2021 MIT Digital Technology and Strategy Conference - Dina-Katabi
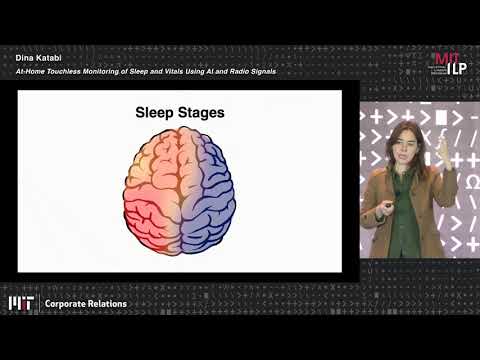
uh so our next speaker is professor dina katabi she is uh professor of he she is angel and uh victor b professor and also makassar fellow she's also one of the youngest members of american academy of engineering she's going to introduce her wonderful research innovative research in at home touchless monitoring of sleep and vitals using ai and radio signals let's welcome dina [Applause] hello everyone my name is dina kitabi i'm professor at mit and i work in the area of machine learning that analyze radio signals and analyze them particularly for in the context of two types of applications one of them is health care and the other one is iot related applications so i'm really happy to be today with you and it has been long time uh since we got to present in person so it's great to see all of you guys here so when i tell people that i work on application that can sense your sleep your vital signs and all of these kind of like physiological signals people think about wearables so they think oh computer scientist this is like a new wearable technology and i tell them no actually it's like a new concept that we think about in my group at mit which we refer to as the invisibles so if you think about the last two decades like basically we saw the rise of the wearables we think that the next two decades probably we are going to see novel technologies that we refer to them as invisibles basically can we monitor things that are related to our activities behavior emotion vital signs sleep all of that stuff without asking people to wear sensors or to do anything just let people live their lives and let the environment be smart and be able to analyze all these physiological signals in a touchless manner so how do we do this so all of you guys have a wi-fi box at home in fact now you are sitting here you are immersed in radio signals all types of these radio signals whether wi-fi cellular etc so what we do in my research group is we design new machine learning algorithms that analyze radio signals that bounce off people's bodies and from that you can get breathing heartbeats sleep sleep stages movements activities and many other things in a touch-less manner we call these new boxes they are very much similar to your wi-fi box it sits in the environment and we call them emerald wireless box so i'm going to start by showing you an illustrative example so in this video what you see is a home the wireless signal of course spread inside the home to reverse walls and occlusion it it reflects of our human body because the body is full of water and some of these signals come back to our device we analyze them using machine learning in this case we detect a full we can alert the caregiver via text email or a phone call let me show you some of the experiments from our lab this is actually one of the early experiments that we we did on tracking people completely contactless through walls and be able to see how they are moving so you see one of my students standing in our one of the offices that we have in cesaire and what you see on the side you see this red dot here is where the device thing that he's standing you see also an arrow that is pointing at the wall this is where the device is in the adjacent office as he moves look at the red dot and how the device from the other office through the wall is discovering his motion completely touchless there are no wearables there is nothing so we can track his motion in a completely passive way he doesn't have accelerometers cell phones anything like that on his body and we can do it just because his body changes the electromagnetic waves around him and we can analyze those changes so let's see it again and we are able to localize and position this person as he moves in a completely contactless way through a wall and the accuracy of this is we compare it with the viking motion capture which is a like a whole room full of infrared cameras with a marker on the person and the accuracy of positioning this person is 98 so it's pretty high now if you have such a device in someone's home you can know how the emotion you can know for example when they sit on the tv couch and so that you can use it for smart home environment to tune the tv to their favorite channel but you can use this also for variety if health related application because they prove it for example fatigue and it's very very important and shows up as limited motion and staying in the same place for a long time but not only motion we can actually measure other things that you might not imagine that a wireless signal in the environment is a way to measure someone's sleep so i can analyze the wireless signal in your home and from that not only i can tell whether you are asleep or awake i can tell actually when you enter the stage of dreaming the rem stage without touching just by analyzing the signal that bounces off your body so today if you want to understand the sleep stages so sleep stages are the result of changes in our eeg signal and when when we go to sleep our brain waves change and we enter different stages awake light sleep deep sleep and rapid eye movements rapid eye movements is the stage in which we we dream so of course understanding sleep stages is important to understand sleep and sleep disorders but it was also very important for a variety of diseases just to give you an example depression for example is associated with rem starting too early in sleep alzheimer's there are there is medical research that associate alzheimer with the slow waves in the brain during deep sleep so if we can understand sleep stages not only we can understand whether people are having sleep problems or insomnia but we can get much deeper information about