Eric Siegel | The AI Playbook | Talks at Google
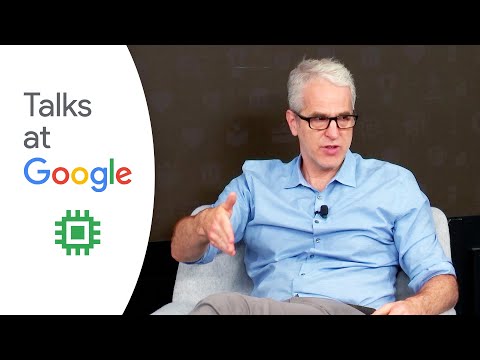
[Music] welcome to toxic Google uh I'm Brett durett and today uh we're welcoming Eric seagull to toxic Google Eric has been in machine learning for over 30 years he's the founder and CEO of gter AI as well as the founder of the long running machine learning week a conference that's coming up in two weeks in Phoenix uh he's known for as the bestseller uh bestselling author of Predictive Analytics and his new book the AI Playbook he's also a Forbes contributor and was previously a professor at Columbia University so everyone please join me in welcoming Eric to our stage thanks Brett thank you very much thanks everybody for coming it's a pleasure to speak here I'm going to present for you A six-step playbook for running uh Enterprise machine learning projects successfully through to deployment because they usually don't get there so here in 20 seconds here's why machine learning is important business needs prediction prediction requires machine learning and machine learning requires data putting that in Reverse we have data we give it to machine learning it generates models that predict and those predictions drive all the main large scale operations that we conduct every day which is why we also call this these types of predictive use cases predictive Ai and Predictive Analytics in that way we cut costs boost sales uh streamline manufacturing combat risk prevent fraud fortify Health Care conquer spam and win elections as Morgan vter of Unilever put it in the forward to the my book the AI Playbook machine learning's practical deployment represents the Forefront of human progress improving operations with science so it was great opportunity to speak talks to Google thank you very much and have a great rest of the day wait actually no hold on a second isn't prediction impossible you know the only thing certain is uncertainty human behavior uh which is what we're predicting for many of these use cases no less challenging to predict how do we put credibility on a um area that has the audacity to call itself Predictive Analytics that's a credibility question that I'll cover partway Through the presentation and a second burning question is look we're making these predictive models that I show as golden eggs in the slides with machine learning otherwise known as predictive modeling that are then meant to get deployed into operations across industry sectors across lines of business for all these different kinds of operations does it work do we get there does it actually make a difference and change these existing large scale Enterprise operations actually not usually most new machine learning projects fail to to achieve deployment so what's missing is a greatly needed unmet uh requirement which is a a um a well- adopted established framework Paradigm playbook for running these projects from an organizational standpoint that's well known to business stakeholders and that's what I'll be covering today I call it bis ml but this sort of no man's land this area both sides are kind of saying hey it's the other person's responsibility both the data science the tech and Biz both the data scientists and their stakeholder their client are kind of saying look that's the other person's job and the root cause for that is that both sides are conceiving these as technology projects their business Endeavors their operations Improvement projects uh that use machine learning as an important part of them um we have to reframe the the way we view these basically it's a Consulting gig not a technology install business Consulting so I'll come back to this so I'm going to cover Predictive Analytics machine learning make sure we're on the same page of how it works how it delivers value the problem which is that it fails to deploy the need for an organizational Paradigm and that I call bis ML and probably more fundamentally um than the actual Paradigm itself is the need for deep collaboration end to end across that Paradigm process practice Playbook that is deeply collaborating with the non- technical or non- dat scientist stakeholders who have ramped up on a certain Semite technical understanding of the way machine learning works so a couple quick slides about myself as Brett mat mentioned I've been running the machine learning week previously Predictive Analytics World Conference series since 2009 we have a new sister conference generative AI application Summit this takes place in two weeks from now in Phoenix and we'd love to see some of you there and I'm the founder of the startup good AI which maximizes machine learning's value by testing and visualizing its business performance so business metrics are key here and I'll tell you a little bit about that I'm also a skier and um this is me skiing in Utah and I'm just a micro risk I'm just one Healthcare consumer so unfortunately this this is the before picture and in the after math I had ruptured my anterior cruciate ligament uh rough okay but I don't put this up to Garner your sympathy I mean you know if you wanted to be a little sympathetic though there we go I knew it was coming thanks no uh my clinical outcome's great I can walk on my knees super important because I've got two toddlers now um who you should feel sorry for is my insurance company which is essentially doing micro risk management just the same as Predictive Analytics deployments across industry sectors so it's the same kind of general gist for each individual office worker people cleaning a window of a highrise putting a risk a score a probability on the potential for a negative outcome for each individual this now Eric Webster State Farm said insurance is nothing but management of information it's pooling of risks whoever can manipulate information the best has a significant competitive Advantage wouldn't it be cool if we could take that same value proposition that same idea that same core competency and apply in general across Industries that's what we're doing with Predictive Analytics what are the chances after you spend $2 contacting somebody with targeted marketing that they're not going to respond you just wasted $2 that they'll defect or cancel as an ongoing customer that they'll default as a as a debtor or commit an act of fraud so basically by putting these kind of risk scores or the chances of something bad happening we're conducting triage for both Healthcare applications and across lines of business and and verticals and industries um and in that way we could sort of view most of these use cases as the antidote to information over overload so it's like look I got so many sales leads how do I prioritize and triage them right and that's what you're doing with search results right Google search is built built on um machine learning models um the order of your of your news feed in social media like Facebook there's so many different posts by your contacts which ones are going to be of most interest to you how does that get ordered filtering spam also machine