Imitation and Innovation in AI: What 4-Year-Olds Can Do and AI Can’t (Yet)
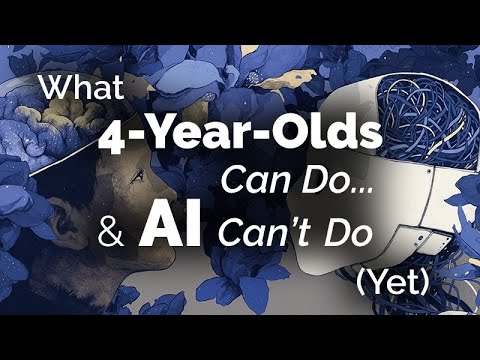
What I'm going to do today is talk about a couple of different ideas about the relationship between AI and childhood. What I'm going to do first is talk about what I think it's a really different way of conceptualizing what AI, particularly with some of the recent developments like the large rich model. How we should think about that, how we should think about them in relationship to humans and to children and to human intelligence.
Then in the second part, what I'm going to do is talk about some of the things that children can do. The kinds of intelligence that children have, which you don't see in these other kinds of systems. Then at the very end, I'm just going to point to some ways that we might be able to develop artificial systems that would have some of the capacities that we see even in very young children.
You can read more about this in a more popular form, in this APS presidential column and then in an academic form, in this paper that's in press and that you can find on archive. All right, so let me start out by thinking about this reconceptualization of AI. A very common way of thinking about artificial intelligence systems including things like large language model, is as if they were individual agents.
As if they were agents with a particular kind of intelligence moving around the world, thinking, deciding, planning, etc. It's interesting that even if linguistically people nowadays refer to an AI as opposed to referring to AI individually. Typically either people think of them as being really incredibly or both incredibly smart agents, genius agents, agents who are even smarter than we are, and also really evil agents like the Gollum. I think it's interesting that even before we had machines, when people thought about what it would be like if you had an intelligent machine, they thought it was going to be evil.
That goes back to Gollum and the Greece. This picture is we have these individual agents. They might be good, they might be bad, they might be smarter than us. We have to figure out what it is that we're going to do with them now that we've released them on the world.
A lot of the conversation is like this. Now, as I'm going to say later, it's not impossible to think about an artificial intelligence that was an agent. But I think that is not the right way of thinking about almost all of the models that we have, and especially things like the large models that have been so successful and intellectual recently. Instead, I think the way to think about these models is as what I've called cultural technologies. What's the cultural technology? Cultural technology is a technology that allows individual humans to take advantage of all the information, knowledge, skills, abilities that other humans have accumulated over millennia of cultural history.
You could argue that language is kind of like the early cultural technology. Language is the thing that humans have that lets us learn from other humans. But over the course of human history, one of the really interesting things that's happened that's linked to massive qualitative changes in how we function is the development of better and better, stronger and stronger, more powerful new cultural technologies. An obvious example is writing.
Writing allows you not only to learn from the post menopausal grandmothers, who by the way, are the people who are really the agents of all this cultural work, not only from the post menopausal grandmothers. I can talk about that a bit later on. That's all I did. That's true, the post menopausal grandmothers who are in your village. Once you've got writing, then you can learn from the grandmothers in very far distant places. Far distant times in the past, we know that writing had a really major effect on giving intelligence. If you think about print, that's an even more dramatic example of something that enables you to get information very quickly from many different kinds of places.
Along with print go institutions like libraries indexes ways that you can access that information. Of course most recently you have things like Internet search, Wikipedia, which are using the digital technology to enable you to much more effectively, much more quickly, swiftly access all of this information from other people around you. Now that's a really different picture than the agent picture.
It would be incoherent, as we used to say in philosophy, to ask, does the Berkeley Library know more than I do, right? Well, in some sense, of course, they have much more information than I do. But it's just silly. It's just a mistake to ask whether the library knows more than I do. The library's a technology, which enables me to get information from lots of other agents. Wikipedia, by the way, I think is a particularly interesting example of this.
This ability to have these kinds of cultural technologies, arguably, is in itself the thing that makes humans so intelligent. There are lots of people in cognitive science who have argued for what's called the cultural niche. This is the idea that what makes us human is exactly this capacity to take information from other people and use it to make progress. People sometimes talk about this as being like the cultural ratchet. A home group of people go out of the environment, they learn all sorts of things.
They pass that information on to the next generation. That next generation takes off from that information, use it to learn yet more. That process is the thing that has actually enabled cities and civilization and all the things, for better or for worse, that humans are capable. That's an idea about something very basic about human intelligence is that we rely on these cultural technologies. I think this is also helpful because when we're thinking about a lot of the pragmatic questions about what the social impact of AI is going to be, how we should deal with it.
