Humans, Data, AI and Ethics – Lightning Talks
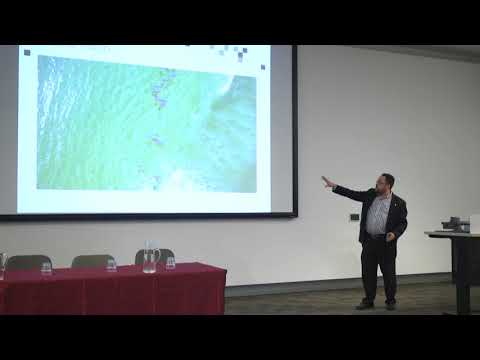
So. Without further ado we've, got Michael bloomin, Stein, talking. About AI, and data ethics, let's not believe the hype thanks, Mike. Thanks. Very much, Simon. Thank you all for being here I won't go through any introductions, so I'll just go straight into it I've only got 12 minutes so, I just wanted to talk to you about artificial, intelligence and, I, so, I know we're, talking about data and we'll get to that in a bit but al official intelligence is where I'd, like to start particularly. Around this huge rise of AI. Popularity. And to. The point where people are getting so freaked out that AI, is gonna you know come, back Terminator, style and do something bad to us so, I'm, here to say that, it's it's not exactly, where. It is at okay that that is that, is not exactly the full story so, you know we we think of artificial intelligence in terms of 2001. A Space Odyssey the. Bad computer, takes, over the spaceship, tries to kill the astronauts, that's terrible, well 2001, has been and gone and guess what that's never happened and the, reality is that you, know we're not even close to that happening you know but there are people out there the, Elon Musk's the Stephen Hawking's just yesterday apparently spoke. Out again that you know AI is gonna kill us in 40 years so, my, view is that we've got to be a little bit more realistic, one, of the major tests, for whether something. Is really. Going to be a computer that can fool humans, was this concept of the Turing test and. The ability, of actually is trying to communicate with the computer to see whether you could tell whether, the computer was actually a computer, and not a human now no, one has managed. To create something that was passed that Turing test yet not, properly so, the question is are we there yet well, we're not. So. You know artificial. Intelligence broadly, speaking these. Days sits, in two communities, one, is the concept of what we call computational. Intelligence that. Includes, things like neural. Networks which are algorithms, that mimic, the simplest, activity. Of the human brain okay or another, way to put it is connectionism, or connection, you, know connections. Science, but, then you've got the old-school artificial, intelligence, which looks at symbolic, techniques logic, fear improving and things like that believe, it or not at the moment the hype is in, this spot, here a hype, about you know these things called, neural, networks and now deep neural networks that are actually producing amazing results, and are scaring people because some people think they're black boxes, and you can actually understand. What they're doing so, this, area has been left behind a little bit unfortunately, and there's, actually come out of the spotlight but you there are your hardcore AI, fans, of the classical, approach that, are still there and that's fine but this is where all the interest is and where, all the money is at the moment so. Deep learning this has come about as a big buzzword who's, heard of deep learning before, right.
Fantastic, Well I'm going to too much detail about it then but I will just say one thing is that, it's the revival, of the old-school neural networks neural networks have been around or the concept, of a neuron in that official sense has been around since 1943. So, this is not new AI, is not new and this is not a new you, know. Transformation. Of the landscape it's, been around all the time we've just had some changes, that have allowed it to flourish so, an ends a large are loosely inspired by, the brains you know most, simplest, interconnected. Neuron behavior, but, the reality is that the. Thing about deep learning when it came along in in around between 2012, 2014, allowed. Us to manipulate, large. Amounts, of data the, algorithms, were improved and the ability to store the data actually manipulated, was, largely improved so. The applications, of deep learning a huge, there's so much around everything. From you know using, the, capabilities, inside drones, human-computer. Interaction, of course autonomous. Vehicles which Stephen, Hawking again is complaining about saying that you know it's gonna be devastating, for the human race don't, entirely agree with him on that one so, the reality is the, 80s actually was, was one of the times where there was a huge revival like, we're experiencing, now in 86, the backpropagation neural, network transformed. Everything every, paper and every artificial, neural network conference, was, in that space but, it's sort of slightly nearly, died down and around the 2000s. Bored and said, what's good what are we gonna do there but. All of a sudden faster, computers, graphical. Processing units, GPUs, that were used for, speeding, up games on people's, computers and laptops could, now be used for, training algorithms. And technology, that could never be done before because there was not the computing grant power to do it and of course Big Data so, 2012. With Alix net and around, that time was it was around the start of when things really went took. Off for the deep learning craze, so. What happens in a deep neural network very, quickly you design an architecture, in the in software. Or a package you train it with, lots and lots of data and then you can end up, with some you know recognition. Say of a face or an object or something like that that's the standard, application that's, where it started, image recognition things. Like that so. These, days you can get some code, seven. Lines of code can can allow you to. Implement. A deep, neural, network, basically that's it so very. Simple, you can almost, anyone. Can do it pretty much so. Many frameworks available cafe, 10:00 to 5:00 you've got a lot, of ways commercial. Products existing, even. Non-commercial. Products and off-the-shelf, software that, you can do it so. I want to show you one example of, where there, may be ethical, considerations. But, where there's a really great application, of this deep neural network technology, so, one of the projects that I'm involved in thankfully, but I've been fortunate enough to be asked to to, work with is.
