Pioneering Medical Research
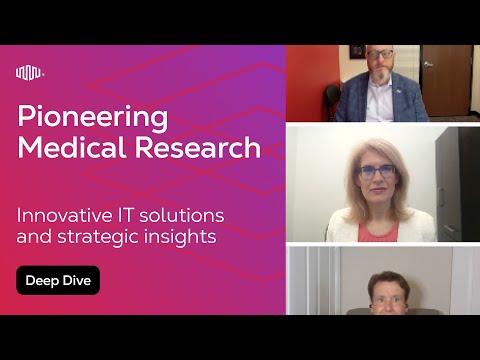
Hello and welcome to today's xtelligent healthcare Fireside Chat presented by Equinix. Pioneering medical research. Innovative IT solutions and strategic insights. Technological advancements have the ability to accelerate medical research and development by improving data collection, analysis, and management processes, thereby speeding the time to scientific insight and innovation. In today's discussion, we'll explore how technology enhances data quality, integrates with existing infrastructures, and supports advanced computational workloads while also ensuring privacy, security, and collaborative efficiency. I'm Kyle Murphy, vice president of editorial exchange and healthcare media.
It is now my pleasure to introduce our speakers. Let's have each one of you briefly describe your work and your organization's role in accelerating health care research and development. Let's begin with Renee Potter, Equinix Director, America's Life Sciences Ecosystem Lead. So Equinix is a global infrastructure company that helps solves challenges for over 10,000 customers. We offer physical co-location for in over 250 data centers around the globe, as well as virtual infrastructure capabilities and healthcare and life sciences. We have over 400 customers and they demand a number of different things.
So they demand access, access to the tools they need. The clouds and the SaaS platforms for AI and analytics, as well as access to the research partners that they're exchanging data around the globe. Flexibility is also key for them. So standing up infrastructure quickly because they're responding to health emergencies or because they're collaborating on a short term projects with research organizations around the globe. And also reach we have data centers in over 30 countries.
And now on to Pure Storage’s Head of Life Sciences, Strategic Alliances and Genomics Bill Lynch. I talk just about every day with clinicians, researchers, bioinformatics and technology heads. And they all have the same kind of thing. How do we make working with data in a research pipeline faster and easier? We need to get to our outcomes much more quickly and simply, well, typically they're using storage that isn't built for AI work or HPC work, and that slows them down. Here at Pure Storage, our philosophical approach to working with data is that it should be super fast and super easy.
You shouldn't need a PhD to work with your data platform. We use the term a faster time to science because that's our focus every day. How do we make it easier and faster for our clients to get their work done? And there are results. What used to take our clients weeks now takes days. And what used to take the days now takes them a few hours. Excellent.
And finally we have Dr. Andrew McArthur, Associate Professor of Biochemistry and Biomedical Sciences and Program Director of the Biomedical Discovery and Commercialization Program at McMaster University. Good morning.
Yes. So, McMaster is in Hamilton, Ontario, Canada. It's the most research intensive university in Canada, and particularly the faculty of Health Science was the birthplace of evidence based medicine. My research program individually is infectious disease surveillance.
So you might be familiar with the idea of a Covid variant. We we write software and do surveillance to track those. But within health science, we have dozens upon dozens of labs using advanced instrumentation on anything from allergy to chronic disease, like heart disease, for example, or mental health. And so massive amounts of highly mobile, highly diverse data is a major research challenge. And my job is, is to sit and keep that sort of sky high view of the overall infrastructure.
How do we move dozens of labs forward? Often when you have conflicting priorities and very conflicting technologies. Excellent. Well, welcome you all to today's presentation. Let's get into the chat and let's start with a faster time to science.
Bill we’ll begin with you. What strategies does, Pure use or employ to minimize the time from data collection to actionable scientific insights? We do a number of things to help our clients speed their pipelines, some of them up to 24 times faster. First, we utilize the fastest flash storage technology available. Next, we design and manufacture our own storage platform to ensure that faster time to science.
For instance, our platform handles very large files and very small files at the same time really well. And we have parallel processing capabilities built into our platform to ensure that multiple researchers, engineers and bioinformaticians can work on their pipelines at the same time, which saves a tremendous amount of time as opposed to doing work in a sequential order. And we take up very little floor space and use far less power than any other options out there. Excellent. And Renée.
