Modernizing finance processes with machine learning in Oracle Cloud EPM | CloudWorld 2022
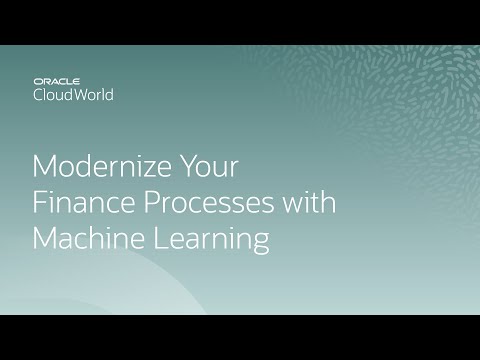
foreign responsible for product management and strategy for oracle's Enterprise Performance Management business in today's session I'll be discussing how we are leveraging emerging Technologies such as machine learning and Predictive Analytics to help modernize the finance function so as all of us know the finance function has been going through a lot of changes especially in the last few years so what do we see when we look uh look out several years say over the next 10 years what sort of changes do we see for the finance function so here's a point of view from the strategy Consultants at McKinsey and Company they see the following as some of the key imperatives for the finance function over the next decade or so the first one is the finance function needs to be able to deliver much better insights in real time the second element they see is that Finance function needs to find ways to help minimize human error and biases and the third imperative in that opinion is finance function needs to be able to drive speed in business processes and especially uh in decision making inside Oracle we agree with this point of view very much in fact the way we see the future of Finance is very much aligned with these ideas so we believe Three core ideas will Define the future of Finance the first one is that the finance function of the future is going to be highly connected it's not the traditional bookkeeping role it's a strategic advisory rule what that means is finance needs to be actively collaborating with operating functions such as sales marketing Human Resources manufacturing supply chain and so on and helping to provide input and influence in actively shaping every strategic decision that is being made in an organization so it's a highly connected function second is that Finance needs to find ways to incorporate what we call data driven intelligence in every aspect of decision making the idea is that data-driven intelligence needs to be blended with human judgment to drive the right decisions and when we talk about data we take a very broad view of data here it's not just financial data it's the combination of financial data and operational data from other functions such as sales marketing human resources and so on it's also not just internal data It's a combination of internal and external data so it's the blending of data driven intelligence with human judgment that'll drive the right decisions going forward the third element of what's going to be different about the finance function of the future is that Finance needs to find ways to automate low value activities so there is a lot of manual labor in a lot of areas of Finance whether you look at Financial Planning and Analysis or whether you look at Financial consolidation and close activities or reporting activities there is a lot of manual labor today so going forward Finance needs to find more and more ways to automate this manual work and free up people in finance whether they're Executives or regular employees and finance free free up their time to focus on higher value-added activities so connected intelligent and automated those are three elements of how the finance function of the future is going to be different so keep those in mind we'll keep coming back to these three ideas throughout this presentation so let's take a look at where um you know Technologies such as artificial intelligence and machine learning um find use in finance so here's a point of view based on a survey conducted by the apqc organization American productivity and quality Center I'm sure many of you are familiar with this organization so they ran a survey of hundreds of organizations as you can see here and these what you see on the slide came out as the top use cases for the use of AI and Automation in finance I'm not going to go through this list of 10 but let me pick out about half of these areas which are closely related to Enterprise Performance Management so the second from the top is closing consolidations uh further down is financial forecasting the one after that is payment matching further down you see the last two Financial Planning and master data management so you can easily see that at least five out of these 10 use cases for AI and automation within Finance are closely related to Enterprise Performance Management which is why you know we are investing heavily in leveraging AI machine learning and automation Technologies in the context of Enterprise Performance Management let's look at another piece of data again from apqc from last year this is a survey of about 1 000 organizations in terms of uh where organizations are adopting Technologies such as artificial intelligence and machine learning in the finance context so the top areas came out as purchase to pay record to report order to cash and most importantly in the context of this presentation Financial Planning and Analysis fpna so if you look at the data in the last row here over 50 percent are highlighting the use of AI in the context of financial planning and Analysis or have already in some fashion implemented Ai and machine learning Technologies for fpna use cases so over half of the 1000 organizations surveyed last year see Ai and machine learning Technologies is very very suitable for fpney processes so keep this in mind that we we completely agree with this because this is what we see as Trends in the market trends in terms of what our customers are doing and which is why we are making significant Investments we've been doing this over the last several years to leverage machine learning Technologies Predictive Analytics Technologies and automation Technologies to help improve uh specific processes within Enterprise Performance Management especially in the context of financial planning and Analysis so let's drill into the details of what we are doing what we have