Plunet Summit 2023: The future of technology in language industry
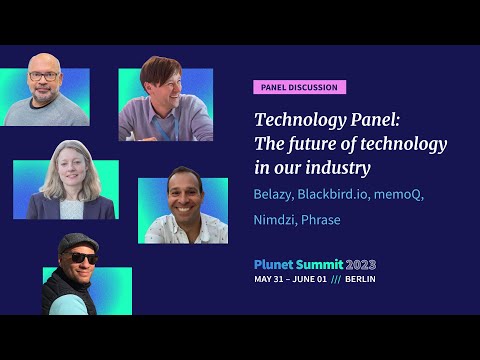
Okay, let's start with a quick round of introduction. Please let us know who you are, what your company is, where you're located, and just one word. Are you excited or scared about the future of technology in our industry? Josef, give you the word.
Good morning, I'm Josef. I'm Josef Kubovsky. I'm from Nimdzi Insights, and thank you for allowing me to be part of the panel, because it's a conversation that we're having every day, whether it's with translation companies, technology providers, or the localization teams. Scared or excited? Combo. A combo? Okay. Istvan, who are you? Where are you located? Good morning. It works right? Good morning. So my name is Istvan and I am located in near Barcelona, generally.
And I'm working for BeLazy, but I think that was already announced. And like when it comes to the future of technology, I'm getting philosophical about it. So like I don't know if I'm scared, depressed or excited about it because it's all at one.
And like we can't even find out that we just live in the matrix. Like that is like more reasonable than ever before. And I'm waiting for the moment that our creators will find out the same time, the singularity when we find it out.
Okay. Wow. That went philosophical quite early in this. Simone, how about you? Hi, everyone. Thank you for allowing me to be on this panel today. So my name is Simone. I'm the chief product officer at Phrase.
I think most of you are familiar with us. We're a localization product suite. Some of you are our users. I'm new to this industry.
So this is my first caveat, right? I've just joined in February. So I'm still learning a lot about all of you guys and your pain points. So I'm trying my best today. My background is in NLP.
I used to be the head of product of an NLP platform in banking in FinTech. And I'm super thrilled and excited about the future because it's all about AI. And that's what I'm here for. Great. So we have one excited. Let's see. One relaxed person here. I'm Florian Sachse.
I'm working for memoQ. I'm not a co-CEO or something. No, sorry. I'm just the chief evangelist. But maybe also not that bad. I'm working from Bonn. Most of the people and the company is in Budapest.
I think you all know us, so I don't need to talk about the company. I would say that I'm frightened and relaxed. So basically you can be both. And let's discussion go on. Frightened and relaxed. Also, sorry that I promoted you. Didn't mean to. It's okay.
I can sense that there's a lot of mixed feelings. Bruno, how about you? Hi, Sophie. Hello, everyone. Pleasure to be here. Thank you for the invitation. My name is Bruno Bitter. I'm the co-founder and CEO of a young technology startup called Blackbird.io. We're active in the workflow, orchestration, automation and integration space. I'm a Hungarian born in Budapest.
I've been living in Canada, Toronto for the past four years. My team is fully remote and global. We have people in Bulgaria, Netherlands, Ukraine, United States. We were founded in the middle of the pandemic, so it was a question, and this is the way to work. I'm very excited, but I have to acknowledge that I have been always excited about new technologies. I was excited when I was nine and the Walkman came out. I was excited when PlayStation One came out and I was excited when Netscape came out.
But now I'm old enough to have some regrets about all these early adopter-type things. My first company was very involved in social media when it was a new thing. In similar panels, I said that I'm super excited in 2008. But now looking back and reading what some really smart people have reflected on, like Hariri, the historian.
Some of these people described social media as the first wave of AI. AI versus humans was 1 to 0. We lost that battle. We didn't really come out top. Our society is not really better.
So I want to tread carefully. I'm excited, but I think we have huge responsibility in how we shape AI, and we should be more like Florian, calm and anxious. Frightened and relaxed, I think. I think they're relaxed. So, new hashtag. Thank you so much. Thank you. So, yeah, you can already tell from just the introductions that this topic is very current and very controversial. So I want to make it even more controversial and start with you, Florian.
