12 Days of OpenAI, NeurIPS, ARC Prize, and Llama 3.3 70B
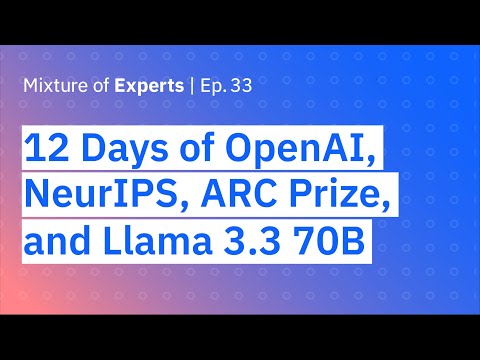
Will you be paying 200 a month for o1 Pro? Marina Danilevsky is a Senior Research Scientist. Marina, welcome to the show. Uh, will you? No, I will not. Vyoma Gajjar is an AI Technical Solutions Architect.
Uh, Vyoma, welcome back. Uh, are you subscribing? Yes, shockingly. And last but not least is Kate Soule, Director of Technical Product Management on the Granite team. Kate, welcome back. Will you be subscribing? Absolutely not. Okay, all that and more on today's Mixture of Experts.
I'm Tim Huang, and welcome to Mixture of Experts. Each week, MoE is dedicated to bringing the top quality banter you need to make sense of the ever evolving landscape of artificial intelligence. Today, in addition to having the best panel, don't tell the other panelists, Kate, Vyoma, Marina, very excited to have you on the show. We're going to talk about the latest hot trends coming out of NeurIPS, designing evaluations for AGI, and the release of LLAMA 3.3 70B. But first, we have to talk about what Sam Altman's been cooking at OpenAI.
If you've been catching the news, OpenAI released, uh, uh, an announcement that for the next 12 days, they'll be making a product release announcement every day, um, to kind of celebrate the end of the year. And there's already been a number of interesting announcements, not least of which is the release of a new 200 a month tier for o1 Pro, which is their kind of creme de la creme of models that they are making available. Um, suffice to say 200 a month is a lot of money, much more than companies who have been providing these services have charged before. And so I really wanted to kind of just start there because I think it's such an intriguing thing. Um, Vyoma, I think you were the standout, you said that you would subscribe.
So I want to hear that argument and then we'll get to Kate and Marina's unwarranted skepticism. Sure. I feel OpenAI's strategy here is to increase more adoption, and that is something that they have been speaking continuously about. Sam has been speaking continuously about in a multiple, uh, conferences and talks that he's been giving. He said that he wants to reach almost like 1 billion users by 2025. And the whole aim behind using and coming up with the 01 Pro with like $200 is to...
try to get like AI developers, who is the majority of the market trying to build these applications, to start using it. Some of the key features that he says are like reduced latency during like peak hours. It gives you like higher speed to implement some of these models and use cases as well. And it'll be surprising that I was reading about it on X and ChatGPT, et cetera, takes like almost 30 times more money, it's more expensive to run. Um, so if you look at it from a perspective as a daily software engineer, developer, engineer, web developer, it, it, it seems to be a steal for those people.
And yeah, that's, that's why I feel that I would pay it. That's great. Yeah.
All right. Maybe Kate, I'll turn to you because I think your response was no hesitation, absolutely not. Um, what's the argument, I guess, for, for not wanting to pay? Because I mean, it sounds like they're, they're like, here, get access to one of the most powerful artificial intelligences in the world. And, you know, it's money, but, you know, I guess what they're trying to encourage is for us to think about this as if it were a luxury product. I think my biggest, uh, umbrage at the price tag is, you know, I can see use cases for a one and having a powerful model in your arsenal and your disposal, but I don't want to run that model for every single task and there's still a lot out there.
So trying to then have unlimited access for a really high cost on a monthly basis just doesn't quite make sense for the usage patterns that I use these models for and that I, that I see out in the world, like, I want to be able to hit that model when I need to on the outlying cases where I really need that power. The rest of the time, I don't want to pay for that cost. Why would I carry that with this really high price tag month to month? Yeah, I was gonna say, I mean, I think one of the funny things about this is the prospect of paying $200 a month and then being like, I need help writing my email. Yeah.
Like, it's kind of like a very silly sort of thing to think about. Um, I guess I have to ask you this cause you work on Granite. Open source, right? I assume one of the arguments is just that open source is better. Getting better and is free.
