is only one thing that you do when you're doing software development and definitely not the only thing that developers spend time on. So for us, in fact, code Copilot is a coding assistant, and you should use that to make pair programming better, not to actually replace pairing. I think, since our last Radar, we increased the quite a little bit on the number of teams that are using Copilot inside of Alteryx.
And it's helping them to write code faster. We are doing a lot of sharing on the learnings that we are having, and one of the things that stuck with me is-- prompt engineering is a whole kind of new skill, if you will, and yeah. It takes time to get it right. So some of the material that we put together actually have some tips on how to do it and how to take the best out of it. But if it's taking too long to get the outcome or the code that you would like by prompting, then perhaps you should give up on Copilot and go write the code yourself. Something that is new to Copilot this time is the chat interface. So GitHub Copilot Chat, which is available inside of the [? IDs. ?]
And it helps find the commonly used information around that code that's in focus and even what you can do with that. We do think that it is quite a powerful addition to GitHub Copilot inline assistant that it has been here for a little bit of while. The next two code assistants that we featured this addition of the Radar is Podium and Tabnine. So both are in Assess, and they do-- we do see them as quite promising, and they do address one of the biggest concerns that usually organizations have around coding assistants, which is the use of open source licenses and how the model is trained.
Both tools do not use or don't train their models with repos that have non permissive licenses. And also, they don't send code snippets to third party services. One thing that's worth highlighting is, both of them have free versions that you can use.
Tabnine has one paid offer, and it, of course, has more and better suggestions than the free one. And Tabnine can also give you the ability to use it in your local environment without internet, which is not the case for Podium. Tabnine is a much kind of-- it has been around since Copilot. Podium is a new one in the very busy space of code assistants, and therefore, Tabnine is a much more mature product. But we do believe that coding is very promising, and we should keep an eye on that.
- I don't know if you saw. There was a question asking whether for you guys that were both involved in putting the Radar together. Did Thoughtworks use any AI as part of that process or to generate anything for the addition? - Yeah. So we do translate the decorator to Chinese, Portuguese, and Spanish.
And in Brazil, to translate it to Brazilian Portuguese, we used one of the chatbot assistants to help with the translation. It does a pretty good job, actually, for a first version. So yeah, we did.
Now, Neil, you can go over and cover the open source LLMs for coding, which-- yeah. Got a good use cases. - Yes. So toward the question that came up before, we did not use it to do any of the writing besides translation on this Radar. We did take a stab at letting
one of the tools create a first draft for a theme in the last Radar, and then we ended up throwing that away and rewriting it from scratch because it wasn't terrific. And it had a few of the issues that you sometimes see in these generated stuff. And some issues that I'm going to talk about later in one of the related themes. So let's talk about open source LLMs for coding, which is another-- made it onto our Radar in Assess. Camilla was just talking about Copilot and those similar family of tools, but we're software developers. And we love digging underneath a level of abstraction and fiddling around with the parts and seeing how they work, and all that stuff. So we figured that--
and we got a lot of nominations for open source LLMs for coding because LLMs, of course, the large language models, are the things behind ChatGPT and, to a degree, Copilot, et cetera. But getting underneath those things can be interesting to experiment and play with. Of course, you can use the existing ones, bar, ChatGPT, et cetera, Open Llama.
But a lot of those models are proprietary and only can be used via subscription. They also have issues where, if you're sending proprietary information to them, you're basically sending it to an insecure place. And of course, you can do that with subscriptions and licenses, but that's complicated. The good news is there are a bunch of these open source LLMs you can use to customize for coding. And this is really applicable not only for the tinkerer amongst you, but what if you're in a highly regulated industry where you're not allowed to send proprietary information over a link to a subscription service like that? Or you're in a highly rarefied field, where language has a slightly different meaning like a medical or legal or something like that. Then there are a bunch of these open source LLMs that you can use to build your own ecosystem.
So there are models like Starcoder and Wizardcoder, which are trained by large data sets maintained by big code. And there's a-- Wizardcoder is a tune star coder model. We've actually used this in some experiments and found it really useful for generating structured engineering artifacts. Certainly source code, but there's a lot more applicability for non source code-- things that you still need syntax for like YAML or SQL for example. This is actually a really, I think, immediate great use for a lot of this family of tools. So let's say that you're a software developer, and you're not fantastic at SQL.