delivered what the roadmap looks like for the adoption of machine learning Technologies in Enterprise Performance Management so the overall initiative that drives our vision our strategy and our investments in this area this area being the use of Predictive Analytics AI machine learning and automation Technologies uh specifically in support of our vision for the future of Finance the vision as you as you remember consists of three key elements Finance is going to be highly connected leveraging data-driven intelligence and looking for automation opportunities in the context of Finance processes right so intelligent Performance Management or IPM is the umbrella initiative that drives our vision our strategy and our investments in this area so IPM you will hear This Acronym IPM a lot IPM is the umbrella initiative under which we are making a lot of these Investments to deliver functionality today and into the future so let's take a look at IPM what it's about what we've delivered and what the roadmap looks like so here is a high level functional architecture of intelligent Performance Management so this is a sort of a complex diagram on the face of it so let me sort of tease apart some of the details on this picture so you see the red line in the middle above the red line are functional capabilities that we are delivering to business users in finance below the red line or platform capabilities or technology infrastructure that helps deliver the functional capabilities that business users see and use So Below the red line is technology above the red line is business user functionality so let's start from above the red line take a look at what do we what do we mean by business user where accessible functionality these are things like predictions so we want to use machine learning models to look at data and make predictions so forecasting is inherently a hard thing so can we help improve human forecasts by providing machine predictions computer generated predictions to help improve the accuracy of forecasts so that's the idea of predictions simulations is can I run simulations as a way to help reduce risk in my planning processes in my forecasting processes and so on correlations when we look at data some of the data may be highly correlated for example my sales may be highly correlated to economic metrics overall how the economy is doing or overall how my customers are doing in their businesses and so on so how can we use learning models Ai and ml Technologies to find these correlations and make use of these correlations to help improve our forecasts the fourth one is an important one we've delivered some very interesting capabilities in this area recently it's insights how can I use machine learning models pattern recognition algorithms to find insights from data without my users having to do a lot of leg work to get the data clean the data analyze the data and find the insights instead can we use pattern recognition algorithms and machine learning models to find these insights and deliver these insights to my users so that they can take action on these insights and focus on taking the action as opposed to finding the insights right those are some examples of what we mean by end user functionality available in this area and below the red line you have platform Technologies and capabilities that help deliver these functionalities you know there are things like Auto predict Auto predict is a is a is a piece of functionality that we've already delivered this is based on statistical regression models we'll talk more about this later uh the second element is auto ml engine so this is a machine learning engine that we are embedding within the EPM platform to help uh Drive machine learning based predictions and these machine learning models can be defined within APM using the automl engine or they can be externally defined in platforms such as Oracle Cloud infrastructure oci specifically the Oracle data science cloud or they can be defined elsewhere using Cloud infrastructure from Amazon or Google or Microsoft so the idea here is data science teams within your organizations may have built these models already so rather than Reinventing Reinventing the Wheel by redefining these models within oracle's EPM platform what we do is allow you to import these models using standards such as the pmml markup language you can import these models into EPM and leverage these models in the context of EPM processes EPM decisions and so on so that's what we mean by ml model import and at the bottom layer you see the orchestration Insight engine that helps deliver the insights that I talked about earlier so the bottom layer is technology both Oracle Technologies in the context of BPM as well as broader Technologies in the context of Oracle Cloud infrastructure or Cloud infrastructure from other vendors right so this is a very open architecture as you can see this is a very open framework the idea ultimately the value comes from the upper half of this picture business user functionality that helps improve decision making that helps deliver insights to business users so that's the that's the idea behind intelligent Performance Management I know we've packed a lot into this one slide but hopefully this makes sense so IPM is a very important initiative at Oracle uh this is not about a single piece of functionality this is a long-running initiative uh through which we've been delivering functional capabilities through which we have an active roadmap for delivering functional capabilities so in today's session we're going to look at some of these elements in more detail so let's start with uh the whole philosophy behind this approach with an IPM the idea here is you know look we're not saying human uh forecasting is bad machine forecasting is good uh both ideas both approaches have their place because if you look at machine learning based predictions machine learning models can be fast they can be very accurate they can they're not