I don't know if you would say that that actually like is one reason why you're more skeptical here. I mean, I think that's certainly a reason how long you know do I want to pay to have that early access or am I willing to wait a couple of months and see what new open source models come out that start to, you know, tear away at the performance margins that o1's been able to gain. I don't have a need to have that today, and I'm willing to wait and to continue working on the open source side of things as the field continues to play catch up. You know, I think with every release we're seeing of proprietary models, it takes less and less time for an open source model to be released that can start to match and be equitable in that capability. Yeah, it feels like they've really kind of gotten out on a little bit of a limb here, right? I didn't even think about it until you mentioned it, is like, once you've gotten all these people paying $200 a month, it will feel really bad to now say, hey, these capabilities are now available for 50 bucks a month all of a sudden. I think there's some, you know, market testing, right? They need to see how far they can push this.
That's, that's a reasonable thing for businesses to do, but I, it's past my, uh, my, my, my my taste. It's a little too fine. Yeah, for sure. Um, I guess Marina, maybe I'll toss it to you as kind of the last person. I know you were also a skeptic being like, no, I don't, I don't really think so. Um, maybe one twist to the question I'll ask you is, uh, you know, when I was working on chatbots back in the day, we often thought like, oh, what we're doing is we're competing with like Netflix.
So we can't really charge on more on a monthly basis than someone would pay for a Netflix because it's like entertainment, basically. Um, and I guess, I don't know, maybe the question is someone who's kind of skeptical of $200, how much would you pay, right? Like, is an AI model worth $100 a month or $50 a month? I guess, how do you think a little bit about that? I think that's about what a lot of them are charging, right? OpenAI's got a lovely $20 a month uh, tier, so does Anthropic, uh, Midjourney has something like that. So honestly, I think the market has said that if you're going to be doing something consistent, that's a kind of a reasonable amount of money, somewhere in that 20 to 50. The 200 seems like a bit of a play from Steve Jobs of do you really, really want to be an early adopter? Okay, you get to say, ha ha, I'm playing with the real model. Realistically though, I agree with Kate. I think most people don't know how to create sophisticated enough use cases to warrant the use of that much of a nuclear bomb, and you don't even know why you're spending the money that you are.
So you can actually get pretty far in figuring out how to make use of all of these models that are coming out and coming out quickly in the lower tier. I mean, if again, if I was in charge of the finances, I'd say give me a reason why this is a 10x quality increase. And I don't see why it's a 10x quality increase when you don't have a 10x better understanding of how to actually make use of it. Um, so I'm, I'm on Kate's side.
I think part of this, and I think the comparison to Apple is quite apt in some ways is, um, you know, like Apple has turned out not necessarily be the most popular phone, but the most profitable the phone, right? And it actually just turns out that a lot of people do really want to pay premium. I guess maybe what we're learning is like, does that actually also apply for AI, because I think, you know, it's hard to imagine other things that you pay $200 a month for. It's like getting like to like commuting expenses, utilities, like you pay that much for your internet bill, I guess, you know, in some cases.
So yeah, I think we're about to find out whether or not like people are going to bid up in that particular way. I guess Vyoma maybe I'll turn it back to you, I mean, with all this criticism, still sticking with it, though. Yeah, I'm telling you, I feel the market niche that the OpenAI wants to stick to is getting people to utilize these APIs, um, for purposes in the case that they want to build a small application, like, uh, they have a black box environment as well, where they can build, uh, something on their own, get it out quick and dirty. Experimentation is much more easier. And let's be honest, OpenAI has the first mover advantage.
So everyone, like majority of the people, know ChatGPT as the go-to thing for generative AI. So they are leeching it and I completely see them, um, doing some of the, these, uh, marketing strategies around the money, et cetera. I feel they are monetizing on it now and, That's one of the key reasons they might be getting some push from investors. I don't know, but that's somehow I feel the strategy that startups do follow.
And that's what everything is doing to as well. Yeah, for sure. The other announcement I kind of quickly wanted to touch on was, uh, OpenAI had been hyping, uh, Sora, which is their kind of video generation, um, model. Um, and it's now finally kind of widely available. Um, and I think this is a little bit interesting just because you know, this is almost like a very different kind of service that they're supporting, right? Like they came up with language models.
Now they kind of want to go multimodal. They want to get into video, you know, in part to kind of compete with all the people that are doing generative AI on the image and video side. And I guess I'm curious if the panel has any, any thoughts on this. Um, Kate, maybe I'll throw it to you is like, it kind of feels like this is like a pretty new front for OpenAI to try to go compete in from a technological standpoint, right? Like I think like, this is like a pretty different set of tools and teams and infrastructure. I guess kind of like, do you think ultimately this is sort of like a smart move on the part of OpenAI? Because it does feel like they're kind of like stretching themselves kind of in every direction to try to compete on every single front in the generative AI market. I mean, I think it does make sense under the broader vision, or OpenAI's broader vision of pursuing AGI.