learning model and then very analogously these kinds of um product recommendations uh movies music all different products even match.com uh presented at our conference on predicting who would be your best romantic m so I could go into like let's here the definition of machine learning and learn from data to predict and this is what the data needs to look like and this is the kind of insight you get but actually let's just jump straight to the to the chase here the value proposition the way it mechanically drives large scale operations which consists of many decisions is to order and prioritize from in the case of marketing instead of the highest risk rather the highest opportunity sometimes when I teach I have everybody stand up and hold a piece of paper with the size of their television so now they get them all in order by biggest TV down to smallest and a zero means they don't have a television right but they can still watch on their laptop and then I ask a yes no question how many people something like how many people are um paying subscribers for Netflix and then what you see is the kind of thing you see with all these use cases you see a bunch of yeses towards the top much more concentrated and then it Withers down to next to none of them by the end of the list or this is a small sample so it's not terribly conclusive but that's the gist right so for example if I were trying to Market something that had a close Affinity to Netflix I'd probably get a lot better bang for my marketing Buck by only containg the top third so that's the mechanics it's that simple you order the list and then you draw a line somewhere that's called the decision threshold so here we've ordered from left to right prospects for targeting marketing and this is a pro curve so it kind of makes sense as you start at the left side and following the top Arc this is the profit you're getting if you were to be contacting with marketing the customers in that order from left to right most likely to buy down to least likely you're going to get your better bang for marketing Buck towards the early parts of the list those most likely to respond and there's going to be more yeses than NOS up there and then you're going to have diminishing returns and to the point where you only start losing money and in this particular case it's a lose if you did Mass Market without targeting you'd have an overall lack a negative profit it would' be a losing campaign so you might be thinking okay let's draw the line here that's the decision threshold and that's where you would maximize profit but this tells a story right and it depends on the business context a business stakeholder might say no let's draw the line here for a medium-term uh goal it's this means we basically get to Market to uh whatever it's like 73% of the list for free we break even arguably a lot better than marketing to 100% at at a cost of $550,000 so that idea of ranking it and drawing a decision threshold that's sort of the universal name of the game and that's how all these different operations and decisions and treatments of us as individual consumers are determined right our experience in modern society is dictated by how we're treated and served by organizations in these ways and more and more the exact method I just described is indeed being applied to make those decisions millions of times a day based on that predicted outcome for each individual decide look at whether it's above some predetermined decision threshold and if it's high enough then you're going to decide whether to call mail proof test diagnose warrant investigate incarcerate set up on a date or medicate so this is this is how it works right pretty straightforward so let's let's go to a more complete definition um we'll turn to the book it's book machine learning for babies and toddlers which I highly recommend for babies but not really for toddlers um because you know the def definitions of the field are sometimes so lacking or vague or what have you let's turn to this practical applied definition which is technology that learns from experience and by experience I mean data data is a recording of previous events it's a long list of things that have happened and encodes the collective experience of an organization from which it's possible to learn that is to say to derive a par model which can then be used to predict the outcome or behavior of each individual an individual is a general concept it could be a human it could be organizational element could be a satellite that might run out of battery it could be a train wheel that might fail each individual customer patient business vehicle image piece of equipment other on that level of granularity that's sort of what differentiates it from forecasting which would be an overall like is the economy going to go up or down or how many ice cream cones are we going to sell this is who's going to be most likely to buy an ice cream cone so each individual gets a number usually in the form of probability and the higher the number the higher expected chance that they will Click by lie or die commit an act of fraud turn out to be a bad debt or any kind of outcome or behavior that could be useful for the organization in order to drive better decisions and that last part of the definition is of course super key because the number crunching doesn't isn't valuable unless you act on it so that's the deployment piece that's where we're actually changing existing operations which don't improve unless you actually change them so that's what ends up being the biggest CH unmet challenge is taking the number crunching and the predictions you get from that and integrating them so that operations actually change and thereby improve so in a nutshell the data comes in from the left and machine learning algorithms also known as predictive modeling generates that golden egg predictive model which then by Design is meant to be used in deployment for one individual at a time and you provide the input which is a bunch of independent variables the factors the things you know about each one individual and then it provides a number pertaining to the probability of whatever outcome you happen to be predicting for that particular project so for example in marketing you're saying hey should I spend the cost of contact should I spend $2 sending a brochure to this customer if I do what are the chances there'll be a positive outcome and they'll buy the um shoes so look prediction obviously a valuable thing to do so let's go to that credibility question uh how well can we really predict uh Nobel prize winning physicist Neil's bore prediction is very difficult especially if it's about the future and Jay Leno how come you never see a headline like psychic wins Lottery okay it's real simple the way you put credibility on this is that you don't have to predict ex with extreme high confidence or Precision or like a magic crystal ball predicting better than guessing generally more than sufficient to drive uh these large scale numbers games that we play with business business is a numbers game you tip the odds slightly in your flavor uh favor you get this great impact on the bottom line so let's do a quick back of the napkin arithmetic to show that let's say you've got a million prospects that you're going to potentially do Mass marketing to so no machine learning if it costs $2 for each contact then I'm going to spend $2 million contacting everybody and if the resp response rates 1% then 10,000 are going to respond and I'm going to get 220 back so I'm going to get 2.2 million after spending 2 million so my bottom line profit is 0.2