In The area of using. Drones and analyzing. Video from the drones to detect sharks, so, of course sharks are, a really, big problem a real-world problem particularly. Australia, where we can see the statistics for, 2017. It actually improved in 2017, but actually if you look at it the, number of fatalities that year before was more and so as in 2015. But, basically, it's a big emotional, and political issue it's, something huge for our population, and there's a country that enjoys the beach it's, something important for us so, the question was can we use UAVs. Or drones to. Provide real-time monitoring, of beaches using. Some, sort of software, and. And artificial. Intelligence so. In, order for us to get. This project, to work we, have to collect a lot of data at the moment we've collected about 10,000. Video frames which have been annotated and so, there's understanding, of what, we're looking at so in other words is this a shark is this a fish is this a dolphin. Is this a while, we've, got a number of labels, so our our technology. Can now distinguish. Between, every. Type of well, main, you, know marine. Animal, group but, also other things humans, cars everything, like that so, we use deep learning based object detection to actually allow us to undertake, that, so. Where. We are up to now is that we're actually we. Haven't just developed the software and sitting in a in a closet in a some sort of research lab we're actually deployed, this software now the, company, we're working with is called little Ripper or now, transformed, to be the Ripper group it, is now can. Sign. An agreement with New South Wales government, that we're going to deploy this in 11 beaches. In New South Wales and, basically. This is going to allow people so, it's going to be launched in December 15th across. Those 11 beaches swimmers and beachgoers, are. Gonna probably, experience, another, level of safety like, a non-invasive. Level, of safety that doesn't hurt the Sharks, but also doesn't affect them with. This technology so. We're about to commence an international, collaboration where, some of my team. Are going to go over to Reunion Island and actually, test it internationally, and possibly. Get more, contracts internationally, so, this is the graphical, user interface, where it looks like very simple, you. Can see that things, are being detected in the ocean the boxes, labeled, suggest what they are these, are detected in real-time that, means that you know the window when the things deployed as soon as it sees it bang it's reported, back so at the moment the drone is able to send SMS text. It's it sends alerts it, can, be fitted to deploy a. Lifeboat. An inflatable, boat it can also use a megaphone to immediately, tell. The swimmer that there's something in the ocean that's approaching them. So. If we don't look, here here's some sample results, this isn't some, a large number of sharks detected as you can see there are a couple of unknowns. There, that, just suggests, that the system thinks there's a shark there but it's either too blurry or too difficult to tell dolphins. Plenty, you can it detects, that it detects boats it detects, kayaks, it, also can detect sharks. In really, difficult, conditions, particularly. Around things like you know where there's blur lots, of glare and and, even when the the water is murky so. We're very pleased, so I forgot to mention the accuracy, is around over 90% in. In Australian, oceans to be able to detect sharks. It. Can look at eagle rays drones. People, everything, else as well so, here's. A first. Audience in the history of UTS, to see this these, are the first snapshots of, detecting. Whales and sharks at, Reunion Island so, as you can see the water is quite different offering. An island and also it's, actually a totally different condition so of course, as with, any other imperfect. Day.i you've got to retrain it and you've got to provide it with different data to be able to work under different conditions so, that's some challenges, we're experiencing, particularly murky water brown looking. Water it's. Actually quite a bit of a challenge so I'd, like to finish off with this video and I'll just going to show you some. Some, real time shark, detection, in action and as. You can see there are some free chunky, difficult. Conditions, so it lost it there and then. It gets it back but it tracks it so it understands. That if it's lost it knows, where to find it again it, can look at it can detect people on the beaches are going to take vehicles, it. You know it's labeled those, are surfers but you know basically humans, eagle. Rays now. These are obviously, some of these are quite shots.
