Next-Generation Sequencing Technologies (2016) - Elaine Mardis
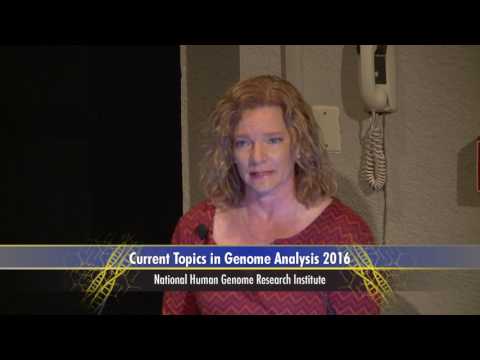
Please join me in welcoming today's, speaker dr., Elaine, Mardis. All. Right thanks so much Andy, I always feel so much better about myself after, I hear your, introductions. It's. Fantastic, to be here and thank you for joining me today to talk about my. First love which is sequencing, technologies. And, so, I think we should just get, going without, hesitation, here, and and jump right in now I do have one conflict, to announce and just to keep in mind throughout the presentation. Is that I am on the supervisory. Board for Kaia gen which, is a company, based over, in Europe, okay. So, let's just jump, right in I think and talk about what, I like to refer to as massively, parallel sequencing, you'll, also hear me slip up sometimes, and talk about next-generation, sequencing. There. The sort of same thing and, I just want to sort of walk you through the. Basics, behind this then, move forward into some newer sequencing, technologies. And finish up with just a little vignette, on how we're using all, of these technologies. The, Associated bioinformatics. Pipelines. And really, beginning to make inroads and changing, the course of outcomes. For cancer patients so I'll. Just leave you with that little teaser and you can hopefully. Enjoy it at the end so. As Andy said beautifully, in. His introduction. You know massively, parallel sequencing and, next-generation sequencing. Have really transformed by a medical inquiry, you. Can see this output. Per instrument, run. Figure. Shown here, from a little. Perspective, that I wrote for nature, in 2011. Cited at the bottom of the slide that really, shows the Magnificent. Jump in the amount of sequence data that, we could generate in. The advent. Of next generation, sequencing, devices, between 2004. And 2006. But. Above and beyond just the sequence output, which has continued, to climb in a radical, way as I'll talk about in just a moment they're really other you know sort of procedural, aspects, of next-generation sequencing. That have freed us from some of the old ways and really. Contribute to this overall. Acceleration. In our ability, to Jenner. Eight sequencing, data so. Just, for purposes, of illustration you, can see sort of sequencing, as I learned it back in the day where, we did a lot of bacterial. Work with sub cloning. Plating. And DNA, preparation. Of individual, sub clones and then importantly we did a separate, set of sequencing. Reactions, on those subclones, followed. By a separate. Electrophoresis. And, detection step, so really, decoupling. The molecular, biology of, sequencing. Away, from the actual. Sequence data generation. So. The contrast, here is remarkable. If you look in panel B which. Sort of illustrates, the stepwise process for, next-generation. Sequencing. Which, starts with just standard. Fragmentation. Of DNA which is done using soundwaves, or. Other, shearing based approaches, we, do some repair. On the ends of the DNA and, adapt. Put. Adapters, on to the ends with a, ligase. Enzyme, attach. These to some kind of a surface, amplify. Those insights, you and then proceed, importantly, to a combined, molecular. Biology, and sequencing. Detection, step so rather than the separate, process, appear in old-style Sanger, sequencing next. Generation or massively, parallel sequencing does, everything together at the same time so. Let me just illustrate the, differences, in, a little bit more detail, between, in. Terms of how massively, parallel DNA, sequencing, works, so. First of all as I already alluded to you have to create a library, to, do sequencing on but actually library generation, is a very rapid, process it. Can be completed, even by a high school senior, with a reasonable, attention, to detail my daughter is a testament, to this back in the day and. Can be done sort of in the period, of time of an afternoon. Or maybe even a day if you're not, in a super big hurry so this. Library approach, is really just using. These custom, linkers, or adapters, as they're called they're. Attached to the ends of the DNA with ligase, enzyme, as I mentioned, and over, time the genesis, of different library kits has led to kids with really, much. More efficient, ligation, procedures. This is important, for low input DNA which we'll talk a little bit about and. This is sort of really now a while, wide, open field for additional.
Commercial. Development, in terms of improving library, kits into. Next-gen, sequencing, platforms. The. Other aspect, which I showed in that mini, figure at the bottom of the last slide is that we do need an amplification, step, for these resulting. Adapter. Ligated, fragments. So why is that well. Unlike, some of the technologies, I'll talk about in a few minutes this is sort of sequencing. Of a population. Of fragments, so we require. Amplification. Of each, one of these library, fragments, so that the downstream, molecular, biology, and detection actually. Works in other words the instruments, that I'll be talking about here for the next few minutes aren't, sufficiently, sensitive to, sequence, from a single, molecule rather. They need the amplification. Of that molecule into multiple, copies in, order to generate sufficient, signal. To be seen by, the imaging. Optics or, other other. Detectors. That are on the sequencing, instrument, so, the, way this amplification, is, accomplished, is by enzymology, first. There's a attachment. To a solid, surface this, can be either a bead which is round of course, spherical. Or a flat, silicon, derived surface, and this just depends on the different technologies. As I'll illustrate in, a few minutes and. The way that this attachment happens, is that the surface of, the bead or the flat glass is actually, covalently. Has. These, complimentary. Adapters, on its surface and so they're available there, for the, hybridization, of, those library, fragments. Followed. By an enzymatic, amplification. And so it's a really straightforward way. To attach, these library. Fragments, onto the surface, and. And get them amplified, up so that you can see them on the. Next step is really this combined, molecular, biology, of sequencing, often, referred to as sequencing, by synthesis. Or SBS. With. The detection, of the nucleotide, base or bases, that have been incorporated in, the, molecular biology. Reaction. And so this. Is a stepwise, process, as, you'll see through from the illustrations. That follow where, you sort of provide, the substrates, for sequencing, let. The sequencing, reaction happen. And then, detect, it as a subsequent. Step in. The process, and. Really. The thing that distinguishes. Massively, parallel sequencing, from, Sanger, sequencing as, I've already alluded.