million great so how much better would it be if I targeted with predictive modeling well if I had a sample of say 40,000 representative sample I did marketing I waited I found out later who did and didn't respond that's the training data those are the examples from which to learn I know how it turned out and from that I generate the predictive model and then use it to score all million prospects and then order them from most likely down to least likely and then draw that decision trol excuse me decision threshold so now I've drawn the line I'm just going to Target the top Echelon the top 25% say and if the modeling was sound if the data was sound I might get something like a lift of three so a lift it means how many it's a predictive multiplier how many times better than guessing so if our overall all average is 1% this hot pocket has 3% it's a lift of three three times as many responses in general over that smaller portion so now if I just Target to that top 25% I save 75% of the marketing cost um and uh that means I'm in in this scenario anyway I'm forsaking all the potential sales there but there's so many concentrated in the top quarter now if I do that same bit of arithmetic that I did a second ago it turns out that the bottom line profit skyro rockets by a factor more than 5 to 1.15 million right no new product no new marketing creative right just more let's call it intelligent targeting um but hold on this model totally stinks right it's not highly confident about any individual it's not saying we know this person is going to definitely buy it's a 3% response rate but the difference is significant and then it pays off in this bottom line calculation so another way to define Predictive Analytics is a skunk with bling you're not writing that down so I call that the prediction effect a little prediction goes a long way predicting better than guessing generally more than sufficient to render a a great Improvement to the bottom line in um improving the efficiencies of large scale operations and it's built on these kind of discoveries from data so if you're observed using your credit card at a drinking establishment your higher credit rate RIS in the sense of being more likely than to to miss repeated credit card bill payments whereas if you're observed spending at the dentist um you're lower credit risk and if you buy the little felt pads to protect the floor how many people here have bought the little felt pads that well I'm really proud of you guys um I've never bought them myself but I should I got to get around on to that so you're a lower credit risk awesome if you like curly fries on Facebook you're more intelligent and if you skip breakfast and you're a male of some same some age I think a younger age range higher risk of coronary heart disease not necessarily because of the health benefits of the meal right they cons it's considered a proxy for lifestyle so if you're more busy living a high Pac stressful life uh then you're both more likely to be skipping breakfast and more likely to be developing coronary heart disease so but even if it's not irly causal the length there it's the correlation it's predictive if you know one it increases chance of the other and these types of insights serve as the building blocks the job of machine learning the rocket science part is to figure out how to put all those things together so like the TV size thing right that was pretty well correlated but wouldn't it be nice to consider a whole bunch of factors together at once for each individual that's the job of what the model does is to do multiple variables so I call that the data effect data's always predict as data scientists we can sleep well at night knowing that if we get the data together and ju toosee something that was known in the past next to something that turned out to happen later you know we're going to find these correlations to help predict and then putting them together can be something like this this is a business rule often embedded within a decision tree so this is based on um real data from Chase bank before they merged with ch B Morgan and it's um predicting whether you'll defect as a mortgage holder so are you going to go refinance at a competing bank so this particular rule is if your mortgage is within a certain range uh 67 to 183,000 and your interest rate is learn is bigger than 8.7% and the ratio of the loan to the value of the home is less than 87% then it puts you in a bucket which is 25% 25.6% chance of defection and then when you put a bunch of those rules together it's in the form of decision upside down tree decision tree you start at the Top If answer is yes you go left it gets you down to an end point this is about a third of the size of the AL of the optimal tree but relatively legible to human eyes it's just a way to consolidate a bunch of business rules together um so this is one form just to make a concrete of what a predictive model could can look like how it can operate um one really nice elegant way to scale this up is to have a whole population of trees and have them all basically vote that's very effective it's called an ensemble model neural networks are sort of a kind of Ensemble model anyway when you go to neural networks and other kinds of more numerical methods it becomes a soup of math and you lose it becomes more opaque you lose transparency it can be harder to interpret but sometimes depending on the context it can be worth it because you improve your predictive performance um they get a lot more complex than this right they get deeper that's deep learning and then this is a Transformer obviously uh do anybody here invent the Transformer because I know a couple of you did right here at Google this is a this is a Google talk right um uh which is the basis for generative AI okay so now that we can predict as well as possible using more than just one variable at a time then we deploy it and this is the kind of this is where the money is this is the use case um so for example UPS predicts tomorrow's deliveries in order to optimize them so at each of the Thousand shipping centers in the US they have this problem of how do I allocate all these packages and this Fleet of trucks maybe 50 trucks that are going out tomorrow morning and get them loaded overnight so they're on time for tomorrow's departure so that overall the number of miles driven is relatively optimal and the amount of time spent by by drivers