That Are taken from different distances obviously, when, it's not it was very close obviously, you get a better resolution you get better imagery, but when it's far away and particularly, when there's waves crashing, and other things really, challenging. Type. Of environment. To actually take so paddle, boats and so forth so, the we're. Very proud of where that's got to at. The moment the people that are testing it on our beaches are actually surf lifesavers, their command. Earing the, actual drone the drone technology so. It's actually, in the hands, of the people that save lives and and. Basically they're the ones not you know the technologists, that make the decisions on where to go next so, I will finish off very quickly. With. Just my perspective on the future of AI and basically, the the reality is that all, everything, I've showed you works, great you know here's a real-life example of something that's being deployed commercially. And for the benefit of the public good but. You know that is we are we are a blip in one spot of the AI spectrum, the reality, is there's more to come so, yes it, there's, going to be some controversial stuff down the track but we're a bit further from that then, we might think, so, for example one of the inventors of the backpropagation neural, network who probably is one of the biggest you know characters, at the moment he's now been employed by Google, you. Know now this. Guy's come up with a new concept of capsule, networks capsule. Met works I just been in neural networks but guess what they're on the drawing board since the 70s couldn't, get them to work but they were just sitting there in a theoretical sort. Of you know, situation. Where you couldn't actually get them to be, implemented, in real time, now, that's that's now working, the point where it's better and actually is mimicking, closer to the human brains function. The. Last the second one here is neuromorphic, AI so. The real in my opinion the real spot where we need to go to, get get. AI that we should be really scared of was, we can't be right now because I don't think we're even in that realm is AI, that really replicates, brain, like function, and actually understands. What the brain is doing and transfers. That into some sort of brain, like chip and neuromorphic ai is moving, in that direction the, reality, is that neurobiologists. Will tell you that we don't understand we only understand 10% of the human brain as it is now so it's, very difficult if you don't understand the human brain to actually replicate it so we're pretty far there the, last one of course in. The Faculty of engineering and IT we have the Center for quantum software, they have just, released two months ago a new quantum, programming, environment, you, can actually download it here this. Is one of the future directions they are using, quantum computers, beat, to be used in artificial intelligence so. We've actually got a really bright future from the technology, side and I would argue we have, a very bright future from the point of view of human safe thank you very much. Questions. My. Skinny run. Correct. My. Argument, is, that I, would find that to actually. Unpack. The. Evil, in us you'd have to understand, it and so, my view is that you know you're not you're not going to be I don't believe there is there is sufficient technology. Out there to be able to do that so, if. You're suggesting that maybe there'll be you, know a computer. Or technology, that can actually you. Know come about by. Not understanding our, brain as the basis. I'm. Actually, very. Very skeptical. Of that I'm, not saying it can't happen because then I couldn't. Be a scientist, but I would, say that it the probability of that happening is low I mean, to be realistic to be understimated understand, how. We can uh you, know really get something, that will be close, to what people are being really scared of at the moment we, really have to understand, our own biological. Function, better because. At the moment deep, learning and all the hype is on the basis of of a very poor, replica, and just. Like backpropagation, in 1986, went down, the tubes and no one even looked at since, 2000, this is this new generation, is going to go is, the expectations. Of what, what they think again we're gonna be able to get out of this they're, gonna be disappointed, so there'll, be a next big thing sure when, will that happen twenty, years forty years not sure but.