I mean, I think you're going to need to be able to have better, uh, video understanding and generation capabilities to kind of handle this more multimodal task. And we're starting to see models being able, one single model being able to handle multiple different modalities and capabilities. So you need to develop models that can handle that right before you can start to merge it all together. So I think under that broader pursuit and umbrella, it does make sense to try and develop those technologies and techniques. Yeah, I think it's kind of like, well, we'll have to see. I mean, I think again, like part of the goal is just like whether or not AGI itself is is the right bid, um, to kind of take on this market, um, and, and whether or not this market really will be kind of like one company to rule them all, if it will be like, you know, he's the winner on video, and you have the winner on text, and it'll kind of break down in a multimodal way.
I mean, I'm really skeptical that there's like the right economic incentive to develop AGI in the way that a lot of people are pursuing it. So we'll, we'll see, you know, but if that's your broader vision, I don't think you can have a language-only model for AGI. Right? It needs to have better, different domain understanding. Um, how about this announcement? I mean, Vyoma, Marina, are you more excited about this than having the prospect of having to pay, you know, your, your internet's bills worth each month for a language model? Yeah. Uh, I feel like the Sora announcement that we saw, and I was going through the videos and I was actually playing through it. The way that they've created, if you look at the UI, it looks very, very similar to your iCloud photos UI.
Again, they're trying to drive more and more people to, um, use it seamlessly and also, uh, it, it creates an, um, era of creativity, like people are going over there playing a little bit with their prompts, increases the nuances around prompt engineering as well. I saw a lot of that, uh, happening with different, uh, AI developers that I work with day in and day out. They're like, if I tweak this in a different manner, uh, will that particular frame in which it is being developed change, et cetera.
So it's, I feel it's also coming up with a whole different, um, arena of doing much more better prompt engineering, prompt tuning as well. I'll second that in saying that it's a really good way again to get a better understanding of what this space really is and a lot of data. This is something that we don't have an entire text's worth of internet or internet's worth of text stuff for, whereas here trying to see whether anecdotally or if people are willing to share what they've done, people will get a much better sense of what can these models do and then maybe economic things will come where you have a true multimodal model that can understand, you know, graphs and charts and pictures and videos at the same time. Um, but this is a good way to get a lot of data of what comes to people's minds and what they think the technology ought to be useful for. And that is interesting and it'll be really interesting to see what comes out from, from this capability.
Yeah, I think kind of the model discovery, like you kind of build the model, but it's sort of interesting that the people who design it are not necessarily well positioned to know what it will be used for effectively. That's absolutely true. yeah, and the market's just like, all right, well, let's just like throw it out there. And then they're kind of just sort of like waiting, hoping that something will pop out. That's a great point that Marina brought about that, and I know Kate also spoke on the same point about AGI. Imagine, like, I just thought about it.
All the users are writing their prompt creativity onto that particular, uh, Sora interface. That is data itself. Imagine that data being utilized to gauge human creativity and getting much more closer to AGI, so. And building on that, then also that model that you've trained can now generate more synthetic data that you can then, even if you don't want an AGI model to be able to generate videos, you still need an AGI model that can understand videos. And for that, you need more training data through either collecting data that's been generated by, you know, prompts, creating synthetic data from the model itself, Sora, to create some larger model.
So it all, all I think is certainly related. Yeah, for sure. And yeah, there's kind of a cool point there about, I think, like, If we think synthetic data is going to be one big component to the future, um, there's almost like a first mover advantage, right? Well, yeah, okay, maybe it's uncontroversial, right? But it's kind of just like, well, you actually, if you're the first mover, you can acquire the data that helps you make the synthetic data.
And so there's kind of this interesting dynamic of like who gets there first actually ends up having a big impact on your ability. And this is OpenAI's playbook, like one of the reasons they were able to scale so quickly is they had first mover advantage and their terms and conditions allow them to use every single prompt that was originally put into the model when it first released. It wasn't a little bit later until they started to have more terms to protect the user's privacy with those prompts. So yeah, definitely a model they can rinse and repeat here, so to speak. And now everyone else is caught on and is like, Oh, any model you put out where we can't store the data or don't you dare store my data.
So OpenAI got in there before people caught up with critical thinking of, oh, that's what you're doing. Yes. Yeah.