and all the time a day requirements are hit right the challenge is they have um uh only partial information so there's a lot of uncertainty because some of these packages they don't know yet right they're not in hand at the shipping center yet and there's a lot of sort of information system problems and time zones differences and issues that come up so that there may they're just they don't have the complete picture late afternoon or early evening when they have to start planning and then actually loading the trucks overnight for on time departure so they augment the known packages with predicted ones and now they have tentative you know tentatively presumed basically so now I have a more complete pretty reliable picture of what the likely deliveries are and they can get it all done in time and this had a dramatic Improvement um the technology I just described is called package flow technology and then in conjunction with Orion which is the time newly introduced basically GPS driving instructions which which they go hand inand because one thing to say hey this truck has been well packed enough that potentially has an optimal route tomorrow it's another thing to say it'll actually be driven optimally right so now we're prescribing the driving directions to the drivers and together this amounts to an overall savings that UPS enjoys now of 185 million miles a year $350 million 8 million gallons of fuel and 185,000 metric tons of emissions so um now this didn't come for free in the sense that it seemed like a great idea and then they just did it right there were a lot of battles getting it approved and then there were also a lot of battles getting it deployed in fact it's it's the leading and then basic almost trailing um story in my book the AI Playbook um where you know they did a pretty good job being proactive and anticipating the challenges of what it would mean to actually get it deployed on the scale of things relatively very well but they didn't hadn't quite swung follow through with the swing well enough and they had to kind of backtrack so there was a bunch of drama it's like a riveting soap opera I'm really hoping that you all uh read the book um but along those lines why is that such a challenge what's the deal right why don't they get deployed because it's about probability in a nutshell predictive models the the predictive scores are typically probabilities you know a number between zero and one or zero and 100 same difference um so you can consider it a probability calculator but the world's not that awesomely excited about or comfortable with probabilities it's Arcane it's it's intimidating it's it's theoretical right so case in point Empire Strikes Back C3PO right very helpful I wish I had one and he's like sir the chances of navigating this asteroid field are 3,160 again to one against right and then our beloved hero Han Solo never tell me the odds right and I'm like thanks George Lucas right for helping further stigmatize now you would think maybe on the flip side we've got Moneyball here right which glamorized the union of the Beauty and the geek successfully bridging that Gap getting the number crunching actually deployed right you you can probably guess which actor played the data scientist in this movie um so and by by way of doing this the Oakland A's baseball team did a lot better than anybody uh expected given the budget of the team but this movie and even the book are really the epitome of glossing over the math we got to get past that and in the case of predictive AI deployments it comes down to these three things so this is the semi- technical understanding that you or if you're a data scientist your stakeholder needs to wrap their head around and it's really straightforward it's what's predicted how well and what's done about it it's super accessible much more accessible than High school algebra more pertinent interesting cool right like it shouldn't be a black box we need to get involved in this level of detail right which to data scientists are known as the dependent variable metrics and the deployment what's done about it so the first and third of these what's predicting what's done about that defines the use case right that's why machine learning is you know Harvard Business Review said it's the most important general purpose technology of the century and it's so widely applicable because there's so many pairs like that like you predict this thing and it helps decide on this operational decision right there's so many ways that it applies across sectors um so let's just step through that with I've already been talking about targeting marketing so you predict will the customer buy of contacted and then what's done is you mail it for for sure we're not quite done but this sort of defines at least in Broad steps the action the actual actionable particular way that you're going to use this awesome number crunching to actually improve the business right so essentially that's the first step in Reverse planning we want to plan for the deployment for what we're predicting and what we're doing about it but in what you'll see is the second step of the six-step Playbook as I formalized it we need to be more specific about the first of those two we need to be very we have to get the business side involved and defining what data scientists call the dependent variable so instead of just saying will they buy it's okay let's let's be really specific here if you send them a brochure are they going to buy within some set time window of 13 days with a purchase of at least 125 after shipping and not return the product um you know for refund within some other established time window let's say 45 days so all the caveats and qualifiers right it's one thing to say we're going to act on these predictions but predictions of what you have to be really specific often at least three times as many caveats and qualifiers so sort of a long runon yes no question uh it's often a binary yes no question um so you want to get U everyone on the same page because the thing you're going to be doing doing about those predictions only makes sense with respect to predicting exactly the right thing with business considerations data scientists can't do this alone in a in a vacuum in a cubicle or what have you they there's got to be deep collaboration to is to flesh out this level of detail so this is a example of what I mean about this overarching theme of deep collaboration and the stakeholders ramping up on this level of semi-technical accessible understanding so that they can actually collaborate successfully in that way put it another way if you're data scientist um if your stakeholder doesn't get their hands dirty then their feet will get cold right because it's it's too abstract they don't understand it they're not going to be ready to authorize deployment they're not involved enough now of these three kind of just touched on the first and third so now let's talk about metrics how good is it how good is AI how well does it perform and it turns out