And the question is, what is your opinion on the future of CAT? Computer Aided Translation. I know all of the CAT tool providers rebranded to TMS for multiple reasons. We also now have MT, and we don't really hear the word CAT as much anymore. Is CAT dead? What is your opinion on that? What is the future when it comes to that term and technology? Okay, so we just had rebranding, right? You also had rebranding, right? Our rebranding was about making sure that we are not recognized as a CAT tool. So CAT is basically the part of the solution which is used by translators.
The equally important, let's say like this, the equally important part is translation management, supporting the translation supply chain. And in that sense, I would like to use more the term TMS. I think you also like the term TMS more than CAT tool. 100%! Look! Who knows what will develop out of that? And whatever happens in technology, so as long as there will be translation supply chains, you need translation management systems. And our focus as memoQ is to support companies who are doing, we call it premium translation, so this is a fuzzy year. Whatever we use is a little fuzzy year.
But basically it means the focus is on companies needing humans in the loop. If it is translators, if it is project managers, whatever. But it means it's not fully automated. And we have cases where the actual supply chain is quite complex and this needs also quite powerful tools. And this will stay. So whatever will happen, we will have these use cases where at least for sure in regulated industries where brand is a little more complicated than checking if we use the right terms. So many cases where we have obligations, regulated industries.
And what is also important for us is that we focus on repeatability and predictability of workflows, also of translations. For that, for example, you will also need in the future for sure TMs. So without TMs, what do you want to feed into MT systems? And if you actually want to have predictability in your workflows, the main source of predictability are TMs. So we are looking more, and this is why we do not invest ourselves in MT.
We have tons of partners who use MT, and I think that the solid foundation, the solid backbone we can provide will stay relevant. So long answer. I have a short answer as well, can I? Yeah, if somebody hands you a microphone. So the translation memory, no matter how you call it, is still and will for at least for a short time be the cheapest way of processing our type of job.
So I'm seeing a chance for it to stay. I would have also summarized, and you can keep the microphone because you will get the next question, but I would have also summarized that the term CAT is definitely dead. Translation memories are not dead though. Well, you can say something about that next. Okay, hearing that, though, I think that's a big change, right? I mean, we have used that term for a really long time, and I mean, I don't want to pronounce it dead today.
I don't think I have the authority to do that, but with that in mind, Josef, I mean, you talk to so many people, to so many companies in your research, what would be your advice to project managers and translators, what to do to stay relevant in the future with all of the tools and changes that are coming up? Okay, thanks. Great question. I'll put it on a little higher level, and I'll divide it into two. I'll try to be faster than Florian. So first thing, looking at the enterprise customers, whenever we're consulting the localization departments and the way that they're testing out things, it's mainly when they play with their own data.
They're not willing to play around with the company data because they're afraid of potentially putting themselves in a risk of not being allowed to. But then if we go and we do the conversations with the other stakeholders that are somehow related to the localization departments, we see that they're testing these features as well. So I would say what you really need to see internally is to run around the different stakeholders that you're communicating and make sure that they understand that you are the ones who should be leading the conversation about what are the next steps, what are the next trends in using the latest trends, especially the large language models. The second thing for the translation companies, if you are not using, I would even say not machine translation is definitely something you must use.
And the second thing for large language models, don't just play with it, put it in an action. I've seen so many great scenarios of amazing benefits of using it. Talk about it. The customers are interested. Now is the time. Now is the moment for our industry to step up because everyone is interested in talking about this topic, hearing about the content, hearing about their appearance on different areas. Now is the time. Thank you. Thank you. That was for the higher level. Did you also?
No, I already did both. Did I miss the translators? Oh, the translators. Sorry, I forgot the translators. How many translators do we have here? I was like, did I black out for a second? The translators were not mentioned. Yeah, what about the translators? What were your messages for them? The answer there is there's a lot of development. There's a lot of new things coming out. Don't wait. Try it out. See how it works. Be ready. The focus, as we're seeing in any technology development, is simplicity, the intuitiveness of the technology that's coming out for us to be using it in a simple way.
But you need to understand the meaning behind it. So, do not wait, implement, try to be aware of what's coming out, to be able to use it when the time comes. Thank you. You can give it to Simona, that's perfect. And as Daniel said, spoon by spoon, yesterday.