To Is that, we're not sort of sequencing, 96. Reactions, at a time which was sort of the maximum, per, machine throughput. In, the past for electrophoresis. And, detection approaches. Rather, because, we can sort of use, multiple, beads or decorate. The surface of this flat silicon, glass with hundreds. Of thousands. Of these library, fragments, we can literally generate, the. The. DNA. Sequence, needed, to sequence, an entire organism's. Genome, in a single run of the instrument, so you're, really talking, about massively, parallel sequencing as, being hundreds, of thousands to hundreds of millions of reactions, that are detected, happening, in the stepwise process, on, the, instrument, all at the same time so. The throughput acceleration. As you saw from that graph is extraordinary. Over, what we used to be able to do a Sanger, where we would just buy more and more machines the more sequencing, that we needed to do, and. Then lastly and I'll talk about this, a little bit but I'm keep in mind that because each one of these amplified, fragments. Starts. As an original single, molecule, you're, really getting digital. Read type information, so, what that means is that if we have for example a, portion, of the human genome that's amplified. So multiple, copies beyond, the normal deployed, er there a great, example would be her2, amplification in, specific, subtypes, of breast cancer, you can literally go in and count the fold amplification. Of that locus, in the genome relative. To diploid regions, and really. Understand, with exquisite. Detail, the, number, of amplifications. That are there the number of extra copies, and actually. In whole genome sequencing, the boundaries, so the exact start, and stop regions. On that chromosome where, the amplification, has occurred. So this is incredibly, powerful and, in, going to RNA you can also calculate, very, exact, digital expression, values, for, given genes from RNA sequencing, data which. Starts with RNA converts. The DNA and goes through very similar processes, for the sequencing, as I'll describe for DNA here in just a moment. Lastly. And we'll get to this important, point in just a moment when it comes to talking about bioinformatics. Or, the analysis, of sequencing, data but. Unlike the Sanger, sequencers, of the past one, of the downsides or, one of the confounders. Of, massively. Parallel sequencing is, that the overall length of read the number of bases, that you sequence from any DNA fragment, is actually, quite a bit shorter, than we're used to seeing from conventional, Sanger sequencing. So typically back in the day the Sanger read lengths were on the order of about 800. Base pairs let's just say for the sake of argument most. Kapler or most um massively. Parallel sequences. Will give you round about 100. To 200 maybe, 300 base, pairs so significantly. Less and when we're talking about analyzing. Data from a whole human genome this. Can actually lead to some, significant. Consequences. In the analysis. Of that data, okay. So let's get deep in the weeds a little bit on the molecular, biology, steps and other aspects, of massively, parallel sequencing so. I mentioned the need for constructing. A library, I'm prior to sequencing, as, I talked about already we fragment, the DNA into, smaller pieces starting. From high molecular weight, isolated. Genomic, DNA for example there. Are a variety of different steps. That are listed here that, are enzymatic, steps that are a work up to this adapter. Ligation, that's. Important, for the purposes, of the, subsequent. Amplification. Of fragments, and sequencing. As well I mean so these are really just the stepwise processes. We. Also can, now. Include. In this adapter, a so-called, DNA, barcode, which is a stretch of eight or more. Nucleotides. That have a defined, sequence, to it what, that means is that we can ultimately take fragments. From different, libraries mix, them together into, an equal molar pool, and sequence, those all together with. Increasing, throughput that I've told you about and. Actually generate data from a multitude, of different individuals. All at the same time and then, deconvolute. That, pool using, the DNA barcode, information. Once we have the sequence data available, to us so this just takes the place as another read, that. Is actually. Sampling, the DNA barcode, information. To identify the library, that that actual. Fragment. Came from, once. We have these libraries. Created, we do a quantitation. That, tells us the dilution, of the, library that should go on to. The sequencer, or into, the pool and then, we either proceed, directly. To whole genome sequencing, or we, can perform exome. Sequencing, or specific, gene hybrid, capture, approaches, which, I'll tell you about just next. So. I want to talk first about this. Amplification. Of the library, that's required, which, uses, PCR, and the adapter, sequences, to just increase, the number of fragments that are in the sequencing.
Population. And the, reason for mentioning this is because there are some confounders. Here that you have to know about in terms, of the downstream, data analysis. So let me walk you through this so, obviously, PCR, has been with us for quite some time now since the mid 80s and it's a very effective way for amplifying, DNA but there are some downsides to, it in. Massively, parallel construction, because. We're doing PCR, after, the adapter, ligation you. Can actually get preferential. Amplification. This is sometimes referred to as Jack potting, and what, this means is that just smaller fragments, tend to amplify better. And so, you may get an over-representation. Of, those fragments, in, the read population. That can lead, to duplication. And, the problem, here is that if you also incorporate. For example, a polymerase error early. On in the PCR, amplification. The. Multiple, components. That are coming, from duplicate, reads can make that masquerade. As a true variant, when you go downstream, to analyzing the data so. We need to be aware that Jack, potting can occur um this, used to be a problem but, we now have good, algorithms. Essentially, look for exact, same start and stop sites from. Aligned reads onto the genome and eliminate. All but one representative. Copy of those duplicate, reads so this is algorithmic. Now whereas we used to have to do very careful, examination of. The alignment. Of reads back in the day and. I think about Jack potting a lot in my work because. In cancer, samples you often receive a very very tiny amount of tissue, from. Which DNA can be extracted, and of course the less DNA you have to put into a library the. More of a problem this becomes, and with, formalin, fixation, and paraffin, embedding, often the DNA is also fragmented. Into small pieces even, before the fragmentation, step, and smaller. Pieces actually, lead to an increase, in jak potting and duplicate, reads so this is a real concern for the, data analysis, piece I've. Already kind of alluded to this in my previous comments, about Jack potting but we can get some false positive, art artifacts, because, PCR, is an enzymatic, process. And it can introduce errors, it's not perfect, and. If it goes in early PCR, cycles, this will appear as a true variant, and then. Lastly, is I'll talk about cluster. Formation, or amplification. Once. You get these fragments. On to the solid, surface that, you're going to do the amplification, on is actually, a type of PCR. And. This can introduce bias in amplifying, high or low G + C content fragments. Since there's no guarantee, of the base content, of any. Given fragment. Some will actually, amplify. Better or worse depending upon the percentage, GC, and as a result, you may not actually detect, these fragments, from the library, as well as something that has a more balanced, ATGC. Component. To it so, these are all considerations, that, come, from the use of PCR the. Trend over time now. Has been actually to make the DNA sequencing, instruments, more sensitive, so that you actually have to do fewer cycles of PCR in, the library amplification. And, solid-surface. Amplification. Processes. And this, can lead to a reduction in, jak potting and also better representation. Of high, GC, low, GC, fragments, but I would argue that it's not still, perfect, just yet so these are things to be aware of now.