You can let these tools generate SQL for you, and you don't have to try to debug it and paste error messages in Google. And everybody knows what that cycle feels like. Having these tools generate some of that structured or semi-structured artifacts is really nice. And you can use these tools to customize the way you generate that code. So for specific downstream tasks like SQL generation for a specific database, you can fine tune these models so that they produce more applicable-- not just general and SQL, but more specific to that particular flavor of database. Recently, Meta unveiled a Code LlaMa, which is a code specialized version of LlaMa 2, which is a way to play with this and other of these open source LLMs for coding.
But-- and this is a topic that came up a lot during our conversations and all these AI related things. Where did the data come from? Who or what large language model are they parsing to be able to do their magic trick? So it's really important to look at the license and make sure the license, the code, and the data sets used to train this model. There's going to be a lot of sticky IP issues around training sets. There was a lot of regret from the commercial publishing world when they allowed Google just to open their doors and slurp up all their data so you could search it. But then,
it turns out if you can search it, you don't have to pay for it anymore. You're going to see a reaction to the sources for a lot of this intelligence, I think, they don't want the same thing to happen again. So we're going to see-- so be careful about license models, et cetera. But having said that, these are really nice tools to play with, particularly for generating any structured element that's not source code. Terraform, for example, is another one of the plugins existing for these. And it's great not to have to be a syntax level expert on all the different technologies we touch incidentally in the course of a day. And so using these
customized tools are really, really handy for that. All right. This is another one for me. This is actually a great example of good engineering practices that take on a whole new flavor when you add new, complicated things to the mix. So the blip here, which is in Assess, is dependency health checks to counter packaged hallucinations. Now, as I mentioned before, we try to get blips down to a nice tight paragraph.
And we also try to get titles down to something that's a lot less cumbersome than this. But in this particular case, we failed. And so this ended up being a title for this blip. Dependency health checks to counter packaged hallucinations. So what are we talking about here? So one of the topics that we've actually addressed multiple times on our radar is a bigger and bigger deal with organizations, which is software supply chain.
Everybody woke up to this because of the Log4j problem that came out recently. And a lot of business people went to technologists and said, how many tools are using this particular piece of software? And the technologists were like, beats us. And they said, that's not a good answer. We need to know how many of these things are using, because there's a problem with it. This is one of those things that, for some reason, a lot of development teams have turned a little bit of a naive blind eye toward, which is attack vectors that can happen through software development dependencies. Software development dependencies typically have more permissions than other tools because you need broader permissions. But that makes them a more attractive attack vector. Now,
with AI, there's a brand new attack vector that's possible and available here, which are packaged hallucinations. So if you end up using a bunch of these GenAI tools, they are trying to be very helpful. And they are so helpful sometimes that they become unhelpful.
Because if some really nefarious person realizes that this hallucination happens fairly often, then all they have to do is put an actual nefarious resource behind that hallucinated dependency. And all of a sudden, you have an attack vector that you just accidentally opened up because you were being too useful for your AI to allow you to do that. So the purpose of this theme is to basically broaden the normal, healthy dependency of software supply chain checks that you make using tools like a Snyk or a Dependabot, which is part of the GitHub ecosystem now. We talked about Snyk on-- and deps.dev on previous Radars.
These are all tools that help you scan and get a good handle on your software supply chain. You should add capabilities to that to check to see, is this a real package? Has someone hallucinated this, and does this resolve to an actual resource? So you may start thinking about validating through checksums or some other verification or extra authentication mechanism to make sure that you're not looking at a hallucinated package that someone has put an attack vector in its place. And suddenly, you've opened up something for an attack vector. So this is just-- more than anything, it's pointing out to the software development ecosystem. Hey, there's a new vulnerability that has become possible because of AI. And you should always remain diligent for your
engineering practices, particularly around sensitive things like security and those kinds of headaches. So that's the long, complicated title for this particular assessment. - Yeah. We need improvements on what Rebecca calls department of naming or something around that. Yeah. - Yeah we have-- so we pass things to the Department of Naming department when we're on the Radar. And this one failed.
