Learning in Data Scarce Visual and Multimodal Applications Using Vectorized
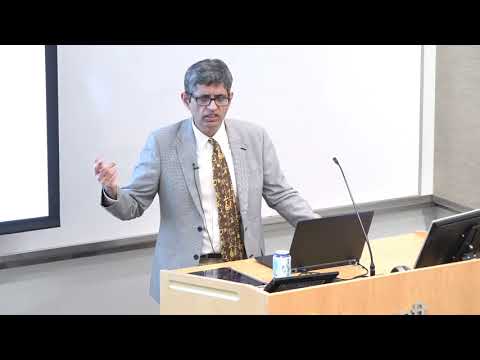
You. So. It's. Great to have a, JD Walker in India from, SR, I in, Princeton, New Jersey. RJ, will give, a talk on I, guess some, of the recent work they've been doing with the. U-dub, and some. Other universities. On. Vision. And language and. So. RJ is the head of, vision. And learning at SR. I he. Did his PhD at RPI, and then. He went to I think. That was in the days of the startup business, so he he did his wavelet, compression stuff. Went. To a start-up and then got, disillusioned and then. Went to Merle in Cambridge. And. Then he came to SR I in 2008. And, since then he's been there and I. Remember, IJ. Was the first one at SII, to, start. A, completely. New thread on human. Understanding what, we call human understanding, in. Hololens, and, here. And I. Still remember that you. Know us had just gone in not, just but for a few years they had been in Iraq US Army and, one of the biggest problems they used to face is they they, knew how to fight they, didn't know how to deal with civilians, and that was, the major, problem in Iraq so, DARPA started, a project called good strangers, how, can you train people to, be, to, be good strangers. You know in a strange land so, AJ was the first one who went in this concept, to DARPA and said. You know we'll mount connects. And other sensors, and we'll, have a feedback loop where. There, is some scenarios, being played in virtual reality and people, start interacting and, that was that became a hit really. He built a system with connects, and other. Kinds of sensors and people would get trained in doing. Good strangers, and I think since then he's. Continued, and he also has worked on many many things. Both at sra and she used to be a GE and, great. To have you here so a JD Walker. Thank. You very much for, that. Kind. Introduction, I should, point out that I. Prepared. Me into a Sarah and he was my, direct. Supervisor, there, for a very. Long time. So. I'll, start. Off by first talking and just a little bit about SSRI. And. In particular the, scent. Of a vision technologies. Then, I will commerce cover some of our work on applications. Of joint multimodal. Embeddings, and then. I'll hand it over to you who will describe to you some of our work on using, a. Graph, based modeling, of activity. So. So. Asri is a non-profit, research institute, the. Business model is contract, research. The. Clients. Are primarily, government, but a. Reasonable, number. Of. Private clients or commercial. Clients also the. Model being that. You. Incubate. The technology, with government, funding and then over time transition, it to commercial, activity. I, guess. These statistics. Are interesting, in the sense that about half, of the company consists, of people who have at least a master's so, it's definitely, a bit of a PhD. Heavy organization, but. You see that the other half is not which means that, there's an emphasis on. Development. Or advanced prototype, so rather than just research. Pure. Research and there. Is all this you. Know these are all the, startups, that I come out the most famous one I guess is Siri, the. IPhone. So. Now we're in the information in computing Sciences Division it has four labs and, it is you can say it's a sort of microcosm. Of a Sarah at large very similar composition, of, skills. And, so. Forth and. I'll. Jump, directly to the Center for vision technology, so one big. Claim to fame we have is that the ten-yard line in four always developed so. This hadith has himself presented, this slide, many times so it, kind of feels strange showing it to, you folks and. We. Have about 80 plus people this number might be off a thing has gone back up now some. 87. 86, or some yeah. And. So. Now, currently. The, work, you. Know here are some examples, so one is the GPS denied. Navigation. Activity, augmented, reality and.
Then At the bottom. Bottom. Right is the human behavior modeling that had proposed referring, to so, it started, off with that project, and, it. Has now been parlayed, into the, driver behavior modeling, for Toyota's concept, car, so. It will be featured in at, the 2020, Olympics. As part of Toyotas. So. And, then on the left there we'd reduced. You know typical. Video retrieval, which to this crowd you know I don't have to. Explain. This is another way of looking at the. Technologies. So intelligent, there. Are some cat technology. Under intelligent mobile, platforms. Human. Understanding human interaction, and then. Multimodal. Data analytics, and machine learning what's, happening is that in the process of developing these technologies, we are also developing, certain. Core machine learning techniques and offline I'll be happy. To engage with you on those so this is the augs. And. Let's. See yeah so. There. Are four managers, here, who. Report, to me and so. Mohammed, amar does some I'll just give you some sample project, so he does joined computer humans storytelling. Under. A DARPA program so. That's why he likes to call it creative, AI, Amir. Works. On the human behavior understanding. Which in which is. Working. On both driver behavior and also on human-computer. Dialogue. Management. He. Works on multi. Modal analytics, both, of, ground. Imagery as well as overhead imagery. We work for government client, imagery is an important, part of what we do and Nick, is a systems, person. Now. What, I will do is just, play. Since you, know we mentioned. No. No the the, video over. Here where I'll just just. Show these couple of videos so this, is our. You. Know this. Is the driver behavior console. And so. I just wanted to give you a flavor of how, so. The main challenge in this kind of problem really is the tremendous lighting, variation, that. The. Auto, environment. Offers you that's. The biggest that's the absolute biggest challenge, right because. The variation. Is just immense and, so Amira's developed pretty strong I mean, what we have is way beyond this but it's not ready for public release, and. Then on the other side of this is the. The. Full-body behavior, which is what we developed in that project that I picked was referring to which is you know we are looking at facial expressions, gears posture. Gestures. And also. Speech tone so, we do some amount of audio also in this lab and you. Will see later we do some text and then. We are also interested, in interaction.