I'm going to move us on. So this week is the Lollapalooza, maybe that's too old of a reference. The Coachella of machine learning is happening. This week, uh, NeurIPS, the annual machine learning conference, uh, one of the big ones next to, you know, ICML and ICLR, um, and, uh, there's a ton of papers being presented, a ton of awards going on, a ton of industry action happening at this conference, certainly more than we're going to have time to cover today. But I do think I did want to take a little bit of time just because I think it is a big research event and we have a number of folks who are in the research space, uh, here with us. On the episode.
Um, I guess maybe Kate, I know we were talking about before the episode, maybe I'll kick it to you is, you know, given the many thousands, thousands of papers circulating around coming out of NeurIPS. Um, I'm curious if there's things that have caught your eye, things you're like, oh, that's what I'm reading. That's what I'm excited by.
Um, what are pointers? Because I think for me personally, it's just like overwhelming. Like you look on Twitter, it's like, this is the big paper that's going to change everything. And then pretty soon you have like more papers than you're ever going to read. So maybe I'll tee you up as I'm curious if there's like particular things you point people to take a look at I mean, I think there's some really exciting work that our colleagues at IBM are presenting right now that I'm just really, really fascinated by and think has a lot of potential.
So I definitely encourage people to check out the paper called Waggle, which is a paper on on learning that are our own panel expert Nathalie, uh, is representing talking about unlearning in large language models and they've got a new method there. Uh, there's also a paper called Trans-LoRA that was produced by some of my colleagues who sit right in, uh, Cambridge, Mass. And I'm really excited by this one because it's all about how do you take a LoRA adapter that's been fine tuned for a very specific model and represents a bunch of different capabilities and skills that you've added to this model and you've trained it. And transfer it to a new model that it wasn't originally trained for, because normally LoRA adapters are pairwise kind of designed for an exact model during their training process.
And so I think that's going to be super critical as we start to look at how do we make generative AI and building on top of generative AI more modular? How do we keep pace with like these, breakneck releases, you know, every month. It seems like we're getting new Llama models like Granite we're continuing to release a bunch of updates similarly, And I think that's just where the field is headed. And if we have to fine tune something from scratch or retrain LoRA from scratch every single time a new model is released It's just going to be unsustainable um, if we want to be able to keep pace.
So having more universal type of LoRA's that can better adapt to these new models Um all I think is going to be a really important Uh, part moving forward to the broader ecosystem. That's great. So, yeah, we definitely, uh, listeners, you should check those out. Um, Vyoma, Marina, I'm curious if there's other kind of things that caught your eye, papers that you're of interest or, or otherwise. So, one of the papers that I was looking into was the understanding the bias in large scale visual data sets.
So, we've been working a lot with large language models, uh, and, uh, uh, data, which is, uh, language data. But here, this was based on of some data set or an experiment, which was done in 2011, which was called name that data set and what I, what they showcased in this entire paper is how you can break down the image by doing certain transformations, such as like semantic segmentation, object detection, finding that boundary and edge detection, and then kind of doing some sort of color and frequency transformation on a piece of particular image to break it down such that you are able to, uh, ingest that data in such a better manner that a model that is being created on that data is much more accurate and precise. So very, very, um, old techniques I might say, but like the order in which they performed it was. Great in a visual use case. I think that was one of the papers that really got my eye.
I think that's interesting to me lately is the increase in structured now not just data but the structured execution of language models for various tasks as we continue to get more and more multimodal not even just text, you know, text, image, video, but just text, uh, with functions, with tool calling, with things of that nature. I think we talked about this on a previous episode as well. There's now some interesting work going forward. Uh, one particular paper I think I read recently, uh, SGLang. on how to actually execute the language model in what your state is, and how to have it be forking and going in different directions.
I think that there's a lot to be said here about how to make these models work for you in a way that's not just sequential, and not just, oh, chain of thought, first do this, then do this, then do this. No, let's turn it into a proper programming language and a proper structure with a definition with some intrinsic capabilities that the model has besides just text generation. So that happens to be a particular topic that I'm looking at with interest. Yeah, and IBM actually has a demo, I think, on that topic.
Yes, it does. So how do we use SG Lang and some LoRA adapters, uh, coming back into play, uh, different LoRA's in order to set up models that can run different things like uncertainty quantification, safety checks, all within one workflow. Using some clever masking to make sure you're not running inferences multiple times and to kind of set up this really nice programmatic flow for more advanced executions, uh, with the, with the model in question. So if anyone's at NeurIPS, definitely recommend checking out the booth. That's great. Yeah.