So the flexibility and agility that our colo affords our customers is unparalleled. So customers can collect data from a single cloud or a multi-hybrid cloud or even data marketplaces. So we enable that capability while still being mindful of the data sovereignty issues as well as the regulatory constraints. At Equinix, we can adjust all that data because we have 1800 network service providers coming through our data centers. Really the heart of networking.
Excellent. Can you share a specific example? You know where the technology has helped reduce the research cycle in terms of a health care project? Yeah, I think what a great example is Harrison.ai, that's a med tech company that wanted to scale with over 1 million patients per day. And this clinical led AI platform deployed Nvidia's DJX server in platform Equinix to support their hybrid cloud infrastructure.
And this private AI deployment resulted in eight times the data process and capability, and required extremely fast storage to ensure that compute power was fully, fully utilized. So what they did is, used a FlashBlade storage to connect to their racks and then used an Equinix interconnection solution. And this high performance compute solution reduce their training time for their AI models from months to days, and enabled them to launch their AI solution for their chest x-ray solution that found and detected 124 findings with greater than 45% accuracy compared to humans bringing that solution to market that much quicker. So the results were that clinicians were able to make the right diagnosis faster, that they were able to treat patients sooner and actually able to, allow access to healthcare solutions to almost everyone.
Excellent. All right. Let's shift to the importance of data, as it pertains to AI. Dr. McArthur, let's start with you. How do you ensure the quality and accessibility of data for the AI processes in your healthcare research? Yeah, we're really undergoing like a generational change. Particularly the newer faculty that come are at the bleeding edge of this work.
Research is a fairly chaotic environment. And we do this basically by having sort of a layers of infrastructure. So you may have studies studying frailty that, you know, are going for ten years long.
So there's no urgency to the data. There's data collection in storage. You don't need anything particularly elegant until the 10th year is up. And suddenly you need to work with all of it. So you need a a high access memory environment.
But other clinical point of this spear, you may be dealing with massive data sets coming in robotics with a five day turnaround. And so this assessing quality we actually use AI to look at data quality. But then we use sort of layers of storage and processor. So flash storage is, you know, the favorite for the high volume high speed particularly that powers AI.
If you if you need an underlying data set of billions of molecules designed a new antibiotic, you need that high speed access as well as high memory storage. So the the challenge of a research environment is not enterprise. It literally changes day to day.
And then if a pandemic hits, it changes hour to hour. So you have to really focus on a very layered and nimble approach. But you need to be able to swing a big hammer when the big priorities come up. Excellent. Renee, what about you?
So at Equinix, we have over half of the healthcare and life sciences customers in the Fortune 500, as well as nearly 40% of the public cloud on-ramps, as well as access to just a number of SaaS, providers out there. So we allow access to partners like NVIDIA, so you can access the BioNeMo’s gen AI models for drug discovery. We actually recently announced a partnership with NVIDIA in which we are offering an NVIDIA DGX private cloud in our data centers, where Equinix manages it for the customer. And we currently have a global pharma company that's running a number of workloads on that.
So all in all, we're really providing access to the tools, the tech partners and the research partners that the customers need for a successful AI program. Excellent. Next question. Let's have everyone chime in on this one. And we'll start with Dr. McArthur.
Can you discuss the future direction of AI in healthcare. And you know, where do you see you know what are your plans where that when it comes to supporting this evolution. Yeah, this is pretty well an open and exciting world I would say, you know, getting better outcomes for people or even veterinarian animals really is two sided. You have to generate knowledge and then you be able to effectively apply that knowledge to make your decision making in the AI is involved in all aspects of that. So the other aspect in science is we're increasing robotic in the data generation.
So if we want to say cure a superbug, an infection that we have no working antibiotic, we have teams mass, you know, massive amount of generation. Then an AI that designs an antibiotic at the end is a generative AI for antibiotics. And then you test in the lab, it works. So you have that sort of underground research to it.
But then you have the diagnostic piece, you know, can you if you take a blood draw, can you actually diagnose it to say, no, I need this new antibiotic for this particular patient. That's a large gap. Again, AI is opening up a lot of that because we use large sensor technologies. Even on a simple blood draw we're moving into genomic sequencing from a blood draw. And so this is pretty well unlimited.