Between Individuals, so for example on the Left we have looked at children doing pair programming, on, the, right people building, a tower playing, at our game together so this was all done that. Stuff was published at the learning and knowledge conference, this was published at IDC me. For. Example so. In addition to the real-time work that I showed. You this. Will this. Part of the talk zeroes. In on our social media analytics work. So. This, is something I do with my young colleague Karen, chica this is like. Of. Those four groups this is yet another group where basically, just the two of us, and. So. Are, interested, in social media analytics from the government, side comes from detecting. People, who are trying to recruit for. Extremist, causes, right. And so we look for extremist, content, extremist, behavior and, so forth that's, why the government is interested and the. Key is that these platforms have, two things that make them really stand out one is the sheer volume. And. The. Other is the. Complete. Lack of. Constraints. Meaning, that people can put whatever content, they like people even may put a day's, worth of Fitbit data on social, media anything. Goes, right, so, it's truly multimodal, truly, vast and, so. What it does is that it stretches the boundaries of whatever standard, problems you want to solve you're, going to solve a much, more. Magnified version. Of, that problem so that is sort. Of by. You know in the way of, motivation. And platforms. That we have looked at include, Twitter Instagram. We. Have put Facebook over there but no we don't have access to Facebook, but and, surprisingly, YouTube, functions, as a social, medium. The. Next time you go to YouTube just, keep. This observation in mind now, the problem, that we were interested in was modeling, content, reaction, so, given a piece of content, who would be interested, in it. And. Given. A group of users what kind of content would they be interested in we. Were interested in this problem because if you are trying to track extremist, activity, when you see extremist, content, you, should know which group is going to be triggered by this kind of content. Right so, that now obviously, on the commercial, side it has an application to advertising. And so forth but. For. Now, so. So. More on the technical side then what we are interested in is taking. The, modalities, of speech of, text, and images, and then. Projecting them into a common multi modal semantic, embedding, space so. Basically you can say this is a multi modal generalization. Of embedding, such as word to back you can just see that that's pretty much almost exactly, what's happening there that. You are you. Have paired data and, the, pairing is what is important, so, now why we are interested, in applying it to social media is that this is a highly unsupervised. Kind of technique so. In social media that is good because getting, labeled social, media data is a you.
Know Basically not possible. And. The, social media has in and pairing so. Those. Are two things that give. Us encourage, us to. So. Now there are some research questions associated, with. Multimodal. Embedding, so. You. Have seen work. I'm sure on zero short learning on captioning, visual, question on selling learning, better word. Embeddings, and so forth so. Now. Our interest, in this came from the social media analytics so. We were interested in, first, of all seeing if we could push 0 short learning in a new direction. And we, were also, interested. In, finding. Out if you could embed the users and the content, in the same space so treat users as an additional, modality, and then. Have a three-way embedding, so. That I could have seamless three-way, retrieval, between all of those modalities so. That, led. Us to first, this problem, called 0 short object detection, so. This, is something we published at ECC. 2018. This, came out of a. Project. That we started as summer intern, under the DARPA metaphor, project, and. What. We are interested in here is the not. Merely object. Classification, we want to also localize. The. Object, and it should be previously, unseen. So. I'll. Just flip through these in the interests. Of time this is just some background. Material. Right. That. When. You want to this. Is basically saying that there. Are these existing, techniques like yolo faster, our CN n SSD. Etcetera, but. Scaling. Beyond, a few hundred objects and detecting, completely, new objects, still beyond the reach of these kinds of techniques, so. Now. What is zero short learning so. Let's. You, know I'm going to state the obvious just, so at least we have common terminology, so. The. Idea is that you learn ik learn from scene classes, but then you predict. On unseen, classes, so that is the, can say a good, definition of. Of. Zero short classification. So then the question is what is zero short detection, so. This illustration, is telling you what that is our. Detection, is that in addition to saying, that there is a cat in. The picture you are also saying exactly where the cat is are exactly where the dog is right, and so, now. And now you're going to do this in a zero shot, fashion. Now. All. The other problems such as occlusion, viewpoint clutter etcetera, still come. In play. There. Are some certainly. Obvious applications, like robotics, and, surveillance. And. The. Question is do we really need thousands, or training examples, to deal with a new category and our. Answer. Based on what we have looked at is no so. This is just a sketch of our, approach, to this problem so. What, are some. You. Know so first of all we are we, want to take previous, work in zero short, learning we don't want to create something out of whole cloth.