I Alluded, to hybrid, capture, and so just historically. When we first had next-generation, sequencing. Instruments, whole, genome sequencing, was sort of the only option, but, around, about 2009. Several, groups actually developed, this approach in various forms and flavors and it gives us an opportunity to, actually use, DNA. DNA or RNA DNA, hybridization. Kinetics. To, subset, the genome, into just the regions, of the genome that we care about so this is often referred to as exome, sequencing. Where, the probes that are used in hybrid capture. Correspond. To all of the genes annotated in, the reference genome of, course you don't have to look at all the genes you can actually look at a subset, of the, exomes such as alkyne aces for example, and generate. Sort. Of custom, hybrid, capture, probe. Sets to do this so how does this work well. Like everything, we start from whole genome sequencing. Libraries, but, in this case the way the sub setting happens, is we combine the whole genome library first. With, this sort of a. Capture. Reagent, which really consists, of just synthetic. Probes that are synthesized, to correspond, to exons, of the exons, of the genes that you're interested in and the, secret sauce here are these blue circles, that are shown on the surface, of the probes. That really reflect, the presence, of biotin. Elated. Nucleotides. That are part, of those probe sequences. So. In mixing. Together the the. Synthetic probes, with, the whole genome library under appropriate, conditions for. DNA DNA or DNA RNA probe, hybridization. Sometimes, these probes can be RNA, instead of DNA you, get you effective, hybridization. Between the. DNA, fragments. In the library, and the. Corresponding. Sequences, of the probes which represent, the regions that you want to focus on in your sequencing. The. Secret sauce biotin. Comes into play when you now actually, want to isolate out, the hybrid capture. Fragments. Of your library, and this, is by mixing, the a whole. Hybridization. Mixture with streptavidin, linked magnetic, beads and of course biotin, binds very tightly to streptavidin, so, then by applying this horseshoe, magnet, or some facsimile, thereof, you can actually pull, down selectively. The hybridized. Fragments. From your library and throw, away all of the regions of the genome that you don't want a sequence, this. Isn't perfect so we do several washes, to actually, release additional, spurious. Hybridized, fragments. And then, we can just simply. Denature. Away, the library fragments, from these captured, probes, they, stay with the magnetic beads and the, hybridized, library, fragments, that have been selected, out float, free and solution, and then can be amplified and sequenced after, their quantitated. So. This has actually reached a fairly high degree of art again, where we can take these bar-coded DNA libraries, now make, an equal molar pool, and just, go against, one aliquot, of the exome, capture reagent. For all of the library, molecules, from all of the individuals, that we want a sequence, sequence. Those out on a single lane of the sequencer, for example. And then d convolute, the data into different pots that correspond. To the DNA barcodes, and indeed, to the different individuals, that have been sequence so this is now very very, high throughput where we can combine 12. To 14 individuals. From an exome capture into. A single, lane of a, high-throughput, sequencer. And get that information, very very rapidly, and then, just to finish, as I mentioned, we don't have to do, the whole exome, we can design custom, capture reagents, just. For specific loci. Or genes of interest and only study, those from the standpoint. Of winnowing down the whole genome to the regions that we care most about now.
That's, Not a perfect, or infinite, winnowing. So just to be clear we, there's. A sort of lower limit, of about three or four hundred mega. Bases of sequencing, information, that you can efficiently. Sequence, through a hybrid capture, process below. That you really need to go for, purposes, of sequencing. Efficiency. And decreasing. The amount of spurious, hybrid. Or off-target effects, to, something that's more like this, I'm so, back to our old friend PC, are we now have ways to design, probes. Sorry. Primers. For PCR amplification. Across. Different, low sigh in the genome, to amplify out small, numbers, of genes in their, entirety, and. Take these multiplex. PCR products. Turn those into a library and sequence, those directly, and this is really best for very small regions. Of the genome as I, said below about 300, mega bases where you don't, want to pay a price in off target sequencing, effects this. Is also not a perfect, approach because of course it's, hard to come up with pcr primers that all play well together in, the same PCR. Amplification, you, know GC, bias and, those sorts of things coming into play but, actually most manufacturers. Of these primer. Sets, now have the, ability to either sell, you something that's already configured and, well, you. Know sort of tested. In terms of giving good representation. Or you can actually work with the manufacturers, to design a custom set, of multiplex. PCR. Amplify. Primer, sets as well. Okay. So back to the actual sequencing, reaction, now that we've even either decided, to do old genome sequencing. Exome, or a subset, or a multiplex. PCR how. Does the actual sequencing, reaction, work and since, I'm a chemist, by training this is the part that I really enjoy. Talking about so hopefully, you'll indulge me here so, in this illustration. What. We're going to be focused on is really the Illumina, sequencing. Process. In, particular so, let me walk you through this now, earlier I said that we have this flat, silicon, surface that has adapters, ligated. Covalently. Rather attached to the surface and those, sequences, correspond, to the adapter, sequences, on our library ok, so once we've got our library sort of quantitated. The. The, instrument, will introduce the, library fragments, onto the silicon, surface and, you'll, get just hybridization. Under the appropriate conditions of, individual. Fragments and of course the reason for carefully. Diluting, these fragments, is so that you get the right distribution. Of, these amplified, clusters, across the, surface of the silicon, that's then going to be viewable by the instrument, optics, what.
Follows, Next to a series, of amplification, steps. Which I referred to earlier is bridge amplification. The, reason, for that is that in the course of this amplification, of the the free end of the, library, fragments, finds the complement, down on the surface of the, chip and then you get essentially, a polymerase, by. A stepwise. Process, that. Builds, increasing. Numbers of fragments. In sites you for each one of these hundreds. Of millions of library, fragments, that are down on the surface of your chip so, at the end of this bridge amplification. Cycle, you might end up with a cluster of fragments, that looks like this, so sort of on the order of a hundred thousand, or so copies. Of the exact same molecule and, if. You image this cluster, it would look like this sort of bright dot and if you look at a bunch of clusters, that are all together in, one small, area of that chip they, would look a little bit like a star, field and indeed, the oldest. Versions, of the software for, this type of sequencing, were really derived, from, individuals. Who had previously. Been studying, deep, space images, so it's a little bit like D convoluting. That where you have to identify, the, cluster, and then isolate its signal from all other adjacent, clusters, to the best possible, so that you get the truest, set of signals coming out of it, and, then so we don't amplitude. On sequence, this amplified, cluster, like this we, actually have to go through a series of steps that releases. One free end of all of the molecules in, the cluster there's just a single representative here but, on that freed end we then. Hybridize. A sequencing. Primer that corresponds, to part of the adapter, sequence, and this, is pointing now down towards, the surface of the chip and as, you'll see here. In this blow-up, of this sequence, to be sequence, fragment, we can then get a polymerase, molecule to. Recognize, that DNA DNA hybrid. And now, with the inclusion of sequencing. Substrate such as these labeled. Deoxynucleotides. We can start, our sequencing, process so. This is now the amplified. Fragment shown, in isolation, but imagine, that there are hundreds of thousands, of copies of this in the cluster, they've all been hybridized, by, this very specific. Primer sequence, here and we've got at it's in the free three prime hydroxyl for. The polymerase, to begin adding on nucleotides. In. The Illumina, process, these nucleotides, are very. Specialized. As. They are in. Other platforms. But, in particular, they have two attributes, that, are shown here one, is that they have a floor, that's specific. For the identity, of the nucleotide, so a fluoresce, is at a different wavelength and, CG and T the. Second thing that's specialized, about them is the three prime hydroxyl group. Is actually blocked, with a chemical blocker the, reason for this is that in each of the sequencing, by synthesis, steps for the Illumina process, you, just want to ideally, add in a single nucleotide at a time detect. It with the optics, and then remove, the block from the three prime ends so that you can now bring in the next nucleotide G, in this example and cleave. The floor so that when this new G nucleotide, gets imaged, by the optics. Of the instrument. There's no leftover. Residual. T fluorescence. To interfere, with the identification.