that for most projects what happens is the data scientist makes a model and only evaluates it in terms of technical metrics Precision recall area under the curve how many people know what area under the receiver operating characteristic curve is okay good if if if only if only uh 20% of you are data scientists then you're the Right audience because I need to first talk to business people and and and then data scientists or or to business people through data scientists so um even accuracy is just a technical metric and the technical metrics only tell you the abst abstract pure predictive performance of the model how well it does relatively in comparison to a baseline like random guessing rather than what we really need is the absolute business value you could get depending on how and whether you deploy the model which are Super straightforward metrics top and bottom line profit uh Revenue profit savings returns whatever it is the kind of stuff that any business person is familiar with it speaks their language it speaks to the actual purposes and strategic directions of the organization and the translation from one to the other is almost never to be seen um and one of the reasons for that so this kind of curve that we've already gone through um a profit curve like I've been in the field for whatever 32 or 33 years and I've probably seen these curves like three times other than in my own slides like it it there there's a certain um pervasive problem that we haven't quite gotten past here and then similarly a a loss curve which often ends up being a u in both cases there kind of a goalie log Zone not too much too not too little um depending on how you can I've basically seen that zero times but these are accessible um and they uh they speak to the business needs now I think that the biggest problem and the thing that's preventing this from happening is that you can't just look at a model and say it's worth a million bucks so you can with these abstract technical metrics you can measure how well it does in a vacuum abstractly but when it comes to the business metrics it depends on how you use it how you're going to act on it how it's going to be integrated and deployed and therefore change business operations that and that's why I'm going to show just a couple quick pictures of my early stage startup good or AI where we have the ability to draw profit curves because we allow the user to parameterize the deployment and that's what it takes so you can put whatever business metrics Define them however you want um you can move that decision threshold around see how it changes competing metrics at the top and create business inputs and whatever pertinent business inputs make the difference which are all sub totally subject to change partly because of uncertainty partly because of business changes that are intentional and partly sometimes there's a subjectivity to them so in other words what you need in order to make this much needed abstract move or um sorry fundamental move to business metrics is a very particular type of specialized user interface to visualize the effects and get an intuitive sense of what it means to move those change those business inputs and see how it changes the story and then within a story change the decision threshold so this is the this is why we created good or AI so if any of you are working on predictive use cases and would like to be a trial Early Access customer that's where we are we're super early stage and we're just moving to trials with sort of a couple dozen companies so I'd love to to talk to you and also that particular sort of problem not the solution I just mentioned but at least the problem is laid out in my mit2 slow management review article uh shown here and uh also a little more long- winded in the metrics chapter which is chapter three of the AI Playbook that you have there so before I wrap up and we go back to some um discussion with Brett let me actually cover the six steps with based on what everything I've told you they're super straight St forward the first three simply correspond to those three things I've already been talking about that need to be understood by stakeholders what's predicted how well and what's done about it not quite in that order so the first is what's the deployment goal in other words that pair what's what's predicted and what's done about it um in other words you're starting you're planning you're starting with the end goal you're planning in reverse and then the second is get more detailed about that what's predicted part right the definition of the technical objective that the data sciencetist pursuing with the number crunching and then number three is the metrics both the technical and business metrics that are pertinent and where they need to land before you could deploy and then the other three so those are kind of pre-production the other three are the main production steps which have always been the same all data scientists know these it's always been this way ever since the 1960s when they were first using you know doing credit scoring and targeting uh marketing with predictive models which is prepare the data train the model over the data and deploy it so training the model of the data that's the machine learning part right that's the actual rocket science deploying the model though that's the Pay Dirt right that's the part that matters that's the whole point that's where you're actually not only generating potential value but capturing it that's the thing you're planning from step one that's the part that ends up being the biggest challenge and the thing that most often fails to get done but the world is super focused on the awesome rocket science it's like we're fetishizing this stuff and who wouldn't I mean it's so cool most data scientists including myself got into it because it's so awesome right I mean the idea of automatically generalizing from a some set of previous examples and deriving patterns or formulas that actually do hold in general over new unseen cases that's a like well- defined sense of the word learn right learn is a human word but now we've defined it in a specific way that a computer can do successfully that's the most cool awesome type of stem period like that's why I got into it a few uh decades ago um but more recently as a business Consulting I'm more focused on step step six and that that lack of focus is the problem right so data scientists are like look this whole thing you're talking about that's not my responsibility that's a management issue my expertise is to do the number crunching make the model its potential value is you know a no-brainer speaks for itself of course the organization is going to act on it get it deployed use it right that's not my job I do the number crunching whereas the business professionals nope that I delegate all that technical stuff that's why I have data scientists right so the faucet and the hose fail to to connect this is the routine failure for most new machine learning projects um at least outside