Leveraging Digital Technologies to Improve Management Decision in Dairy Farms
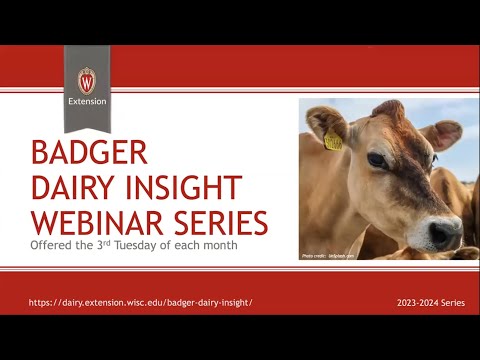
welcome everyone thank you for joining us today on this month's University of Wisconsin division of extension Badger Insight today we'll have a topic called leveraging digital Technologies to improve management decisions on Dairy Farms Badger Dairy Insight is a monthly webinar series offered on the third Tuesday of each month it provides the latest research Dairy based information to improve Animal Welfare breeding and genetic selection Automation and modernization and nutritional decisions for Producers Dairy workers and managers along with agricultural professionals so welcome my name is Angie alness education um extension educator for the University of Wisconsin division of extension and my colleague and I Ryan stere are your hosts today we hope to provide this opportunity as an informal decision um information discussion on today's topic as this is a webinar format please add any questions or comments as we move along through the Q&A button at the bottom of your screen and the presenter also um noted that if you have to jump off early and you want a question to be asked um he will ask answer those questions at the end of the webinar as this webinar is being recorded we'll provide a link of the record of the recorded webinar to you within a week in hopes of sharing uh with others you work with so um all without further Ado I'd like to um introduce our first Speaker or our speaker today is Dr joal Doria and he will speak like I mentioned before on leveraging digital Technologies to improve improve management decisions on Dairy Farm Dr Doria is an assistant professor in Precision Agriculture and data analytics at W Madison he obtained his BS in Agronomy in from the University in Brazil and Masters and PhD degree in animal science from the University of San Paulo in Brazil Dr Doria spent two years coordinating Dairy and beef research in Latin America and in 2019 he was hired as an assistant professor in the department of an animal andary Sciences here at University of Wisconsin Madison Dr Doria developed research focused on digital technology and Predictive Analytics to improve farm management decisions his research group is interested in large-scale implementation of computer vision systems wearable and other sensors to monitor animals on livestock Farms examples of application include the use of digital Technologies to monitor animal behavior growth development and social interactions his Innovative research program has been extremely well accepted by the livestock industry and scientific community so we're really um excited um Dr um dor if you want to take it away thank you very much thank you for the introduction Angel and Ryan thank for the invitation and good morning everybody so today I will talk about the digital technology as Angel mentioned um how we have used that to improve management decision so I'll show a few examples and why we interested on developing those um those Technologies for for da the day industry so if you think about technology um and we look at the progress that genomics had uh in the da industry over the past years uh it's very clear that technology can really make progress and Advance uh our production systems um in agriculture as a whole but you know specifically here today in terms of dairy production H as you can see the amount of milk production per cow increasing from 1931 um and the am number of cows decreasing over time from 1931 up to 2021 and so we are producing more milk and with less uh cow few cows and so that shows the progress in management of course right we we managing the herds better uh the cows better but we also have a tremendous impact of genomics and and now we see all the omics as we call uh making progress as well and people more interested in doing research and understanding how that can bring some advanc improvement in the dairy sector but one interesting component of the advance uh of genetics and genomics was that the cost to genotype the animals drastically decrease over time so um as you can see here in 2001 and 2002 the cost of genotyping animals was high and then as you get to 20078 uh these keep decreasing dramatically up to 2021 and so as this is a good illustration to show as time goes on uh Technologies advance and the cost of a lot of those Technologies keep decreasing which make them uh easier to adopt and more attractive uh to be adopted because now uh the cost to to use this Tech technology is really low and the return on the investment of this technology keep getting better and better as the cost of the technology decrease and so um if we if we look for this particular example here where we have the number of cows uh being reduced and the amount of milk being produced uh Higher and Higher and and a lot of these uh because of better management and and genetic progress we right now um have a bottleneck on this gen genetic progress for example that is related to the phenotypes right so it was easy to collect milk and milk components in the past and make progress on that particular area but now as we aim to improve Behavior welfare Animal Health we do need to collect those meas measurements at the animal level and that's what we call the phenotypes or the traits that we want to improve right and in order to make Improvement in that particular trade or that particular genotype we do need to collect them and collect them in large scale is really difficult uh so if you imagine dry met intake or feed deficiency you want to select for feed deficiency you need to measure feed efficiency in every single animal and so measuring these in thousands of animals um we requires some work and and and is expensive and labor intensive and and that's the area we also work developing technology so we can measure those phenotypes and from from trades or phenotypes that were not measure before and then we