That That is in fact a G that's been incorporated. So. If. We look at this sort of then ideally. What we would end up with at the end of these two cleavage. Steps is a free, three prime hydroxyl and. A, the, absence, of a fluorescent group, where there was one on so, that the next step of incorporation. Can be successfully. Detected. Now. This is a point where you might be asking yourself well this sounds really great why, can't we just sequence this entire fragment, it you know and make, the fragments, even longer than 300. Or 400, base pairs and then we could get really really long reads out of this technology and, our lives would be simpler, would. That be the case I would, love it the limitation. Here is signal to noise okay. So two, things contribute, to that one, chemistry. Is never a hundred percent so although you try to cleave all of these floors. Off there, we'll be some residual, fluorescence. That remains, and that will interfere with subsequent, imaging, cycles, they, might disappear, in subsequent cycles, but they may be there to interfere, a nonetheless, it's, it's it's. Unclear. And. Not not, a hundred percent as I pointed out similarly. There. May actually be the, absence, of a blocking, group on some of the nucleotides, so rather than just incorporating. The T in this first cycle, I might, actually incorporate, a T or set of T's without, the blocker and then G's can come in right away because. Everything, is supplied at once in this type of sequencing. And. Then I would get a set. Of fragments, that are so-called out of phase that, means they're now sequencing, one nucleotide, ahead, of everybody else in the population and, over, time this is an increasing, phenomenology. So what, happens, over time and increasing, cycles, of incorporation. With this approach is that noise, increases. And so, at some point becomes, equal to the signal that's being produced by all of these fragments, that are being sequenced, in. The cluster and so, you begin to lose the ability to define, with. High. Accuracy, which, nucleotide. Just got incorporated into, the fragment, and so, this is increased, over time the first aluminous. Alexis, sequencers, that we use back in the day in 2007. Had, read lengths of about 25, base pairs the, current, read lengths are now, 150. Base pairs so, there has been an improvement over time in the read lengths that are available and, similarly. After, we go through one set of sequencing. Like I've just showed you coming, from this end of the adapter we, can actually go through now with some additional, amplification. Cycles, and release, the other end through different, chemical. Cleavage, prime. It with a different primer. And now sequence. The opposite, into the fragment, so this is so-called paired-end, sequencing. Where we can now collect 150. Base pairs from each end of the fragment, and those, pairs as I'll talk about in a minute can map back to the to, the genome of interest. Now. Just one a couple last slides on Illumina, there, overall approach has changed whereas they used to just have these empty. Lanes. On the silicon. Derive surface, shown, here, now, they're actually patterned, into these little pits, on. The surface, of the flow cell so each Lane and consists. Of a hundreds, of millions, of these that are in a very defined, order sort. Of like a honeycomb and this. Is a so-called pattern flow, cell and. What this allows you to do is now pack the clusters. Very very closely together to one another and also, to not, have to find the clusters, you know where they are essentially. Based, on how that flow. Cell sits in the instrument and the fact that this set. Of patterned. Pits, on the surface, or is a very uniform, array I mean, so now what we get and this is highly. Idealized shot, from their website, is, just sort of this is where the amplification, reaction.
Takes Place and in a best possible world all of the regions, around this particular, portion. Of the pattern flow cell are entirely clean, and so you get a very clean, distinct. Signature, from, that and all of its companion, wells as well. Okay. And then just to finish with Illumina, and this is just a shot from their website to show you one thing which is that you, can sequence a little or you can sequence a lot I think just basically, to cut to the chase this. Is sort of their highest throughput, instrument, the hi-c jacks which which can sequence on the order of I. Think. It's like 12 human genomes, you know in a 24 hour period so, it's, very very high throughput this. Is more like the desktop sequencer. And if you talk to people in the field about Illumina. Is you know strengths, and weaknesses, you'll. Find that the accuracy of the sequencing, is high so less than 1% error rate I'm collectively. On both reads there's, a range as you can see of capacity, and throughput some. Of these platforms actually the my seek has very relatively. Long read lengths you can get 300, base pair paired-end reads from it so, that improves, part, of the problem as well but it's a lower throughput, sequencer, so you can't sequence a whole human genome on, it and. Then. Are some improvements that have been coming along over, time including, the ability to do cloud computing, which we'll talk about in a minute. And. Now let me shift gears to a different type of sequencer. Than the fluorescence. Based Illumina, sequencer, just, for the series of completeness, this, is using a different, idea which is actually. The fact that when, you incorporate, a nucleotide, into, a growing, chain, that's being sequence. There's actually, the release of hydrogen ions, so this is using that, release, of hydrogen ions the resultant, change, in pH to, actually detect when, and how many nucleotides, have been incorporated in the sequencing, reaction and. This is offered out in the form of an ion torrent sequencer, which, is available commercially. As well and. So the idea here is be, based amplification. So you can see the round bead here with the derivative, surface, having these adapters, to which the library. Molecules. Are individually. Amplified. So, the best-case scenario is that each bead, represents. Multiple, copies again, of the same library, fragment, this, is done in an emulsion PCR approach. Where you mix together and, make, my cells that contain in the best-case scenario the. Single bead a single. Library fragment. In all of the PCR. Amplification, reagents. That are, necessary, going, through PCR, type cycles, to, decorate. The surface of this bead which with each of the library fragments, that you would like to sequence, these. Are then loaded onto a chip which is this sort of idealized, structure, here, and consists. Of two parts the, upper part where the bead sits in the nucleotides, flow across is, really. Sort of the molecular. Biology, part of the action, if you will and the, lower part is really just a very miniaturized. PH, meter, that. Senses, the release of these hydrogen ions in. Flows. Of different nucleotides, and registers. The, corresponding. Amount of signal to, tell you which nucleotide, was incorporated. Based on which one is flowing, across at the time and. How many of those were incorporated. How does it get to how many now, these are native nucleotides. So they have no fluorescent, groups no modifications. Whatsoever so. Keep that in mind so, if you have a string, of A's for, example, in your template, that's, being sequenced you can incorporate as many T's as possible, to correspond, to the number of A's that are there the. Way that we discern, what. Got incorporated and how many is based, on a key sequence, which is shown here this is on the adapter itself that's used to make the library and, is, the, point at which the sequencing. Begins and so, when we flow through a defined, set of the four nucleotides we. Will get a signal, from each one of these in corporations. That's equivalent to a single base, worth, of pH change, if you will and that's it's the standard, for what a single nucleotide, incorporation looks.