I think it's actually very helpful to think about these historical examples of other kinds of cultural technologies and their impact on us. Again, I think that's a much more helpful, insightful way of thinking about it than thinking about it as has the Gollum come to life and is it going to come and kill us all, or are we all going to disappear and just have the super intelligence instead? It's interesting that if you look at conversation about these technologies, people always point out that they have good features and bad features. Famously, Socrates thought that writing was a really bad idea. I won't go to this quote, but I love this quote. I think it's interesting, especially like that last sentence about, they seem to talk to you as though they were intelligent books do.
But if you ask them anything, they just go on telling you the same thing forever. Which sounds so much like talking to ChatGPT. It looks as if it's intelligent, but then it just keeps producing the same nonsense over and over again. You can already see, and Socrates pointed out, that if we relied too much on writing, we would lose our capacity to memorize all of Homer, for example, which is indeed something that very few people can do nowadays. Also and interestingly, that writing would be a great source of misinformation. Socrates pointed out, if something was written in a book, you couldn't interrogate it the same way that you could interrogate a person.
That would mean that you would tend to think that things that were written down were true, even when they weren't, and those issues are issues about how they're going to affect us. Also about misinformation of the possibility of transmitting information that's really useful and accurate, but also transmitting information that isn't useful or accurate. That's an issue that comes up with all of these cultural technologies. The example of print, I think, is a really interesting one. In the late 18th century, there were enormous technological changes, which meant that although print had been around since Gutenberg, suddenly everybody essentially could print anybody with a little bit of capital. You could set up a print shop and you could produce pamphlets.
Historians have argued that this had a transformative effect on society. Many of the things that we think of as the Enlightenment, for example, really depended on having this technology printing available. On one side of this picture. that's actually Benjamin Franklin, who famously was a printer's apprentice, and it was his capacity to print that arguably enabled things like the American Revolution to take place.
I was recently reading this wonderful biography of Samuel Adams, and literally Adams was in the print shop the night of the Boston Massacre, making up the Boston Massacre. This thing happened there were a bunch of protesters, there were a bunch of soldiers, something happened. Adams went to the print shop like 10 hours later and wrote up the story about what was going to be the Boston Massacre and distributed it with print. On the other hand, even though we think about print as this way to underpin things like the Enlightenment diffusion of knowledge. He also, like writing, had the capacity for misinformation from the very beginning.
I highlight this is by now an ancient classic book. Robert Dartin's book at the literary underground Old Regime. Another nice Berkeley issued example of how humanities and science can speak to each other.
What Dartin did was go back and read not just, common sense and Benjamin Franklin, but everything that those printing presses produced. You will be amazed to hear that most of it was really terrible. A lot of it was softcore porn, and the rest of it was libel. Let them eat cake, for example, was actually a meme. It was actually a meme that was invented in the context of print.
Marie Antoinette never said it, but it was obviously a meme that like memes and misinformation nowadays can have really strong and in this case, arguably very terrible effect. Now, one of the things that I'll just go through this quickly, but I think it's interesting, is that you might say, "Well, okay, why is it if what's going on in these cultural technologies is that we're agglomerating information from lots of different sources? Why do we so often think of them as if they're agents? So why don't you treat them as if they're agents? Why do we say ChatGPT said this?" It's almost impossible when you're talking about these systems not to say it said this, it did this, it could do this, it can't do this other thing. I think it's interesting that if you look at past cultural technologies, very often the form of information that a cultural technology uses is to have a fictional agent. It's hard to tell people very general things about all the information that other people have accumulated. Often it's much more effective to have a made-up agent who's actually illustrating the information that you want to tell. It's interesting that if you look even back in the place to seeing, look at 100 data communities.
One of the really important things that happens is, especially in the evening, people sit around and the postmenopoltic grandmothers who are always the agents that change, talk to the little kids and some of the adults as well about what are the myths? What are the stories? What are the things that are important in our culture? What are the things that you need to know? What are the big things that you need to know? The way that you do that is through telling stories. The way you do it is by having a fictional agent who can exemplify the things that you think are really culturally important. That's actually something that we have quite characteristically done. Another interesting, an anthropologist of religion just has an interesting paper about this in the context of LL. Another example if you think about God, gods serve the same function, even though gods don't actually exist, there are ways of passing on information about what you think is important in the context of culture. Don't say that, I said that the large language models were gods.
Or maybe you could say there only gods exist. They're God, God that doesn't exist. Last to me, as that I love as the daughter of an English professor, is that in fact, that 18th century print revolution that I talked about also came with this new literary form, which was the novel. It turns out that the very first novels like said, this is a Samuel Richardson. Pamela, even though Pamela was a made-up person, she became incredibly popular.
People would line up in the streets to find out the next installment up what had happened to her. Senator Richardson was a printer. The printing and these new fictional characters in novels, who could tell you something about what's going on around you happened in concert. What I want to argue is that that's the way that we should be thinking about these large loves. They're different.