I feel like that's such a beautiful picture and kind of applies to everything one step at a time. I think very often when people do not use any tools at all, it seems like so crazy and scary especially if you don't understand, but just go little by little, just like with automation. I think that's a very good advice. Simone, you and your position at Phrase, what do you think, what is the impact of AI on your product? What do you see in the future when it comes to all of these topics, TM, CAT, machine translation? Yeah, that's a very loaded question.
Very loaded. But I think to answer it, I'd like to depict how I see the future actually playing out. So bear with me for a couple of minutes. So I think if we think about the future, we cannot ignore large language models. They've been around for a long time, but we had some breakthroughs with ChatGPT. Most of you will be playing with that personally, which is fantastic, keep on doing that. But I think what it really means for our end customers, the enterprise customers, that we'll see a new proliferation of content.
In the last couple of years, we had seen a lot of new content through digitization, blog posts, everyone can put something on the web, right? But this will go in hyperdrive right now. This will go on steroids. What that means is that our enterprise customers will see a proliferation of content that's generated through LLMs, but it's also hyperpersonalized.
So if we all go on the same website, we'll see very different personalized messages for all of us. That's incredible. That's amazing. But it's also deeply scary for enterprise customers, because to manage that proliferation of content, they still need to make sure that you have your tone of voice, that's high-stakes content on a website.
It's a fight for other customers. You need to get the message right, and often you're also a regulated entity. So if you're getting that wrong, you have a huge problem.
And that's the underlying problem that in machine learning is still around and is not going away, and where I see massive opportunity for the people in this room. So machine learning is probabilistic, so our large language models, what does that mean? I'm not going to bore you with additional detail. It's statistical regression all the way, and it means sometimes if you use ChatGPT, you should use the same query and question and run it twice.
And if you do that, you will get two different answers, and you will get two different summaries. And for me, that's okay, because I use it at home. I ask stupid questions like, hey, I have a new wooden floor, it has an oil stain, how do I get rid of it? And one day, ChatGPT tells me, sand the floor, and the next day, it doesn't tell me that. And that's okay, because it's low stakes. But for an enterprise customer, that's really scary, and I think the quality assurance, the quality control will become ever more important.
The second piece that will become more important is that we will see, a proliferation of use cases, which require configuration, stitching together new systems. And that is a really, really critical piece that our enterprise customers need to get. So beyond the proliferation of use cases, we also see a massive complexity in machine learning tools. So to Josef's point, an LLM is a really expensive way to solve a problem. So in the end of the day, you have a huge pool of data that's being fed into an algorithm that needs to deliver an answer. LLMs are huge if you want to get it precise and reliable, which is what enterprise customers want.
You need to feed it more data, you need to be more precise in your prompts, then the underlying model changes and you don't know why. You can't debug it. It's a super expensive way of solving a problem. There are different ML approaches that can do much better, much cheaper. And I think the future means we need to become advisors and use the domain expertise that we have to understand what data do you need to use, for what use case, and what machine learning to get to the results you want, and how do we ensure the quality is right. And so at Phrase, what we're doing is we're positioning ourselves for that future in different elements. So in the past, that was before me already, we've doubled down on machine translation, so what we're doing is we are agnostic to what ML approach we give our customers.
What we do is we take best in market, we make it future-proof and reliable, and put guard wheels around it to make it really solid for enterprise customers and yourselves. But we also put the workflows around it so you can stitch it together with your internal systems and do what you need to do, right? And we're continuing to double down on that journey. So I think the future is great, it will be hyper-personalized , but it will be difficult to manage, and it will require a lot of domain expertise which sits in this room. Wow. I need a moment to formulate the answer, because... What you said was basically you predict that in the future, which I never thought about, but I think you're right, you go to a website and the website knows this is Sophie.
Sophie is in her late 20s. Yes, lives in New York, loves to go to happy hours and has very liberal political views, so the website is tailoring to my persona, like my social media feed is. Actually not, because what you just said is generalizing, so it would know much more about you. It would not combine you with the other people who are 20s, it would personalize. Can you say that in the microphone, please?