And you'll see that the spectrum of researchers are all the way back to, you know, basic research in chemists all the way to frontline clinicians interested in AI for both the discovery and the decision making process to to lead to better outcomes. Renee. So the rapid pace of change in AI models, due to the compute power is really increasing the number, just the vast amount of data that's required to train those models. I think currently there is, trepidation in the algorithms, for those that directly impacts the decision on patient care.
So going forward, I think there's going to be more emphasis on the transparency of that. Those algorithms, and those data sets, along with the lineage of that data for audit purposes. I think furthermore, the quantum compute, will play a role in this evolution. We have one quantum provider that's actually focus specifically on life sciences and we have others that are accessible on the platform. So whether it's AI or quantum or a combination of both, I think Equinix will continue to provide the ability to easily, easily connect to these partners, and provide them that provide those leading edge tools as well as cost effective and secure solutions to store and access that data, and really bringing that faster time to science that everyone's looking for.
And Bill. I want to echo what Dr. McArthur said. It's it's a very open and exciting time with, with AI and what Renee was saying with Equinix and their partnership with NVIDIA. At Pure Storage we also have a very strong relationship with NVIDIA and our 1400 healthcare and life sciences clients tell us that AI is and will continue to be a very crucial part of their work, whether it's for clinical operations or clinical research or ongoing operations. And the foundational lifeblood of the AI is data. That's fairly obvious, and Pure Storage is proven to have profound positive effects on pipelines and underlying infrastructure.
So our direction is to continue to provide a scale-up and scale-out data storage platform that's super fast, easy to work with, can be utilized on prem, in the cloud, or a combination of the two. We essentially want to future-proof our clients. So regardless of the AI and cloud decisions that they make, Pure Storage adapts with them. Excellent.
Let's move the conversation on to cloud flexibility. And Renee, let's start with you. How does cloud or how does the cloud adapt to fluctuating demands of health care research especially you know you've mentioned scalability but we also have computational power as well. Yeah. So clouds are agile but we're the integration point that enables the agility.
And across all those clouds we have over 220 cloud on-ramps across the globe. So we are enabling these organizations to quickly deploy infrastructure virtually, within minutes if needed, so they can leverage their existing infrastructure or they can burst into the cloud, providing flexible scalability with computation. We actually did a really interesting POC, as well as a white paper with Pure Storage, along with Illumina and Microsoft Azure in which we compared, Illumina’s Dragon's bioinformatics software on prem and compared that to a burstable solution.
So what we did was we took Pure Storage’s FlashBlade put it into Equinix Metal and burst it up to Azure, where Dragon was was sitting. And what we found was it uploaded data 38 times faster, with 25 times more samples analyzed in parallel at 50% reduction in cost, which is pretty remarkable. Additionally, if you need exchange between the clouds, because you have a hybrid cloud architecture, you know you can do so using Equinix Fabric Cloud Router. Excellent. Next questions for Dr. McArthur and Bill. You know what challenges Dr. McArthur have you encountered when it comes
to integrating cloud technologies with your existing IT infrastructure? You know, and what were your strategies for for addressing these and overcoming them? Yeah, I’d say, I will highlight there's been a lot of successes as well. But, I think some of the challenges, honestly, from an academic research lab, sometimes it's outright cost, can be a challenge for the researchers because they live off research grants and the rents, Two examples I think that are problematic. You know, when we talk about robotics to generate data as the training set, that works well. But often in health care, the data set comes from patients.
People who are ill in that are consenting. And a lot of the times the consent blocks cloud based computing. They wanted on a server on the campus, you know, in the basement of the hospital. And so you end up with data that's cloud based and data, it's not cloud based. And you run into a lot of technical challenges of pulling that together.
So part of it's education around data security and data handling and why it's okay for some of your data in an anonymous forward to be put on a cloud server? In the pandemic, the most obvious we had is when you're trying to track and hunt variants on the national scale. When you get a sequencing lab in North Bay, Ontario, network is a problem. And so we tend to sitting in a big city like McMaster never even notice network when it comes to cloud based applications. But when you're doing surveillance work like mine on a national scale, the actual national backbone can become a challenge.
When it comes to using cloud storage, once you get the data there, it's great. But it's getting it there. That could be the challenge. Excellent. Bill.