But It, I think it's firmly, based on on our understanding of the, most complex thing, in in the universe which is our brain. Thanks. Simon hi I'm cash yes I've spent most, of the past two years working, at the New South Wales data analytics Center which, is the New South Wales government, data. Science, hub, and. The opportunity, to be there a rose thanks, to the. MDS. I'd course director Theresa Anderson's, really, deep ties with. Industry and the degree has completely, changed my life, during. This time at the data analytics Center I worked, on proof, of concepts. Projects. To try. And apply machine, learning, to. Extremely sensitive domains. Including. Child, Protection and in. Particular in. Contexts, where machines, are replacing. Human decision-making so. Today I'm not at liberty to go into specific. Details but Simon, Buckinghamshire, was. Keen for me to share something key, learnings. From, the activities so. They, are presented, with sufficient. Abstraction, to one of my contract of employment but. With enough insight, to benefit, the, interest, that is palpable. In this room today. So. What, are we going today. We're looking at the ethics of the machine or why. Your machine learning algorithm, is associated Pathak psychopaths and what you can do about it, now. We're. Gonna have to do with some not so simple questions to get there so firstly what is ethics can. Machines learn ethics and to get to that we're gonna have to cover how much influence so. What is ethics ethics. Is a philosophical. Discourse on. What. Is right what, one ought to do and unlike, other philosophical. Conversations. About what is beauty what it's logic what is knowledge at. The difference, with ethics is that it compels action, you can't very well have a conversation about what the right thing to do is without, them going out and doing it, so. Can. We teach machines like this well firstly how do they learn. Teaching, a machine is much like teaching a child so. You show, them a. Picture of cat or, cats with, the label cat and. Repeated, enough times until, they're able to see a cat, without, a label cat for. Example out in the street and the child says cat that's a cat. So in supervised, machine learning the, pictures of the cats with, the, word cat next to them the, training, set and the, picture of the cat without the label is the chess set so. What's. Different one. Thing that's going on here is outside, of that there's no context, and a spectacular, example of what that means, is. Just, a few weeks ago in 7,700. Paint, colors we're fed into a neural net and these, are the some the ones that it created some of the good ones feel. Like. Dusty, pink and naval. Tan, and. Birth pink but these are some the ones that got wrong so I mistook. Blue, for gray and. Green. For brown, so. What, it was missing is, it. Didn't have any external truths about the. Sky is blue the grass is green or. Rose. Is red, and also white and yellow in pink. Another. Type of machine. Learning is unsupervised, learning and what. Are the advantage, and in. This process we're. Not giving. The Machine any labels, we're, letting it discover. Patterns. For. Itself and one of the advantages of that is that it's open to new, things. Or, new signals coming along. But. One of the disadvantages, is that. Because. There's an element of dimensionality, reduction what, it finds may, not be interpreted or, it. May not be actionable. I'm. Certain example if I put, on YouTube usage, data into, a clustering algorithm it, to. Find new segments, it, finds the segment of six.
And Sixty year olds that behave in exactly the same way but, that's no good to me if I can't market to them in, the same way so. Now. We know how they learn can we teach well. What's, going on with. Both of those methods is that essentially, there's. Algebraic. Operations, happening, within a representation. Space or, in other words concepts, are represented mathematically and, their, accuracy is is, tested, with statistical, methods so, in, this context, can we teach machines ethics, well, unless we're able to reduce ethics. Or in this case. Fairness. To. A. Mathematical, definition. Or, equation, that works in every context, for every subgroup then at this stage I'm gonna go with what our first speaker was saying which, is no we, can't teach a machine it means ethics which basically means we're dealing with a bunch of sociopathic, psychopaths so. Still. Don't believe me let's look at some of the common traits of, these. People, so, one is that they are. Socially. Awkward, boom. Machines. Totally. Socially awkward as well another. Thing is is that they don't show remorse. Look. At that ice cold. Another, one is. They. Have no self-control and we also heard earlier today now, when Robo traders wipe out a hundred billion dollars in market cap in a few minutes they clearly have no self-control, and don't, believe me this guy also seems to think that his computer is a psychopath, so. If that's how they operate. Then. What can we do about it. You may have heard of the interesting, case of James, Fallon who was a neuroscientist. Who inadvertently, discovered. That's, another story that he had the gene for full-blown psychopathy. Being. A psychopath and. Yet. He was successful, university professor, and a happy family man so what. He worked out was the difference between that. Gene being activated, or not once, he had an extremely happy childhood so. What do we need to do we need to give our machines an, extremely, good childhood, and. What does that mean well. This, has also been a theme that we've heard here today that, we need to be picky about the data that, we give our machines to learn from so, in my domain, we. Should show them only what we want them to learn in, my ptomaine we had these huge longitudinal, data sets of decision. That have been made in the administration, of government services. And. There's. An equally huge temptation to, pass those into a machine but. First of all we have to stand back and say. You. Know were. There some people that were poorly trained so, we've got some poor decisions and that set or worse. Where there was, their occasional, bias for prejudice being exercised in those decisions and the important thing there is that, a. Machine learning algorithm, will pick up occasional, bias and apply it systematically, what, the visiting chair of data mining from Eindhoven.