big Tech I don't really have the data but it's impossible to imagine there aren't that phenomenon doesn't get that Pitfall doesn't get fallen into at least in some corners of a company like Google so it's so ironic right it's like we're so excited about the rocket science it's like we're more excited about that than the actual launch of the rocket business professionals also put up their hands and say well to drive a car I don't need to know how the engine works I don't have to look under the hood okay so that's totally true and I personally don't know how and I mean I know the general concept of internal combustion but I open the hood of my car once and I was like whoa look at all the parts like I don't know where the spark plug is but I'm an expert I know momentum and friction and the way the car operates and the rules of the road and mutual expectations of drivers the analogous thing here also holds true there's a certain expertise semi- technical understanding in order to drive machine learning project projects successfully through to deployment which is not the rocket science part but it takes more than a half hour so that's the point of the book right it's a book's worth of material the book's first objective is to yes it's organize around the six steps by six main chapters but the main point is to deliver that semi-technical understanding to business readers and also probably help data scientists realize the sort of scope of what their clients better understand if the thing's going to get deployed so these projects predictive AI Enterprise ml they're a Consulting gig not a technology install and they depend on deep collaboration across end to end a a Project Life Cycle I've defined in terms of those six steps I coined a really nice little five letter buzzword bis ml trying to Brand the concept and get it out to the world that there does need to be a very specialized practice but more to the point that collaboration across those steps must be deep and involve business side stakeholders people in charge of the operations meant to be improved by a predictive model who have ramped up on that semi- technical understanding thank you very [Applause] much that was great thank you thanks Brett as a Netflix subscriber I've learned that I need a larger TV so that was oh good yeah yeah yeah so it's valuable for you yeah um so um wanted to get so read the book uh and had a bunch of things one of the first things that struck me is you you really start with the don't get attached to the solution but but focus on the problem yeah and you gave some examples of it's like you know we're not you know building this with AI we're big we're solving this problem and we'll use AI to solve that um at the same time like I I you know occasionally open open up a web browser and I see that AI is kind of a thing you see a bunch of companies that are doing Ai and we even we even had a kind of a a thing earlier called IO and AI got mentioned once or twice at that thing so with all the emphasis and the hype and everything around this how do what is your advice for not falling into the it's the AI solution but the problem with AI yeah I mean so solutionism is you know all to the to a hammer the whole world's a nail um right fall in love with the problem not the solution AI first means using AI last um can't remember who I'm quoting there but um uh this is a pitfall like we all like what's your AI strategy but we you know you wouldn't say that what's your Excel strategy right why is that happening with AI well because it's a big exercise in anthropomorphism right the ai's original sin was calling it AI and if it were up to me we would use that term only for science fiction philosophy and maybe anthropology of like hey these humans are trying to replicate themselves um I love it in science fiction for example but if you're trying to define a engineering goal it has to be well defined and intelligence is nothing but a if if anything it's a word that defines humans that are very particular in very many complex ways um but that side narrative it the idea that we're getting things that not only have human level capabilities in one narrow area but in general right artificial general intelligence which I like to call artificial humans you could on board them the same as any employee and let them rip autonomously um that's just such a compelling narrative and we can't quash it but if you all have any ideas to to uh to rename the field I'm on board with you I think uh another thing that um really caught me was sort of the need to kind of measure the business impact from the work that you're doing and uh I work with generative AI with sort of developer productivity and this is kind of a consistent Challenge and I imagine that there's some some things that you can apply ml to um like marketing and such which is a little more straightforward and like understanding like what is the business impact um and then there are things like developer productivity which is notoriously challenging but I imagine there's a lot of other business pro processes that are difficult to understand you know the the actual business impact from the actions that you're generating like um do you have great examples of like where you've seen this done well or complicated situations where that's been established yeah yeah I got a couple here um but um so yes the antidote to Hype is focusing on value that is actionable change to operations or introduction of a new concrete operation um which also means measuring the value right you can call it metrics intelligence is not a metric right it is a subjective concept and anytime you define it well enough to measure it it loses its charm once you get the computer to play chess or drive a car it's no longer AI the way it was intended in the first place so you've got this the goal post intrinsically keep moving we will keep having um AI Winters so long as we call it AI um but uh generative Ai and predictive AI or Predictive Analytics and I mean these are perfectly well defined things basically um and they both have value the bis ml 6ep Playbook is defined is is customized specifically for predictive use cases but the general concept applies for generative generative AI deployment just as well in the sense that from the get-go you need to have a concrete idea of how it's going to deliver value exactly what operations is it going to enact um and then how are you going to measure that value or Improvement you get there and then you have to actually follow through and measure it right which in the case of generative which is of course much newer predictive AI I'd like to say is is older but not old school lots of untapped opportunity there generative AI it's relatively new and we are seeing some um use cases I I I referred to a couple in a recent Forbes article um and then just to make sure I remembered the the specific um uh examples of them since i' mostly talk about generative here oh yeah so Ali Financial Bank improved its marketing Camp um campaign uh creating