It's all like they're just the same as print. Each one of these new cultural technologies has different characteristics, different kinds of powers. I think they're arguably more powerful than print because they can generalize, they can agglomerate enormous, giant amounts of information, all the information that's there on the Internet.
But I think the structure of how they work is the same. I think in that context, again we can get some important historical insight by thinking about how we manage the previous cultural technology. Every time the cultural technology develops, you're faced with these problems about things like different information. Typically what's happened is that various kinds of norms, rules, regulations, laws, principles develop in parallel that actually enable you to get the benefit rather than the cost of the new cultural technology. If you think about things like just norms of truth, that you should tell the truth instead of lying. Or things like editors, journalism schools, fact-checkers.
The developments of print in the 18th century came with new things called newspapers and editors which hadn't existed before. Instead of just looking at all the pamphlets, you could say, "Okay, this printed paper is The New York Times, and therefore, I'm going to give it authority that I wouldn't give to just a random pamphlet that was on the street." I think that's the thing that we have to do with AI. You can't just say, "Yeah, it'll be fine. These aren't like evil goes, so everything's okay.
What we need to do is to have the same mechanisms that we have for other cultural technologies." That's the first part of the talk, making this argument about AI as these cultural technologies that are accumulating lots and lots of in all the information on the Internet. Why isn't that enough? Why isn't that enough to be intelligent? As I've argued, so much of human intelligence comes from this capacity to other people to extract information from other people, why is it that a system that can do that really well and much better than any individual human cat? Why wouldn't that [BACKGROUND] To answer that question, I want to turn from thinking about AI systems as technologies, to thinking about children. Why children in particular? Well, famously, in the sus Turing paperwork, it talks about the Turing Test. There's this wonderful segue that nobody ever notices or didn't notice until recently.
Which is, he starts out saying. Do you want to know if the system is intelligent? You do the invitation test. But then he suddenly shifts and said maybe that's not the right test. If we really wanted to know whether our system was intelligent, we'd have to know whether it could learn from its experience the way that a human child, for example, alerts.
He very explicitly says, "Instead of producing a program that can simulate the adult, why not try to simulate the child?" In a sense, what something like large models are doing is accumulating all the information from all those adult minds that are out there. That's the great power of those systems. But although children do that, children do something rather different. What children do is actually go out into the world and learn about the world independently of all the information that they're getting from other people. Over the past several years, more and more people within AI are trying to use children who are the best learners up as a model for how we could design AI systems that could learn in this way as well. The title of the paper that I mentioned is Transmission Versus Truth.
What large models and the typical AI models that are traded on large amounts of data can do is transmit information very well. What they're not good at doing is going out into the world and finding the truth, which is the thing that kids are very good at. From that perspective, for example, when people talk about the problem of something like ChatGPT hallucinating, which is something that everyone around has seen. It's not that they're hallucinating, it's that they just don't care. There's nothing in their objective function that makes a difference for truth, out of falsehood.
If you just imagine what at Socrates said books. The books in the library don't tell you whether what's in them is true or not. They're just there to transmit information.
But as we'll see, kids care tremendously about what's true. Arguably, kids that's their greatest motivation is going out in the world and trying to figure out how the world works. We know a bit from developmental cognitive science about how they do that. In this DARPA Machine Common Sense program that we've had at Berkeley with great AI people like Tendra Malik, we've heard in this series before.
I discovered that working with DARPA, the most important thing is to get good acronyms. [LAUGHTER] Then you've got DARPA worked out. Our acronym for what we're doing is MESS, which is appropriate for children.
Which is to try to design these model-building, exploratory social learning systems. How do children learn so much and how do they learn the truths about the world? They first of all, don't just get statistics, although we have a lot of evidence that children are amazingly good at statistical inference, much better than we ever would have thought before. But they don't just aggregate statistics the way that something like a large language model does. Instead, they actually pull out abstract causal models, intuitive theories from that statistical evidence.
My first book is called The Scientist in the Crib. I and lots of other cognitive scientists have used this metaphor. They're not just looking at statistics and making predictions. They're pulling out causal structure and then using that causal structure to understand how the world works.
That's one of the most important ways that humans in science but also in everyday life figure out to chose about the world. The other thing that makes what children are doing very different from what typical AI systems and particularly large models do is that they're active lures. They actually go out into the world and actively try to get the data that will be relevant to the problems that they're trying to solve.
There's more and more evidence, I'll give you an example of this in a bit, that they're super sophisticated in doing this. When scientists do this, of course, we call it having an experimental research program and when two-year-olds do it, we call it getting into everything. But it turns out that if you actually study the getting into everything, that what's going on is something that's much more like a scientific research program than you might imagine. Children aren't just passively absorbing data from the program around them, for example. They're actually actively going out and trying to make discovery to find out about things going on in the external world, not just say in the Internet.