But. Then the task is how do we model. The background, so. What happens is that when you have all only its previously, seen objects, then the definition of background becomes very clear because. It is, whatever. Is not in. The class of seen objects is the background the, moment you have objects that you've never seen before, now. There is a new class so there is the typical background which, you could call a stuffed class like, sky or, wall. Or water. You know right. Etc but. Now you have a class of objects, that you are not seen before and that may also merge into the background, so, that presents, a bit of a challenge and you, will, now, see the how we have. Dealt with this. Kind of problem as we go forward and then. What we have also done is that. You. Know you will never have enough training data that is for sure for any problem, of this nature, just. Because it is so. Big. In scale so, what. We. Have done is you explored this business of dense sampling, of the semantic, space so wherever you are, certain. Of, what. You know what you do is that you try to sample close. To that and make so, even though you don't have label, data but. You try to sample over there and try to make the embedding very dense around, that particular. Location. So. Now. What is our baseline method, we use this our CNN, architecture, but that's, really not the point I mean you know you could use any. Other. Architecture. Also for this kind of method, and. We are just showing you some sort. Of you. Can say already. Some result where what we have done is we carried out training. On the scene classes, and then. When we trust test. On the unseen classes, so shoulder. And skirt were part of the set, of unseen classes, and you, see that this actually does in fact detect. The. Skirt and the shoulder, presumably. Presumably. Because in, the world embeddings. There. Was enough similarity, seen between say skirt and shirt. Or you know proximity, between short and shoulder, and so forth and then, there was some joint learning, or the visual attributes as well which, managed. To give you this kind of result. Embedding. Space yes. All these, concepts, will be nearest, neighbors versus, other concepts, that's right so the idea is that when, you're trained is embedding, what we are trying to do is you are trying to pull similar things together and, you are trying to pull the similar things apart so, you are depending, very heavily on the pairing if it, did not have paired data the embedding would not work. What. Is the testing scenario, like like in object detection. It's. Basically bombing. Boxes, being. In. This case when you say testing on unseen classes. Is. It exactly the same yeah. There. Were born boxes and being labeled that's, right there boundary basa there being labeled and then you say that okay I was able to see that there's a skirt there even though it was not part of I didn't, train on skirts now. On the lighter side. You know at. Another. Place where I gave this talk this young lady in the audience pointed. Out that that's not a skirt that's a dress so. So. Yes so what I always says, that that's a reflection on the gender composition of, the people who made this algorithm. And. The, sizes of the body box are basically, whatever these networks, generate, yes. That's. Right. And. Back of boxes, right so there is no structural, information embedded. Into our system. Right now so, what would prevent let's, say shoulder, getting a dress. No. That is very true. You, know so. Partly. With. These deep learning systems you can come only so close to giving some kind of a, an. Intuitive, answer after the fact I could try to tell you that. Somehow, you know this, fabric, need to be a bit floppy and you. Know I mean overall the nature of the relationship. In fabric, and skin with. Lower body or tire is different from upper body attire so, if. You have enough of training data that kind of thing will be learnt, but. Having, said that I'm not telling you that that is the answer to the question it's it's. A it's. An educated guess I would, say frankly yeah. So, that's the general idea and now here are some.
Mathematical. Details. Here that what you are doing is your first. You. Know you you first trained. The. Embedding. You. Know at once and now you are projecting it using the linear projection and, then. What you are asking me right you are taking, the G box and then comparing, it to the label so basically it is a box by box. Result. As, it was right and then. What you are doing is you are pushing, embedding, for similar. Boxes, and class labels, your time random, together and so. Forth so. Now. The. Interesting part. Comes in which is one of the innovations, in this approach which is what allowed the, solution, to the problem, so. What. Detection. Model is with a fixed number of classes do is that they will add an additional background, class, and. That's like some kind of container class that just takes care of whatever is not in the same classes this, is not like that so when we try to. Just. Use a single, background. Class. We. End up not getting very good results in, certain cases and the, reason, for that is that. These. Previously. Unseen objects, merged into the background. So. They get classified as background and they are not seen at all so. What. We are done basically is come up with a way. That. Assigns. Latent, classes, it allows us latent, classes to emerge so we these are not labeled. But. What you do is you make a distinction, between background. That are background. Areas. That are just stuff and background. Areas that actually. Correspond, to some, latent. Classes, that may or may not correspond. To previously, unseen, objects. So. Once. You do this. Kind of a thing we find that, we. Are in fact able to. Get. Better results and now in the densely embedded, space what we are doing is. You. Know the the issue is that you have. This. Embedding, space can be very sparse, you have no control over the, density, and we. All know that any kind of interpolation method, will work well if it is being done in a dense region, you want the points to be dense so, what we are done over here is basically we augmented, the training, data set with. Just additional. Classes where. We are no there. Is no overlap with the unseen classes we you know do our best to not cheat and. But. Then what you are trying to do basically is you are trying to take whatever, areas you know and you, want to pack them more data so that those areas become. Denser. So. Now. What we have done is that we have worked. With the Miss cocoa and visual genome data set and now. We did this split right where with. MS cocoa we had total. Of 65, so. 48 seen and, 79, say no visual genome, has. More classes, in it and we. See an immediate. Consequence. Of this now. These. Are some more details but I think in the interest of time I can skip some of these details but the. Idea basically is that. You. Know this. Is sort of you can say our experimental, framework this is the. This. Is the framework that we are using to run these tests, and. So. What happens is that for, the visual genome having, this kind of procedure. Where you are letting these latent classes immerse in the background, actually. Helps, and you get the best results, that is. Not quite evident, in the MS. Cocoa case and that is our. Theory. Is that that is because in visual genome you have a larger number of objects. Presumably. When you have a larger number of objects to present a greater challenge and that is when, some. Benefit, is seen, for. This kind of method of. Having. A latent. Background. Classes, and so forth. And. So here is some more now, there. Are some good classes, and there are some bad classes, and these are obviously. Obviously. You. Know you all agree with me that mistakes, are more interesting, than what you get right and so. When you look at this so. You see for example over here that it gets zebra, wrong and later on you will see, you. Know it persistently. Called zebras on this just somehow, does not, recognize. Zebras, right whereas. It does is saw you saw that it is able to detect things like skirts, and buildings and, cakes. Chairs so forth so. Yeah. There's a little more detail but I'll skip ahead to some visual. Results, so. The. Result most of these results are as expected, I mean for example this R as a result, is fairly obvious uh-huh. You know it is picking up a bus my. Favorite. Result is this one I had to, you. Know I made, a double-take with this one I first thought it was a dog, myself. But. It's actually a cat but, this system thinks it's a dog but. That's good I mean you know. You. Know Hong. Joo young Zhang used to be with Microsoft, and he once told me that when, your, system makes a mistake you failed it okay it's real it's not. So. This is a fascinating mistake, in the sense that I think I think that is the pointy ears, that. Somehow this thing is learning and. It, thinks that it's a dog right, but. For. The most part it's not bad at all is actually getting a lot of these things. Now. Here you see. There. Are you, add this question right look it made exactly, that mistake see if you think the XS skirt, it. Saw the fabric and skin boundary, and it thinks it's a skirt but it's not right. So it does get those things wrong because probably.