the uh contents of these campaigns the creatives uh with a Time Savings of 34% so they actually measured it and then reported it publicly and the thing that's really amazing is that that's very rarely done like I've barely seen that one other one uh maybe the only other one that's actually crossed my desk um is a Fortune 500 software company um for customer service and you can imagine this works really well if you're a human customer service agent um doing chat service and then you've got generative AI like pre-drafted and then you can use it or not use it or or or edit it or what have you it turns out that improves the number of issues resolved per hour that's a nice metric by 14% definitely not nothing right it's not a robot that's going to do everything for you but that's certainly not nothing and maybe more interestingly for novice and low skilled workers improved by 34% which kind of makes sense because they get they needed that much extra help on the job um so that is to say that what generative generative uh is not a reference to any really to any particular technology it's a reference to the way we're using machine learning to generate new content items writing video graphics right so it's generating that that's the use case right the underlying technolog is machine learning um as with predictive but instead of predicting for each let's say customer it's predicting hey how should I change this pixel I being the computer as I'm iteratively uh generating a new image or what should the next word be or token whatever on that level of detail so it's just you're using machine learning probably more sophisticated machine learning over bigger data in a different way um the irony is that although the results are amazing um dumbfounding uncanny right so seemingly humanlike often times but ironically that actually lends less to autonomy in the predictive predictive use cases because you're improving that's the technology you turn to predictive to to improve your largest existing operations um so for example for fraud detection should I should the system automatically on the Fly approve this credit card charge based on predicting How likely it is to be fraudulent that's fully autonomous no human in the loop happens instantly but generative doesn't work that way right um You got to proofread everything it generates so leads me to a little bit of the trying to lure you from the Predictive Analytics into the GI conversation are you saying is this a job offer trying trying to establish like what's your take is gener of AI more hype than than value or oh yeah it it's uh I I told Brett talk in the green room I told Brett if he asked me that question I would just say yes next question um no I think it's I think it's overhype by factor of 10 or 20 right so if it's 10% as valuable as the hype says that's really really valuable right but the hype I believe underlying the typ one way or another is the AGI narrative the fact that it's going to become General human level capability I believe that as astounding it is as it is none none no human no technology progress represents a concrete step towards AGI that's my belief I don't I don't mean to say that we're not algorithmic or necessarily that we could never do it but I don't think that we're headed that direction specifically um right so I'd put in another way to try to sort of temper the hype because I do think that that that me that narrative underlies the hype so putting it another way um it's become clear that these neural networks scale so well over lots of examples of human behavior like a human wrote this word next and then R wrote this word next right so it's learning from that human behavior to emulate human behavior extraordinarily well in an unprecedented way I was in the natural language processing research group of Columbia in the 90s I never thought see anything like this it's unbelievable but there's still this overall question of it's like well how much of the overall human capability can be reversed engineered by observing a limited amount of our Behavior like a lot of our writing right how much well obviously a fair amount but there's got to be a ceiling on that let's go to some of the audience questions uh see the next one was along the lines um it's what do you think is the biggest current opportunity area for product or service to be improved by ml um it's a great question um so there's this weird there's kind of this long tail of of use cases right so targeting Marketing in terms of marketing the other sort of main use cases is what they call churn modeling so predicting who's going to cancel or defect in order to expend a retention offer like a discount that you couldn't afford to give all your all your your customers uh prediction is the only recourse for targeting that and that's probably the other main hot marketing application um probably the lower hanging fruit usually the higher potential returns depending on the business context um it's obviously credit scoring and fraud detection so these are sort of top of the tail and then there's a million other ones I'm not even getting into Healthcare but just like you know where should I drill for oil um which train Wheels should I inspect which satellite should I inspect that might run out of battery there's just immense long tail so the particular use case really just depends on the organization what are the largest scale operations whatever it whatever that large scale operation which generally consists of many decisions well it's already being conducted so by definition you've already been collecting or likely collecting exactly the data that you need enough positive and negative examples from which to learn and that's what the modeling method does it use it leverages those historical cases um so there's not really it just depends on the company and and the context um but I would say that in general you know it's only being tapped especially cons in consideration of how often it fails to actually reach deployment and deliver value um those failures get adeptly often or usually get swept under the rug very well um in no small part because of the AI hype but that's not sustainable um so there's a huge amount of untapped opportunities across sector across organizations um I think I'll just sort of pivot that from that question to say that I think that the biggest change within the predictive space um that's coming or should be coming um is to organize lines of businesses or even companies around these predictive use cases because you're going to get there eventually all all roads lead to these the original ml app uses right um if the organization grows or the operation grows sufficiently large but the way it works now is it's like almost an ad hoc afterthought so the company's doing its thing it's churning away and then the the data that now you're going to Leverage is collected as a side effect of doing business as usual so you haven't really created the infrastructure or the data collection that's really meant for this it just