They do also have these capacities for social learning, as I mentioned before, for extracting information from the other people around them. There's some lovely studies of this. Sometimes what they do is just mindlessly try to agglomerate that information. But a lot of times what happens is that they're balancing that as we'll see in a minute, again since this drive for the truth.
As opposed to just accepting what other people tell them, they try to balance that against other information they've got including the information they've got through their own experimentation and exploratory delay. Let's say a bit about how does that picture of what children are doing, how does that interact with this really remarkable set of stories we've had over the last year or so about how powerful these large models are. What can large models tell us about how children are learning, and what can children tell us about how large models work? Well, I think one way of thinking about it is that we can figure out what information are available through just looking at the information that other people give you and what information do you need to discover for yourself? I think syntax is a really good example where typically people in linguistics, for example, had thought you can't get to syntax just by looking at the statistics of linguistic input.
It turns out that actually, no, you can't. This is something that the systems genuinely are incredibly good at. It looks as if there's enough information in the data to extract the structure that you need to be able to produce grammatical sentences. Which is not something that I think we would have known before. Another thing to say which makes this problem a little more difficult in some ways from the cognitive science perspective, the old fashioned as in like last year or three months ago or yesterday. Vanilla large language models, the ones that just work by predicting what the next word or token is going to be are more useful than some of these more recent ones.
What's happened more recently is that rather than just extracting the statistics of the information, you've added things like reinforcement learning from human feedback. Which means that now we have humans. I highly recommend a piece in New York Magazine recently that was describing this. Giant factories worth of people in places like Nigerian and India who are just sitting there and looking at the output of the systems and saying, that's good or that's not good. That's reinforcement learning through [inaudible] [NOISE] and we have things like prompt engineering and fine tuning that are also designed to do it. Now what that means is it becomes very hard to figure out what's actually going on under the hood in the assistant.
Whereas if you just have a classic LLL, you can say, this is information that's there in the language, This is the information that's there in large image model. Once you get reinforcement learning from human feedback, it's much harder to know what exactly is the information that this system is encoding. But from a conceptual perspective. From this perspective, is it an agent, agricultural technology? It's still true that what something like our LHF is doing is not enabling a system to go out to find out about the truth. All it's doing it is giving it yet another way of getting information from other people.
Somewhat less obvious and transparent way than the classic LLM systems are. Another thing that large models can teach us, which I think is really interesting and under has been further study under officiated is what cognitive capacities are facilitated by a new technology? What things we can we do when we have print or when we have writing that we couldn't do before? You can probably already think about the fact that we can remember things and we can set things down and go back to them. There's all cognitive capacities we have from those technologies. A really interesting empirical question is mathematics is another nice example where we couldn't have math until we had a notation system that would let us write down equations. I think a really interesting question is, are there cognitive capacities that will be enabled by these new cultural technologies that would be different from the cognitive capacities that were available to us in the past.
But again, that's a very different way of structuring the problem than saying are they smarter than us or are they not smarter than us? Do they whoever they are have this cognitive capabilities. But I also think that children in developmental cover of science can teach us a lot of things about how to think about large language models and understand them and one of the first things that I think is really important, I will jump up and down about this, is that there's no such thing as general intelligence. There's little bits of stuff about IQ in the psychometric literature.
But from a cover of science perspective, there just isn't anything that's general intelligence, artificial or natural. There's this intuitive theory of intelligence, which is like a fluid or it's like a force that's out there. You have the force of intelligence and you don't have the force of intelligence. One of those guys being the relevant category here, who's got a whole lot of the force or else here a guy who doesn't have so much of the force. That's just not the way that cognitive scientists think about intelligence. Instead, what you've got are a whole parcel, a whole lot of different Cognitive Capacities, and very characteristically they're intention with one another.
Being really good at exploration, for instance can make you worse at exploitation and vice versa. Being really good at focused attention makes you worse at extracting general information. What we need to do is to actually work out in detail what are the underlying processes that a particular system, artificially natural, is using in a particular context and try to say something about that. Rather than saying, do they have sparks of intelligence or do they not have sparks of intelligence? From a methodological point of view as well, children can tell us something about how to understand the cognitive capacities of an Alien system.
I highly recommend this paper by Mike Frank. One of the great things about being a developmental cove scientist is we can't just assume things about the cognitive capacities of the very intelligent creatures that we're studying. We actually have to go out and do very systematic experimentation to try and figure that out. I think importantly, we have to do something that I think of as anti foreign.
When we're doing a developmental experiment, what we have to do is make sure that we don't have any prompts for the children. Because what we want to do is figure out what's the underlying cognitive capacities. Not are the children capable of using our prompts to give us the answer that we want them to give us? It's the opposite of what happens in a lot of LLMs. We really want to understand cognitive capacities, We need to have control conditions.