Okay, so what Josef just said is, if you didn't hear, that it's even more personalized. So basically it's not the persona of the young woman in New York, it's personalized to me as a person, to actual Sophie. Okay, so you go a step further. Who in this room hears this for the first time? Just me? Oh, shit. Okay, maybe somebody else should moderate the panel on the future of technology next time around.
I guess let's go to the next person here, Istvan. What are your thoughts? What do you think is the biggest challenge of our industry, or what you are doing, what you are seeing with your customers? What is your take? What's interesting is that this industry is a very small industry. At the same time, I mean, most industries are small, so this is no exception. But there is a very large number of interconnected systems because of the supply chain aspect, which is very special about this industry.
The AI training is something similar, but I have yet to find something which is also dissimilar. And because of the supply chain being composed of independent contractors, there are a lot of cogwheels that systems cannot really solve very easily. And there is an approach that is happening that the top level is controlling everything, and the bottom level they don't have access to. So they don't have the information at the moment to be able to control everything on the bottom level because there is like several middleman, like removing them from there.
Think about translator availability. That is like a typical problem. Who knows the translator availability to translator? Nobody else knows whether you will be able to do this. And this is the scaling part.
You can only scale if within a certain amount of time you have enough people at the right level being able to work on your jobs. And then comes like the multitude of different systems, the multitude of training. And for a lot of these atomic, like Sufian was yesterday talking about the atomic levels of like the applications are becoming smaller and smaller. But the thing is that they are having connections to very large systems, very complex systems which are somewhat different. And there is this standardization. I mean we are we are in this game like trying to standardize those very simple steps that a lot of companies need to do because you need to assign a vendor, you need to take a job, you need to create an invoice, you need to deliver something.
I mean there are a couple of these small tasks that are implemented very differently in each and every tool in our industry. And just from our industry's perspective and I'm not talking here about the enterprise perspective which is obviously very very different but here I think a lot of you are language service providers. This kind of working in multiple tools has created a lot of learning necessity. So a lot of people are using these tools on a sub-par level because there is no embodied knowledge there.
They are just using the basic functionality and I think that getting these cog wheels like a little bit oiled is something that that I see is necessary in order for us to establish the efficiencies. And those are the efficiencies that can later bring in things like really efficient AI-based learning and moving forward. So there is this maturity that is always going within the industry in order to serve a higher being if you like. Thank you so much. How would you rate the maturity level where we are today on your scale? I think it always depends on which reality are we talking about because the thing is that generally yes I could say like oh that's terrible but there are some really well-oiled machines in this industry.
And I think it's also interesting that maturity is something that enterprises go through together with their vendors, translators go through as well and they go through separately. And finding the right match at the right time is sometimes very hard because there is a lack of communication, there is a lack of standardization, there is a lack of I don't really know how I should be communicating to those people who are to level up from me. So that is like I think there are some very very good workflows already but they are just not so often there. Yeah and of course yeah to make things integratable it's important to kind of standardize how people are working because if everybody is highly individualized it's very hard to do those connections. On the other hand everybody also needs to individualize to stay competitive
so that's definitely one of those challenges that we see. That you probably also see Bruno. I don't know what your take is on yeah the impact of AI and also the need for integration. Yeah what's your opinion? Also can you please talk about what you did with my little Google Translate? The cool cyclist on the Rhine but yeah maybe the question first or maybe it's related we can start with that it's actually fun. So, we were all here during the keynote and Sophie shared a fun anecdote about a machine translation going wrong right we laughed about the Radler and the cyclist and the beer relationship going away and that actually with Florian as we were walking towards the boat we opened our open AI application our phone and asked open AI. Well first we looked at DeepL and was able to confirm that the problem still persists and then we checked what open AI ChatGPT has to say about it and using the GPT-4 model we asked to do a post edit and review and change it and it was able to do that and a few hours later around midnight in the bar with my colleague Matais we quickly built a whole workflow orchestration where we put that original German text it to Zendesk and built a trigger went into DeepL went into open AI went back into Zendesk.