I feel like, uh, I don't know, my main hope right now is like to have more time to read papers. I do miss that period of my life when I was able to do that. Um, I guess maybe the final question, I mean, Marina, maybe I'll kick it to you is, uh, how do you keep up with all the papers in the space, just as a meta question? Uh, I think I can't possibly, but, uh, in general, giant shout out to my IBM colleagues. We have some real good active Slack channels where people post the things that they like, and there's particular folks with particular areas of expertise that I can look to and see, oh, what has, uh, some particular researcher been posting. Lately.
And that is the way because, um, yeah, it's, it's a lot of things, especially now that there's, uh, a very welcome shift to people posting research even early, just, you know, preprints on archive and things of that nature. And you really need the human curation to let you know what's noise and what's worth paying attention to. And yeah, I can't beat human curation for that right now.
. Yeah. It feels like, I feel like the, the, the key infrastructure is group chats. Like that's all I have now. Yes. just gonna add that- this is gonna make Kate very happy on this.
I use, uh, the, as a true AI developer, I go to watsonx, the AI platform. I use the Granite model. I feed in my papers one by one. First I ask, okay, summarize this for me. Then I'm like, tell me the key points.
And then I go deeper, deeper, deeper. I mean, I go the other way around to reverse engineer the paper to kind of figure out what to do with it. There's a script that I've written for it, which I'm very proud of. So I usually- You should open source that. Yeah, you gotta open source that.
I need that in my life. Maybe I could do that. Yes, you should.
Absolutely. Okay. Thank you. You heard it here first on Mixture of Experts.
I'm going to move us to our third topic of the day. Um, so, uh, ARC Prize, uh, which is an effort that was set up by Mike Knoop of Zapier and Francois Chalet of, uh, Keras, um, is a benchmark that attempts to evaluate whether or not models can learn new skills. And ostensibly what it's trying to do is to be a benchmark for AGI. In practice, what it means is that you're asking the machine to solve a puzzle with these colored squares.
Um, and this is very interesting. I bring it up today just because I think they did the latest round of kind of competition against the benchmark and showed the results and a technical report came out. But I think this effort is just so intriguing because you know, we've done it to this on the show where people say, AGI, what does it really even mean? And I think in most cases, people have no idea what it really means or can't really point to how they would measure it.
And this seems to me to be like at least one of the efforts that say, well, here's maybe one way we could go about measuring this. Um, and so I did want to kind of just like bring this up to kind of maybe square the circle, particularly with this group, um, about sort of evals for AGI. Like, does that even make sense as a category? Are people even looking for those types of evals? There's just a bunch of really interesting questions there.
And I guess Vyoma, maybe I'll turn it to you first. I'm kind of curious about like, when you see this kind of eval, you know, is it helpful eval? Is it mostly a research curiosity? Like how do you think about something like ARC Prize? Yeah, so when I look at ARC Prize, it was, I think, um, it was founded in 2019, created back then, when generative AI, large language models weren't a thing back then, and I think, um, it helps because it's like one of the first in the game again as well, so people kind of relate immediately back to it that this is the benchmark to, um, evaluate AGI, but AGI is way more bigger and better, um, in doing things such as there are so many things that, uh, clients like OpenAI and then other companies such as Mistral, etc., they're coming up with these models, which can annotate human data to help you act like human. And there are different methods to do that. So are AGI even, I won't say is the pristine benchmark or standard, but I do get the point as to why people refer back to it a lot. Yeah, it sounds right.
I mean, I think that's kind of, I mean, we talked about it earlier in this episode. I think Kate, you were like, it makes sense as if you were an AGI company, this is the strategy you would pursue. As yeah, it's kind of interesting, even though we can think about it and talk about it in those concrete terms, when it comes down to like the nitty gritty of machine learning, it's like, what do we even use to kind of measure progress against this? And no one really knows, I guess, you know, kind of you're indicating that it's like, well, we kind of fall back to this metric because we don't have anything else. Um, yeah, I kind of curious, Kate, how you think about that? I think there are, there's a number of different ways you can think about it.
One, we're always going to need new benchmarks to continue to have some targets we can solve for. So having a benchmark that hasn't been like, cracked, so to speak, is interesting. I don't know that that means it's more than a research curiosity, honestly, but there is something of value there.
There's something that we're measuring that models can't do today. Is that thing valuable? I don't know. The, we're really talking about solving puzzles with colored dots. How well can people who solve those puzzles with colored dots correlate to the different tasks outside of solving those puzzles? I'm not too sure.