Because It the thing that you pointed out there, is no, structural. Information I captured. Now. It persistently. Thinks that you, know it has found cattle, over here. At. Some level, this. Is reassuring, in, fact later on you, will see we have tried some matrix where what I. Encourage. My folks to think about was that you. Maybe you think of an ontology and try to look for similarity. One level above so, problem, is taking a zebra for cattle is not as bad as mistaking. Zebra, for doorknob. So, that's a much more serious mistake. So. There are levels at which you can test how well this. Kind of technique is working. No. But. In cases where they were overlapping, in the two datasets. But, but. Some other domain would be very different right like, let's say the cats to, my knowledge no mine. All is not but I'd have to go back and check them. If. I allow, a few, oh, right. Right right right so, that's a very good question I have wrestled with that all the time I work with machine learning and what, I found is that, it's. It's kind of hard to say I mean he, did try current, tried some top cat type experiments, right very, so. There are there we did get some results. Like. That back. There yeah. Possible. That you. Know what, are you calling unseen, concepts, yes what you saying unseen is in the visual domain but they are there, in the X domain right absolutely. So they are not unread, they are unseen, but they are not undressed. Much. At all in. Which. Case in, which case it goes off course like this no wonder it's still fascinating, that it's somehow thought it was cattle, but. Again I won't read too much into that who knows you know what it, you. Know what it let's don't do we don't necessarily know. And. This, actually gives us the cue to ask another questions, how do you judge the success of an embedding, because that's our next problem here, because. We start off with this multimodal embedding for social media now and the. Reason we are interested, in an embedding, is that we want to have we want to solve the problem I mention earlier which is that given. A piece of content, who would be interested, in this content given. A user what, kind of content, would they be interested we are interested, in solving, that problem and so. Yes, we can certainly if we had a joint embedding, that would be very nice and. Now. Why are we interested in this kind of thing specifically, there's. Another reason also like I was telling you earlier there. Is absolutely no constraint, in social, media prep, solutely, think and pop up and so you need to deal with that so, it's a much harsher. Environment. Than. Relatively. Domesticated. Environments such as I miss Coco or. Vgg. So. What. So that's what we are interested in over here and. So. We. Come up with. This. Kind of. Embedding. Technique where what we are doing is we are using. Images. Posted by the user, as a stand-in, for the user so we really, are not you. Know obviously. I have to take some user attribute, and use, that as a stand-in, for the embedding, you don't literally embed, the, user.
So, In this case what we are doing is we are just taking the images that the user has posted, and then. Embedding. Those so we are using the same framework as we did earlier but, now we are attaching. This user label, with that image so that we are maintaining it as a. Separate. Modality, and. So. Now. I'll. Play you a video of this, demo, so now what this allows you to do is carry out a four-way, retrieval, so what we have is a so. We have text we have users, we have images and then, we have also done, some, unsupervised. Clustering of, the users, right, and so we have user clusters, also again. What we do is that we use an image as a stand-in for the user cluster, and, we. Are able to embed user clusters, also in the same framework so, what this allows you to do therefore is that you can retrieve based on text you can retrieve, the other three modalities. Right. So, so. What has it typed over. There. I cannot read the thing too small yeah it says Amazon, rainforest. And so you get some images related. To that you get the users, who are interested. In that kind of topic and so forth then you can go all over the place I play you that we do in a moment and. So. There. Is there are some details, here on this slide on how the, embedding. What kind of metric are being used and so forth now, what we are done here is, we. Have taken one month or Twitter data right. And we wanted all this to unsupervised. So what we did was we collected, one month or Twitter data and, we. Took the, top. Few, hashtags. Right. So. We just chose some arbitrary, numbers if we want because we wanted, some we. Wanted some density to the data you know if there's a hashtag with just one entry that's going, to come in and just sit there right so we, got that so. We ended up with about. 10. To 12 million tweets about. A million, images and 40,000. Users these are months worth of data. And. It's. Surprising, what this kind of thing actually ends, up learning. Now. This. Is where we carry out the. Kind of experiments, that you were talking about where what we do is we use ranking, and we try to figure out well you. Know whether the rank makes sense or not and just as a sanity check so. We first test only with text, only with the images or only with users, and we find we're at least much better than random. And, then. As we start adding. Modalities. As we start pairing them up you see that the, rank. Starts to improve and then. Here are some results with. With. Other with. Other variations. And. This. Is I mean. This is in general it's a. Bit. Of an interesting problem, that you know exactly how do you how. Do you evaluate an embedding, right, how. Do you believe in embedding, how do you know what it is learnt and all that so our operational. Definition, is that if for our task it gives results that make sense then. We. Believe this embedding, and and that's you know, we. Sort of have a restricted definition of the. Efficacy. Of the. Now. You see that we have played around with different kind of things right you can use it with glove which is pre trained on a very large corpus, or you, can limit it because. There are some interesting aspects, on the text side also the, interesting aspects, are, that. The kind of language you see in social media is very different, from, what you see in the, formal, document, that things like glove and were Tuvok have. Been trained on so, lots of informal, language lots, of these so-called stop, words. So. You have to do some cleaning processing. And all that so. There is that on, on that side of things. And. So, here, there. Are some more detailed.