variety of diseases today if you want to understand someone's sleep stages what you have to do is to send them to a sleep lab they put these electrodes on his head and body and they asked them to sleep like this so you can tell he's not happy and in fact when i first started working with medical doctor and they told me this is how they evaluate sleep i was really shocked because i mean can imagine that for many people this might not be a representative of their sleep because they are sleeping with all of these electrodes in a new bed in your new environment with all the sensor on their body so it's not very representative but it's not just that also you cannot measure it repeatedly you cannot send someone to have all of this on them and sleep in a sleep lab in like for a long period for example if you want to detect how a drug is affecting their sleep and sleep behavior so here's what we do this is our device it transmits very low power wireless signal 1000 times lower power than wi-fi analyzes the reflection using machine learning and neural networks and spit out the sleep stages throughout the night so you can see he's sleeping in his own bed in his own bedroom very uh non-obtrusive and we can do this every night and not only this we can do it accurately we did a study with math general hospital in which we analyzed our system in comparison to the gold standard which is called psu and we also looked at comparison with fda approved devices for measuring sleep based on eeg as we mentioned and actually is pretty accurate the accuracy on characterizing every six 30-second epoch is 80 which is pretty high because at the end of the day sleep characterization sleep staging is based on a technician looking at every 30 seconds saying light sleep deep sleep and if you take the same signal from the same person on the same night and give it to two different sleep technicians the inconsistency between them is about 83 percent so 80 is actually comparable to what you get with fda approved mechanism so okay so we can measure sleep without touching your body and we can also know your sleep stages what else can we measure using these radio signals so this person is sitting like you guys here and he's reading what you see on the screen is nothing but his inhales exhales we ask him to hold his breath and you see the signal stays at a steady level because he exhaled he did not inhale and of course it's not just visual we compare this to fda approved devices for modular respiration in this case is a chest belt and the accuracy is 97 percent and we can zoom in on the signal so this is the same breathing signal that i showed you before you see the inhales you see the exhale you see the blibs on that signal those blips are his heart beats so this electromagnetic waves that are around us they are actually very sensitive even the pulsing of our blood leaves the signature on those electromagnetic waves and what you need to do is to reverse engineer that to understand the breathing of someone their pulsing their sleep their movement all of that stuff now uh at some point i was talking about this new technology and i was in the audience it happened to to be somebody from one of the pharmaceutical companies called leo farmer and they were particularly very interested in itching and scratching he came to me after my talk like this and he said oh this is great but can you measure scratching using wireless signal today the only way we can evaluate how bad someone's itch and atopic dermatitis is by asking them to rate it to score it on a level from 0 to 10 and you just ask people and you get something that is very subjective because my like me saying that my scratching is six and maybe very different from your sex may be very different from some other person so if they can measure scratching they can actually objectively measure that and characterize each level and at the time i said i don't know but if we can get pulsing maybe we can get scratching so let us try and in fact we worked with them and we were able from the bouncing of the radio signal in people's own bedrooms and uh of course we don't need light or anything like so we look at their nocturnal scratching and we can actually evaluate it as accurately as if you had a camera there but of course you don't want to put camera in someone's bedroom in reality so we did it for the experiments and and let me show you this video so so this is from the labs this is one of my students and with this very similar experiment so here the the classifier says no scratching but as he scratches you see that he says it's a classifier says scratching but if the movement is not scratching like when he puts back his hand it knows that this is a movement but is not scratching movement and in fact we can look at the false positives the false negatives and as you can see the privacy the precision and the recall both are very high around 90 percent so i want to say a bit about how it works like what is the underlying technology here now of course i don't have time here in in this short meeting to tell you exactly the details of the technology but just at very very high level so basically we transmit as i said very low power wireless signal now you know that wireless signal traverse walls and actually they reflect of our bodies because as i said the body has water and as a result the signal reflect of the body but this minute reflection imagine this is reflected of the body traversing walls and occlusions and it's very minor to reflections and now it comes back to us and we need to analyze them now at a high level one thing that we all know and even like uh people who are not in like wireless expert we all know that distance is equal to time multiplied by the speed of light okay that's a very simple equation so if remember that dot