I also think there's something wrong calling that a test for AGI because like, general is in the name. That task in that benchmark is very specific. It's like oddly specific. And it's one that humans can't do very well today either. So, you know, it doesn't quite resonate to me as a quote general intelligence where I think breadth is super important, um, if that's what we're really after.
Yeah, it almost feels like you need like the the pentathlon or something. It's got to be like a bunch of different tasks, I guess, in theory. Um, I guess Marina, do you, do you think, I was talking with a friend recently, I was like, I was like, do you think evals are just broken right now? Like, um, there's kind of a received wisdom that most of the market benchmarks or the understood benchmarks are all super saturated.
Um, and then like, it's very clear that the vibes evals, like you play around with it or like not. comprehensive in the way we want. And then so there's kind of this big blurry thing about like, well, what's the next generation evals that we think are going to be useful here? And how broken is it? Maybe I'm being too much of a pessimist.
It's a very hard thing to do. I mean, even this particular benchmark that we're talking about, it is one specific way to instantiate a few assumptions about intelligence that they said. I was refreshing my memory on what they had said.
And I was like, okay, there are objects and objects have goals. And there's something about the topology of space. Okay.
Yes, this is all. True, and this is one way to go there. It's certainly not a comprehensive way, but with research It's all about well, we got to have some instantiation of it or we're never gonna make any progress So I think you always have to take every benchmark with a grain of salt. A benchmark is not an actual measure of quality It's a proxy if you want to really get into ML speak quality is hidden, benchmark is observed.
And it is a limited proxy in a smaller space than what the quality is. Think about all the hidden layers of quality. We get a specific proxy. Um, the more variety you can do the better and the more you can also, uh, understand that if something's been around for several months, as you said, it's been learned.
Um, you, that's it, you, you've learned it, you need to move on and, and do something else. But the problem is, if we don't have something that's quantitative, then people are just going to argue over vibes. Like, "well, I had these five examples in my head," "well, I had these five examples in my head."
And then you really do just say, I don't, I don't trust it, or I don't believe it, or, but can't these things be faked? That way lies madness, as far as the actual use of these things go. We have to agree on something and put out the limitations and put out the constraints and still be able to agree that there is something to compare on. Um, so I, there's, there's no way around it with evaluation. It's never been easy. It's never going to be easy.
I don't think it's more broken than it ever really used to be. Yeah, exactly. It's exactly as broken as it always has been. It's as broken as it's been. Yeah.
I think, cause I think you're seeing two meta trends. I feel like one of them is, We talked about the hard math benchmark that this group called Epoch put out. Um, and you know, it feels like one, one bit of meta is like, we're going to just make the difficulty so difficult that like, it's almost like a way of us recreating that consensus where we're kind of like, Oh, well, if a machine can do that, something is really happening. But it feels to me, that's like a very, almost a very crude way of going at eval is like, all we do to try to get some agreement to move beyond the vibes is to try to create something that's so difficult that it's indisputable that if you hit it, it would, it would be like a breakthrough in progress. But, you know, on a day-to-day basis, it's like, how useful is a metric like that? You know? Well, so ultimately, uh, ARC Prize, I guess, are we pretty sympathetic to it? It kind of sounds like ultimately, it's like, it's measuring something.
We're just not quite sure what it is just yet. I don't know if I'm a million dollars sympathetic to it. I'm sympathetic to it as a benchmark, but I guess it's up to them.
Yeah. I like how large dollar amounts have just been this theme for the episode. I feel, I feel once the AI agents, um, are utilized to kind of, uh, make these AGI concepts much more simplified, I feel that I wouldn't go to that extent saying that that particular benchmark can be achieved and someone will win that prize. But I feel that with multiple permutation transformations with AI agents, let's say someone used generalization and some sort of transfer learning and then created an agent to understand the human's way to learn, maybe, maybe not, but I feel that that's a gray area right now and we don't know what can be achieved. So let's say I'm not here to say that it's here to stay or not, but there's something new comes along. I feel that's something that we're measuring against.
I think I mean, and to Marina's point, I think one of the theories I've been sort of chasing after is AI is just being used in so many different ways by so many different people now that like, we will just end up seeing this like vast fragmentation and evals, right? Like it won't be old days where it's like, it was good on MMLU, so I guess it's just good in general. Like everything is going to just be measured by like very local needs and constraints. And, you know, talk, talk about group chats. I've been like encouraging all of my group chats, like we need our own bench, you know, because I think it's just like every community is so specific that like we just should have our own bespoke eval that we just run against models as they come out. So for our next topic, I really want to focus on the release of Llama 3.3 70B. Uh, background here is that Meta announced that it was launching, uh, another generation of its own Llama models, um, and most notably a sort of 70B version of the model that promised 405B performance, but in a much more compact format.