Like So that's the key sequence which. Then forms the template, off of which all subsequent, and corporations, are gauged so. In that sense where you have 4a nucleotides. In a row you'll have a signal, that's approximately, four times greater in terms of the pH change, compared. To a single nucleotide incorporation and. The software, can go through after the run and evaluate, this and the resulting, sequence comes, out and is available for. Downstream, interpretation. So you can kind of see this idealized, here, where we have the key sequence and then multiple, incorporation. Some of which spike higher, degrees. Of signal. PH, change, than. Others do and so this is really the way that the sequencing, takes place in. The Ion Torrent system. This. Has two, platforms available again, more of a sort of desktop, sequencer, called the PGM, and then, a larger, throughput, sequencer, called the proton, and you can see the different attributes here just. To point out this is not paired, in sequencing, but because of the read lengths, here, up to about 400 base pairs, you just sequence from a single end so. There's not a read pairing, that happens with this type of an approach but the read length is longer and if, you look across the various, attributes of, this sequencing, platform, we really like to use this, oftentimes. To counter check the Illumina, sequencer, because, it has a very low substitution. Error rate since, each nucleotide, type, flows, one, at a time with a wash in between you almost never see a substitution. Error where the wrong nucleotide. Got incorporated. Because. Of the way that a reagent, flow works however, because. Of the sort of relative. PH, change, that I just talked about when, you get above a certain number of the same nucleotide. Occurring, in a row you, can actually lose, the linearity of response, and so, this masquerades. As a problem, with insertion, deletion errors. In a single, read however.
An Averaging, approach with multiple, coverage, across that region of a homo, polymer, run can, usually get, you to the right answer so it's a consensus, accuracy, approach that's. Really needed in. This in this type. Of sequencing, and. This is a relatively, inexpensive fast. Turnaround, platform, for data production so as I said we typically use this in the lab for. Focused. Sets like the multiplex, PCR that, I talked about earlier and, also, for, I'm just data checking, for. Variants that we want to proceed with from, our Illumina sequencing, pipeline. Okay. Let's talk just a little bit about bioinformatics, I, don't want to get too deep in the weeds here but to give you an appreciation of, the challenges, of short read sequencing, so, I'll focus my attention here on the human genome which is the one that I study the most you can substitute any reference, genome in place of the human genome in these slides, really. What we're doing here now with these short read technologies. Unlike. Back in the day with Sanger, long reads is we're not doing an assembly, of the sequencing, reads where we try to match up long stretches, of similar, nucleotide. Sequence, and sort of build a contig, or a fragment. Of long sequence, over time here. With short reads sequencing, and especially with genomes that are complicated, as the human genome sequence you. Actually have to align. Reads, on to the sequence rather than, assemble. Reads the lower, you get in terms of your genome size so viruses, and some simple, bacterial, genomes you actually can do assembly, but for large complicated, gems it's really, not a formal, possibility. So, alignment, of reeds to the human or other reference, sequences, is really the first step before. You go and identify, where the variants, exist, and. In the spectrum. Of using, paired end reads we actually, can identify for, example a, chromosomal. Translocation. Where a group of reads on one end actually maps to one chromosome, and the other end of the fragments, maps to another chromosome, thereby, identifying that, there's something that's gone on there to marry up those two chromosomal. Segments, together and, I alluded to RNA, sequencing, earlier RNA, sequencing, data goes through the exact same process of, alignment. Followed, by downstream. Interpretation. So there are no differences, and in fact across, all the things you can do with ohmic data. Alignment. To the reference, genome is really always the first step so. Just. Think about it like this because human genome is large 3 billion base pairs, lots, of repeats, about 48%, repetitive. So, it looks a little bit like this jigsaw, puzzle, where here's all the repeat spaced in the, grass and the sky and the tree is the genes that. Are you know are sort of interspersed, into all of this so here, are all of your short read sequence, data that you have to figure out where the original came, from or. Then when you made your library, and a lot of the pieces look a lot like each other so it's sort of difficult to figure out exactly where, they go but when you find this tree you can accurately place, that with, a pretty high degree of certainty and so, how do we deal with this sort, of confusion, about mapping, where, and, and how accurately, and. That's really just because. We've been able to come up with a variety of statistical. Measures, of certainty, that. Tell us that if the read can map here, or here. Our. Best possible guess, comes. From a variety of mapping, scores, it sort of tells us that the read is most likely to map here as opposed to the other places this. Has been tremendously. Enhanced. I should point out by pair to end sequencing. Because, oftentimes you can get a read, end, that maps into a repetitive, sequence but, as long as that other read from the other end of the fragment, maps out into unique sequence, you increase, the certainty, of mapping, for. That particular, sequence in the genome. So. Once we have the read align then we have to go through a series of steps that I won't spend a lot of time on but, just by. The sheer number to let you know that this is an important, aspect before, you go actually into variant detection so. If, you're interested, in structural, variation, or other aspects, of read pairing, you have to go find the read, pairs that are properly. Mapped. At the distance, you expect, based on your library fragment, size, on. Average, and the ones that are not if you want to then go subsequently. To identify, M structural, variations, as I talked about you, also want to eliminate and mark and eliminate, the duplicate, reads this is done through an algorithmic, approach.