that was moving like positioning someone in space so if we can analyze these my new signal and from that understand the time it took the signal to to go and come back then i can multiply that time of light with the uh the speed of light and i can get the distance of a person and then i can localize a person that seems to be very simple and in fact this is actually underlying something like radar for example we all know about radar and like you track aircraft in the sky the principle like that are used in radar now unfortunately it's not that simple if it were that simple people would have done this long long time ago so apart from the fact that we cannot really blast power like cradle like it has to be very low power so that it is safe for all people uh the other thing that is very important is that actually the sky is empty okay so you have like few aircraft there but it's mostly empty like when you are talking about an indoor environment there are so many things that reflect these wireless signal not just our bodies and that create a huge uh challenge so this is what we call like if you come like me from a background in communications this is called the multi-pass effect so i come from communication from wireless cellular networks and communication system and in that case we we tell you that the signal bounds off everything and that creates a multi-pass effect so as i said the signal not only bounces of the person but it bounces off many things in the environment and also could bounce first off an object and then off my body then on off another object and start getting all of these fictitious target so you don't get just one reflection you get so many reflection and fictitious target so these are the multi-pass effect and then you have to ask yourself now how to how do i just take that signal and from that extract the human body and not just i want to extract the human body again i the dot is good i want to position someone but that's not the only thing i want to do i want to know also whether they are asleep or awake and i want to know whether they are in realm in the dreaming stage versus other stages so i want to ask many other more complex questions than just position how do i do this any guess okay i'm sure all of you guys have heard about advancement in machine learning and url networks and we have shown over the last decade that neural networks have completely revolutionized areas such as computer vision and natural language processing can neural network also revolutionize the way we think about electromagnetic waves so this is what we do we do it we take these radio signals we analyze them through a neural network that is particular custom neural network and then we ask questions to these neural networks we train them to answer those questions such as like is this person asleep or awake and if he's asleep is he in deep sleep light sleep or what is their pulsing all of those questions now of course this is not very simple this is not just you're taking your neural network that you would use for analyzing images of cats and dogs and applying it here there are many differences between the two types of signal between electromagnetic waves and images there are many similarities but there are also differences so i don't have time to get into the details i just want to show you one thing that is really important if you really want to take a neural network and to ask like question make that neural network take it to a new environment and be able to use it in my home to analyze my sleep in your home to analyze your sleep in chris's home to analyze his his sleep that it has to generalize across people and across homes correct how do we do that so say that you trained your neural network with some set of people like say bob and alice and many other people so those are the training people and then you took your neural network to a new person chris and this is a person in new home a new environment so we call these like the training examples the source environments and chris is the target environment and now you want this neural network to generalize and one of the problem of course i told you radio signal reflects of everything in the environment so they don't generalize well to new environments so you have to design your neural network such that they can remove extraneous information irrelevant to the task let's say the task was like to monitor someone breathing or sleep this is the relevant information and irrelevant information are the information that are related to the environment so of course how you do that is quite complex but i'm just going to give you the first initial idea what we do actually is more complex than that this is the only slide that i'm going to show some like technical information like uh so basically if you are taking if you are doing machine learning then you are taking some input whatever that input in our case is radio signal you have some neural network typically you have an encoder you are trying to change your input to some representation that is easy to work with so let's call this the representation e of x and then you apply a predictor in our case let's say that you are you want to predict whether someone is sleep or awake or whatever you want to predict now what we want we want this representation this intermediate representation to have the relevant information but to be free of extraneous information so here's what we're gonna do we're gonna make this work like a game so we're gonna add another neural network which we call the discriminator the job of this neural network is to look at this representation and try to predict whose home that was okay so the discriminator is going to look at this is going to say this is alice's home this is bob's home this is uh chris's home so he's going to look at