This is a trend that we've been seeing for a while. And I guess maybe ultimately, um, you know, Kate, maybe I'll kick it to you is, I guess the question I want to ask is like, do we think that we're going to eventually just be able to have our cake and eat it too? Like that, like we've been operating under this trade off of big model, hard to run, but good. Little model, not so good, but fast to run. And, you know, where everything seems to be going in my mind is like, maybe that's just a total historical artifacts? Like, I don't know, do you think that's the case? I think that we often conflate size as the only driver of performance in a model. And I think with this release of Llama 3.3 70B, comparing it to the older 3.1
405B, we're seeing firsthand that size isn't the only way to drive performance, and that increasingly the quality of the data used in the alignment step of the model training is going to be incredibly important for driving performance, particularly in key areas. So if you look at the eval results, right, the 3.3 70B, uh, is matching or you're actually exceeding on some benchmarks the older 405B in places like math reasoning.
And so I think that really speaks to the fact that you don't need a big model to do every task. Smaller models can be just as good for different areas. And if we increasingly invest in the data versus just letting it sit on a compute for longer, training it at a bigger size, we can find new ways to unlock performance. Yeah, that's a really interesting outcome. My friend was commenting that it's like, uh, it's almost kind of like a very heartening message.
You know, the kind of ideas like you don't need to be born with a big brain so long as you've got good, good training. Like you've got like a good pedagogy is like actually what makes the difference. And, you know, I think we are kind of seeing that in some ways, right? That like, I guess like the dream of massive, massive architectures may not be like the ultimate lever that kind of gets us to really increase performance. Um, uh, I guess, I think one idea I think I want to kind of run by you is just whether or not you think that this, this will be the trend, right? Like I guess to Kate's point, like you can imagine in the future that companies end up spending just a lot more time on their data more than anything else.
Um, which is a little bit of a flip. I mean, I think most of my experience with machine learning people was like, I don't really know where the data comes from. So long as there's a lot of it, it will work. Um, and this is like almost points towards a pretty other different discriminating kind of like approach to doing this work. Yeah.
So I, I work with clients day in and day out, and I feel that the trend is catching on. The clients no longer want to be paying so much money amount of dollars for every API call to like a large model and on, on something which is lying, not in their control. Even though we, they say that, oh, we say we indemnify the data, which you are not, we are not storing your data for them in their head. It's still not there yet. So people want it on their own prem, a smaller model trained on their own specific data. There have been so many times that I've sat with them and then curated the data flow.
That listen, this is what we'll get in. This is how we'll get it. So the trend is definitely, definitely catching on.
And sometimes often, like historically, I've seen that efficiency gains that we see, they are promising, but sometimes some of these models, they, uh, there, there are some trade offs in like context handling and then adaptability, et cetera. So now I feel if we have a smaller model with good amount of data, that the domain-specific data, they are getting better value out of it. And I see that happening.
So yeah, I feel it's good and refreshing to see that no longer everyone, every time I used to walk into a board meeting and everyone would like, Ooh, 70 billion, Oh, 13 billion, I will be comparing it under 405 billion. I no longer have to have that conversation anymore. So good for us. Yeah, I think it's kind of like, it's almost like people want the metric.
They're like, oh, that's a lot of B. Like, where is this 405 B? That's a lot. Because now they have the legal team, the finance team, as Marina was mentioning, breathing down their necks. They're like, why do you have such a big model? Why is it inflating our resources and the money that we have to write a check on every month. So everything's coming back to that. Yeah.
There's a little bit of a race against time here though. I don't know if Marina's got views on how this will evolve as like, part of this is driven by just the cost of API calls. And so there's kind of almost this game where it's like, how cheap will the API become versus how much work are people willing to do upfront around their data? I guess kind of you almost saying is like, it seems like companies are really tending towards the data direction.
Uh, so as a committed data centric researcher, I'm very pleased to see this direction of, uh, of things. Is it good? Excellent. Um, again, I'll just, uh, re, uh, say what Kate had said, which is that the 3.3 model, uh, versus the 3.1, it's only post-training.
It's not, you know, reach, you know, making a new model. It is differences in post-training techniques. So fine tuning alignment, things of that nature. And this also shows the value of going in the directions of the small different ways of adapting LoRA's because yeah, clients want things that are not just good on the general benchmark. They want things that are good for them. And look, the big was good because whenever you have new technology, first you want to get it to work.