We need to do these very careful experiments. I think development can provide a lot of examples of how you do this. If we want to say, is this system intelligent, or can the system do X? Can the system do theory mind, for example? To take one that is dear to my heart, we need to actually go out and like developmental psychologists, do you spend 20 years doing experiments to figure out whether and what children understand about the mind? My life would have been much easier if I could have sat down with a three year old in 1986 and talked to them for a couple of days, and then decided whether they had their [inaudible] So far we've been talking about some of the capacities that children have and I mentioned before that these cognitive capacities for faithful cultural transmission are in tension with cognitive capacities that enable new discoveries about the certain things like exploration or experimentation, or causal discovery or induction. What we've been doing is using studies of children, and then comparing them to studies of artificial systems to work out how is it that that innovation works? What is it that children are doing that enables them to innovate, that enables them to learn new things about the world? We know that children are learning these intuitive causal models and they seem to do this through exploration and the research program we've had is to put children and agents in the same online environments and that way we can say unrestricted online environments. Let the children and the agents explore freely in those environments and then see what kinds of discoveries can the children or the agents make about how about how these environments work. One of the first examples of this, this is an experiment with a bunch of people here in computer science.
What we were trying to do was see if children could figure out causal structure. As I mentioned, causal structure is really important and in our developmental work for a long time, we've used this little box, the Blicket detector. The Blicket detector is a little box that lights up and plays music when you put some things on it and not others and with this very simple device, you can actually ask quite sophisticated questions about the causal structure of this device. How does it work? Which ones are Blickets? What will happen? Can you make the device go? So what we've done in the past is to show children different patterns of evidence about how the system works. Again, as if they were little scientists and then we've asked them to draw the right causal conclusions about how the system works. In this experiment, because we now have the online environment, we did something different.
What we did was to actually let the children explore themselves. [inaudible] The invented a virtual detector, so now you can put things on the machine in various combinations and permutations and sometimes the machine lights up and sometimes it doesn't, and here's a child actually playing. This is a four-year old. Perhaps the most striking thing about these experiments is that current day four-year olds are absolutely perfectly happy to figure out and explore in this virtual online environment. I'm not sure that would have been true 20 years ago when we first invented.
Then even more remarkably what we discovered. I won't go into all the details of this experiment, what we discovered was that the children were very good at doing exactly the right things to figure out how the system worked. Most of the children, just in the space of about 20 trials, 20 things that they did, 20 choices about what to put on and what not to put on, figured out what the underlying causal structure was. They were really good at doing this. They figured out which ones were Blickets, they figured out what they had to do to make the machine go and they were much better at this than any of the large language models that were tested. Again, maybe you could do a bunch of prompt engineering to persuade the model to be able to solve this particular task, but just based on their general knowledge, they did incredibly badly in this task.
They were not good at this task, and you can see why. Even though they gave us back references to our Blicket detector papers as a way of answering what was going on in this task, of course, this was a new machine that didn't work like any of the machines that we had in our previous split detector papers. It could tell you, here's this topic, Blicket detector, here's how it works from reading the papers, what it couldn't do was do a bunch of experiments and use it to find out that this detector works really differently from any detector that you've seen before.
I did a new series of experiments that we're doing. That's also in that prospectus paper. We've been doing a similar project with toys. In this project, what we wanted to do was try to look at another way that you could use causal information to try to understand novel possibility than the word and this involved a tasks that involve tool innovation, which again, is one of those things that incredibly powerful, important human capacity.
We were interested in this from the perspective of development as well as from AI. We wanted to know if children would be able to do that just as we were when we were looking at the children on the Blicket detector. What we did was to give children examples where there was two objects that were highly associated with one another. For example, the Scotch tape is much more associated with the scissors than with the band aids and if you could ask people what goes with the scotch tape, scissors that's what triggers. But you then present a particular causal problem, here's an example, like tearing up paper, and then you can ask, which would be the right thing to solve this problem? Would you be better off solving this problem with scissors or would you be better off solving this problem with a mandate even though you haven't already associated the mandate with scissors. Again, I'm sure that with RLIHF and prompt engineering, you could get systems to do this.
But when we first tested this with three, which was just looking at the associations in the language, what you could see was that the children were much better at it, at GPT3 and GPT3 was actually not any better than chance. Again, if you think about it, if you're just looking at association language, scissors and Scotch tape are going to be much more closely associated. If you want to know what word should you produce next after you've heard Scotch tape, scissors is a much likelier outcome than band aids. But if you're actually trying to figure out the causal properties of band aids and scissors and paper, then you're going to have to think about that information in a different way.