all the things that are irrelevant to the task that come from the environment and what we're going to do we're going to train this combination of multiple neural network in a star that is look that what we call an adversarial game so the objective of that game is to make the discriminator lose at the end and make the predictor win so eventually the predictor will be able to predict while the discriminator the the neural network that's trying to extract all this irrelevant information is just gonna lose will not be able to extract the irrelevant information and we can do that through a training function that this combination of trying to uh if the predictor loss and the negative of the uh discriminator loss okay so that's what i'm going to say about how it works now let's see what we can do with it so i showed you that we can track someone's location and you saw this video but when he was moving you saw the dot you know where he is but you can't tell whether he's standing or sitting like you look at this dot you know where he is but you don't know is he standing or not also when he was moving he was sliding you didn't see like whether he was tipping with his right foot or left foot so what we can do this is our older version now here is our new system our new system can get the full skeleton of the person and this is swu and i'm going to play this video this this uh image up there is just inside the room just for you so you can visualize and the big frame is the white what the wireless device gets okay so let's play this video so you see when he sits the device now sees that he's sitting and you can see like when he stands actually it knows that he's standing when he's moving it knows when he advances the right foot when he advances the left hood so not only we are getting a dot through a wall now we are getting a full representation of the human body through a wall effectively we are getting a form of x-ray vision like if you think about if you read about superman when you are a kid and we we are moving taking one step closer to that superman seeing the full skeleton of a person and their movements through a wall so we also look at whether we can predict their actions so let me play this video here so you see like when the person for example try to emulate combing his hair the skeleton is combing his hair and the machine learning model says can be the hair when he tries to emulate drinking water you can see the skeleton looks like he's drinking water uh and then he tries to like pick up something and put it in his pocket you see that in the skeleton so we are getting very much information that is related to behavior and action now there are many application of this kind of technology and in fact the way to think about this technology is really it's a novel invisible iot technology it's like these iot devices now we actually we have them deployed in people homes and they extract information about health and behavior and one if the biggest application that we are we have partnered with many institutions and both in pharma and in hospitals as well as other institutions in the healthcare and they have been using these devices to analyze diseases and analyze how how people behave and different biomarkers for different diseases i'm going to talk about some of these experiments in actually with people in people's own homes within certain diseases now let's look at how effective that is so this is our device and as you can see i mean it's just like a very non-obtrusive device it's on the wall and just the person can forget about them and of course as i said it's an iot network this is a device in the home but it's connected to an iot network i want to show you first some of the results from our parkinson's study because this was one of the earlier studies that we started with so let me first show you this example so this patients are you can see the green rectangle at the top this is our device we call it the emerald device and you see blue line this line is the trajectory of movements of this person for about two hours and you can see it goes between the bed the chair and the bathroom etc now this is this is just a dot even that dot and we are beyond that dot but that that on its own is very useful so let me show you what we can do with this so we put this device with patients who have parkinson's for a long period of time and the doctor was interested in understanding their behavior and how the disease impact the behavior so let me explain this graph so this graph is for the same patient on this slide right so every circle in this graph is one day the top number you see 0 at the top this is midnight and at the bottom you see 12 which is noon time so every circle is one day the innermost circle is the first day of the study the outermost circle is the last day of the study so the colors refer to different activities and locations so the blue for example refers to when this patient is asleep in bed and as you can see if you look at the blue color in the inner circle you see that it is very fragmented so the sleep was terrible at the beginning of the study but as the study continued again this is two months of data you can see that the blue i don't know whether this works but you can see the blue there is consolidated so his sleep has improved drastically throughout the study you can see also that the whole graph is full of green color so green is with refers to when he sits on this chair like you see this chair here like where the the blue is so he really like to sit on that chair and what you can see here is that this is a very sedentary person he wakes up sits on a chair that's almost all that he does in his life this is very worrisome of course because once you have an elderly person and they are very sedentary it escalates their problems