subject to biases unlike human beings but they do not have the domain knowledge or the business context that human beings have so sometimes they can come up with the recommendations or predictions that do not make any sense because they do not have the business context or the domain knowledge right so they have many positive attributes but they also have some negative attributes on the other hand human forecasters in finance they may be slower they may be less accurate they may be subject to their own biases but they can be very insightful because they understand the business very well they're experience based insights can be brilliant so the idea uh that IPM comes to the table with is really how can we combine the best elements of machine learning models and human judgment-based decisions to come up with forecasts that are really combining the best of both warps right so that's the idea machine predictions will complement human forecasting and that's the core idea in IPM another tenant tenant with an IPM is that you know we know people in finance uh are skeptical because if there is a black box machine learning model that makes a prediction people in finance are not going to just accept that prediction and run with it they will want to know why the model is predicting or recommending what it's recommending right so we'll talk about this idea of transparency or explainability laters but keep that idea in mind as well okay so now let's talk about a couple of key elements of functionality that we've already delivered through IPM um predictive planning this capability has been available in Oracle Cloud EPM for a number of years now hopefully many of you are already using it um and in the last few years we've already also introduced a capability called Auto predict where as new data comes into the planning system comes into the EPM system we automatically use uh statistical models to generate predictions and load those predictions so you know we all know about uh doing variance analysis which typically compares actuals to the forecast right and emerging new best practice which many of you are actually already doing I'm sure is a three-way comparison where you're comparing actuals against not only human forecasts but also machine generated predictions so actual versus forecast versus prediction that three-way comparison has become the new best practice right so when you start doing that you're shifting analysis to be more forward-looking as opposed to backward looking because variance analysis is inherently backward looking you're just looking at okay I made a forecast how did that turn out is all you're doing for the most part um in this new model you're looking ahead to see okay if the machine learning model is doing a much better job of predicting the future compared to my human forecast should I make adjustments to my human forecast using the output of the machine learning model that's the core idea here so predictive planning and auto predict or capabilities that are already available in Oracle EPM hope if you're using them if not take a look at these there's a lot of value to be gained by deploying these best practices the sites like I said before is a new capability that we introduced in 2021 this is a very uh exciting piece of functionality in fact many of our customers have already deployed IPM insights in the past several months and many more are piloting this capability to see how they can make use of it in their business context so let me talk about the core idea behind IPM insights the core idea is that today the process of finding insights from data is very complex very cumbersome involves a lot of manual work so let's take a look at what's involved in finding insights from data oftentimes you know people in finance employees and analysts and Executives in finance have to consolidate and pull together data from multiple systems Erp systems internal systems external systems and so on then you have to clean the data you have to consolidate the data you have to analyze the data run some reports Port through these reports to find patterns data patterns or observations that look interesting right so loading the data cleaning the data prepping the data analyzing the data running reports finding patterns finding interesting observations so you've spent a lot of times oftentimes days worth of work before you've come to identify insights that look interesting and then you have to review these insights with business stakeholders and then finally take action this process is so tedious so time consuming so manual that oftentimes when you find an Insight the time for taking action based on that Insight is actually passed so what we are trying to do with IPM insights is flip this equation where you have to spend a lot less time finding the insight and you have a lot more time to take action based on the Insight so the core idea here is we have a machine learning based data science based pattern recognition engine that's always running in the background and looking for insights and finding insights and it always presents the insights to you in near real time so we find the insights for you we present the insights to you and again you know we do intelligent things like you know okay not every Insight is equally interesting so you can set threshold you can Define what materiality means um so we do a lot of the you know heavy lifting in terms of finding the insights filtering the insights and presenting to you in prioritized order the interesting insights at every any given point in time so every day you come in you take a look at six or eight or ten insights that are interesting to you and then you focus on collaborating with business stakeholders and taking action based on these insights so algorithms do the heavy lifting we free up your time to fix issues and take action so this really opens the potential for uh you know faster cycle times this enables and empowers the finance function to be a strategic