could use that to make genetic Improvement and to of course uh make uh better management decisions at at the farm and so our lab is really interested on develop uh sensing technology for phenotyping and as I said phenotyping is this animal level information that we are interest and with that information in our hands then we can integrate this with all information related to price and crop and the feed that feeding the animals and we can also use that information for uh genetic Improvement and the only way we can collect large scale um data for for at the animal level is using sensors right there is no way we can keep watching animals uh eating and say okay eating time of this cow 200 minutes eating time of the other cow 300 minutes that's impossible you cannot sit there and keep watching cows and taking notes the only way we can measure this in L scale is by deploying sensing technology and monitor this in lar scale now this will vary from using robots or using wearable sensors or using drone drones and grazing systems or microphones of cameras and so this is all U necessary in order to obtain phenotypes as or or traits related to animal behavior infectious disease body composition methan emission feed efficiency feed intake social interaction all these traits that people may have interest and that are important from the genetic standpoint and for improvement and also from the management standpoint and so as we sorry as we collect uh that data this will have a positive impact in F management uh so for example if I have sensors that can give me an alert that this car will get sick this is extremely important and so these will have impact on profitability and economics at the farm and long term collecting that data at the individual animal level will bring us um to we will help us to better select animals um Genetically speaking that we will um stay in the herd and and make Improvement uh for that particular trate that has been selected or measured on top of all of this uh it's been really hard to hire uh people in all sectors and in agriculture and that indust is not different and so uh know maybe people don't want to stay um as in the past at the farm working with the animals and so the technology can really help uh on that aspect as well so what do we need in order to build this technolog and start developing we definitely need what we call capacity building we actually are having that or developing this at at w medison so we have Farms with cameras deployed we have more than 100 cameras deployed at the farm where we trying to track animals for Behavior body composition growth development and so this is extremely necessary in order to have robust systems that will be developed and commercialized by by companies um in the in the their industry so today today I want to show you um few examples um that we have they go in our lab to develop related to farm management decisions and they are related to nutrition and health and how we can use for example in today's example computer vision and image analysis to improve animal monitoring and with that generat some decisions that could could um make our systems a little more efficient okay so this is a picture of our research Farm I will show you an example of what we have uh deployed there have run some uh experiments with that that um that infrastructure that we we we built and then we have uh in our farm these cameras installed they are death cameras we what we call them death cameras I will explain in a bit what it is they are installed at the exit of the milking parallel so every time this SCS are leaving the milking parallel here uh the camera will acquire picture automatically of this cows and these cameras they generate uh regular pictures as you can see here like this what we call this gray scale it's a infrared picture it's a grayish black and white as you can see here and then the same camera generate this death image so the death image give us uh the distance from where the camera is located to the animal in the in the picture Okay so each pixel here each value on this image in this image will be the distance from where the camera is located to the object so if you see here dark blue it means that the distance is it's it's closer to the camera and then if you see this uh strong light green here it means that this is far from the camera and so we can map we can have a 3D map of the objects in the scene when you use these cameras now the way it works is every time the cows are walking out of the Parlor this camera will take pictures automatically uh we detect that is an object while walking uh here in the lane and then you acquire a sequence of pictures because we are not stopping the animal we it's all completely automatically uh the way the camera Works we're gonna have what we call bad image and we're gonna have good image and bad image are basically image where you have the cow corpet no cow two cows in the same image and a good image is the image where we have the whole body of the cow as you can see here we want to have a complete uh um the whole body the complete body information of the C within the image in the image and so to in order to automate that process we train a model that basically select what is a good image and what is a bad image so every time you collect the sequence of pictures the model will automatically collect the good image as we collect the good image another model that we train will find the pixels or that that belongs to the animal body basically we segment the animal body um identifying the p pixel that are part of the body by doing this I remove the whole background that's not important it's a bunch of metal bars and concrete I I don't need that I just need the body of the animal and so this second model will you segment the body perfectly and then by segmenting the body this will produce these two pictures um one remember the infrared or the regular picture of the cow and this death image