Well, as you can imagine, a large percentage of Pure Storage clients utilize these services, and we want to help them do that as cost effectively and efficiently as possible. In cloud storage, costs, including for repositories and archives, can be very expensive. And we have a number of ways that our clients can work with their data in the cloud without having to store their data in the cloud, thereby ensuring they can keep their storage costs under control. And that's for both scratch space and archival repository and our partnership that Renee mentioned with Equinix, enabling cloud adjacent storage so that data can be used in the cloud without having to store that data in the cloud is just one example of this.
Excellent. All right. Let's move on to handling new types of AI and high performance computing work. Renee. Let's let's start with you. In what ways is your technology evolving to accommodate the increasing complexity of AI in HPC workloads, in health care research? So at Equinix, we're not necessarily thinking about seeing ourselves as evolving the technology, but really aggregating the access to all that tech evolution in the industry. Our goal is to ensure that you have access to all the AI and high performance compute providers that's necessary to conduct digital business. So we have, HPC as a service, GPU as a service, and we're even starting quantum as a service.
So what we're really doing is future proofing your high performance compute evolution. So for example, the DGX, NVIDIA, private AI solution I mentioned earlier where customers deploy their data in a private cloud. Equinix is managing that for the customer on a subscription basis.
So that allows them predictable pricing, high performance compute, but also the adjacency to the clouds and the business partners so they can take advantage of the fast paced, evolving world. So if you're doing some or all your analytics in public clouds, we can enable that proprietary data to be stored outside the cloud and then connect it to the public cloud in a low latency fashion. And we can even help you access and transit that data between clouds. And Dr. McArthur.
How how is this kind of playing out at McMaster and some of the work that you're doing, as you know, talking about the complexity of AI in HPC? Yeah, it's, you know, we talk about AI being a revolution and it's one of the ones where definitely the demand for better and better in large and larger training sets and therefore, you know, GPU based platforms it’s at a huge pressure point for us. Both on the storage and on on the compute side? We essentially know it's a little bit of hanging on by your fingernails, to be honest, at this stage, but I think it's because we're in a period of massive change. I see things getting better. The other revolution is not as obvious. Before the pandemic,
DNA sequencing, was at large institutions, large centers. Post-pandemic. You have DNA sequencers at your local hospital because they're tracking variants, and now they're starting to track flu. Data generation sequencing, the price is just plummeting.
We passed Moore's and Kryder’s law many years ago. And so high performance compute is now a demand. So that means we really adapt around management techniques and integration with Slurm and other technologies to really make us as nimble and environment as responsive, and environment scale remains still a challenge.
I have a joke that we have Kryder’s law that if I build it, 1800 people will show up to use it. So that's the nature of scientists. If you give them high performance compute, they will simply do a bigger experiment or a bigger study. And so when we talked about affordability, deployability, cloud these are key issues that we're constantly looking at and trying to come up with an effective business plan.
Kind of following up on that. Dr. McArthur. If specific to storage, how do you ensure that it's efficient and cost effective as these, you know, AI and HPC workloads start to generate and proliferate data at kind of new levels. Yeah, and so this is the difference between an enterprise environment and a research environment. We essentially have to do this on a continuous basis redeploying, redesigning rethinking.
How do we do the research making very difficult decisions around priority, especially if patients are on the other end. You can have conflicting needs. And so, what's important to me behind that is if you have, if you're using, cloud based storage, that it isn't working in the enterprise level, that it's highly stable, that it's highly reliable, it has good archival and good backup, and therefore, it's very fast, is a key aspect to it that allows me to be sort of nimble and even at times I would say chaotic on the, on the research end. All right let's get into privacy, security, which are obviously two major issues. When you get into clinical research, you get into intellectual property in the scientific fields. Dr. McArthur we’ll stay with you for a moment.
What measures are you taking to ensure the privacy and security of sensitive health care data? Obviously, you're in Canada, but still some high, protections and complexity of of rules. Yeah. And you mentioned commercialization piece. This is the increasingly an important part of this conversation that with AI and the rest, we are seeing increasingly and commercialization of research, and therefore the underlying data and how you handle privacy and security.
Yeah, we are in Canada. We have pretty strong laws about this. That, particularly between, between provinces, it can be hard to share data. The consent law is pretty strong.