In the most recent experiment that we've done, we had 47 different scenarios, each with a different set of objects and within each of these sets, we have a reference object, like a tape, and there's a superficially related object or a functional object that's not superficially related or a totally unrelated object, and then people have to rate both adults and children, and LLMs have to rate them zero to five, how useful each of these objects will be in trying to solve this tab. I ripped up a paper, I have to decide what would be most useful glue, which is obvious sticky thing,a bandage, scissors, or cotton bolts. What we discovered was that when we simply asked the association a question, so we said which goes with which? Does the scissors go more with the Scotch tape or do the cotton balls or the band aids go with the Scotch tape? Although the models were as good as people, or at least many of the models were as good as people, both children and adults. They seem to be able to pick out you association on the data, but when it came to generating the novel functional capacities, even GPT4, which has all this extra RLHF was not as good as children or adults at solving these tasks. It was interesting that even four year olds were actually quite good at solving these tasks, so although not quite as good as adults. So far what I've been suggesting is the capacities that you get, especially from large models, involve this transmission of information rather than exploration and truth.
This has been a critical enterprise, and we could give lots of other examples, but how about the positive part of the project? The positive part of the project is, are there other techniques that we could use in artificial intelligence, or ways that we could design new artificial intelligences that would solve some of these problems in the way that children do and we therefore, we hope have some of the capacities that children have. I'm just going to just briefly gesture to projects that we're doing right now and bear. In fact, all the projects we're doing now use this methodology that I think is very exciting of having online environments where both humans and children and agents get to freely explore those environments rather than dictating here's what the solutions are or here's what the choices are providing a supervised environment. We're trying to set up unsupervised environments that can be explored by either agents or children.
Let me just mention two techniques we're trying to use to try to get the agents to solve these problems in a way that's more like children. One is with [inaudible] what we're trying to do is to get intrinsically motivated reinforcement learning, so classic reinforcement learning involves getting a particular reward and that turns out to be really powerful, you can use those systems to do things like learning [inaudible], learn how to play go. But what children are doing when they're exploring is not being, it's also doing things that actually leave them to have less reward in any straightforward way and we've done a bunch of empirical studies that have shown this, what children do play, they go around, they try things, sometimes they work, sometimes they don't, isn't get to good at doing something, they get bored or they try for something else, their motivation seems to be this intrinsic motivation, they're doing things for the sake of learning rather than doing things for the sake of being rewarded in the long run. What we've been doing is trying to take formal ideas like information gain and one that I'm very excited about is empowerment and turn those into rewards. Make the reward be the fact that you are more competent [inaudible], or make the reward be the fact that you know something that you didn't know before. It's a way of trying to put things like curiosity and exploration into artificial systems, that's one line of research, that's one set of techniques that we've been doing.
We've also been doing this in the context of play for example, that we have recordings of children playing in these online environments and then we can use their play to try to figure out what an agent could do in that environment that would enable them to master the environment. The second thing that we've been doing is taking this ideas about, particularly emphasizing these ideas about causality, this is work with Roche Key, a Deep Mind and also with Blake Richards at Neil have been looking at causal infuse. Trying to see if we could get an automated curriculum. If we could design a system that could have what's called an automated curriculum, what does that mean? What that means is another thing that children are extremely good at doing, is figuring out what they need to do to be able to learn something that in the long run, is going to enable them to learn something else.
Instead, the way that these experiments have gone is, we are using the ProGen environment, which is a video game environment, we start out by showing a really hard level that the kids can't do, and then we say, could you decide how to play a game that will help you to be able to play this other game that you'll eventually be rewarded from. Again, if you know children, children are actually turned out to be quite good at saying, first I have to do the simpler thing and doing the simpler thing, it's going to give me the skills that I'll need to be able to do the more complicated thing. The same thing happens with agents, so if you give agents these high dimensional, really difficult tasks, it takes them forever to be able to solve it, but if you could get them to have a curriculum where they'd say, I can't solve this task yet, let me try getting skills in the simpler context and then applying them to the task, that would be another example of something that children are doing that should enable agents. Again, those are some of the things that we're seeing at the moment. That will work on at the moment. The overarching point, I don't want to say, and I don't think it's true that artificial systems are never going to be able to do the things that humans are doing or that human children are doing.
But I think the very progress that we've made gives us a sense of the dimensions, the landscape of the things that are going to turn out to be much easier to do or at least the things that we're going to be able to do with sufficient data, sufficient compute, and the things that are still going to be very challenging, even with very large amounts of data, large amounts of compute. The things that we're going to need other capacities to solve and in particular, the things that are going to demand, these truth secrets that are going to demand going out into the real world, getting new data changing, modifying what you do. Those are exactly the things that the incredibly brilliant, but strange little agents all around us that we don't pay much attention to are doing everyday and the interesting challenge would be to try and figure out what computations are those brains in those beautiful little fuzzy heads doing that we could actually use in artificial cycle.