University Pointed. Out to us is that it, actually increases, the rate of biased decision-making increases. When it's fed to a machine and. Then finally what about less than optimal decisions, so. The example there is I may have a client I have the ideal service that I want to give that to that client but, it's either fully booked or it's, not available in the area so at the time or you have a record of isn't me giving them, not. The ideal service so do we want our machines to learn that so, really there, is this curation. That needs to go on. Bearing. In mind well these machines are capable of the. Other thing we need to do is socialize them, fully so, that means what, I'm talking about there is we, need to give them all the data they need to learn from and. The. Test there is are all the, factors, that are available for, a human, to consider when, making the decision and, they also available in data form for. The machine to replace them in making that decision and. Around the same time that we were discovering that, we possibly had a potentially. Fatal flaw in the design of one of our projects this, paper came out from the, National Bureau of Economic Research, in, the, US and they in, January this year and they basically had, an. Incredibly similar, but, better articulation, of the problem which is they. Wanted to apply again, machine learning. To replace human decisions they had a choice between. Judges. Deciding. To grant, bail or judges, deciding. To. Deciding. Sentences, and they. Chose to. Train the Machine on bail decisions, because everything, the judge takes into account in, granting bail so, whether. The perpetrator. Is about mr., court appearance before whether the perpetrator has ever skipped bail before whether, the, sorry, the severity of the offense it, all exists in the data. In. Contrast, with fencin sentencing, other things are taken into account for example. There. Are more of, the perpetrator, and often that is something that the judge reads through. Body. Language and. Other. Non. Data elements, so. There two things we can doing that in childhood, so in that learning, training.
Phase Now. What do we do when these psychopaths. Grow up so. When they're out in the wild, don't. Tell them where you live. And. What I mean by that is, wherever. Possible we. Should be withholding, and discriminating, characteristics, what I mean by that is like personal information, which we otherwise shouldn't, discriminate. On. We. Shouldn't be giving them to the machines and then we also need to test, as. We may say we've done that I don't have gender in there I don't have age in there I don't have ethnicity, but, it could be that they're still proxies that in, that data and, these. Academics. From across five universities, in the USA came. Up with a test. A machine learning algorithm, test, where. If they can predict. Based. On your data set which you think is clean of these personal characteristics, if they can then predict gender or predict your. Race then. It's. Failing the test, okay. And. Then. The, second thing is don't let them prey on the weak and what I mean by that is. You. Need to test your model accuracies on different, kinds of people, so because algorithm. Accuracy, is, only. Cares about the size of its mistakes every day about all of the training data um it, may have very different accuracies, on different groups and that's. Actually amplified, on minorities, because they're fewer of them within, the data set and so. It doesn't care as much if it's getting them wrong so. The example there is I may have 90% accuracy on men but only 50%, accuracy on women and with, these two methods. That I've just described there's a slight logistical, challenge which is on, the one hand we want to put our hands on our hearts and say we haven't used personal information, in, the running of these algorithms, but at the same time, we. Also need to retain that personal, information to, keep testing and making, sure that they aren't exhibiting. Discrimination, advice and we can do that and the, separation of principle, that has been developed in health research can. Allow us to do that so. In conclusion, we. Need to be careful about how we are raising and training these algorithms we, need to keep on testing their inputs and outputs and I. Think. An ultimate test of the ethics of the machine might be to say, you. Know would you trust your, children with the machine but moreover, now, that you can see that the structures of ethics come, from what people, put, in place around the machine the, question shouldn't should, instead be do. You trust your children with the makers of the algorithm. Thank. You. See. Yes, so to repeat the question the, question is how how, can you, be sure that you remove factors, that exhibit bias and in, particular for, example in, the legal examples I gave that, remorse and interpretation, and remorse, may contain, wise or even. Some of the factors that you took into account in. Granting bail so, previous, offenses, may contain bias I think, the, methodology, there's. Two separate things going on so, first of all really. You. Have I'm. Gonna put my legal, hat on because I'm a solicitor and say that it's, about removing. The. Elements, that we. Know by law you can't discriminate on as well. As what. Else has said which you said early today which is you then test for those to see if it's been in cox's in your data set but, insofar as what the judge was taking into account what, the judge is taking into account in either sentence, no bail I mean that's the legal system so, that's not. You. Know we're data scientists we can't solve everything, and. So in my example there I wasn't talking about. One. Set of, factors. For each of those use. Cases being biased or not it was just about the. Scenario that was best suited for training. A machine learning algorithm it was one where all the, factors that he used are actually in the data but, yeah beyond, what's.