- Yeah. So now, looking at other opportunities to reduce waste and friction with GenAI across the whole end to end product life cycle and not only focus on coding. When we do use UX platforms, we want to help organizations understand and improve their customer experience. So gain insight on customer needs, preferences, behaviors and act on and have access to segment analysis and things of that nature.
And knowing that Gen AI and LLMs, and things of that are really good at advanced pattern matching and synthesis, we oversee that this area can be particularly disrupted by those technologies. One tool that-- in fact, a platform that took the lead on this and is an incorporated LLMs gen AI, those techniques inside of it, is Kraftful. This platform is in Assess, and it basically describes itself as Copilot for product builders. So you can use this platform to plug your customer data and feedback.
So it can be like the mobile App Store that you use, and then it will analyze your data for you. Basically, it can identify features that your customers love, hate, any features requests that they may have. And to get a more details, you can even go to a chat interface just like ChatGPT or Bard and ask more questions and dig in.
And the good thing here is that-- and the benefit is that it's actually optimized for your own data. So it's not generic stuff. It is based on the data that you've provided. Something that actually calls out my attention on this is that, once you prioritize the user feedback that you want to address, you can ask Kraftful to write the user stories for you. And it gets the underlying data that it captured, and then it can write the context and even the acceptance criteria.
So we do find this quite handy from novice to very expert product managers and business analysts. Because you can use as an assistant to say, OK, am I forgetting scenario here? Or should I be looking at this or that? Right? So it is much more augmenting the work than, in fact, replacing the work. But it is quite powerful. Unfortunately, it is only in private data, so you need to provide your email address in order to have access and have a look at even the features that they provide. But we do see that this can be very helpful for those people working on products. And yeah, I think you should take advantage of it and have a look.
Now the topic of the-- theme of the hour. Let's say like that. So now we're going to go to very interesting topic. Definitely not new, but something that is getting more and more attention now as those gen AI tools get in place and how AI can actually help get developers more productive. Can they do that or not? So this is a topic that comes up once in a while, or pretty much every meeting, I'd say. Not because of productivity per se, but when we are talking about team cognitive load or 10 times engineers, which we put on hold some time ago, or we are pursuing on techniques like internal platform engineer as a way to improve the developer experience and things like that.
So we know that measuring productivity is not an easy task, and it should not be seen as one only aspect or perspective, let's say. It is a much, much more complex thing, and there is no actually output metric that will answer that how productive are your developers. Even though you can find lots of articles online promising you that. One thing is, we are glad that no one is actually trying to measure lines of code anymore as a sign of productivity.
Because in fact, we've learned over time that solutions with less codes are better than the ones with more code, right? So it is not actually pushing for the right behavior, and it can be pushing for undesired behaviors. And one thing that you should note and keep in mind is whatever you measure tells people how they should behave. It's going to drive behavior in your organization, so you should be very, very careful about what you're measuring. Otherwise, it can be very beneficial, but it can also hurt a lot your engineer organization. We are developers, and we are very smart.
I like to think that way, at least. And depending on what we are being measured, we certainly find ways to hack the game. Because this is going to say whether you're going to get a salary increase or not or a promotion or not, right? So you want to be well to the overall management managers.
At Thoughtworks, we do look at this as in the perspective of not measuring developer productivity per se. Much more looking at the waste that is in the system or the frictions that the developer face in their workflow. And I know, Vanessa, that you've been in quite a few assessments, looking at engineering effectiveness.
Can you give us some insight from the work that you're doing with some of our clients? - I have. That has been a recurring theme for this year so far. Yeah, I can share a little bit about my experiences. I think, as you're saying, Camilla, Thoughtworks approach is a lot less about measuring individual developers, right? We recognize that developers don't work alone when it comes to developing software. And so that approach that we take when we're doing assessments around engineering effectiveness-- we look at the value stream network.