So we live in interesting times and we can be both excited and scared about these things but to answer the bigger question two parts but quickly first to assess the breadth of the challenge I want to refer to G2, G2.com formerly known as G2 crowd you probably all have used it. It's a review site for B2B software so it doesn't include B2C software like Nike run or Spotify and today it has over 2000 categories of software and almost 150000 software applications. So while it's evident and clear to everyone today in the industry that we need to connect better and automate better and orchestrate better when it's almost an infinite number of applications where do you start and how do you tackle them so our Answer to this on a strategic level at Blackbird is to really build a platform, so not just build connectors, but offer an SDK that enables us, but also any of our users, to build anything they need. Because if it's only us building things, then there will always be a bottleneck, because the number of applications will proliferate faster, even just if we zoom into one category in the 2000, just large language models, that proliferates faster than anyone's ability to really churn out those connectors.
So we need to do it in a distributed way, where we offer a platform, we offer an SDK, and we can do it in a networked way, where every user can contribute. It also means that when we build connectors, they are expendable, so we always continuously build new and new versions, and any of our users can pick it back on our public app, so they can essentially clone it, take what we have, and build on top of it, and add what they need. So we believe that this could be a flexible model to resolve this question. And the second part of my answer is what to focus on, and in this regard we like to be customer centric, so it's not what we want to focus on, what our partners want to focus on. And there we see a very interesting maturity journey, or customer journey, smaller companies like to focus on the chaos of getting too many emails, and they want to automate that, then SLVs want to automate the supply chain between the MLV and SLVs, and larger companies want to connect directly to content management systems, and then with the Nimdzi 100, crowd and enterprises, we start to get very complex, very sophisticated questions. We see some readiness to get into large language models, to chain it to other AI models, but also somehow chain it to the traditional supply chains of the industry.
So you do see a big difference in the questions coming from the larger Nimdzi 100 LSPs. Are they similar to what enterprises are asking for versus LSPs, in your opinion? Very much so. So much so that I often get the sense that it's not even the same industry.
Classic business question, people at MBA learn, you know, to answer what business are we in and I seem to hear very different answers to that when I speak with small language providers and enterprises or large language. For the smaller companies it's still about the linguists, the love of language, the joy of communication and everything beyond that is some pain point that that is annoying and would be great to go back a couple of years or decades and not have that distraction. So at the heart of it is still the linguist and the joy of speaking. So they're in the business of language and communication. With large companies I see this shift from Just talent, yeah, talent is there, but it's increasingly more about technology and processes and data and the ability to have that. So the large companies are increasingly in the business of technology consulting, workflow consulting, being partners not just for language operations, but data and content operations, becoming advisors, that's where the added value opportunities are, and I think that's where increasingly the margin will be coming from.
And the key people in those organizations, the heroes in those organizations, are not the translators, in my opinion, but the solution architects. So we have different roles in the center of it, and they ask different questions and want to resolve different problems, and they might not even speak multiple languages, right? Yeah, two industries. That's super interesting. The question is, of course, can you have both, right? Can you have the best of both worlds? Can you still be linguist-centric and have the love for languages and the joy of translation and still become that super technology-enabled language service provider? Some people are smiling, and they're just like, yeah, that's me. Others are looking at me quite shocked. Josef, I want to look at you here, because you also have a lot of data, of course, speaking to so many companies.
Do you confirm this notion of the smaller providers and the larger ones in the enterprises that do very different things? Do you think the smaller LSPs, I mean, we have many here as well, do you think they need to look into becoming more technology-focused, or do you think there's a market for both and a world where we can have both of those providers? So thank you. I'll be a little bit controversial. And I'm going to say that the advantage of the large players, and Nimdzi 100, as I've already mentioned here, is of course they have the finance in the background, right? So they can play around, they can invest into what things can be done. We've talked with Anna earlier about, yes, but there's still going to be customers that they are not going to be interested, but through the automation, through the different integrations, they can actually support and find ways how to close also those small clients that Most of you here are working with today. So there's definitely the necessary and I'm saying again, but I still feel the importance for you guys to play around with these tools and not just play, but start implementing it. One of the cases that I say for all of these examples is that custom MT, the engine being built for your type of customers, and then using ChatGPT-4 and later higher for doing the final post editing has better quality results than a human post editing. If you have a high quality machine translation, and then you put it in the ChatGPT, it has a higher quality results than post-editing done by a human, okay? So, keep that in mind, go out, try it out, play with it, and put it into practice. The source of that,
can we? The source of that state. The source. I have an American example. Okay, we have two answers from the panel, I just want to say I really appreciate that you said the controversial thing and then said it again. No, Simone, I want to hear your answer.