Legally. Compliant and as, that, academic, pointed out like what is the definition of fairness that we can how can we teach machines to be fair if we can't even decide annotation of fairness like. Those big questions and, we should keep asking them. Okay. Next up we have Rebecca Cunningham. From the Institute for sustainable, futures, and. Rebecca's going to do something slightly different in, her slot, we're, actually going to watch, a recording, of something. She's prepared while, she wanders. Around the auditorium doing. Something mysterious. Transfers. You're again breathe the, water we drink but. Everything, in places to get by. The. Majority, of us are these informations. To make too many indents. And a checkbox, and. There. Are. Solutions. Operation, temperatures, being, mr., Davis are increasing, every long right we. Know that this woman in. Young region. Means not to talk about which is quite still arise. What's, I mean the country's, most exposed. Sea. Level rise is the density, including, million speakers and, this. Is energy breeze one of the three contacts, infected, thing, there. Never said it's being, powered budgets, the most and school, so Antonio is easy. But, all inside, wallets, information, is what, we had known before. Century. This. A. Million bucks a painting. Of a picture for, the future of that line. Are. Left, wondering birth. Units, as. Far. As its in 2014. I was like and. They, told me they, can't. Anymore. At. Least not the, way they used to these. Handed. Down from generation to, generation to, follow the seasons for planting, and harvesting. These. Songs don't, work anymore. What. Almost broke my heart was that one farmer, a leader of their community, spoke. About the cultural significance of, rice they. Need the, community, events but marriages. His. Mother had recently died and, the. Corporate veil and he. Had. He's. Felt. By everyone, in that room, climate. Change even, something that happens to other people in other places he's. Happening to us here and now. More. Recently, earlier. This year I, had the privilege to travel to care of us, remote, series of coral atolls in the Pacific, along. With another researcher from, the Institute, the sustainable, teachers we were mapping the knowledge networks around, water salinity and use Kiribati. Has one of the highest rates, of chances more ddb tjuta for drinking water in. Paragraphs, the land is too salty to grab food the, fish stocks are declining and, on, the outer islands, people are more likely to find out the water was bad once they were already said, although. The project was successful or needs to be done, 1930. Years old by 2100. But now people. Are dying sea, level rise is an everyday reality, and every. Morning we, had our company, anything, out on the sea that. Was literally about, doorstep it was a constant, reminder. For. These people climate change is an extract. Children. That I saw playing by the beach side near them their members that, can enhance their. Pain such a high price that our lack of action, and how. Is that ethical. Commenced. And whose in 90s. Provide. This quote from a meeting of the Institute, of the International, and European affairs. We. Knew everything we needed to know about climate change in regards to mitigation in 1990. 25. Years a quarter of a century of complete, and utter failure to. Address climate change and, I think we need to remind ourselves Jeff. That failure we have presided whatever, has actually, seen. Communication. Happened isn't, happening fast enough the. Two-degree cocktail, still exists and countries. Have agreed to attempt, to meet this target however. Our missions continue, to increase and, the, models of future climate changes, became four degrees of temperature rise it's. Now likely and, the. Impacts which. Are just, beyond it's devastating. So. What can we do long side, mitigation. Adaptation. Which. Is making changes, to the way we live which. Allows us to survive until starring, lands to effectively, reduce, and. In, local contextual. And happening. We can make changes now both in the country and in the city but. We've seen a few years I, have, tasted a local scale for example solar panel it is an adaptation in, both with the mitigating effect. Either. In Australia, and in New South Wales we are very conscious of heat this. Is a surface, temperature map of like up on, a hot summer day the, areas, that are perform deep, purple are urban heat islands some, of the cooler yellow shaded areas on the edges of coastal, but not all of them you, can see cooler, areas even within the built environment, in particular the, bottom right hand corner of the image is, the cooler suburb, in this local government authority, why. Because. It is retaining substantial. Levels of tree canopy cover, down. Temple but, why, aren't we doing it everywhere, the. Heat has an impact on human health and well-being but, also on infrastructure, and energy systems, as we, need to use energy to kill an environment, as.