We look for bottlenecks there. And what we're really trying to do is identify friction, hotspots, so that we can then go further and actually start to measure waste. And that's because, as you're sharing, we all know that it's very, very difficult to measure productivity in any direct or accurate way over time for all the reasons that you were sharing. However, measuring waste is straightforward. And if you work to eliminate waste from the value stream, you can actually effectively transform that effort into higher value developer activities and higher value work for the organization. So
what that means and what we're finding is-- we get asked a lot, what are the metrics I should use? Where should I start? And the reality is that it looks really different for every client. When we do this exercise, we find that, for some of them, the bottlenecks are very early on in the software delivery life cycle. Maybe around onboarding or discovering knowledge and knowledge management. For other clients, it may be really big bottlenecks in development testing and deploy, right? So we look, we find where those bottlenecks are, and then we look to identify that friction, that waste, and then set key indicators to drive to waste removal in those key areas. And that's the approach that we take.
Yeah, happy to take any more questions on that if it's helpful. But back to you. - Awesome. Yeah. One thing that I argue is that whether your
organization or team is trying to improve or want to see the benefit of AI assisted software development and things of that nature, is that, well, you need a baseline, right? So you start measuring. And to do that, we do use DevEx 360. It is a tool that is survey based, and it comes from well known researchers in the industry, such as Nicole Forsgren from Dora, and Marguerite in Story from Space, among other experts that we know. And what it does is, it captures information about what they call the three dimensions of developer experience, which is feedback loop, cognitive load, and flow state. And it gives you leading signals of developer productivity, developer experience by looking at friction, waste, and how is the day to day developer workflow.
One thing that it sets these two apart, and that's why we are featuring this specific tool in the Radar on trial, is that we've got much higher engagement rates using it. Over 90% of developers have given us responses to the survey with very detailed information and even suggestions on how to improve their workflow or what the internal platform could do, and things of that nature. And unlike other tools, the results of the survey are available for the whole organization. So let's say teams can have a look at their collective results. And they can look and see how they can go to a continuous improvement journey and improve their own productivity or developer experience by doing small tweaks on whatever they have control of rather than be waiting for org wide developer experience initiative that sometimes are very beneficial. But it may take some time to see the results.
We do use in our platform engineer teams this information from these surveys to inform the platform roadmap. So we do see engineering platform and internal platforms as a way to improve developer experience as-- it should be self-service, so on and so forth, things that we've been talking about for a long time now. But one thing to note-- and this is more like behind the scenes of the Tech Radar-- is oh, even though productivity as a whole is a theme for this edition, you only have one blip? This is weird. Yeah, but we did discuss quite a while during the meeting about productivity and what we should be careful about, and what's the actual advice that we need to provide to people. And in fact, the DevEx framework, DX framework was proposed as a blip for techniques. But a general principle of the decorator is-- usually, we do prefer to go with specific tools and specific advice rather than the generic technique or generic principles that you may not know how to apply.
So this is one case of that. Neil will provide some counter examples of what I'm doing just because AI assisted tools. But I'll hand over to you, Neil, to talk about the too complex to blips and things that did not make to the final edition of the Radar because they were way too complex. - One last thing about the previous topic about productivity, I have skin in this game as well. So as Camilla was saying, we've been talking about this forever. I actually wrote a book about this in 2008 called The Productive Programmer. Our chief scientist, Martin Fowler,
wrote on his blog about measuring productivity in 2012. So we've been obsessed with this idea about productivity forever. I was lucky in that my productive programmer book-- I didn't talk about concrete metrics, but I did talk about things like flow.
And I'm really glad to see that the modern tools are measuring actual useful things. I worked at a project of many, many years ago where the project manager was counting lines of code, and he thought he was clever. He was counting semicolons in Java files. The developers found out, so they started doing Ascii art with semicolons and comment sections. And hey, everybody was super productive, and nobody was writing any code. They were just doing Ascii art and semicolon. So this is--
it's good to see that we're now trying to do this in a serious way. And Camilla and I were talking about this, and it came up during the tab meeting. And it became a theme because one of the questions was, why now? And we think, maybe, that there's a more obsession about measuring this now because of the AI augmented software development.