So as long as you, you know, the patient is willing to consent, you can do a lot with the data. But honestly, there is a tendency to, move away from cloud because of that, because you get a heterogeneous response from your population. Therefore, I you know, there's a push to keep the server farm on campus, on your own network.
In a practical reality that can't last it, the sheer volumes of data is just not working for an institution. Try to maintain its infrastructure. You really need to work in a cloud based setting. We’re early days, figuring that out. But, I would say we haven't figured that out and occasionally it's a straight up barrier. Right.
And then that we're not doing research as well as we put. And then, Renee, given that Equinix is spread across the globe, you know, how do you how do you approach privacy and security considerations in research? So at Equinix we provide detailed information on the compliance of all our sites. And then we work with our customers to make sure that we meet the needs of their security teams. Excellent.
Bill, let's let's move on to you discuss some of the specific challenges related to data security, health care research, and what you're doing at Pure Storage to overcome them. It's it's a huge problem. And, you know, recent ransomware attacks have crippled several hospitals across the world and in the U.S. And U.S.
government agencies have alerted the health care industry to expect increased attacks in the coming months. Plus, it's estimated that a new organization falls victim to ransomware every 11 seconds. So keeping clinical and research data really all data safe and accessible only to those people who should see it is paramount to our clients and then to us. That's why we deploy innovative cyber security protection like ransomware protection and snapshot replication to ensure that our clients data, including their intellectual property, is always safe and private. Excellent. Renee, do you have some specific challenges you're seeing around data security? Well, we allow our customers to really own their own physical infrastructure and control their data and then integrate it with the clouds when and where and how they want.
So we provide that control with our customers. Additionally, we provide private interconnection, whether that's physically or through programable software. Excellent. Let's move on to collaboration. And Dr. McArthur, let's start with you. How does technology facilitate these different researchers, stakeholders, institutions to work together in health care research in a convenient and effective way? Yeah, this has been a sea change.
I left industry and I joined McMaster about ten years ago to lead some of the others. When I came collaboration around data science, in IT was pretty minimal. Labs often did their own thing.
It has just been a dramatic change through, you know, cloud based technologies about, you know, just having the high performance, research infrastructure, collaboration is kind of the key. And because of the nature work that a lab that wants to say, look, in immunology and use AI to predict, you know, immune response of patients, they're immunologists. They need to collaborate with AI specialists, but they also need to collaborate with IT specialists. Right.
And so it is forced to collaborative nature, the design of the technology, particularly projects that do use cloud based solutions, it just allows almost endless collaboration. It's really changing that. And that's also leading to lots of commercialization as I mentioned. And, Renee, your work in terms of facilitating collaboration. So we facilitate collaboration by providing access to the healthcare and life sciences ecosystem. So we have a breadth and depth across that ecosystem, to facilitate data federation and data exchange.
So for instance, we have six of the top eight global genomics companies as well as like nine of the top ten global pharma companies on our platform. We also provide flexibility for our customers so that they can provide virtual deployments for their immediate infrastructure needs to enable that collaboration and scaling their compute and storage, as well as that easy access to the public clouds, and then also the reach. So, you know, we're currently enabling global pharmas and biotechs to securely exchange data within their orgs or across the globe with with other organizations. So we also have genomics projects that are happening right now on platform Equinix, where they're collaborating and exchanging data using these solutions.
Dr. McArthur, do you have any examples of some of the collaborative projects that you've worked on that have been enabled by technology? Yeah, I think, you know, so we have a sort of long game collaboration is one I'll highlight is Covid variants. So early on the pandemic, we were, of course, all worried that the variants would change in their behavior, either become more lethal and more effective. So like many countries, we built out a sequencing infrastructure to, you know, take a nasal swab, figure out what's in there. But the data that comes out, a sequencing enabled swab is extremely messy.
And biotech's workflows there, there are a lot of successful ones, but they work in a low throughput environment. But we knew a pandemic was coming that we would have ten thousands of patients a day. Right. And so we think from a computer science point of view. So we set up a national collaboration. We had scientists from all coasts and everything in between. We had a black hole physicist, we had a good, you know, computer science program or infectious diseases.
And we built the national software platform for predicting variants from sequencing from a nasal swab. And the end product is we focused on the infrastructure was it is just as accurate, but ten times faster than any platform, elsewhere on the planet. And it became the national standard in Canada and a few other countries because it was designed, knowing a pandemic was coming. That's unfortunately my job to keep an eye out and know these things are coming.