another thing that is really interesting is to like there is a yellow cone around 8 a.m in the morning so you see between 6 and 9 there is this yellow cone here yellow the first two when he goes to the bathroom to do his toileting and shower so this is the person you see the blue stops around 4 a.m in the morning he he wakes up around 4 a.m sits on a chair and waits exactly until 8 a.m to go to the bathroom to do her his shower and toileting can you guys guess why what does that mean i can't hear properly most likely because of the mask but i'm guessing some people probably have guessed it this is someone who actually cannot shower and toilet on his own he's waiting for someone to come to help him and if you remember i told you that he lives in an assisted living community so actually the worker comes around 8 am so the person wakes up and he's he's not healthy enough just to do simple things that we take for granted like showering and he's waiting for someone else to come and help him and another thing that you can see there is a corn of white before 18 18 is 6 p.m so between 17 and 18 between 5 and 6 p.m
he actually is outside the room white refers to being outside the coverage area of our wireless device so he's outside his unit and he likes every night to go and have dinner with the rest of the assisted living community and he tries to do this every every day as you can see except actually there was one day you can see like here here actually he came back early he wasn't feeling well and again imagine like all of this information about his health and behavior without even asking him to wear a sensor or to do anything just by putting something that is that looks like a wi-fi router but just slightly smarter than that now one of the other thing that is really interesting is that clinically meaningful okay so these are behavioral symptoms but actually we we use a the clinical gold standard which is something called updrs in parkinson for you guys all that you need to know is that on this x-axis is the gold standard used for assessing parkinson on the y-axis is the unscripted gate speed that we measure and as you can see they are highly correlated there is no need to go to the clinic to do those measurements you are getting very highly correlated from measure from the patient's home i am going to very quickly tell you something about our investigation of covet 19 so uh included as you know this is a respiratory uh disease and we were asked to monitor people in assisted living communities because i mean as you remember in retirement home there was a lot of many cases and it was pretty dangerous so we uh we monitored those patients and our interest was to discover whether the recovery was smooth or they are having difficulty because they are quarantined when you have coven the person is quarantined and people these are old people and the nurses were very worried that the person may have escalation in their symptoms and there is no way to detect it so this is the breathing of a patient and as you can see the breathing on uh breathing on april 7 and you see her breathing on april 11. and as you can see the breeze in four days later like from april 7 to april 11 is much lower lower rate so her breathing rate went down from 23 breaths per minute to 18 breasts per minute which is significant and this is nice i mean basically this is what happens when you have smooth recovery the breathing is going back to its baseline similarly when you have smoother recovery the person is moving much faster as you can see here so the green dot here is the patient and both cases you see april 8 and april 11 and you see how much faster the patient is moving on april 11. so that means they have less fatigue they are more agile and so on the recovery is good it's not always good sometimes we see like these breathing this is actually the breathing of person you remember like before like i showed you the breathing the nice sinusoid of the healthy person this is actually the policing breathing of one of the corvette patients is very irregular and has like problems both in amplitude and in periodicity of the breath breathing actually is very important of course covate is a respiratory uh infection at the end of the day so one of the things that we discovered is that if you can monitor breathing you can tell a lot about the recovery and whether it's smooth or not so let me explain this graph which each one of these blue curves is a histogram of the breathing rate on one day of the patient so the first one is on april 8 and as you can see the breezing was first was improving the breezing rate was decreasing moving away from 20. then on one day the breathing rate jumped all the way and indeed the patient ended up in the hospital the patient spent about one week in the hospital and then came back and again you can see the breathing rate is decreasing and eventually the patient has recovered so one of the things that you know when when you have the ability to measure breathing like this it was covered is that you hear all the time about these three types of patients patients whose recovery is not smooth and they actually end up in the hospital like the one that i showed you patients who actually had severe symptoms but eventually recover and the breezy weight keeps improving until they recover and asymptomatic patients whose breathing was always good i mean if you look at their vital signs everything is fine they are completely asymptomatic with this i will stop here and take questions actually one one thing before taking questions that i want to emphasize of course we have a technology that is very um it's powerful it's getting information about health and getting and get to see a skeleton of a person's wall so one of the things that are very very important to us is