now they are segmented they don't have background so I have the perfect image of the of the cow for these two types of image the infrared and the dep now with that in hand I can do several things which actually it's the object of our studies is I can run animal identification for example using the code color pattern like the black and white of the animal and I can for example predict body condition score body weight body volume of this animal using the death image here so I'll show you more details about that so a question you may have is um for example um how good is the model to identify cows based on the black and white the code color pattern here right and so we have this experiment with 92 lactating cows and we achieve an accuracy of 96% to identify individual animals so the model works very well identifying the animal based on this unique code color because you have a unique signal ature um as because they hosting cows they black and white and this will work some sort of like C code or barcode of each individual animal that we then can identify them using um um the cameras and the computer vision models that we train the problem is as you have Jersey cows black angles or all the animals up that you have very similar or solid homogeneous code color then the model will get very confused in in this case for example imagine if you have four cows these are four different black cows uh hosing cows but all black and the model get very confused to recognize them so what do we trying to solve this problem what we did was uh we did not use uh the black and white coat color to identify the cow but we did use the body shape only the the shape of the cow uh the body surface as a unique signature a unique identifier to to be us it and then we can distinguish and separate the individual animals um using the the body surface now um as we can see here we test different body shape body representations I will not go in detail about Point clouds and and the type of things we tested but what is important to mention here is that we achieve an accuracy or F1 score uh in this case of 08 meaning that the the performance of the models were very good distinguishing uh among different animals we we have a total of 40 animals here that we identify them based on the body surface only and then obvious question that usually ask it is okay what is the animal is changing shape what is like a a there a cath or heer that is growing and the body surface is changing so how these models would behave to identify the animals in the production system as the animal change shape so in this particular tribe here we started skipping we did this with caves from two weeks of age to eight weeks of age so then were really changing uh body confirmation and we started skipping one two or three weeks and so we trained the models with week one and then we skipped two three weeks and got the animal older and and tried to identify the animal when the body was already changed and the model stayed uh contined to predict well the individual animals even in this uh shortterm period were this interval that we had so that the animal changed the body a little bit so recently we we tried to do a little a more complicated identification we skip um 423 days so almost two years um of uh of interval between when the animal the pictures were Acquired and and when the animal was tried to be identified later on so here for example you can see uh this is the animal really really early on with 26 days average age and this is the animal uh 423 days uh of age every age so we try to identify them so you can see for example the difference in body weight 43 kilograms of these Cales and then we try to identify the animal for with 426 kilogram so it's a huge difference in body shape in body confirmation and so but what you want to do is can I train a model when I have a baby CF and then when this animal it's older it's a cow lactating cow if I need to recognize that automatically one two years later can I do that and so what we achieved was an accuracy of 72% precision 76 uh recall 75 F1 score 73 which is very promising results um given the huge interval that we are Computing here and the other thing we try to do is train a model that would receive pictures of animals that would not part of the herd before and the motel should be able say I don't know these animals and so we include 14 new animals and the model should say this doesn't belong to my her and the model was 85% accurate to say I don't know these animals these animals never here before and so all these identification uh tries and experiments we have done with image they are very important for um connecting the phenotypes or the behavior the intake whatever we measure with the cameras with it individual ID and is also important for traceability if you think about developing these for a regional level or for n National level uh would be incredible to have a picture of the animal at the first day of life and then four or five years later you can identify this animal no matter what the animal uh showed up so that that's that's the the long-term goal of developing these uh identification systems based on on computer vision cameras okay so now that we have this pop line um we are very interested on the body conditioning scor now that we can know some some somehow identify these animals let's look to change in body shape and how this can inform for example um for early detection of disease in da Farms so if you go in the literature you're going to find um very similar prediction performance as we found here where researchers have uh reported accuracy to classify body condition score between 81% or 96% uh in five point scale body conditioning score and these will vary depending how much error you assume uh if you if you want to tolerate a 0 25 error in your classification so from you know 3.25 or or three if you consider them the same thing U then you get an accuracy of 81% and or if you consider a 0.5 ER then you go to 96% and this is very close to what is been uh reported in the literature and so you can definitely use the the cameras to classify the body conditioning score given our results and other results that are already published but we are not very interest on doing these at this point we although we recognize the importance of a body condition score body condition score you have the problem of being subjective so I may think this C is 2.5 you may think this car is 3.0 and so that is all this