Correct. Any local misalignments. This is just getting rid of sequences. That are aligned, to properly, calculate. Quality. Scores and then finally, go through a variant, detection process. Which is again an algorithmic, look, at how, well the sequence. Of your target. Map's. Back to the reference, and this allows you to identify single. Nucleotide. Differences. Between your, sequence, and the reference, sequence and on average in the human genome you, see about 3 million of these per individual. Sequence, we. Then need to evaluate coverage. Because coverage, is everything, coverage. Really reflects, to the number of times that you've over, sampled, that genome, how deep, is the sequencing, on any given region of the genome and what, this really goes to ultimately. Is your certainty, of identifying. A variant that's real as opposed. To something that simply doesn't have enough coverage, to really support that. That variant, is, is accurate. And. So one of the things that we do in terms of evaluating, coverage, is we compare these snips that we've identified from, our next-gen, coverage, to, snips that come from array data like a genotyping. Array so if you have a high concordance, there you, probably have sufficient, coverage on your genome, to, look at those data downstream. In. An interpretive. Way and we, can also compare, snips from tumor to normal, and the cancer sequencing around, where we've sequenced both the the cancer, genome and the normal genome and identify. The number that are shared between, those two genomes because of course the constitutional. Snips of any individual. Will, come through in that tumor genome as well as the somatic, variants that are unique to the tumor itself we. Can also look at the data so this is comforting, to people like me who over, the years we're, used to looking at you know autoradiogram. X' and then chromatograms. On a computer, screen and now you're sort of like what do i look at but as i'll show you in a minute there's a nice viewer that we often use for what we call manual. Inspection of sequencing, data and we can also generate, tools to give us information about coverage, as I'll show and. When all of these things check out then and only then can we finally analyze, the data, to. Interpret the variants that we find there so here's just a quick look at igv which is sort of the commonly used tool across next-gen, sequencing. Laboratories. To look at coverage and, other aspects, you can get a whole, chromosome. View or you can zoom in to specific, areas you, can see here in the gray bars all of the coverage that's resulted, in this area, and you can even get down to the single nucleotide level, to identify a clear, presence. Of a variant, compared, back to the human reference genome and. Here's. Another igv, shot just showing what I normally do, which is look at the normal, coverage, and the tumor coverage where you're clearly identifying. A somatic, variant, here that's. Unique to the tumor genome itself. And. Then lastly, just to give a plug for a tool that we've come up with there's a very long list here but my slides are available or, a URL, here this is just a tool that takes the bulk of a bunch of captured. Data that, I talked about earlier and, really compares, the coverage, levels, according. To a variety of color coded, depth, levels, here and looks, at the breadth. Of sequencing, coverage and also the amount of enrichment that you've been able to do and these bulk tools are really necessary in high-throughput sequencing so.
You Can rapidly, evaluate. Whether these are data that now need to go downstream, to subsequent analysis. Or back, to the sequencing, queue to generate, additional coverage, if the coverage levels are inadequate. One. Of the things I get asked a lot is sort of well what's better whole genome sequencing, or exome sequencing. I guess. My typical, answer is it depends, on what, you want to do with the data so. I won't try to bias you one way or the other but just give you some facts and figures here. So, this is just sort of looking at the sequencing, data how much exome, is about six Giga. Bases whole, genome much larger, and this can increase when you're sequencing a cancer genome because more coverage is always better there you. Can see obviously the different target spaces, and this number varies, depending upon the exome reagent, that you're using so this is sort of an averaged, amount, mapping. Rates are higher for exome. Than whole genome because whole genome is harder to map because of all the repetitive, sequences, that I mentioned, already, duplication. Rates tend to be a little higher for exome, sequencing, it's less of a unique set of molecules and, these, are the kinds of coverages, that we typically, achieve. Although they can be higher if you want it's really just a matter of economics. How, many of this coding. Regions, or c.d.s are covered at greater than 10x, on average, you get about this much coverage for exome, significantly. Higher for whole genome and that's just because we're. The probes hybridized. From in the genome is sometimes, differential. And. You may get better coverage, just by sequencing. Across the whole genome as well and then, really important, is what you can get from exomes sequencing, which is good point mutation, and in Bell calling, but not so much resolution, on copy number, and really, it's difficult, but not impossible to, call, structural. Variants where, is really pretty much everything, is available from whole genome which, is intuitive, the, most challenging, thing is calling structural, variants, just because there's a very high false positive rate, on to, this type of an approach and, then. Lastly, you know what do you worry about well, it depends on if you're in a clinical, setting or in a research setting in a clinical setting we worry about false, negatives, because we don't want to miss anything and most, of these are actually due to lack of coverage, so an exact examination, or, post, variant, filtering, approach to read move, lack.
Of Coverage read that. Are indicating. Mutations. That we've missed is a problem. False. Positives, are more important, maybe in the research space where. These are some of the sources of false positive, 'ti but, we've actually found, over time that going back and revisiting these, sites can lead us to, filters. That can actually remove, the sources, of false positive, 'ti such as variants that are only called on one strand or right, at the end of the read where your signal-to-noise, is, starting to approach each other so. I'll end here with the bioinformatics, diatribe. But I just want you to sort of appreciate, in a. Microcosm, all of the factors, that go into this and why. Incorporating. Massively parallel sequencing. Especially. In the clinical, space is actually fairly problematic. Because, you have to not only understand. And appreciate all of these factors, you actually, have to build into your sequence analysis, pipelines, the ability, to deal with all of them so that the result you get out is as high quality and, high certainty as, possible. Because often, therapeutic. Decisions and, other types of clinical, decisions, are being made off of this and so, one of the current trends in the marketplace, is. To sort of package, together for clinical. Utility. Multiple. Systems, that allow you like this chitin system shown here that allow you to sort of produce, the library, as sorry produce the DNA RNA produce, the library, do, the sequencing and then have all of the bioinformatics, and, analysis, packaged, for you at the end of it so it's sort of a sample, to inside as they call it type. Of solution, so this is just one example, where. You have modules for, all of these things and then you have onboard analysis. And interpretation. Software. For clinical, utility of. Sequencing. Data, okay. I want to turn my attention to third-generation. Sequencers. And, focus, really on single, molecule, detection now, as opposed to bulk, molecule, detection which is what we've been talking about and what, you're staring at here is the is, the, so-called smart, cell of the PacBio sequencer. Which, is. Use for real-time sequencing, of single DNA molecules, this, is the. Surface of the smart. Cell that is the action, part, of the the operation, here, a man consists, of about. 150,000. So called zero mode waveguides. Little, isolated, pockets. That individual. DNA and polymerases can, fit down into, and. Can be sequenced and watched in real time as the, sequencing, reaction occurs, so how does this work, first. Of all you make a DNA polymerase complex. And this gets immobilized down. At the bottom of the zmw, where the bottom is specifically, derivatized. The, sides of the zmw, are not and so on average what, ends, up is that on this polymerase, is sitting right at the bottom of the, zero mode waveguide, well why do we want it there well, because, we want to add in now fluorescent. Labeled nucleotides. That the polymerase can incorporate relative. To the Strand that it's glommed, onto and, these, fluorescent nucleotides. Are, evaluated. As they come into the active, site of the polymerase, which, is in the viewing area, of the optics, of the instrument. Which is focused, on each one of these 150,000. 0 mode wave guides what. Happens when you get an incorporation. Of course is that the, nucleotide. Sits for, long enough in the active side of the polymerase, for, their fluorescence. To be excited, by the.