Results. Where the joint model, you know are. You using a GRU, instead of convolutional. Excuse. Me. Network. And so forth. The. User clusters, that I showed you what we do is that those. Are unsupervised, but we find that interestingly these, clusters, actually, seem, to make some sort of semantic. Sense for example this seems to be around. You. Know British. Politics. This. Box over here. The. Other ones we have put some images so we see soccer images, and, soccer, related, themes are, sort. Of falling together and so forth and. So. Basically. What. We are doing right now is, we, are trying. To write all this up and then. The. The, easy CV part of course is done now but this part with the social media this will, we. Are waiting for the right kind of forum to publish. This what. I will do is I will quickly. Play. You a video, and then. I'll hand it over to you to talk to you about. So. Now you. See this is the so I already told you about this data this is one month or Twitter data and so forth and now, see types in over there heal, type in the box. She, types in say. Vegan food so many types in vegan food with, the embedding he gets this retrieval, so, it's interesting about one month or Twitter seems to have images related, to vegan photos. Use. Labels, associated. With images when their meetings are created. Yeah. Basically. Captions. Yeah. Caption. Sometimes, it's loose also, if there's no caption, but there is a some. Kind of text associated with that tweet, that's. The difficulty. With this kind of data but now you see that it is doing retrieval. All over the place right retail starting, from the image side. Writable. Again starting on the tech side but also from the user side or the user cluster side so. We. Are done this kind of work where we are interested, only in rabble-rousing, video, we've published at ICM i but this is, a generalization. Of that work now you have a general. Content. Model, which. Allows you to predict, given. A piece of content who would be, interested. In it so. What. I'll do is that I. Will. Pause over. Here, and I, can certainly take a couple of questions and let me. The. Surprising, results are the kinds of topics that pop up for example we typed in fashion, not, quite knowing what to expect anyway, very surprised, you, type in some designer or something the. Breadth, of topics is a big surprise with this because. There is no way for us to actually prove that there, is no easy. Way for us to probe. We're, yeah. So. I'll. Hand it over to you she'll tell you about. Okay. Next. I will switch, to gear a little bit talking about some, of the video analysis. Work. We have done so, this is also included, in the multimedia because video is one, source of the data. Format. So. In, here we just use action, segmentation, as our task this is, a slide to show that.
Action Segmentation. Needs, to be done at different. Granularity. Levels, and. To segment, long, videos, when. To do inference over long temporal. Spell, in, order to include the, dependencies. Causalities. And also, some temporal, ordering of the actions, but, at at the same time we, need to provide sufficient details. To. Differentiate. Two similar, actions, even, at very short temporal. Span so. To, support, these two requirements. Actually. We started to work on this graph, based a representation, so, we cut the activity, object. Attribute. Graph so, basically, it has two levels on the, top we have the record activity graph, so, it treats traces, multiple, threads of activities, so, this, level we can do inference. Over long temporal. Span to. Include. Causality. Dependencies. And ordering. Of the actions, on. The bottom level we have the object, attribute. Graph this. One we have detailed, information, about the objects. Associated. With the activity, or action as. Well as their attributes. Status. Or status, changes. So. With this level with. This amount of detailed, information, we. Can differentiate. Two, similar. Actions, and also. In our, object. Attributes, graph as I mentioned we also check the status change, of the objects. Or even, the actor in, this way we can not. Only infer. The, explicit. Observations. But also implicit, consequences. So, this is important, for some. Applications. Where the observations, are noisy, we have mister detections or even, sometimes we don't have the observation, at all because of the non. Overlapping camera. Field of view. So. This is a high level. Like. Illustration, of our, framework. On the bottom we have the input, image. Frames then, we extract different. Kinds of all kinds of descriptors. Including. Objects. Attributes. Actors. Actions, motions, and also, sometimes, we included the seeing descriptors, then. Based on this. Spatial. Temporal graph, then, we apply, what we call the stacked, spatial. Temporal graphic, illusional Network I will, give you more details. Later but. Our. Network. Has. Three. Major. Improvements. The, first we, allow arbitrary temporal. Connections, so, that we can account, for large amount, of, graph. Deformation. And also. As I mentioned we. Will use all kinds of descriptors. Object. Actions, things to. Deal with complex, activities. So. We need a method to support, features, or support nodes with different. Feature. Length. Yes. So. I think here, we, have different kinds of nodes you. Will see we have like object, notes here. And also, actual, notes actually. In our implant, implementation. We also include, the sing notes and. Motion, notes so, these nodes it's, not. It. Can be like, actor, specific, then, we will have all kinds of notes to describe, different, expect, of the actions, so it's, not like we are dividing, the notes per, actor. But. We will have. Take, your chair, yeah that's object, notes so, for action you will have object, notes so. If we go back here. So. On the top we have the this. Is the. Activity. Notes, so. This add actions, but, on there for each activities, we, will have the actors, as well, as the objects, so, actually in our in our implementation we, also add the scene so, all kinds of discreet. Good descriptors. So, this notes can be like a. Bunch. Of collection. Of the, information, that is carried, in the frame. The. Edges we. Have two types of edges, so, as you can see the solid edges here we called the spacial edges so, this is just. Connect, the sum, of the edges connect the. Activities. To the object to the objects this, will specify, the role of the object in the activities, so. The dashed, edges. We call it the temporal, edges so, this is connected, across frame. So. We also because, in the surveillance, scenarios. Sometimes, the, cameras, will not will not have overlapped field views so, the red ones is still, the temporal, connections, but, that is sometimes. We may have missed detections, or, even, don't have observer condition, continuous, observation, of the object or detector, so. These are the edges for. The, graph. Yes. Yes. I will in the experiment, I will show you what kind of algorithm. Algorithms. We use to extract all the descriptors. Of the notes. Okay. Then. Back. To this on top of that we use this our class, structure. To improve, generalization. Performance. Localization. Accuracy and, also. Be. Able to handle the spatial, temporal at, different. Skills. So, I will have slides for these three improvements, in, the following slides. Meanwhile. Working solo what is this I wrote me so. From the image, some. Features are going to get computed, yes and. Something. Is going to get updated right at the dawn's yes.