A Society. We have the ability to design for anticipated, change this. Is, an example of a change model developed, for energy systems here in Sydney and. Attempts, to move away from business as usual by, following a series of transition pathways, to a design and desirable, future this. Is actually part of a broath stands for teaching planning expires in collaboration, with art theft ETF and the, New South Wales state government, during which the. Researchers. Have spoken to 1,500. Government, decision makers covering. At 91% of, the state level government narratives, it did that making, change local, context. And. That. Contribute. To medication, but also improves, health well-being and survival, of local communities. Understanding. The lived experience, of people allows, us to design conflate. To a transform, future. The. Traditional. Scientific. Academies. And seventh of nine knowledge, don't engage publics escape with. A society, and the scientific, society need to engage in conversation, now. More than ever we've got the tools to engage industries. And civil society, and transitioning, to adapt to climate change, companies. Such as the cross dependency, and a stable extract, any launched. Only last week here at ETS in the data arena and then working with state and local governments, research organizations. Like oxidizer, and. The industry, to collaboratively, adapt, our essential, infrastructure, to climate change bringing. To get a lifestyle. Lifestyle. By approaching. Like a petabyte of locally. Scale, climate, change data using. Immersive, technologies, to understand, Greece. Manchester. Manchester Museum. Incorporated. With the Tyndall Center for climate change. Scientists. On an exhibition called climate control it was open to all ages and, the exhibit in cash town is ridiculous, we, presented climate, sovereign artistic, forms such as performances. And films, working in particular Masai, preparing, for not a man exists from Australia, even, if completion, is between food of the future we. Also work with the Manchester City Council, and a climate change agent in order to engage civil, society, in imagining, a city three play layering, and each of these buildings were annotated. And offered to the City Council simple they're playing Georgie. Said and. 20. Frame buildings transport, education energy. Transport, systems, and even. The working environment. Yet. Here in sustainable. Futures we're working with creative studio instructions. A blue truck based in our exploring. Virtual reality, allowing. People to experience, environments. Like coral. Reefs adapting, in Belize. Allowing. People to interact with the science, that is involving. These transformation. In an immersive. Environment. Like never before. You. May have been wondering it's, all time well this is a TEDx talk so, where's. The speaker. Like. Billions around the planet without action on climate change. We. Have the tools it. Is up to after that. Hopefully. By now you. Will have in your hand a small pebble, and. I'll leave you with this quote I. Own. Cannot, change the world but. I can cast a stone across, the waters to create many, ripples.
Where's. Rebecca. Rebecca. Thank you very much that was fab um, so, my concern and I've been thinking about this a lot lately and I just wanted to put it out there is I think the problem for, all of us with climate change is there's a bunch of people who aren't doing their job that's. My expression. Of the problem I do what I have to do I get, paid to pay my taxes I vote I vote people I think should be doing. Things to. Fix. This and they're, not doing, their job. Look. I'm welcome, comments from, you and anyone else. This. Is written regards, to everything that needs to be done on the planet and should have started back in 1990, when we knew what we know now to fix this and no one's doing their. Job. The. Good news is things are happening, now just. Not. At the rate that they should have all quarter 4, yeah. Should have the. Political will hasn't been there because people were making money and the, system that we live in always prioritized, money, over people. And. I. Think now that we're getting to the point where in, the West we are experiencing. The very harsh, edge of climate change and it, is now affecting, our economic, systems, because. Of people rocking up to a. GM's. At banks. And insurance places. We're. Working with insurers, and, the finance sector. Because. These. Types of extreme, climate events. Cost. Their money and so they're worried about that now and they're, rolling out the xgi project is also because there's a they, have to put climate change in their business plan now I think. These are some letters that will finally, force businesses to act but. Because there was no political, incentive, or or stick. Unfortunately. There hasn't been enough but in the next few years that will simply have to be. Because. The the, economic system I survive I, guess. I guess what we all need to do then is say two things so to our banks things like I'm not banking with you if you don't have sustainable, practices, put, the put our money where our mouth is and and I have spoken to people from a bank and an insurance company, that said it is because activists. Rock up to our AGMs, and say why, are you paying your executives, this what are you doing about climate change what, are you doing about this what are you doing about climate change and asking that question every, single time and finally. Finally, there are there's, some forward. Motion. You're. Doing a good job thank. You. The. Center. For new, media studies. I got. That right no no media. Transition, thank, you Peter. For. The next few minutes I want you to trust me because I'm a journalist. So. The, power of journalism, rests in the in, the, gotcha moment, the. Gotcha moment, the gotcha moment when the person, is laid bare an institution, is laid bare here's a few recent, examples of gotcha moments, as the dual citizen. By ASCO is, the adding of sexual harassers and this, even the tax affairs of the Queen and Bono, who would have thought a name anyway. So my, first gotcha, moment. Came. When I was very young and, it, was the first time I understood the power of, journalism. It, happened when I was a primary school student and a, kid came up to me in the school room and said your mother is a thief and. Before, I could I sort of offer a boyish exploitive, he. Said she. Says, she is and he brandished, a copy of the local newspaper. In there, indeed was a story, about my mother who, had stolen a can of baked beans. It's. A sad story but I'm not telling this story to, say. Isn't it amazing that a son of a thief could rise to the professorial ranks at utsa, I'm, really talking about as I'm actually talking about why I think journalism, works, journalism.