Imposing. Wavelength. That's coming into the bottom of the zmw, you. Get a fluorescent, readout that's captured, by the optics, and the detection system, of the instrument, and. The. Phosphate, group. Is actually cleave during incorporation. And it contains, the fluorophore so it diffuses, away and, now, you're ready for the next incoming. Nucleotide, to. Sit in that active, site long, enough to, also. Excite, its fluorescence, and get a readout and so you get a base, by base readout, that occurs, based, on the fluorescent, emission wavelength. Of each of the nucleotides, which are specifically, labeled and this. Is a process, that's occurring, in parallel, in all of the ZM W's that contain a polymerase, with, a DNA fragment that's, properly primed. And what you're essentially doing with this device is really taking a movie, of all, of these zmw, so verda find a period of time, during. Which the data, is accumulated. And. I'm not going to walk through this extensive workflow. In any, way shape or form but just to give you an appreciation of, what it takes to process. The, library, some of these steps are very similar, to what we've already talked about for massively, parallel sequencing instruments. But, the big difference here is that you actually polymerase. Mix. The polymerase, the primer and the library, fragments, together and, then. Apply, that onto the sequencing, instrument, as you can see here we collect data in the area of four to six hours of collection, time. From. The from the zero mode waveguides in the smart cell so. This, is a really. Different. Departure. From what we've been talking about for a variety of reasons, first. Of all the idea here is to start with again high molecular weight genomic. DNA but. We actually share to very long, read length sizes, about thirty to fifty even, up to eighty kilobases. The, reason why is that during that four to six hour run, from, individual, fragments, that go into the library prep that are this long we, can literally generate. Sequence, reads that are in excess of 30,000, base pairs averages, about 15,000. So as you can see this is diametrically, opposed to all of that short read sequencing, that we were just talking about so. The. So we've worked out methods, for consistent, sharing of DNA to these long read lengths as you can imagine it's reasonably, fragile, so, there are a variety of devices. That we use to maintain the stability and, also to make the library as. High, quality as possible and, this, does take a pretty, good amount of DNA so to, sequence a whole human genome as I'll talk about in a minute is a considerable, investment that really at. This point in time does not render - you, know an individual. Core. Biopsy, from a cancer sample, for example now we're talking about sequencing. Really here from, cell lines where you can generate lots and lots of DNA to. Get the kind of coverage that we need 60 fold or higher, how to sequence the human genome, this. Is just an example of some of the read lengths that are attainable. From. Some recent examples where, you can see the mean read lengths are sort of in the 13 15,000, base pair reads and. Some of these bins go extraordinarily, high. Towards. Generating, this and the reason for pointing this out is now we're moving from a realm where we need to align reads back to a human reference genome to.
Rather Coming, up with algorithms, that can assemble, these reads into portions. Of entire human, chromosomes, and this is of course really important, for a variety of reasons, one, that I'll talk about today, is that we're trying to use these long read technologies. To, not. Only improve the existing, current human, genome reference but to also produce additional. High-quality. Human, genomes, to, sort of spread out the knowledge, about diversity. In the human genome across, different, populations. Across. The world and also, to really understand. The unique, content, in genomes that you can't get by, simply aligning reads back to a fixed, reference, so. This is just a snapshot, from, our website, that talks about our reference, genomes, improvement. Project, which, is funded by NHGRI. And. Shows you that we plan to produce gold, reference. And already, have produced, some platinum reference, genomes, and the difference here is that these are haploid, genomes, they come from a an. Abnormality. Called, a high data to form mole where you get a single a nucleoid egg that's. Fertilized. By a sperm and this really grows out to a certain stage and then gets turned into a cell line so, these are haploid high-quality. Human genomes, these will be from, diploid individuals. As. You can see across different. Populations of, the world the. Plan is sort of outlined, here and this is again linkable to our website, of the URL which I just showed, starts. From PacBio, sequencing. Reads de, novo, assembly of those reads which will be contiguous. Across, long stretches, but not perfect, and then. Using, a different technology called, the bio nano which, makes maps of the human reference genome we, can actually get. An accurate. Presentation. Of how good our PacBio, assembly, is and how big the gaps are that we still have left to fill and in, some cases as I'll show you we're also using, the PacBio sequencer, to sequence from bacterial. Artificial chromosomes, made. From these same cell lines we, fill in the gaps using these data here, and come, up with a high quality gold. Reference, genome, that's highly contiguous. On to, the extent possible across. The chromosomes, so. This is just an example of the first gold genome that we produced. This is from a Yoruba, individual, in the thousand genomes project and. You can see all kinds of metrics here for the quality of the assembly, but, our biggest contig, is 20 million base pairs of, assembled, sequence, data that's pretty remarkable when you stop and think about it I mean on average the, contigs, are about 6 million base pairs. We. Can align, this as I said to the maps that are created, using the bio Nano which really takes similar, long pieces of DNA doesn't, in silica, or does a restriction. Digest, and then calculates, the restriction, fragment, sizes and maps, those back and when you compare, in this particular, example there's, a conflict, between the, PacBio assembly, and the bio nano data, which, you can see is now resolved.