partner with the lines of business whether it's sales or marketing or human resources right so that's the idea so it's a very powerful powerful idea we find the insights for you you focus on action it's a very powerful idea and that is why it's capturing the imagination of many of our customers so let's take a look at this in a little more detail the reason we are using machine learning models to find these insights is that data analysis is inherently difficult right because we we say data analysis it's actually a complex process because when you see a pattern in the data you need to teach support several elements in this data pattern so so the the diagram on the left shows you a quick example of this when you see a pattern in data there is Trend there is a trend that's going on there's seasonality that's going on here seasonality means you know okay during holiday season my sales Peak so we need to capture that right and then there is this residual information which is what we need to pour into to understand if there is anything interesting going on here so we need to taste apart Trend information seasonality information and other significant information for example if you made a price change that may result in uh a downward download movement or an upward movement in your volume of sales so all those things need to be teased apart before you see what is interesting in the data that you're looking at so human beings are inherently not very good at doing this it takes a lot of effort for human beings to find these patterns and Trends in seasonality and isolate them and find the interesting nuggets so essentially what IPM is all about is really you know Finding insights is really like finding needle finding a needle in a haystack machines are much better at doing that so we are employing machine learning models to do that that's the core idea here so what do we mean by insights there are many different types of insights that are available here the first is uh you know forecast variance and bias uh so really you know we look for um variances variance analysis is easy machines can do it very well bias is an interesting concept because you know let's say you're assembling sales forecast based on input input from different regions of your organization the North America region may be inherently optimistic and they may be giving biases that they may be giving forecasts that have an optimistic bias on the other hand the European organization May be a little more conservative and they may be giving forecast input that's a little pessimistic in nature so if you understand this pattern then you can correct these biases and make your forecast more accurate that's the whole idea prediction Insight is really as I said before if machine learning models are doing a better job of aligning with actuals then you may want to correct human forecast using the machine learning model as an input anomalies are outliers machines are machine learning models are very good at detecting these outliers so you can remove these and improve the forecast accuracy so these are some examples of the types of insights that we already delivered today and we're working to deliver a growing set of these kinds of insights over time so what is the benefit of IPM insights at the end of the day is really about helping you reduce the amount of manual labor a ground grunt work focus on value-added work eventually leading to better decisions so the chart you see here is really about how the finance function has been steadily improving the amount of time spent on value-added activities you know nine percent Improvement in FP a over 30 Improvement in certain other areas such as tax and Treasury and so on so insights is yet another tool in the toolkit for the finance function to improve their ability to focus further on value-added work so less Grant work better decisions so as I said uh some prediction options were already available in Cloud EPM we talked about predictive planning and auto predict these are based on statistical algorithms uh which are called you know univariate statistical algorithms uh if you wanted to do multivariate analysis things like you know regression or classification using multiple variables for instance sales may be affected by price and promotions and economic activity and Industry level activity and so on if you wanted to do this multivariate analysis today we support the import of machine learning models from other systems Oracle systems or non-oracle systems going forward what we're going to be doing is embedding an auto ml engine within Oracle Cloud EPM to allow you to Define and manage machine learning models directly in Oracle Cloud EPM so you can Define machine learning models you can train these models using sample data you can deploy these models and manage these models within Oracle Cloud EPM in making in defining and making use of these models we have two approaches the first approach is what I show on the bottom called recipe based machine learning which means at Oracle we identify specific use cases say cache forecasting or cash management build a machine learning model for you we let you configure it using your data but we do all the heavy lifting in terms of data preparation feature engineering to find the elements of the model that are interesting do predefined data integration and build the machine learning model that can be configured and tuned using your data right so that's what we call recipe based machine learning the other idea is open machine learning which is where you come up with a different problem that's not broadly applicable to a lot of customers that's very unique to your business so you train the model using your data and you create this model and use that machine learning model for predictions so recipe based model machine learning and openml both are available in both cases there are two there are a couple of elements that I want highlight the first one is the idea of explainability