[APPLAUSE]. That's a great question, the empowerment idea is a technical idea in reinforcement Larry that I think is very interesting, the idea is that you get rewarded for doing something that has an outcome. The idea is, it doesn't really matter what the outcome is or whether it's going to be positive, or even whether it's going to be negative, what you're getting rewarded for is do something that has an effect on the world. I think there's a lot of evidence, we've shown this in things that we've done that children, and again, anybody who has a two year old like mine, I now, by the way, have five grandchildren, including a somewhat terrible two year old. [LAUGHTER] Anyone with two year old will recognize that just mickey things happen is the thing that makes them more excited than anything else and I think that's interesting because from the perspective of causal inference, when we say you're going out into the world and discovering a causal structure, what you're doing is discovering the things that you can intervene on to bring about up. Being very interested in doing that, our whole engineering program, that's what an engineering school is all about, is about how can you make thing happen and I think we just don't know exactly how and when children are doing that or whether some children are doing it or others.
Here's an idea that I think might also be relevant in AI, we're just starting to test this, which is that the reason why children can do that empowered exploration is because they know that they have caregivers as well. Again, post memo puzzle, grandmothers are the key to all of this, if you know that grandma is there and you're going to be taken care of and nothing really terrible is going to happen to you, then you can go out and you can try things, even if you think the outcomes might actually be bad outcomes, but you know that you are [inaudible]. I think the human strategy is we have these incredibly exploratory children, and then we have these very nurturing adults and you need both sides of that to solve problem. Hi, such an interesting talk, I'm curious on the element of how children's learn through play, I'm thinking of imaginative play or transformative play, where they're constantly reinventing the rules and how that could be taught or related to these large systems or language models. I think that's a great example and we have a whole project that's called a computational counter play.
One of the things we're looking at is this play where we're just mastering new ideas, but we've looked at things like pretend play. Pretend play again, has these very interesting connections to causal inference because what we've discovered is that when children are pretending, what they're doing is generating counterfactuals and generating counterfactuals is one of the things that you can do if you have a causal theory and it's one of the things that's much harder for something like an LLM to do. Generating a counterfactual and seeing something new that isn't actually something that you've already observed in the world before, if the world were different, what would happen, and that's something that's very hard to do if all you've got is data from what has actually already happened in the world. It's something that you do all the time when you're doing pretend play and it's a really interesting question about when we've studied this, when you look at children's pretend play, it's crazy, it's not true, but it makes sense, it's not random, it's not just that they say anything, they say things that wouldn't make sense if you were in that other alternative environment and that's one of the cues to human intelligence. An interesting question will be, you thought would be, if you could get a system that could generate counterfactuals, that could pretend in a systematic way, you might predict that as with the children, that would enable you to generate counterfactuals in a real life situation where you need to use counterfactuals to stop the public. This is fascinating as a parent of three sons, I think a lot, but they were two years old 20 years ago, [LAUGHTER] eventually.
But I was curious right away for the branches. Is there equivalence of neurodivergence in this thing of thinking of cognitive systems? I'm not quite sure what you mean by neurodivergence, but one thing that I think is really interesting and I had a slide in, there were too many slides. But if you think about this trade-off idea, the brain that you need to be able to do this wide-ranging exploration it's quite different from the brain that you need to be able to say act effectively in the world. If you actually look at the neuroscience of development, what you see is that young kids up to about five have brains that are functioning in very different ways from adults.
You actually sort this curve where up to about five, you get many new synapses being formed. Then after five, you start this pruning process where so the ones that are there get to be stronger, but the ones that aren't there disappear. You have this young brain that's very flexible, very plastic, very good at working. Not very good at putting on its jacket and getting to preschool in the morning. [LAUGHTER] Then you have this old brain that's very good at organizing a symposium series. Not very good at learning a new language, for example.
We can see that even in your size about the differences between children and adults. You have things that are what are my slogans is things that are bugs from the exploit perspective, or features from the exploit perspective and vice versa. A lot of things that children do that have traditionally been seen as being signs of lack of intelligence. They're random and noisy and all over the place actually are signs of intelligence from the exploited perspective.
A lot of things that they're bad at doing, like focusing on one thing at a time and doing long term planning, are things that are great from the perspective of the exploited perspective, but still not so good for release. Thank you so much for this very interesting presentation. My question is this, if we look at DCI as a baby and compare that with a small child, my question is this, the computation power between machine and human there's a big gap.
Because of the gap, could artificial intelligence develop, I find a different way, a pass to developing intelligence compared to human. I think again what you mean by intelligence is solving particular problems. That's what you mean. The question is, what problem is it that you're trying to solve? What capacities do you need? Of course, as people have pointed out, if you want to do something like add very large amounts of numbers, artificial intelligence already is surpassing us by many orders of magnitude. There's no question. Your definition of intelligence,
as it might have been in the olden days, was doing things like playing chess. Then it's clear that the systems you can design systems that are extremely good at doing this. If you're interested in some of the things that are characteristically, things that children, for instance, are solving, like being in a very high dimensional space in the real world and figuring out what the structure of that space is in a very relatively short time with relatively few data samples, that's something that these systems are not particularly good to at. I don't think there's any reason to believe that simply adding more compute power is going to be the thing that will help enable them to solve those problems. In fact, I think there's reason to believe that that's probably not going to happen.