And but if we did not have that infrastructure and if we did not have collaborators that understood that infrastructure, we probably would have made something a lot less effective. Excellent. And, Renee, do you have an example of some of the collaboration that you guys are enabling? Yeah, I think, Children's Cancer Institute of Australia is a great example here. So, some of their challenges were they're trying to scale their high performance compute and storage, along with supporting a collaborative model. So they decided to store their genomic data in platform Equinix. So they would be close to the cloud so they can leverage AWS and Azure as well as use our Fabric, which is our secure data transport, to share data with their business partner.
And some of the benefits that they saw not only was it a cost effective solution, it streamlined their collaboration process. It was scalable to meet their future needs. But most importantly, it provided that faster time to patient care. And additionally, that new platform that they deployed, was designed to be resilient to system failure to avoid any impact on future pair.
Excellent. All right. Let's shift gears to preparing data for analysis.
And Bill, let's start with you. What role does you know Pure Storage technology play in the extract transform and load process. You know especially in terms of data quality and readiness for analysis? Yeah, the ETL process can be very burdensome with the wrong technology.
So there's several things that we do to make it easier for our health care and life sciences clients to work with and prepare data. First, you make it easy to find and access files quickly with millisecond latency. Second, our FlashBlade technology handles all kinds of data from structured data like EMR data to unstructured data like genomic samples and cryo-EM studies. And since it is a scale-out platform, you can use it through all stages of the ETL process, saving your conversions and steps in the pipeline all to the same platform. Excellent.
And Renee, can you speak to Equinix role in ETL? Yeah. So what we do is provide access. Access to the partners like Databricks, Snowflake, you know, those SaaS providers that are playing a critical role in the AI workflow process. Additionally, Equinix Metal can provide an easy on demand platform for data cleansing, translation and decimation. Excellent.
And then Dr. McArthur, you know, how do you address these challenges of integrating and transforming, You know, really a diversity of data types and sources in healthcare research? Yeah. And this is what my group specialize is the, you know, the art of bio curation. How do you get the data ready for the machine learning people or the clinicians? Part of it is reliance on the infrastructure. Right? So increasingly in science, we're robotic based, and now we're having standardized outputs, much more reliable data as opposed to small ad hoc data sets. Traditionally biocuration pressure
on that data was a human enterprise, specialists who knew to look at the data and did data cleaning. We increasingly design AI’s to do it for us, or at least it's AI assisted data preparation and cleaning and quality. I think the role of the human being, particularly in the training data set, is still critical. You need that gold standard.
But we now increasingly have AI is walking us through that process or looking for patterns that should not be in the data, you know, before it goes into the training set. So again, and it's the scale that you need that, as we said, you know, accessibility, high speed access to these data. For the end you need large training sets to training AI, even to evaluate data.
Never mind, you know, generate a hypothesis. Excellent. And then in kind of closing things out here today, let's look to the future. Obviously, it's difficult to predict, but let's let's take a stab at it.
Dr. McArthur, looking forward, you know, what emerging technologies or trends do you believe will have a significant impact on healthcare research and development going forward? Yeah, I think the thing that, surprised me and that I think is amazing is generative AI technologies. I really didn't see their place clearly in this, certainly machine learning. And, you know, having the red light, green light go off based on complex patterns. Very important for diagnostics.
But you take antibiotics, for example. We are in considerable trouble. We're losing antibiotics through drug resistance. Faster than we can discover new one. The easy days of finding them are long past.
But we have researchers now creating generative AI that makes antibiotics that we never even have seen in nature or never even have thought of. And these AI’s are trained to think like a chemist. So they actually the molecule they predict is something that's affordable to make and doesn't make toxic byproducts. I was a skeptic, I'll readily admit. And I think that the idea of generative, you know, the instinct of many is to say it will be like ChatGPT and you can't really trust what it says. When you connect generative AI’s with lab technologies to validate that result, I see we will have a lot of unexpected outcomes and a lot of them that will be very beneficial.
Excellent, Bill. I think essentially, as you heard, two obvious, and these are the cloud and AI. I mean, the cloud provides a number of options for AI based research applications and services like GPU and CPU compute services. But as we mentioned, it can get expensive. AI is the next technology wave that it's risen up and asserted itself.