Because a lot of companies are asking, well, I see developers fiddling with AI. Are they just playing? Is it actually making anything better? So I think that's part of the reason there's an interest in this right now, is because we have all these new shiny things. Are the shiny things making it better? Or are people just using that as a new way to waste time and shave yaks, and other distractions? So-- which brings us to a too complex to blip. This is deep in the jargon, the internal jargon we use in our Radar.
As we mentioned before, each one of our blips on our Radar has to be summarized in a small paragraph. That limitation on size actually comes from the ancient days of the Radar, when it was printed on a PDF and distributed. And so, they need to be small.
And we kept that aesthetic because you could write volumes about each one of these things. And we're trying to avoid doing that. And so there are a lot of things that come up during our conversation. There's a category for a blip called too complex to blip, which just means that there's no way we could capture all the nuance around this thing, even if it's a really good idea or really bad idea. A lot of things that we try to put on hold to tell people to avoid end up being too complex to blip because, well, you should hold in that situation, but in this-- and you can't write that in a paragraph, so we end up with this rich set of things that are too complex to blip. But that's always an interesting set of things because it's all the things that are too nuanced to summarize in a small paragraph. And of course,
there are a lot of things in our technology landscape that are like that. So let's talk about a couple of the things. This is very insider info for our Radar because these are things that did not make it onto our Radar. And this is part of the visualization I talked about.
One slice of it. We ended up having AI augmented software development as being too complex to blip, which you may find interesting because it ended up being a theme. And it was a theme. It was just too complicated to blip as a single blip because, how in the world could you summarize all of augmented-- or what we changed it to is AI assisted software development for the purposes of our theme. This is some of the examples.
So the black ones here that you see are ones that we discussed but did not ultimately make it on the Radar. The other ones that are colored made it in one place or another. I think Copilot was in Trial, and the others are in Assess.
But these are some of the things we talked about. So there's code Tabnine and Kraftful that Camilla just talked about. These are all tools that are used for AI assisted software developer. You see star code and wizard code are there. This goes back to this idea that our themes are based on our entire conversation, not just the things that ended up on our Radar.
Because in this case, we talked about a lot of these things. The next category of these were around hosting LLMs. And I was just mentioning LLMs and open source LLMs in just a second. These are the engines that run all of these tools on top of them. And it turns out we talked about all of these. And there are a bunch of them available. LlaMa is the
Meta. I believe OpenAI, of course, is the one that runs ChatGPT. There's-- Google has one that's behind the scenes for Google. That's the Vertex AI. Amazon has one, so there are a lot of these things around. LlaMa
CPP is one that's written and implemented in C plus plus. So there are lots of these hosting LLMs around, including open source ones that you can use to play with. As you dig into the abstractions around AI, these become more and more important, especially if you're building customized capabilities in one of these things.
And then finally, for our AI stuff, the last category of these were-- Camilla actually mentioned this before as we were talking about this. All of the new things that you end up doing now in the AI augmented world. So prompt engineering showed up as a technique, and we ended up-- this is something else that Camilla mentioned. I'll reiterate. We prefer blooping actual tools than just techniques. Because techniques are a little bit wishy-washy.
They're a little bit weak. We want-- when possible, we want to say not just this technique, but this tool does this technique really well. So prompt engineering came up as a technique, but we wanted to use very specific tools and actually blip those. So we ended up blooping a LangChain, Semantic Kernel, LlaMa, Index, GPTCache, et cetera, Gradio.
And putting some of these others in Trial like semi-structured natural language for LLM that allows you to customize for particular problem domains, legal, medical, et cetera. But the other fascinating category of tools here is around prompt engineering and tools like LangChain and Haystack, et cetera. Because part of expertise in using these AI tools is getting the prompt exactly right to get it to produce useful stuff. And it takes some tweaking, and it takes some experience to get these prompts right.
So this whole idea of prompt engineering is a brand new category of thing that we have to think about as software developers. And it's a great example of-- if I'd said prompt engineering to you two years ago, you'd have no idea what I was talking about. And yet, now, we have a bunch of things on our Radar that are designed to help you do exactly that. So we ended up not creating a single blip for AI augmented software development because there was just way too much of it. But it became a theme, and it became 20 something. Marissa's on this in the chat. She can tell us, but I think it was 23 or 25 different AI related things.