disagreement made made happen because this is a subjective uh measurement so this is an example I have this cow 21 days before cevin or 14 days before cevin three evaluators look at the Cow um not not through the picture they went at the farm they saw the cow and then they evaluated the C's body condition score 4.0 and for seven days later or 14 days before Cavin 4.0 again when we overlay this image perfectly then we saw a difference in body shape so it's clear uh was clear to us that there is a difference in body shape that our eyes cannot detect and so the then the question that came to us is how can you use the death image how can you use the change in body shape to detect disease or to detect CS that get sick because of body tissue mobilization and that by doing body condition score only would be difficult to to detect and so we then um developed this trial uh we did this in collaboration with Dr had white uh was a very nice trial we us it 92 hosing cows we collect uh prear image uh weable sensor data and text and I'll explain what the text was um and we measured those uh things the image and the wearable sensors 21 14 and 7 days before cevin so we have these three time points 21 14 and 7 days before cevin and we collect blood samples after cevin so we then um Define a cow with subclinical ketosis based on the beta hydroxy booty rate bhba values above 1.0 mol popar so
if the cow had that we we call this cow as a subclinical ketos and we collect 52,000 image of this cows again plus a wearable data for for Behavior plus some text information that was in the software and we use the prepartum this is very important we use prepartum dat here to predict subclinical ketos at the post pattern okay so this last time Point here was seven days before Cavin and we are trying to predict the disease up to 14 days after cevin so you see that in the best case scenario the distance between my last data point and the health event will be about a week eight days or something like this okay that's the best and the worst will be even uh longer period between my last data point and the the health event that may happen within these 14 days okay so it was quite a task but we are interested to do this early early detection so I won't go in detail here but the way we we process the image we train a model uh using the death image these models are called uh that we use convolution networks so basically these models you learn features about the body shape of these cows and then we extract uh thousand features the24 features here using um this convolutional process where extract features from the body shape of the animal recognizing uh parts of the body that are related to body conditioning score or to Chang in body shape and then by extracting features from this image we um looked where the model was putting attention on the body so these are the gradients extracted from the model and so you can see uh you know depending classification of the body conditioning score or or the body shape of the cow the more you put attention in different parts of of the of the back of the surface of the animal so then we extract these features that would represent the body shape of the animals from the death image 2 any1 days 14 and seven days before cevin and then we're going to group all these features and then we have what we call Imaging features we then collect weable sensor data we had colors uh on these animals um and these collect data I explained like eating time uh visit visit duration number of visits at the FED bunk total time which is dating time total time span at the fit bunk uh meal interval um rumination all these Behavior data was were collected in the in the last week seven days before cevin and then we went to D comp uh what was the software we using and extract previous licitation information from these animals and notes about the anal that were put as a unstructured text that's what we're calling here so all these free text notes that you type we type in there comp about the animal we extract that and use that as a predictor and so this was a quite an interesting project uh particularly for the analytic standpoint because you merging the structure data with this tabula data like you know Behavior or with columns and and rows and so on with unstructured data which came from the text so imagine a paragraph written you know imagine you combining these with Excel file um to analyze so it's you know in a simple way to illustrate that's what we end up doing here so again uh that's the structure data I was mentioning so we have the cow you have days relative to cevin number of visits eating time meals time duration ration line time Etc all the these Behavior being computed and they are tabular as I said like structure like a square as you can see here and then we obtain these notes as you can see for each animal so basically said okay um uh the cow was um a double double syn was using this cow the cow was open I did this I used that I collect milk spect from this cow these are the responsible here put my name in my my students name uh so Rafael or Jan Daisy milk which pen this C was all these notes that are not very well structured in the system we convert them into text and so the text he is represented with the date and a sentence saying the cow entered the herd as a fresh heer on that date this event happened in P 26 and so we convert all these threee notes or the notes as are