What Gives update so, the feature describing. The notes will be updated. Each. Node is a vector. Yes. Each node is represented. By a vector. These. Nodes are basically, like. Variables. Like. They are basically this whole, thing is kind of like a graphical, model gray yes, we use this graphical. Model so that we can do longer term inference, but. As well as the graph carries, the sufficient, detailed informations, of the objects, attributes, so. That we can differentiate. Like. Similar actions, and short-term, prospect, as well so, this yeah this is a graph representation. So, actually yeah and we should make it clear, that we. Do use some algorithm. So to, extract, the. Descriptors. From the frames then, we construct the graph then. We use some craft convolutional, network on top of, this graph to, do the action. Segmentation. So it's not like and, to, end just, use one giant. Neural, network so, we use some neural network to to, extract, the descriptors, then, constructed, a graph then to the graph collusion on top of that. Yeah. So this is just some, background, information. About graph, collusion, on networks, so. Actually I was idea. Comes. From the spatial. Vertices. Now. For. The original, implementation of. The graph konasana networks, is only under, notes so, it's only propagate. Based on connection, of the edges but, the later implementation. They do consider. The, edges can have, as well you can propagate. Notes. Descriptors. As well as the adjectives critters but for our implementation. We use the original. Doesn't. Change yeah, that's the limitation, of the original, graph. Convolution, Network so, here we kind of still follow the like. The graphic. Needs to be fixed it's not a dynamic, graph. No. I'm. Sorry so. In here it is but in our implementation. Way. Because, we use all kinds of descriptors. We. Cannot allow. That so, we actually generalize. The. Computation. So that we can handle nodes. With different, future, nuts I will come to that point later. Actually. Yeah the edge actually is the matrix. Of the features. That when you do need the, features for, the node to have same. Lines but I will show some like, workaround, to. Solve. The problem where the nodes, have features of different lengths. So. For the original, spatial-temporal graph. Convolution, network it is used for the scanning, and paste action recognition. So, again there. Are two major, limitations. So in the original. Implementation. For, the temporal connection is actually. Degenerates. To the great, like data, structure, it only allows the connection, of the same joint cross, consecutive. Temporal, frames so. That's why there. Are spatial, temporal collusion. Equation. We. Only see, the. Spatial. Adjacency. Matrix but. Temporal. Adjacent matrix, degenerative. And also. The features as you mentioned, the, features they use fixed lens so, actually the feature itself is very simple only the X Y location of the joint as well as the, confidence.