By Alignment, of these back reads I'm using, the pack bio long read assembly. Approach here, I mean we can resolve. Significantly. To do this very complex, region, of the genome which, involves, a segmental, duplication. These are historically, the hardest, parts of the human genome to actually, finish to high-quality and, contiguity, but, here we've actually done, it I mean. So these are the. Approaches. That we're using sorry the different genomes the, source, or origin and. The, level of coverage that's planned and you can sort of see a current snapshot from. Just a couple of weeks ago of where we're at with producing, these data which of course will be available. For use, from. The NCBI. Ok, I just want to finish up here with a couple of new technologies. To mention, and this, is again 10x. Genomics, a company, that's really aimed at, high-quality. Contiguity. But, using a different approach than long read technology so how does this work well. What, the idea here is to generate. These little. Segments. So starting, with a long, piece of DNA similar, to what we would be using for a packed bio library, you, combine this in, a, isothermal. Incubation. In. My cells so similar, to the amplification. Approach, that we used for ion torrent so oil and buffer. Micelles, where, you have these little molecular barcodes. That sit down on the surface of this long molecule, and then get extended, for a certain period of time you then turn these into full, sequence, Abul libraries, by adapting. Is, already ligating, on these adaptors to the ends, of with. A molecular barcode. At one end and then the adapter, at the other end you can sequence and analyze these, and then using bioinformatics, take, these finish sequence reads and actually, combine them back using. A linked read approach, into. A full, contig. Similar, to what I just showed from the Assembly, of PacBio reads so, this is getting long range information from, short read technology and indeed this platform, this uses the Illumina, platform to, read out you're, starting, with this gel and beet emulsion, or gems approach, that I just showed you to take, long molecules. Into. A micelle. Partition. Amplify. Off of these different, molecular barcodes. In sequence, and then, use. These in the, sequencing, library that then gets read out and, the barcodes. Then identify. Those, individuals, short, sequence, reads as having come from that original long, fragment. That's isolated in that micelle, and, so using, this approach you can actually generate very long contiguity, by. Just mapping up, the barcodes, that from, the short reads and linking them together I'm using, specific. Algorithms. So. There are lots of things that you can do with this I see that this didn't animate properly. So sorry, about that but just go to the website for this approach, and it will show you the different things that you can do including. Long-range haplotypic. Information. And then, getting information like, I just talked about from, diploid de novo assemblies, I'm using, the supernova. Assembler, that's. Been created. By the 10x, genomics, crew. Last. But not least I'll just talk about Oxford nanopore sequencing briefly. To. Give you an update this, is a protein, based nanopore which is meant, to pull a DNA, fragment through. I'm using, a variety of mechanisms each. Nanopore. Is linked to a specific application, specific. Integrated circuit, that, collects data and basically. Fits. The data to a model, of what. Each nucleotide. Combination. Looks like inside, of that pore to.
Call The base sequences. And so during, the run you basically get a little output that looks like this which, reflects, the translocation, of the DNA sequence through, the pore and. You. Can also. Sequence, these reads twice, one. Direction, and then the other to get higher accuracy, information. On the, read links from this are variable, so. There's no sort of set read length it's really just sequence, until you have the amount of sequence information that you need for the coverage that you need and as, I mentioned this this. Data, collection really, is based on electrical. Current differential. Across this membrane that the pore is sitting in so, as the DNA translocates. Different, combinations. Of nucleotides, give, differential. Changes, in, the electrical, current, and then this can be fit back to a model. Of all, possible, multimers, this, has been evolving over time the error rates initially, were quite high with, improvements, in pores and software, I'm the newest. Iterations. Of this type of approach. Or error, rates, of around 10% for, the dual read where you sample, the sequence, twice and about, 20%, if you're just able to sequence through that sequence. Data at one, time so, you. Know probably in the same realm at this point in time as the error rate on PacBio. Sequencing. Which I apologize have forgot to mention earlier this. Is kind of what the device, looks like so this is a small. Sort of the size of a, stick. Drive or a thumb drive that you might put in your computer, USB. Port it actually fits and connects, to the computer via USB and. Then this is the actual sequencing, device here the flow cell which contains the array of nanopores. That the DNA translocates. Through and that, the data is collected and, fed, into this the. Associated. Computer, and then, can be analyzed, after. The run is completed, to. Give you information and. In, a promised, next, version, you, know will basically the. The company will basically put together a lot of these different, nanopore devices, into, a very large sort, of compute cluster, looking device that's shown here which. Has the formidable name, of Promethean. Okay. So I'm going to just finish up and with three more slides and, then we'll open for, questions so just give you a feel for kind of where things are going with regard to the application of, next-generation sequencing. In, the clinical cancer care of patients, and this, just, refers, to what. I call amino genomics. In. The past people. Who know a lot more about immunity, and cancer than I do teri. Boone Hans Schreiber and others actually predicted. That because, you have specific, mutations, as we've talked about in tumor genomes, that, produce proteins. That are mutated and, therefore have a different, sequence these, proteins, actually might look different to the immune system if he could sort of tell the patient's, immune system, about them if you, will and this could happen through some sort of a vaccine, mediated. Approach or otherwise. That, could, alert, the, immune. System, to the presence, of these abnormal, cells and lead, to their destruction the, problem in the passes, that identifying. These neo antigens. These, proteins. Or peptides that, look most different, to the patient's, immune system, was extraordinarily difficult, as, I'll illustrate in, a few quick slides here this is largely been overcome, by next-generation, sequencing. And bioinformatic, analysis. So. In the immuno genic realm, and in identifying, these neo antigens, we have three sources of data we, have exome, sequencing, from cancer and normal to identify, cancer unique peptides we. Can also obtain from next-gen sequencing the. La haplotypes, of the individual, so what are their specific HLA. Molecules, that. Will bind these cancer unique peptides and present them to the immune system and then, what are the RNA sequencing, data because most of the samples we'll be talking about are.
Very High mutation level. Samples, so not every DNA, mutation, results in an, Express gene or that Express, mutation. We, combine all three of these data types into an algorithmic, approach, that compares, the binding, of these altered. Peptides, the mutants, to, the wild-type peptides, and gives, us the ones that look most different, to the immune system based on just binding, to the to the MHC. And we call these, TS. Mas or neo antigens, and this can describe the cancers neo antigen, load i'm just, for those of you in the bioinformatics realm, we have a github, available. Pipeline, for doing everything that i just described. Including, the RNA filtering, and coverage based filtering, and that was just published in January this year so. Why do we care about this well, there's evidence from the medical literature that actually, patients, with the highest mutation rate, the highest neo antigen, load are the. Ones that are most responsive. To a remarkable, new class of drugs that are commonly, referred to as checkpoint blockade therapy. These, are the types of molecules that, antibody-based. Drugs that, release the brakes, on the immune system and actually, allow t-cells, to infiltrate. Identify. And selectively, kill cancer cells in, most cases and these are just two, publications, from the literature, showing what I just told you which is that the response. Curves look dramatically, different in patients, with high, versus, low mutation loads, here's, just another study, from the Hopkins, group again, looking at patients, with either mi
2019-03-17 19:04
Wow, really great.
LOL "it's so easy, even a high schoooolar can do it; even my daughter did it, back in the day" common attitude of directors!
Great talk!
Amazing!
Wow the nanopore device looks exciting, can't wait to hear what will unveil from it. Thank you for the upload.
The best presenter on NGS....period.
Hi It was really enjoyable presentation. I would like to ask you a confused question for me that is about ngs data analysis. Many commend line platforms, which are not easy to follow for non computational researchers, are available for analysis the ngs data. However, many ready programs such as galaxy and genus that are easy to use are available. So why we are still using the commend line programs? Your answer will be very appreciated. Regards Dr. Adnan Lahuf