here in this text sentence and then we use a large language model to extract features from this text and so basically these features uh were basically converting the text into numerical representation and that now is tabular and this is a structure and then I can combine these with my image features and my weable sensor feature so just in summary we use this un instructure piece of information we convert these into a numerical representation so the text now became uh numbers and these numbers were connected to the other numbers coming from the image and coming from the variable sensors so what we found was in accuracy F1 score of 68 and an accuracy of 76% uh to early detect of clinical Kos seven days in advance so that was very promising results uh and um when we evaluate the the the impact of adding unstructured notes or these text noes to the model we observe uh an improvement in the model accuracy and F1 score so there was relevant information in the text note um that was added here and usually this is not very um considered or us it in those uh traditional analysis because it's just difficult to integrate free taxt unstructured taxt uh with structure uh data set so so that was a very nice uh result we found and and very promising with 76% accurate see um too early theack cows U that get sick now we do know that 21 days in advance it's not enough and ideally we want to detect this even earlier so we are running trials now where we are looking to six days before cevin as a Time series and see how early we can detect CS that you get sick so we can we can take uh we can suggest or or propose um management um decisions that that could change the course of this scout that we get sick uh in the last uh in the previous uh example I mentioned now about the subclinical ketos I showed you a behavior from weable sensors here is just a paper we published with Behavior now feeding Behavior being monitored through cameras so as you can see we have every time the animal is going to visit the feed Bank We compare uh total time visit duration interval between visits and visits and the way we do this is basically by identifying the individual calf and if the animal cross the head on the headlock we place this bounding box so the motor detects the animal and then by detecting every single indidual animal that is uh coming to the FED bunk we then compute these metric which are uh total eting time visit duration and so on so forth and if you look the correlation of the I SCP between predicted and observed here pretty High showing that models are very um has a has a good performance and and can work quite well for behavior and this oops this is an example um let me this is an example here with not looking to individual animal but looking to a group of animals so this are you know all the animals that are eating lying standing and drinking um in the pen so here you're looking to the whole pen and not your individual animal which may may have uh value um as well um by monitoring the management strategy or the man management being applied at the at the pay level here's another example of things we are interest we interested in monitoring respiration rate using computer vision so we have a camera that automatically detect the flank of the animal so you can see this flank going up and down up and down and then this will search for another animal here and then get this flank going up and down as you can see this signal here is the signal of the respiration rate so this raw signal is being converted to a frequency domain so there's a lot of um uh some uh mathematical uh functions being implemented here that will convert this to a frequency and then we count the Peaks and we with then determine uh what's the respiration rate of individual animal using a single camera so here is the correlation between the predicted uh breaths per minute and observed so you can see the respiration rates predicted and observed so very good prediction here you have infrared and RGB basically this is night image oops let me put this back this is night image so that measuring respiration rate in the dark with the night vision of the camera and this is along the day with RGB which means like doing the color regular picture and this is their correlation between predicted and observed uh respiration rates and so the last example I want to show you um with the computer vision for management is using the computer vision for detecting lameness or Mobility um problems at the farm and this is a big cause of economic loss in the data industry uh it annually is over 11 billion dollar and uh globally and and so so the total cost can go up to 177 per Cas and if you look to the worldwide prevalence um it's about 22% so one lame cow um know in every five and so the way the mobility score would be um applied it's by watching this cows walk and then look uh to some characteristics of the of the way the cow is walking or the mobility of the cow and then you can sign a score from 0er to three where the zero is a good mobility and three is a severely impaired mobility this is really hard to do at the farm because you need to be there watching cows and tracking cows and we know um this is really hard to be implemented uh systematically so some technologies have me sorry some technology have been U proposed as pressure mapping system where the cow is walking and then based on the pressure the cow is applying to the map uh to the m then you know if this cow has a has a problem or not or you can attach for example markers for motion track so here you can see some um markers in this cow and then new track this is specific marks but this is Libor intensive is timeconsuming it's really hard to apply these uh in real life at the farm so what we did here was we train a Model A computer vision model using the camera system to detect 25 key points on this cow so nose head neck back tail head tail all the joints of the animal that we could see and