so happens it's a lot of work to take that data and put it in the right form and format to then make it useful but instead new initiatives new large lines of business even new companies could be or from the get-go of how exactly these uh machine learning um Endeavors are going to be integrated later so plan it instead of it being sort of an afterthought hack with a certain hackness to it you know have it integral from the get-go one thing you just brought up was uh talk about the difficulty of actually getting things deployed and you actually do some informal research I think on your site about asking you know like why did this not get deployed or such but like the number was a little bit depressing when I I first saw it like what what percent of of models don't get deployed yeah so uh my partner firm very small boutique uh consulting firm rexer analytics does this does one of the biggest um periodic data science surveys and they let me participate and help craft add more questions pertaining to that like the deployment success or lack thereof and then yeah when we all looked at the data together we were all like oh my God right like it is like woo um and then another hand it wasn't that surprising because I had done a very similar one through KD nuggets which is a big data science portal uh like a year prior to that um and in general I have the sense right I've been running these conferences which are all about these um use cases and a lot of them get deployed and they show the results after deployment but a lot of presentations uh at any data science con conference you're kind of like well did they deploy it yet did they actually measure the value and it you sort of get a feeling over the years that M maybe things really aren't actually deploying as much as people would kind of want you to believe and and I think the reasons that you listed actually you know I've witnessed these types of things happening where it's the exact disconnect between I've built this and then you need someone else to you know it becomes down to a business decision and the disconnect is what if you can do all that work up front you can make sure that you have a successful deploy yeah yeah um more questions from the audience uh what has been your biggest learning experience with feedback loops and launching ml products that change the data landscape they operate in oh um that that's kind of wonky I don't want to bore you guys but like like um you know a lot of things come up so when the biggest B biggest technical bottleneck the biggest technical challenge is the data prep so the core rocket science the actual learning from data once that you know once you've got your data set in the right way which is typically you know for the these types of projects it's just a two-dimensional table one row per example and one column the dependent variable the thing you're trying the prediction goal right off in the rightmost column um next to that the thing that you found out later whether it was fraud fraudulent whether they responded to marketing whether they canceled Click by lie or die or whatever you're trying to predict um getting into that form and format based on the way it is now is hard and one of the hardest part of that is you kind of have to roll back time cuz you need for each row of that data is you need to have what you knew at the time you would have lik to predict not any not including anything that was found or influenced by anything that happened after between that time and the time when you found out that they did buy or that they did cancel or whatever so that rolling back of time thing depending on context that can be really really hairy it's called a Time leak um and uh so one way to solve that is just to take these snapshots and I I've done that with a couple clients where it's an high enough sort of online operation like it was a social network be this was a old project before Facebook one um it wasn't Facebook um maybe it was also for an online dating client but just like there's so much going on that you just have to like collect snapshots for a couple weeks um and then you don't have to roll back time because you're just snapshotting it you're just sort of logging it um it happens so those are the kinds of considerations um that that's a intrinsic irony is that the actual fun part the cool part the actual rocket science of creating the model um is not nearly as challenging as getting the data clean and together in a meaningful way in the first place I think we have time for one more speedr question no um yeah llm based projects have unique challenges for ML development life cycle is this covered in the book look and if not what exists in the industry or on best practices uh I don't know man the llm stuff is so new so running Enterprise projects um I mean as so no the book is focused on predictive um and bis ml is is specialized for predictive projects um creating an analogous more formal and detailed um framework that applies for generative AI including large language models um I think is kind of TBA um right I mean uh it's going to be a different thing um we may have to suffer some disillusionment before something like that takes an po popular hold um but I haven't seen um uh I haven't seen an industry best practice that looks sort of like positioned to take hold for llms yet and while the book is uh on Predictive Analytics I would say a lot of the business practices and sort of the alignment that you mentioned I think is actually relevant uh yeah the broad Concepts totally totally you got to plan for the the business value and the operational change and and the getg go yeah all right you did so well on the speedr I think we get one more question oh cool uh what ongoing research Partnerships between the educational institutions and corporations would be of most interest to you which of today's research projects do you think will be the most impactful for this Workforce well my look the the hill I'm going to die on is that educational need for non- dat scientists and at the same time that entails an education for data scientists who kind of it when it's very easy to see the forest for the trees when you're actually doing the number crunching and not Rel look we got to take a step back these are the broad Concepts that to me as a data science may feel like high school algebra but from an enter price standpoint from a cultural standpoint okay let's let's let's give them a copy of this book because we need business side stakeholders to get what I'm calling a a semi- technical so to business people at first it feels oh this is super technical but then when you look at it actually no it's not that bad and then from just frankly from a data scientist's point of view they're going to be like that's not technical at all so that's that's why there's this Gap and that's where there's an education need all right I think we're about at time so thank you very much for joining us and big round of a hand for exi gold thank you thank you [Music]
2024-05-31 03:37