And we talked about way more of them. These are just the ones that managed to make it through the filtering process to make it under our Radar. So let's talk about another thing that is too complex to blip. And there's a great story that goes along with this one because it shows exactly how this happens a lot in our meetings. So the idea of inner source is using the open source model of pull requests, et cetera, but for internal development for an organization or an enterprise. In other words,
model your software development ecosystem like open source projects like Linux and other projects are managed with pull requests and merges and patches, et cetera. And all that ecosystem. And it's a great example of-- as soon as something like this comes up, there are a lot of green cards that go up.
So when we're doing this meeting, either in person or virtually, we have a green card, which means I agree, a red card means I disagree, and a yellow card means I want to talk about it. And a lot of these too complex to blip things-- initially, a green card goes up because, hey, that sounds like a really good idea. Use a-- Camilla's got some of them right there in front of her. So we-- and so we-- hey, that's a really good idea. Using some of the principles of to manage and get a discreet and manage your software development, your source code ecosystem within an enterprise. And then a few yellows will start showing up for people who have used this, but it's not universally good.
And this is a great example of one of those things that works really well if you're an extremely well disciplined team and a really well disciplined organization. If you're not those things, then you can use it to create a whole bunch of real big messes. One of the people in our meeting said, there are a few cases this could work. But in general, if you want to share something, actually make it open source.
Because the problem you run in with inner source is, it becomes a dumping ground because nobody really cares, and there's no sense of ownership and pride. So without clear ownership and responsibility, it does just become this huge dumping ground. Somebody else in the meeting said, it's hard for me to imagine a less efficient way to deliver software within a company because you don't have ownership around things. And the cost of collaboration ends up being really expensive, and it's hard to build and figure out how all that stuff works. And so while at its core, it's a great idea, hey, there's this open source principle out in the world. And we could use that inside our company.
Unless it's done really precisely-- and in fact, you'll notice that the same precision has to be done internally that makes open source work in the real world. Very small discreet check ins, very focused on general purpose, not specific purpose things. And so unless you can replicate that model and that discipline and granularity in your ecosystem, it will probably end up being some headaches. And so over time, what happens is, the green cards start flipping to red cards. But then, more arguments for it come in, and then they finally flip over for too complex to flip. And it becomes that's the overwhelming vote, and so it ends up on the cutting room floor of our Radar as it were.
It's an idea that has some interesting potential if it's done well, but there's no way you could write all the context and the edge cases into a single blip. And so it ends up not being on our Radar. Some of these end up being as articles on insights, et cetera. But a fair number of fairly nuanced things end up on our Radar cutting room floor. - Neil, I came across a couple examples where people are using inner source for their internal platforms. And in fact, the outer team topologies were ranting a little bit about that, saying do not use inner source as a solution to your under investment in internal platforms.
So I think, perhaps, we have something to talk about in the next meeting, which is how it is not good or efficient to use inner source instead of focusing and investing the right effort in internal platforms. I thought that was interesting. Wanted to share. - Yeah. I also see it come up a lot in that space, where there's a feeling that platform engineering is a bottleneck. And so how do you address that? But yeah, I've also-- just echo what Neil shared as well. It always seems like-- sometimes, our clients have tried this, and it's been problematic and left a bad taste in the mouth.
And we tried it. It didn't work. And so I think Neil's advice is very timely. It needs to be approached really thoughtfully and carefully, perhaps in target areas. Get the model right, prove it out, and be thoughtful before scaling. Also, incentive models are really important. I know I've heard from some clients where there's a lot of effort being put in to contribute, but it's not being recognized, right? As part of incentives and performance management, and things like that.
And that causes a whole bunch of problems, too, so it definitely needs to be thought out really, really well from many dimensions. Yeah. All right. I think with that, we're at the end of our sneak peak. And so we would welcome more Q&A from the audience since we've got a little bit more time. We did have one in the Q&A function, which, as Eugene says, a little bit off topic. Well,
not really off topic. It's about putting together a Tech Radar for your organization. I don't know, Neil or Camilla, if you have any thoughts on Eugene's question here, but he was asking any recommendations when merging tech Radars between child organizations, how to approach that, in terms of organization process or resolving conflicts, anything like that.