then we train the model that automatically place these key points we used 9,000 image was a large data set and the performance of the model was very good so here is the eror uh thean distance which measure the are between the predicted point and the original point which are you know eight pixels distance so it's it's very close so basically the model will do this we place these key points representing the skeleton of the carow or the joints of the carow for this particular key points and then we can measure we can extract after plugging these key points we can extract for example in a segment of video head bobing which is the vertical movement of the head head position which is the vertical distance between the height of the head and the the wi of the C we can get stride length we can get tracking up we can get all these angles uh of the joints like back angle elbow joint angle uh carpus joint angle and so on okay and then by extract this from the video we then classify uh the mobility score so we had 2004 204 cows uh and then we collect 2004 video clips of each individual cow we had we then classify the Cs we score good 0 one two and three and we had to combine the score two and three because we have very few cases of score three so two and three was unfortunately we had to combine those and then I won't go in detail how we did all the training validation but we were very careful on not having data leakage in terms of having the same count the training set on the test in set of things like this uh we try to be as independent as possible and I can go in detail here later that's necessary so we found uh an accuracy to classify I score 0er of 83% uh score 174 uh. n and two and three uh Mobility scores 83.2% so when you look the specificity that we good the sensitivity was a little low for score Mobility score one was 60 close to 60% and the reason is is really hard to to get this one mobility score because it's almost like a health cow so the mot had a little trouble uh discriminating these as as a sick animal but overall the performance of the animal was was was very good very impressive here's an illustration of a carow with Mobility score zero consider it zero with a good mobility and here's the cow walking and that's what the model is doing the back end is basically placing this and measuring head position head bobbing and all the uh features okay um let me so his Mobility score one which is as I said is difficult to to to to differentiate from the zero his Mobility score two so you can see r a c that's not working uh very well and you see um know head position head bobing much higher uh compared to a c that was healthier that was healthy classified as zero and this a Mobility score three U and the C head bobbing is much higher back back curvature uh changed U compared to the other ones and here you can see for example head Bob 4.8 in the first scow these was 0.5 so you can see it's a big change and this being captured by the system the problem uh is you know this side view will create a lot of occlusion so you have multiple cows walking and blocking each other other so we won't be able to see all the cows so this is a limitation of the side view and cows they they don't walk straight that that's something you know that we we learn um and especially uh the guys with more uh computational skills in the lab um they were somehow frustrated when they they saw that but also they are very smart guys and they came up with a new solution say okay let's do top down View and with top down view we don't have occlusion and we don't have have interaction with the animal and the device and so now let's map seven key points in the back of the cow and as the cow moves we track these key points and we do the same but now with the top down view so they they pretty smar guys and so that's what we did here we then map these key points and we start collecting the neck angle back angle hip angle but from a different perspective and then you use these features to to them classify Mobility score so so here before I play the video I want to show you this is a good Mobility score a zero Mobility score that's a that this is the x coordinate in the image that's the y coordinate in the image so you see cows walking very straight in all seven key points and then when the cow has a bad Mobility score score three you see that these key points will build some sort of wave which will be the cow moving the ramp quite a lot because of The Locomotion problem so I will show you this this top graphs here so here is the car walking very quick and straight so walking speed is actually um very important and here is the cow uh look that the ramp of the cow here this region will go left hand side left hand side and and this lateral movement is creating these waves that you see in this graph while the healthier carow Mobility score zero the carow will walk more as a straight line so I can just display that back and you see here so here's the the good cow Mobility score zero and then you see in the sequence uh the cow with a lat lateral movement of the ramp that creates those waves and these are probably the the best predictors we found so performance of these models were very similar to the side view as you look accuracy off score zero one and two and three and again difficult to classify score one but very good to classify score two and three um and performed really well uh compared to the side view which is very very interesting and very promising because now we can do this in large scale we can scan thousands of cows and then classify the score the mobility score of these cows and as I mentioned the back lateral lateral oops the back lateral movement was the most important variable uh in the model and was clear in the video that that was the case so as a final consideration uh you know we have seen that digital technology are are crucial to collect cheaper precise realtime phenotypes um collecting animal live