From The I, believe. It's open post output. So. With these two limitations. The original, spatial-temporal graph, conditional, network cannot, handle the, complexity. Of our. Network. Our graph, representation. So. Again back to the first improvement we want to use, arbitrary, temporal, connections, so, that we allow different, graffity. Of collusion, including. Newly, detected objects. Miss the detections including. Object and activities, so. Basically, we have two layers of, the. Graph conclusion, the blue is the spatial graph. Collusion, and the. Green one is the temporal, graph convolution, by, adding, the. Space. I'm, sorry. Temporal, adjacency. Matrix we, can handle, the connections, in. The temporal. Access. With, different. Graph. Deformations. Here. So. For features which varied lines actually, we. Kind. Of designed two solutions, on. The left-hand side this is a very, straightforward. Implementation. So. We just add a shallow. Layer of the network to. Map the features of different less, to, the same lines, so. This is a very intuitive. Implantation. But. On the right hand side and this is the implementation. We, later used on the Shred data set actually, we because, the, notes itself, some of them note actually may have the, same less so, we group those notes together the, map, them to a one, spatial. Graph. Conditional, Network so, that's why we have several. Special. Craft. Conditional, Network the. Output, from the spatial graph. Convolutional. Network will have the same lines then, we can put them in, put them to one, spatial. And. Temporal a, graphic, emotional, network but. One. Object another, object between. One object and. Are, they all based on special, special. Sort, of weirdness or or. How. Does the grass get set up between so. This for. Multiple objects, we. We kind of build the object. To. Activity. Based on the closeness. The. Proximity of the, object, and activities. So. But, yeah. You you but, here, I think. We, do have problem because when, we group, the spatial. Group. The nodes with the same lens together then put them into one spatial, graph. Collusion or network within that consider the. Connection, cross group actually. We put that connection, model. That connection in the temporal, graph, constrain network because I'm a temporal. Graph consonantal can handle arbitrary, connections, so, this is the kind of the design tricks, behind it. So. I would expect your, network, to optimize, them somehow. You. Actions between actors and objects will define, their action, right. So. Actually when we construct. The. Representation. We consider, that which. Nodes, should be connected, so. That, is the current implementation I, agree we can be, more dynamic, about, how to construct the edges so, actually right now we are working on some attention mechanism. So, yeah, so that we can we. Just do not assign connect. Or connect. Connector. Or not connect we assign some attention so that we have some dynamics, yes, I agree that this is a very good part for the basing permutation, we do not have that but we are working on improvements, to, have the edges more dynamic. So. On intuitively. What you're currently doing we will try to find correlations. These graphs. Temperament. Written that's. What you were coming to do you assume that your representation, is correct, and, you're just trying to correlate. Between the structures, yes. It's kind of because. If. We just use one network to do that when. You go. Into. Higher higher level, or when you go into like longer term. Respect, you lose the details, of, each, individual. Actions, so, in this way we kind of can't do the inference, as, you said kind of just get to the correlation like, frame by frame in your longer term but the. Graph, is self at the, spatial, connection. It also carries, the details, for the actions that is kind of the. Improvements. We see here. Do. You have might be modulating, your audio. Keep. The mic. Okay. Okay. So. Some. Pros and cons. For. The naive implementation again. When we map the features to a comment, and lens, or common space we may lose information but. The network, architecture is, simple though. Is when we use the more advanced. Ones we, reserved. The information, we need but, the design, is more, complex. Then. The, third. Improvement, we have is the hourglass. Architecture. So, we power the idea for, the hourglass, architecture. Usually, used for the convolutional, net, layers so. But we do have some like. Careful. Architectural, design I, want to point, out first. For, these applications, is we. Want to do the action segmentation. So, we, only need the, segmentation, at the temporal, access, so, that's why when we do the encoding we. We. Have the future. Pooling on the graph but. When we do that deconvolution we do not blow up to, the original graph dimension. We only care. About it, Pro, resolution. And also when we do the, the. Convolution. And the deconvolution we also need to adjust the, spatial temporal addresses.
The Matrix the dimension, of these those metrics. Accordingly. Okay. Some. Results. So, we test this. Network. Ultra data sets why is the cat 120. This may not be that. From. Like, femurs, but, we choose this since this data set they give us some, pre. Computed, features, so that we we have fair comparison, comparison, with other, methods. And the, second, one we use the charades, oh yeah. As. You, asked these. Are the networks we used to compute. The, descriptors. For. The spatial temporal graph. Our. Surest intercepts, so. For, the cat, 120, we compare with the state, for the art we, were. Able to achieve about, for. Improvements. In the f1, score and also. For charades when. We use the V GG as our backbone, network, we our, performances. At the steep, the art we, use a 3d we are comparable, to the street art but. A little bit lower. Than the best, number, but, as I the. Patch the number actually they already used the attention mechanism, which, we are working, on it right now so with the introduction of attention. Or more, dynamic. Graph. We. Believe our numbers can be pushed further. So. Here. I, will just show some of the ablation studies. The. First obvious, wise to compare with. Just used the features without any graph. Architecture. We, can see. 4.06. Improvement. In the, mean. Average precision. We. Also want. To see whether the hourglass, structure, do. Does. Improve the performance, actually, reseat, about. 2.5. Improvement. In the map. So. Actually. We also want. To see because. I pointed, out the limitation, from the original. Spatial-temporal, graph, convolutional, network, we. Try to implement. Polina. Version of that. But. Since the original. Algorithm. Is applied to a skeletal, data we don't have a complete. Replica. Here, but. We do just. Degenerate. Our temporal. Connection to adjust to. The same nodes across, one. Time step and also, we just use one type of feature. For. Other node we, do not use hourglass and we see five. Point for improvement. And. Next. Time some ablation. Study with different, types of input features, here. We use one type of feature to type the up to four types of features we can see they gradually, improve. The performance, we. Also tested. Our, network. With different temporal. Connections, because, one of the improvement, is to generalize the network so that it can handle arbitrary. Temporal, connections, so, actually from our experiment. With, different architecture. The, optimal. Number, of. Temporal, connections, as well as the improvement. It introduced, can, vary so, our conclusion, for the time being is that the. We. Do see improvements but, how to optimize, the number of temporal. Connections, that is application, and architecture. Dependent. I think. With that I just show some example. Results. This is the result from cat, 124. Actions documentation. And. Charice, data set so, for streets as you can see the ground truth is not only the action but also actually, some, objects, here, so. I will stop here for any additional questions. You're. Measuring the iuu, of the, detected. Box yes in, time yes. Yes. Have, visual visualization. With this box because. Simulation. Typically refers to coloring, pixels, right oh. Yes. For this segmentation. The action, segmentation, means the segmentation, at the temporal. Spent. Not at the, spatial. Four. For, the current Network no it's. Just a localization, in the temporal access so. It's, not the spatial. Semantic segmentation. It's actual segmentation. In the temporal, access so. We only see. These. We just have, locations. Yes. Yes, you are right actually. With the object, we can look nice but. Yeah, I should say that for, this experiment, we can localize but, for actions, documentation. Task itself, it does not require the spatial. Localization. So. It only requires, the. Temporal. Localization, so when we do, those map so the Lu is also a CIO, you, that's the how you allow the temporal, span not to the spatial, locations. Data. Sense, the. Mep. Numbers, are relatively, low in general, yeah right for sure it's, so. So. Do you think that, an. Evolutionary. Approach will, increase, the performance just, by adding. To these networks, or, something. Completely new is required to, go to the next jump, so. Actually, this, is kind of our motivation, to use graph collusion, network because we think the, end to end maybe it's not the solution we do need some a structural, data but, you use those and to enter to get features, the structure, inference, so, that's our motivation, here, but, again, as. I said this is just the first link plan implementation. We. Want, to introduce, more, complex, graph. That, is adding the that many dynamics. With. The attention we also need to add different. Edge features. So. For now if the edge is just saying these two, nodes connect or not we. Believe with all this extra, information, this. Can guide us to. A better performance. With this graph, structure. Yeah. That's our motivation, here. What. Happens if you make a mistake in populating, it so for instance you are saying that, this person is is is. Interacting, with these two objects for instance right now, what.