Sounds like Eugene might be going through that at the moment within his organization. Any experience with that from [INAUDIBLE]? - Well, it seems like the problem to be solved is less about the actual emerge of the tech Radars, but more about getting consensus or if not a consensus, but at least a path forward on what to do. Well, we have two options.
Which one should be the one go-to option now that we are together? So definitely getting the right people in the room to discuss that and come up with, if not the final guideline, at least something to go with and try and reassess that later. One thing that I find very useful is knowing the use cases where you apply certain tools or techniques because they might be different. And in this case, it makes sense to have different ones to do the same thing, but with different use cases. So I'd say those are more of principles to, perhaps, keep in mind when doing this merging. But I think Neil have done quite a few workshops to put the decorator together for some of our clients.
So he perhaps have more actionable advice. - It sounds like you're trying to do a build your own Radar and do smaller versions and merge it into a bigger version. If that's the case, that certainly works, but it's a mistake to think that the artifacts you create when you build your own Radar is the most important thing. The most important thing is actually the cross-cutting conversation. So if you're going to do that, don't have all the DBAs creative Radar and all the developers create a Radar and all the BAs create a Radar. Because all you're going to get is their bubbles.
What you want is three groups with a huge mix of developers and BAs and project managers, et cetera, so that you get that cross collaboration conversation. And then that, you can merge into a single thing and basically just do an aggregation of all the conversations that came up. And what you'll find, and at least in our experience when you do that, is really, the touch points where the groups disagree.
Because 80% of them will overlap and say, Oh, we agree with this. And then 20% of them will be, well, this side thinks this, and this thing-- and so digging into that. But the real benefit of the build your own Radar exercise is the conversations that it spawns, and the artifact is an accidental side effect.
So you want the rich conversation as much as possible during the process. - Great call out. Thank you, Neil. Thank you, Camilla. And Camilla is looking to respond to, I think, Hardy's question in the chat. Is that right, Camilla?
- Yeah. So I'm not really-- I'm not really sure about what is exactly the topic that he is addressing here. Is it about [INAUDIBLE] in that context? Or-- Yeah. I didn't quite get it. - I was wondering that too. Yeah.
Hardy could you add a little bit more color to your question? I wasn't sure if you were referring to pull requests in the context of the InnerSource model or something else. Just let us know a little bit more. Add a bit more color to what context you were talking about there, in regards to code versioning and we'll revise-- - I can think of at least three different ways to do it or two different ways that are not pull requests that are common. Feature branching and trunk based development using feature toggles or something like that. - I was waiting-- I was waiting. I was waiting for one of us.
[INTERPOSING VOICES] - What you'd would hear from a Thoughtworker. - I didn't advocate for it. I was just asked for if there were options. So I'm definitely in the trunk based development side of the world. But yeah. - Yeah.
With trunk based development is one of our Thoughtworks sensible default practices. So you'll hear that a lot. - And pull requests are feature branching. I mean, it very much is because you're working in isolation and then pulling something in. That's why it only works if it's tiny and discrete. Because you don't want to merge nightmare. So--
- Exactly. OK, and-- - I think this topic will give a whole other webinar to talk about it. So let's not get started. - So absolutely. Monorepo versus non Monorepo comes up
every single Radar, and it ends up too complex to blip every single time, so-- - Absolutely. OK, we're a little bit out of time. Thank you for keeping those questions coming. Maybe Neil and Camilla can try to answer them in the chat if they have a minute. Will do their best. I'm just really like to thank Neil and Camilla for speaking today and joining us, and for all of our participants. It was so cool to see you all dialing in from all across the globe to join us today. So thank you so much.
We really appreciated it. And folks were asking, oh, where can I get the content from today? Well, as we said, this was a sneak peek, right? And so the full Radar edition will be published shortly. You'll be able to download it and go over all the details. So please keep an eye out for that. And also,
this video will be uploaded to YouTube, and so you'll be able to replay if you're interested there as well and find it there. Thank you so much, everyone. Hope to see you next time. time.
2023-10-03 02:29