information will be extremely important to be integrated with economics feed management to be used by geneticist for genetic Improvement I have collaborated with geneticist and I know they are very interested on the animal La information that can be collected large scale um we definitely definitely should leverage AI systems uh in there in beef so we we can not only answer uh new questions but also uh some old older uh questions that will never answer before because we're not have uh we're not able to measure some of the things we can measure now uh and and this is a very nice area um there is a lot of interest of this new generation of students and and professionals that want to learn about the technology and use the technology to address uh biological questions related to animal growth Animal Health genetics and and this been a a very uh pleasured uh place to be and to work area to be in working out I would like to acknowledge USGA for funding most of these tries that are showed their Innovation Hub uh data Science Institute Warf hrle Computing have used a lot of computational resource out there Microsoft for funding um research in our in our lab as well and of course these amazing group uh these are the guys developing all all the trials I presented today so a big thank you to to them and with that um I'll be happy to answer questions thank you so much yes thank you um wow what a what a great presentation and and so in insightful indeed um now we're going to open up uh a short time for questions yeah you want me to jump in here Angie y that sounds great all right so um first question in the Q&A um thanks uh you make technology sound so easy do you have any plans to check cow behavior in holding areas related to the use of crowd Gates and Welfare yeah that's that's a very is that a good point I remember talking to um Dr Jennifer Vos and Dr Katie um we have a student working with robotic in systems and there was this discussion about you know looking to for example uh you know I'm I'm taking your question to to automatic mking systems and looking for know how animals are waiting for all animals inside the robot and this could be used for any other uh regions where you have you know uh crowded space and animal most with problems related to welfare in holding PS or in holding areas um and and also to check behavior for high stocking density uh could also be used we have not done uh to directly answer your question uh research on that particular problem but there is a you know tremendous opportunity there for sure oh good question and um definitely a lot of possibilities right uh going back to maybe closer to the beginning of your presentation uh a couple questions on um with identifying The Animals by the color pattern are you able to pair that with Eid readers as well um and we'll start with that um and then a couple follow-up questions related to that yes so yeah of course I mean the rid is still the most used you know identification technology uh combining this with the camera it can definitely uh improve reliability of the system it could be make the system even more robust I guess um what we're trying to accomplish in the tries that I demonstrated was uh how would be the performance without any other sensors informing us about the idea of the animal and completely using uh computer vision and not any other thing and the reason we try to do that is that as you add and combine more technology you can make systems more robust but also you create other opportunities for failure because now we have two technologies being combined and if you want if you know if you you know it's it's just another layer to manage uh at the farm it creates more robustness in terms of if one fail you have the other one if the other one you have the other one but connecting those and making them to work together uh sometimes it creates another layer but I agree that know RFID is the most user and in our case uh computer vision was the attempt to to see how these would perform if I only have that technology out there no that makes sense I think in related to that um do you have plans or thoughts on how this might be integrated with uh current herd recordkeeping software um and also a question on U herd size is this um is there any scaling to this or all herd sizes should be looking at something like this yeah that's a very good question and that they come very often um related to you know is the small farmer able to use that um the the camera system and and the how we use those uh in my opinion for the examples I showed here they it doesn't really matter uh the the her size you know if you have 30 cows 100 cows uh you may just not need some of this technology because you're able to watch all of them and know all of them and so you may don't want to pay for them but then if you have 300 cows or 500 C cows you not be able to look at those cows individually and then there is no difference from 300 cows that you cannot watch individually and 3,000 cows that you can also not use watch individually and if you have a camera install at the a of the paror uh you know these will work for these 300 cows or these 3,000 cows of course you're going to dilute more the cost of investment if you have 3,000 cows because you still have two lanes where the cows will leave The Parlor now at some point someone with More Cows you need to buy more cameras and the cost will scale up for More Cows too and so it it's I guess it depends the technolog but overall uh the computer vision because you have one uh camera the monitor multiple cows it's usually um um how can I say that um is usually positive for smaller and and larger uh Farms um in terms of adoption but is a good question this is something we have a grant right now we evaluating we are investigating U the economic impact of this technology uh the capacity of them to be scaled and also if the which one would be a good Feit for larger or smaller but it's a difficult answer um to address for all technology but for the computer vision particularly I see positive adoption for small farms and for large Farms no obviously you've had some time to think about this and see see some of these uh questions other presentations but it yeah it's really interesting so this one I'm going to read closely because it's uh very specific to some of the cow Health monitoring uh that you previously talked about uh so two questions is there a reason BHB cutoff was at one and two did the prediction work to find cows above the cutof point but you you consider also postpartum performance uh milk production reproductive performance after the fact yes H very good question yes so there was a reason uh we initially we want to use 1.2 I guess probably the