so this is all about providing transparency in machine learning so we tell you we not only give you a prediction we tell you what's behind that prediction what factors or what variables the most influential in coming over that prediction and these variables can be global applicable across many different predictions or local specific to this given prediction that we're making for example the forecast for November 2022 that we're making right now has been most heavily influenced by these factors or these variables so things like that Global local and business explainability business explainability related to specific events that may be influencing the prediction the key thing is um you know it is very transparent so you can understand why we are making a prediction that we are making so that improves trust in the model the second element is as I mentioned the auto ml engine is built right into Cloud EPM which means it's designed for the needs of finance and we take care of things like data preparation feature engineering to define the machine learning model and we we push data into the planning application into the planning cubes as needed so all of that work is done for you so this is not like using an external machine learning model where you have to do all of this work we take care of all of that work for you so you can focus on using the machine learning model as opposed to dealing with the technical aspects of machine learning and the technical aspects of data integration and data preparation and so on so this is our roadmap we're very excited about this roadmap and uh an important application that we're working to deliver leveraging the automl capability that I just talked about is in the area of cash forecasting cash forecasting or cash management is a very very important use case within Finance for machine learning after all all of us know that cash is king So cash management is very very important business process so we are looking to redefine cash management using machine learning approaches using real-time forecasting so that our users in finance can gain operational insights and business agility and Leverage Advanced approaches such as in collections you can use things like receivables Factory you can use strategies like receivables Factory or in payments you can use approaches like Dynamic discounting and you can leverage your banking relationships very very effectively right so this predictive cash forecasting is an application module that we're building leveraging the auto ml capability that I just talked about we're very excited about this in fact we have another session at cloudworld where we will preview this predictive cash forecasting capability so bookmark your schedule for watching that session as well right so now let me close this session with a few touchs the first one is that we spent a lot of time talking about use cases in the context of financial planning and Analysis and financial planning those are the most obvious ones that many of our customers think about when we talk about machine learning and artificial intelligence but we also have have several other use cases Beyond planning in financial close International consolidation in account reconciliations and in reporting some of those are listed here in financial close for example machine learning models can help identify exceptions not only help identify exceptions but also recommend exception handling actions using patterns that are detected through machine learning models right so this will help in automation the first step may be not automation machine learning model May raise an exception and suggest a corrective action and a human being May review it and say Yep this makes sense I'm going to do it as you gain more confidence you may actually automate them and similarly in account reconciliations we can automate transaction matching using machine learning in narrative reporting we can intelligently generate narratives using natural language processing Technologies or machine learning Technologies for example if you see a variance not only can we highlight the variance we can generate text that tells you here are potential root causes that may be causing those variance so this is very useful for somebody to understand the variance and take action right so those are some use cases Beyond planning so when you look at all of this you're wondering okay where do I get started where do I start first what should I do so here are some you know guidance in terms of what you want to do there the first one is select your target case Target use case with some thought you know oftentimes people think about sales forecasting or Revenue forecasting is the most important use case it could well be but there may be other use cases that are you know better suited within the context of your organization think about data availability always start with a pilot to gain some experience and Trust within your organization and define success measures you know be realistic in how you define success you know your machine learning algorithm is not going to be 100 accurate so then don't set the bar too high so those are some guidances that you can read and incorporate in how you start using machine learning and finally here is a an example set of use cases for machine learning in the context of Finance sales forecasting there are use cases in the tax function for tax planning and tax classification we talked about cash forecasting you can even use it for uh you know Roi prediction for capital investment projects so there is a rich set of use cases beyond the revenue forecasting use case that all of us commonly think about so hopefully this has been a very useful session for you uh we are excited about the potential of machine learning and artificial intelligence in the context of Finance in the context of Enterprise Performance Management uh thank you for listening and we'll wrap it up right there thank you for attending [Music]
2022-12-07 07:07