And we we expect that will be top of mind for many years to come. And AI is so data reliant and so important to our clients near and long term success. It naturally has been a huge focus for us and will continue to be so. We've engineered our platform to enable clients to work with their data in any number of AI workloads and use cases, whether in the cloud or not. We believe it's important that we give our clients the flexibility to take advantage of technology use and approaches that are coming down the line.
As I mentioned earlier, we like to look at that is helping our clients be future proofed for all the changes that are coming forward in technology. Excellent. And then Renee, you obviously mentioned quantum. So that's cutting edge there.
But I'm curious what are some other trends and opportunities you're seeing. Yeah. So I'll take, I agree with both my colleagues here. I think, couple other things that come to mind for me are synthetic data.
I think the techniques have become very much more sophisticated. So this is really becoming more of a viable option. Digital twins. There's multiple use cases out there. I think, one thing that stands out for me is sort of the impact on precision medicine and what that means for an individual with preexisting conditions or, you know, the impact on the drug interactions that are currently taking. And then as, as you mentioned, the advancements in supercomputing, whether that's AI or quantum, you have to remember that there's something like 10 to the 160th potential therapeutic proteins out there, and these technologies will allow us to explore many more of them, as well as pharma companies will be able to to look at them.
And I think if we look in the next decade that pharma companies are going to be looking much more like technology companies than they are, right now. And then lastly, let's let's talk about kind of what's ahead for your individual organizations as you move forward with your health I.T. Dr. McArthur.
What let's start with you kind of where something near-term challenges that you're going to be working on next. Yeah. I think near end long term, our definition of what a biomedical researcher is changing fundamentally ten years ago, I was the odd one because I liked hardware. But now we have young students coming into undergrad that already have some of these skills in their belt, and they want to work in cancer research, or they want to work in Alzheimer's.
And so really, how we view the training and what we consider the data behind the evidence we used to help people is changing fundamentally. We see that in the young professors that are being hired. They're very different from my generation. I also think that organizations like mine will increasingly be collaborative. This is not something you do on your own.
You will see more academic, industrial collaborations. You will also see more federal investment, you know, Data Commons as opposed to going it alone, which is the traditional way of doing things. It's simply it's inefficient. It's not effective, to not work on a sort of a national scale or international scale.
So that's what we're going to see. That's a big challenge for a university who's used to a little bit more navel gazing and worrying about the next semester. Right. So it will be a changing. Excellent. And Bill kind of what's next for Pure.
Well, Pure Storage’s core beliefs are that storage technology should be fast, simple to work with and available in the cloud or on prem. The right storage speeds, workflows, speed pipelines, and we engineer and manufacture our technology to do just that. So we're going to continue to evolve the platform to make it as easy and as fast as possible for clinicians, researchers, data scientists and engineers to use data in their work. For example, as Dr. McArthur mentioned, generative AI is one of the fastest adopted technologies in history, and Pure Storage is working with NVIDIA to bolster the power of AI for enterprise AI applications using retrieval augmented generation, or RAG. In our testing with this video, we learned that RAG document embedding and indexing were completed 36% more quickly when using the Pure Storage FlashBlade S with a native S3 interface and when using local spinning disks.
And as I mentioned before, the cloud and AI are obviously core marketplace drivers of our roadmap as our clients pipelines evolve. Excellent. And Renee, what's going to be going on at Equinix moving forward? Well, we're going to continue to expand access. So continue to grow our list of partners that are offering those data tools and analytics as well as a service provider so you can continue to evolve and improve the models. Also continue with the flexibility.
So the flexibility of the health care ecosystem to deploy virtually or physically in their deployments, whether they're responding to health emergencies or they're just doing short term projects, also going to continue to expand our reach, we are expanding into new markets, most recently Africa, and we're going to continue to do so and be where and serve the customers, where they're doing business. Data is a strategic asset. And so you need to be thinking about how IT is enabling you to succeed and Equinix will be there to support you in that effort. So in closing, I'd like to emphasize that Equinix will continue to provide that ability to easily connect to partners, places and opportunities to enable that faster time to science. Excellent. Well, that concludes today's presentation. Special thanks to our speakers, Renee, Bill, and Dr. McArthur.
And a special thanks to our audience as well. And Equinix, our sponsor. Take care everyone.
2024-09-17 05:34