most uh common threshold cut off report in the literature 1.2 Mill mes the problem is uh we have about 100 cows in this trial was a very timec consuming trial because we had to collect blood samples every other day and plus a lot of other things and we couldn't do that in in a in a much larger scale than we have done and what happened that that we did not use 1.2 is that as we use 1.2 the number of cows above the threshold was not super high uh which means that the model would be super unbalanced to between training so for example I would probably I don't know the numers top my head but let's say if I have 100 cows I would have maybe 80 cows uh consider health and only 20 above 1.2 let's put this way I'm not I'm not this is not the number I don't remember but uh when I say unbalance is very few cars uh above the thresold and because 1.0 is also used as a threshold as a cut off we use 1.0 then we had more cows above the stret so the model was more balanced to be trained and it became a more conservative model because you know a account may be 1.0
and not 1.2 may not you know be considered so clinical Kos for people using 1.2 as a cut off and so you you can look at as a more conservative model that will flag a cow that is like you know in this borderline a little bit about 1.0 and so but the main reason was uh if we use 1.2 we have very few CS 1.0
make the model more balanced and so for training the model and testing these hypotheses uh and because 1.0 is accepted then we adopt 1.0 and your second question is related to if you consider postp performance not for the we only use postpartum performance for the cows for previous liation right so we we we didn't want to use uh the performance uh of the cow was pattern because we are using all the variables to predict the the subclinical Kos pre pattern so then that's the reason so that's why I could not use the postart because all the variables should be obtain prepattern now if the performance was related to previous licitation then it was part of the prepattern uh inputs and then we used uh some of this information in the model yes which we called C history but was the information from previous lactation including healthy events no good thank you a general question that came up a couple times throughout um do you have rediction or sense of how close to commercially available a technology like this would be um obviously the algorithms are proprietary how that process might work to make this available on a wider scale yeah that's a that's a good question too I guess that is uh you know a lot of interest in the indust the industry is trying to develop uh Technologies on that space um sometimes they are kind of protective you know from how they want to collaborate or develop or cor develop um what we do uh here at at the university is we do our research we try to address the questions we have we develop the technology some of these technology get patented by Warf um which is the Wisconsin Research Foundation uh on campus uh some of this technology they're not interest on patent we always publish the the the trials we have so this is all out there and so the industry and companies they can get information they can get how the models were developer or training how implement the technology these are all in the papers and so they can get these and convert these into a commercial product and and and make progress but these will be way more on the hands of the industry than the Academia to convert these into products and and and to basically put this to work at the FI um I I that's my my vision well this was great um Dr Doria the to think of all the possibilities and how the difference of what our um cow eyes tell us um when we're looking at animal behavior and what the cameras show and the the just the natural um way cows you know progress through their lives and you would have that snapshot it's very interesting and um with no other further questions I would like to remind all the attendees that we did record this and um we will have it out uh to everyone that registered within a week's time um and then as always if there's any other questions that you can think of or that you'd like to ask uh please um contact either of us or um Dr joal um and we'll get you the answers um so I hope you enjoyed today's webinar I'd like to invite everyone um next month on August 20th for kle need fiber 2 storage and feeding tips to to minimize nutrient loss we're going to have two speakers next uh month uh Dr Louise and then also uh Jackie mccarville who is a regional Dairy educator and they're going to talk a little bit about um the potential cost of dry matter and nutrient losses occurring during silage um and strategies to mitigate that issue so uh that's more of a nutrition topic uh next month and then as always if you ever are looking for any information or unbiased University based research um please uh take a look at our extension Dairy program on the web or find us on Facebook at extension agriculture so with that thank you everyone for attending thank you very much thank you again R Ryan Angie for for the invitation thank you all thank you yeah thank you great job
2024-08-12 15:30