What you need to do is have systems that now have, as it were, an objective function, which is about going out into the real world and solving problems. [inaudible] You said something early on that really caught my attention that these generative models are not hallucinating, they just don't care and that kids care about truth and how the world works. My question is how do we make it care about truth? Is this a collaborative tool that's going to eventually be a two-way street rather than us fine-tuning is the AI going to ask us, do I have this right? How could I improve? Because kids ask a lot of questions. They do. In fact, there's a beautiful study that I like which showed that your average four-year-old asks 20 questions a minute. I put that in my book and there was a lot of man explaining about, no, you must have got that wrong.
That couldn't be true but that's actually what the statistics look like. No, I think the important point is you could imagine, and we're going to try and design systems that could do things like ask questions or get data from the world elders just don't do that. That's not what hair training is, is ever going to be designed to do. The whole point about LLMs is the things they do is they predict what the next token is going to be. They respond to reinforcement learning from human feedback. They respond to your saying to them, that's good, or that's not good.
In a sense that's answering your question. What they don't do is say why is there a clock way up on top of the campanile which is the thing that children will do, let alone say, this is a nice, real example. The little boy was walking and said, why is the clock way up there? Then said, they must have put it up there so the children wouldn't break it. [LAUGHTER] There's a beautiful example of a really good question and a really good answer, but not an answer that you would have ever gotten from either looking at the statistics of what's out there in text, or from asking someone to give you human feedback about whether that was a good answer. In fact, it's funny, what would you do if a system said, it must have done it because of that? Would you say no, that's the wrong answer, or would you say yes, that's the right answer? It's exactly that space of the things that are not the right answer, but are intelligent, that children are incredibly good at, and I don't think at least as currently constituted, things like large models are going to be able to do.
No, I think that's exactly right. For problems like chess where there's a very clearly defined objective, then you can use some of these things like deep reinforcement learning very effectively to get to that objective. An example that I like to give is what they would not be good at doing was playing Addie chess. Addie chess is one of my grandchildren, and his big brother, of course, plays real chess, but what Addie does is take the chessboard and then he'll take the pieces and he'll put them in the wastebasket. Then he takes them out of the waste basket and puts them back.
Then he puts the black pieces and the white pieces in different orders. Then he gets bored with that, and then he stacks them. Addie is really playing chess.
Addie chess is really what the human game is about. Of course, this also drives his big brother completely insane. That's the thing that you would mean if you were playing chess. That's the thing that is not going to be something that you'll have even though it's really amazing that you can manage to make the right moves in chess as a result of something like deep reinforcement learning.
I think it's interesting because you also almost wonder what was the point of chess in the first place. Probably if you could always get the right, it wouldn't be a good game to play. There's other intrinsic motivations in terms of being empowered, getting to be better at solving the game, but it's not the same game anymore. Really wonderful talk. Especially I think grounding us in some historical perspectives is really useful in terms of Socrates, in terms of post-menopausal grandmothers. It's all very useful.
Particularly because I think a lot of the narratives that we're telling are monotonous, unimaginative, and completely wrong. A much-appreciated perspective in the beginning. My question is around the relationship between the very active exploratory hypothesis-generating behavior that you've been describing and some more passive learning systems. There's years where kids their physiological systems stabilize. Their acuity develops.
There are a lot of learning systems that are not necessarily a question mark, actively generating and evaluating hypotheses. But I'm curious how you might think of the relationship between these, what might be thought of as passive learning systems and these active learning systems. That's a great question. I think syntax is a really lovely example of this because one of the things that we learned in development is that babies seven-month-olds olds are very good at predicting the next token in a sequence from a series even when there's no content, even when there's no semantic, seven-month-olds. They're incredibly good at just picking out statistical patterns from data.
There's a whole field of developmental psychology about this, even when they're not actively exploring, even when they're not actively trying to solve problems. For years, what linguists were saying was I know well, seven-month-olds are really good at doing that. Who knows why seven-month-olds are so good at doing that? It couldn't really have anything to do with their learning language. I think now what you'd say is, I get you don't want to know why those seven-month-olds are so good at doing this. This is how they do. I think it is definitely true that kids are extracting enormous amounts of statistical information in this passive way.
But then the interesting thing is that they're putting it to use, and again, I think the example of thinking of the child, a scientist, is a very helpful one. We use statistics and we couldn't do a lot of our science unless we had the capacity to take a whole bunch of data and pick out what the statistical generalization is in the data is. That's incredibly useful thing to do, but it's just the first step in enabling us, and sometimes it's not just the first step. Sometimes you might as I think a lot of medicine, for example, works that way where they have no idea about causal mechanisms.
You just say this is more likely to produce a good result of something else, but most of the time you want to extract underlined causal metrics through. But I think it's fascinating and interesting that both of those things are present in kids. The important point is it's not just that the kids have the passive capacities they're interacting. [APPLAUSE]
2023-12-29 10:58