Then you want it to get to work better. Then you want it to work cheaper, faster. So we have like, all right, there's a new thing. Okay. Now we're getting those things a little bit smaller, cheaper, faster.
There's a new thing again. Now we're getting it smaller, cheaper, faster. This is normal.
This is a normal cyclic way of having the innovation. Clients are for sure catching up to this fact and saying, yes, okay, I see your 405, but I'm not gonna pay money on that because I already know you're going to figure out ways to bring that down. And I don't need all the things that that model can do. I need really specific things for me.
So this is again, even goes back to our conversation on benchmarks. You look at the benchmark that matters for you. You look at the size of the model that matters for you and how much it costs. And this, this really matters a ton as we try to make use of this technology, not get the technology to work, but to get the technology to work for us. This trend is going to continue and I see it as a as a very good thing a very heartening thing It means people are getting a better intuition of what the point of this tech is going to be which is not size for the sake of size. I also think there's some really interesting like scaling laws that are starting to emerge like you look at the performance of Llama 1 65B versus you know, okay, maybe Lama 2 13B was able to accomplish all of that.
You look at what Llama 3 8B could do compared to Llama 2 70B. You know, again, we were able to take that, shrink it down. Now we're taking Llama 405B and shrinking it down into 70B. And I think these updates are happening more rapidly and we're increasingly.
Uh, decreasing the amount of time, uh, that it takes to take those, that large model performance and shrink it down into fewer parameters. And so it'd be interesting to plot that out at some point and see, cause I think we're seeing a ramp up and as we continue to look at things that are scalable. So like amount of training data and size of the model isn't very scalable. It just, it's cost exponentially more to increase the size of your model, right? But if we are looking at things like investing in data quality and other resources that maybe are.
Uh, we can invest in more easily. I think we're going to continue to see that increase in model performance and shrinking of the, the model size. And to Vyoma's earlier point about, uh, agents, right, the complexity of that is exponential already itself. So you do not want to be having an each agent have a 405 billion parameters. That is, that is not something you can do.
So it's a yet another driver in this direction of motivator. One more driver that I've seen. And I don't know if anyone else has, but I was in a call with one of the banks and they've also, uh, there's a shift towards using some energy efficient training pipelines as well. Everyone's looking into how do we optimize the hardware utilization? Is there any sort of long term environmental effect? And that's also a nuanced topic, which is building up. I saw some papers in NuerIPS also on that, but I've hadn't had the chance to look deeper into it, but I also see these conversations coming up day in and day out.
Although I guess one thing, I mean, maybe Kate to push back a little bit, like it is actually an important thing probably for our listeners to know that you, you kind of need the 405 to get to this new Llama model. Um, and I guess that is one of the interesting dynamics is for all the benefit that these small models provide, is it right to say like we, we still need the, the mega size model to get to, get to this? Again, I think we're conflating size as the only driver of performance. So I think you need more performant models to get to smaller performant models, regardless of what size they are. Um, and if you have something bigger that's performant, it's easier to shrink it down in size. But if I talk about and think of the normal way we'd think about going doing this right taking a big model and shrinking it down is generating synthetic data from it and what's called using it as a teacher model and training a student model on that data you can use a smaller model if it's better at math. You know Llama 3.3 70B is uh you know outperforming 405B according
to a few other benchmarks on on math and instruction following and code so I could, and would prefer to use that smaller model to train a new 70 billion parameter model than 405B. I don't have to go with the bigger one. I want to go wherever performance is highest. Yeah, this all calls to mind, I mean, I want to benchmark or some kind of machine learning competition, which is like you take the smallest amount of data to try to create the highest level of performance. And like, it's almost like a form of like machine learning golf.
It's like, what's the smallest number of strokes that get you to the goal? You know, what's the smallest amount of data that gets you to the model that can actually achieve the task? And it feels like, you know, it sounds like we may just be forced there because. you know, legal and finance are complaining. Now it feels like it's going to become more of an incentive within the space. You're going to promote overfitting, Tim. If you really do that kind of thing, people will just game the benchmark. Well, that's another topic for another day.
As per usual, we're at the end of the episode and there's a lot more to talk about, so we will have to bring this to a future episode panel with you all on. Uh, thanks for joining us. Uh, and thanks to all you listeners out there.
If you enjoyed what you heard, you can get us on Apple Podcasts, Spotify, and podcast platforms everywhere. And we will see you next week on Mixture of Experts.
2024-12-18 08:58