No, just the data point, and that is unfortunately an example for my old industry that I came from. So, in my old company, we automated extraction of data from documents. You have a negotiated contract, you need to understand is there a clause in there, yes or no. And we had a very demanding customer who used us to run a regulatory compliance exercise. And what they did, in true nature of who they are, they took the machine, and then they ran through all the documents, and they took two of their in-house lawyers and had a four-eye review of every single document.
And they realized that the machine was more correct than the lawyers, because we humans just aren't very good at repetitive tasks. We're really great, our brains are really great at outliers, and anything that requires complex thinking, we're super poor when it comes to repetitive tasks, and that's where the machines will always beat us. So translate the same text the same way twice. That's also something similar. Yeah, I think, first of all, I do agree. Humans, that's also when we look at what we wanna do at Plunet, right? Project management by exception, because humans are great for crisis.
Humans are great for exceptions. If things go wrong, you definitely do not want a chat bot to solve that issue. I was always telling the story how I once tried to straighten my teeth.
And it was one of those smile direct companies during the pandemic that sent you everything no doctor needed, and it only had a chat bot. And when things didn't work well, it led me to actually cancel that service. And it almost became a lawsuit, because the chat bot was great for, I wanna update my address, I have a standard problem. But the moment things were getting really hard, I realized, yeah, a human would have been able to solve this, and they would still have a happy customer, the chat bot was not.
So I feel like, yeah, repetitive task, the machines will always be, will be better going forward. But in crisis mode, you still want a human. But I know, Florian, you wanted to say something little early already. I want to reflect a detail on our industry, and if this are actually two industries or not, and the larger are different than the smaller ones.
I must say that I actually do not know much about the smaller ones. But the smaller ones are making 85% of the business in this industry. So they must do things right, still at the moment. And if you think about the distribution of revenue between the top 100 and the rest, this is a quite antifragile system. So I think we are well positioned to survive. And this is why I'm relaxed about AI.
And if you start to think about this as not, these are the top 100, and these are the 19.900, then, and you see this as an ecosystem, then there's room for also just the linguists. And also for just the companies who work on linguists. They do it already for 10 or 20 or 30 years. They most likely will also do it for 10 or 20 more years. But it would be, and this is where I completely agree, it would be stupid to not look into opportunities, new technologies delivered to our industries, also for the small ones.
But I think that the picture of disruption, so there's a slight notion of GPT will disrupt our industry. I don't believe that this will disrupt our industry. There will be room for everyone, but if we are a little smart and use technology in a way that it fits to our values we have as company owners, I think we are fine. Yeah, it's a little different than... Yeah, I wanna comment on that if I can.
You can, sorry. I think this industry will be fine, but I think the role of everyone in this room will change. So for those of you who are skeptic of LLMs, and by the way, there's OpenAI, like the company running ChatGPT is a true misnomer because they're not open anymore, right? They've been taken private and that should all give us pause for thought because it's not gonna be easy to use that. The role of each of all and one of us will change in this world in terms of how do we use machine learning? It's not happening.
If you want to understand what happens now end customers, I'd recommend you read a report like Databricks just came out today, use of AI, they are data lakes. So the first thing you need to do as an enterprise customer to automate is get all your data in one place. So there are many data lakes you can buy, Databricks is just one of them. They ran a study and showed statistically how many of their customers are playing with LLMs, right? So I think this is coming. It's not gonna disrupt this industry so it's not needed anymore.
To the opposite, like the expertise of what language means and how you get it to a certain quality outcome is more important than ever, but the way it's being delivered will change, right? That's, I think, my statement. It's not gonna be disrupted. It doesn't disagree. So I think you come from a different perspective but we come to the same conclusion, I think. Great. I also already feel a little more positive about everything through this conversation. Do you want to comment on what was it? I still owe Hank an answer. So I'm not gonna give you the data point now but at the end of this month because the first day of the month we will have a new report about the different ways how the different ways how ChatGPT or the large language models are coming out and you will find the different solutions including the data in there.