privacy and security so because also particularly we work in the healthcare domain so everything is has to go according to irb approval there is a protocol there is an informed consent and also there are all of the measure about anonymization separation of data from any phi in fact we don't keep in our system any phrase and we pay a lot of attention to issues related to privacy and security i will stop here and if there is any time for question there are seven minutes for questions so i take questions thank you yeah oh there are questions here okay what happened if there were other disturbances in the room such as a cat entering the or enter the bathroom okay and there is a smiley face after that so uh i showed you that uh for example when there were three skeletons moving so we can track different people and different entities of course a cat will like we didn't train with caster will look i think like a person on the floor uh or maybe like crawling on the floor on the floor but basically we can detect different entities and we can separate them based on how they look and their behavior classify them too in fact in our medical study we classify the person of interest and versus everyone else that we are not interested in tracking okay another question over what range can this wireless signal identify people so uh basically we uh it's kind of like it's easy to think about it as slightly lower range than your wi-fi so i'm sure all of you know that there are certain regions in your home that maybe the wi-fi doesn't reach and definitely if you have two floors and the second floor is you need to put either a repeater or another router so it is the same concept so typically we can go up to 14 feet from where the device is if you are measuring motion if you are measuring pulsing of the blood then it is less it is a few meters and but you can put repeaters to um similarly to how you put repeaters for a wireless router for example uh other question if there is a range limitations is it is there a revenge limitation to the technology today so i answered that of course like any wireless signal it has certain range limitation and then you can expand it with repeaters how does this work through how does this work through mediums like concrete and or metal walls like in an industrial warehouse so again the type of wall is like the thicker the wall and uh the more dense it is the more it's going to attenuate the wireless signal so this is not a technological limitation it's really more a limitation of what does the fcc allow for a particular application so you can always increase the power of the signal if the fcc allows you to go for that power level for that application in that particular location seems very similar to xander cardian i don't know some like something millimeter wave radar so uh i mean i don't know the detail of this we don't choose millimeter wave millimeter wave do not traverse walls that will uh this particular uh like i don't know this particular uh web page that you are mentioning but i don't think that this actually that they have been uh proven to like give any health related information i think radar like people have in um in dogpa and military situation try to track people's radar and the best that they can do is a dot on the floor like the first thing that i showed you and even our dot is much more higher accuracy than as far as i know from our publication at the time it was higher accuracy than all the literature which frequency do you use to detect the body so we actually use frequencies that have the same carrier as wi-fi so it is around the same as six gigahertz five six gigahertz uh but our signal is much lower power and it's wider band an obvious application would be for live monitoring in assisted living facilities do you have any research data experience in this environment yeah of course i mean i showed you many of these like many of the data that i showed you comes from assisted living communities we work with some of the assisted living communities here around the boston area and we have helped them during their covered uh the time of covet and the quarantine period okay so i don't see um more questions so if the audience have something then i can answer that as a last question so so this is very interesting i don't know why you are interested in detecting lies i can tell you as a professor like when the student tells you that they didn't cheat on their take-home exam you really want to know whether that's true or not but um so in principle yes although we did not go all the way to like test it in that environment but basically light detection relies on all of these like things that you do like basically your breathing your heartbeat changes uh basically your fidgeting all that stuff that people tend to do when they are not saying the truth so in principle i would say since it detects these kind of features and you can train to to detect lies or like score how likely somebody is saying the truth okay uh do we have more time there is one question okay can you id the person can you id the person so we did our recent work on identification uh so we and we had many more people than what you described but i'm going to point you toward one of our recent publications about identification yes and i'll take the last question the person in the back so that would be a very interesting partnership as you could guess which is basically i would guess like one one application of this is like augmenting existing like um cellular hotspots or applications so that we can have like um a smart environment smart home smart uh analytics and uh we we don't we're currently we are we haven't partnered with any of these um providers but that would be an interesting thing to consider for the future thank you very much
2022-08-02 20:50