Happens If if that early, thing is wrong and the, construct. Is wrong in, term for, the time being the spatial, ones we, kind, of do not have any correction. But. With the attention, we can allow, more connections. The Latin. Network, to like. Attend, to the specific, edges. That's contribute, to the final, decision but. For the temporal, wise we already allow some some, like. Redundant, connections, so. That's. Kind of again, we need the attention to, make it more dynamic for, the time beauty. Of into a learning it. Helps you avoid the sin of any commitment, yes. Committing. To some some interactions. You. Learn. And. For, this one we kind of want to have some structure, in the inference, otherwise. At the, like. That. Semantic, level we. Think when, you need those structures. Instead of like. A black box let you choose. Anything you can but, agree how. To make. The transition, between them, and to, end learning, to how the network, to the kind, of from the black box to the structured, data the interface, how, do you allow. Some. Capability. To correct early errors. They agree, that kind, of also, some research. Direction, we can look into. Graph, structure, lunamon said. Because. I remember Daphne, Koller is to work a lot on that. That's. On its harder problem I guess it's, better to learn their potentials, very, romantic intentions. Sorry. This is where, I was pointing out that ultimately, if your goal is true, we. Will figure out what. Is responsible. For a certain action which objects, which particular, hand. Movements. It. Seems that if you have explicitly. Represented, nodes which can ultimately tell. You that then somehow, you. Need to represent that explicitly, write also, m2 and typically requires way more data and they. Think the last, CBPR, the, best, paper was about how you can combine, different tasks. To, solve any task and this. Combination which between reduces, they. I. Think, it. Also. Makes sense to think here in German if, you have very high correlated. Errors then. You. Have some outliers. So. You. Do everything you did here with the CRF. Or solve of must be ended. Yeah, actually I. Understand, CR and so on trying to really. Understand, conceptually. What, is use. So, the CAF could be again, a fully, connected graph yes, all the nodes you have in the graph could be the Lords. Of the CRF, and you, can have this pairwise, potential. Yes yes, actually I, will. See a couple of years ago I live Behati he is kind, of almost of, I will, say one of the first couple, of papers, try, to use the features from the deep networks, then use the graph. Representation. To provide some structure for, complex. Or the action. Segmentation. I will, see based on all, these, kind of development, of the graph conditional, Network I will, see it's kind of more powerful, it learns more, like. Benefits. Learns, more inference. Capability. From, the data so. Agree. Yeah. We did not do comparison. With the original, paper. Yes. These are all. Like. Deep learning based, methods. I will see yes, but I believe, some paper did to comparison. With the original, deep. Feature plus.
There Have kind. Of implementation, the performance, the. Graph collusion, or network or two performs, those methods. This. Most, challenge, right which one. Of the challenges, there is to localize. Actions, you. Mean special yeah. Making. Two more. Yeah. That. One better. Than the straight okay. Yeah we kind of pick up the streets, because in their notation, they have some a notation, of the objects, those, kind of but for the thermals. They don't. So. This is what they caught I. Cannot. Remember what the super event me but I 3d 3d. Commercial, Network I think. They do some, like hierarchical. Structure, of the events, so that's why they call it is super events but they also do some attention. I do. Lose interest in German said that I don't think so. The. Other work I showed you from. The. IDC. You know that, there. Actually we, had a. Generative. Network. At the bottom and Dennis here at the top in fact trying. To combine the discriminative. And, generated. In one, package. Right but. Our, listeners. Like you quite parallel to whatever years. Found also that first, of all the, first. Of all the distinction, between the, reasoning. Part and this kind of part also is prohibiting. Learning. This. Structures also much more power, and. See. Convolution. Reverbs, are also people. Pinky feed-forward, there's no optimization, and in Princeton right. In the sense that graphical. Models you have to do that's right you ever solve there may be problems let's, find the answer, to some problem here there, is no optimization factors. Again. Yeah. Training. Making. Even more obscure. So. My observation. Is you do something with data you do something with you these and you, act. Or. You Agassi, are up to it and you get to percent point in. The. Solution. Doesn't. Seem to help here yeah. We're a revolution, Sonia mercy honest we have to solve some big, problems, and I, guess here. At. Run time is just. That. Way the optimization. Is done in the training process right, you're kind of yeah yeah. Okay. Thanks, thanks about.
2019-04-13 22:39