Yeah, my next question goes to Istvan, and now, I mean, we talked about, which wasn't the plan at all, but the difference between the large LSPs and the smaller ones. So I think that's super, super interesting. But also, the large LSPs very often work with the smaller ones. So it's not one against the other, they're not competitors, they're partners. So would you, what is your take on that? I mean, you connect TMSs with TMSs exactly for that reason. So do you think there is an ecosystem rather than a competition between the big ones and the small ones? Yes, there is, I mean, there is absolutely an ecosystem because, so there is the MLVs and the SLVs, nobody identifies themselves as an SLV.
It's a very bad sales pitch when you are advertising yourself as that, and it's also your valuation when you get into an M&A that is going to have an impact on. But on the other hand, there are companies where the majority of the revenue is coming from delivering a couple of language pairs to larger translation providers. And this is a choice. The thing is that this is not the only differentiator between language companies, there are many others. But there is this access to the local talent, which is something that MLVs struggle to find. They struggle to find it because, obviously, I come from Hungary originally, and there is a portal for translators.
You get people, about a third of the rates there, than you would get on pros.com. And people like to work with people they get some kind of connection with, and I think that this is in our industry very much, so it's the same thing with the clients buying from these smaller providers than the vendors being able to work with smaller LSPs. And because of this scalability possibility, the resilience is built by having multiple LSPs, because it's easier to manage LSPs just because of the number of the LSPs you are working with than translators.
And how and when this is going to be changing is something which is currently unknown. Because what you want is like a compliance with the process from the beginning to the end, which I'm not sure how much it's happening today, actually. And you want scalability, which is like if you want to throw 50% more to the same supply chain, it should not collapse. And this is a problem that can be solved with mathematical models very easily, but when it comes to people and when it comes to habits and when it comes to multiple systems and tracking and capabilities, there is a very complex puzzle there. Also, did we just have the word SLV die as well? Is it the second one we're pronouncing dead today? There's no single language vendor anymore. I agree. When I started in the industry like a decade ago, there was still such thing as a single language vendor in SLV,
but I really do think you're right. Nobody identifies as that anymore. And maybe, okay, I do agree. And I know that you're very specialized.
The question is, is it more of like, are you like a local language provider? It's like the locale, right? You were saying that the access to local talent is actually the important part rather than that you focus on a single language. I have two comments to that, and that is that the large LSPs, thanks to the discussions regarding integration, regarding the latest developments, possibilities, Facebook, Amazon, all of those are looking for single language vendors. They don't want to work with the MLVs. They want to have the specialisation.
Then the second comment, to confirm what Istvan was saying, it's we're still going to work in relationships. Remember, whatever you're selling, whether you're selling technology, whether you're selling translation services, the person that you are selling it to are risking their career by either changing to you as a vendor or by not changing to you. Okay, so you need to be very good in presenting how you do it, and you have to have the data points. Thank you. Thank you so much. So, okay, SLVs are not dead. I take it back.
I would argue that I'm sure it's not just one language you are offering, but we will talk about that later. According to Sophie, who is 20, late 20s and lives in New York. Okay, one last word from you, Bruno, because we are at the end and we are almost ready for lunch, but I know that's big pressure, right? With everything you heard today, did you change your mind on your outlook, or do you feel the same as you did when we started this panel? I feel the pressure that I'm only the thing standing between you and your lunch. So as we are preparing for the future, we think about non-individual applications or even technologies, but supply chains, value chains. What are the value chains of today and the future that we want to be relevant for? And what's interesting and what the past 48 hours confirmed that these are not zero-sum games, that SLVs and MLVs exist as a value chain in synergy. And we have seen that with machine translation, with the benefit of hindsight, we can't say that it wasn't disruptive, it was co-opted by very resilient value chains of our industry.
And we might see something similar happening with large language models. So we want to prepare for that to understand and serve these value chains by supporting the relevant applications, technologies and all the humans in the loop that are part of it. Thank you so much. So in the end of the day, MLVs and SLVs can exist, can coexist, and humans and the large language models can coexist as well.
I'm definitely taking that away. Thank you so much for your insights. I could go on for another hour, but I feel like probably people would be mad because we are all ready for some lunch. Thank you so much for your knowledge and sharing all of this. Thank you, Sophie.
2023-07-26 10:44