Works Best when it on an emotional level. Well. It provokes. An emotional, experience and in, most cases unless it involves your mother and Kansa baked beans it. Works because it takes you outside of your own experience. It. Takes you to a place where you, probably haven't been to, an understanding. Of an event or a personal, experience in fact journalism. Works when it makes you feel and I apologize for those terrible errors but, you get the drift. Now. In, journalism, we are excuse. Um times this kind of gotcha moment, the emotional, in. The Academy, and the industry, we. Are probably, more likely to wish to talk about you. Know cultural issues, or if you like more intellectual, issues or even craft, issues before we talk about the, power of motion and, there. Is also a tendency. To see gotcha journalism as, a. Rather crass and unthinking. Part. A purview, of the tabloids and of. Course you, know despite the huge amount of thinking that it would have gone into that particular, headline, but, the sinking of the Belgrano. During. The Falklands War, it. Works because it, is unthinking. Because. It works because it makes you feel and it, is the power of emotion, and the, potential, of deep learning. Technologies. To facilitate and. Enable, human, emotional, responses, what I want to talk about briefly today and how. Those responses. Could, actually really be about the future of journalism in, how that very future, requires. Us to consider how the same machines, can, be utilized, to enhance trust, in, journalism, and at the same time that, requires us to increase, our efforts to develop, new, and transparent. Frameworks, for, that trust to be, distributed. We. Live in a very emotional times right. We feel faster, than we think we are receiving news and information, faster. Than we think and we are sharing that news and information often, just as fast often without reading. In. A way of as I've just explained that I hope that you know news has always been emotional, but now it is enriched, by technology. It's. All a bit scary. But there's nothing to run from is if we had the opportunity to run from it I think it's a great opportunity. Because. The unprecedented, disk, the upside of digital disruption is, that, it is gifted us the capacity, to connect. And engage with people and to, understand, what they want and who they are this.
Is What Facebook, knows about me there's, a smidgen, of what Facebook knows about me and it's, essentially, what Facebook uses that in its knowledge about me to sell to advertisers so, advertisers can advertise to me and if. You want to get your own version of that do, a quick search on. ProPublica. And black, box and, you can download a Chrome extension I. Think. The news media both legacy and emerging, can, do other of better things as the. Scholars, mark toyzzar and Charlie Beckett have suggested, a journalism. That links news to emotion, and that connects to people and, that, deploys positive, psychology. To, the sharing instincts, of audiences, could, actually have the makings of a sustainable, model and to, this I would very much add the dynamic that, comes with using. Deep learning, so. These I just want to call briefly about a couple projects, that, the Center for Media transition, is involved with with other, members of fans, as. Other members of affairs other members at UTS and an. Industry, and other. External parties, are first off I like to go to this guy, right. Now he's exhibit a and has. Certain people, you know invoke, an emotional, response. Which. Is of course his power and let's hope we'll also be his downfall. You. Simply can't be ambivalent, about this man and, of. Course as you know Trump. Delivered, initial, upswing. In the circulations, of quality, news media in the United States and that's because they have deeply. Engaged audiences, and they're able to engage more people who cared about the. What was going to happen or under Donald Trump but. What I'd like to see is how we can harness the. Emotional. Power of Trump to, assist people less engaged in the news to. Become even more so to become more so, so. I conceived, of, the. Trump Amina. And, I've enlisted a. PH, students, a PhD, student in in fate. His name is whelming Huang and the. Artists and cartoonists, and my dear friend ROC ofa's re and, this is what we've done up to now Rocco, has drawn a series, of generic, faces, this, is just a couple of them but, emotional, that. Each have a different emotion, and we thrown that into an AI and then. Rocco. Then. Humming, threw it into an AI then Rocco created, a series of Trump's and. He. Threw those into an AI and, then he. Then, we then, humming, asked him to create some more generic. Faces so chuck them into the AI and then. Hamming. Asked, the AI to. Produce its own trump and this is what the AI produced. Now. Well this is all great fun and also why on earth we're doing this right. So so. This is my initial idea can. We make it easy for journalists, to generate, Trump, hints by. Simply speaking at the machine and can. Each can, you use these Trump heads as a way, of engaging people. Who are not necessarily engaged, with the news with the news of the day, so. Ideally, a journalist, would go, three. Happy Trump's and that would be the day for instance that. You could that, Trump's tax bill passed Congress and then, you can say for, angry Trump's which would be the day that Jared Kushner is indicted, for, collusion. With the Russians. Now. So. This is simply a way that. This could be shared. Out. On social or shared, on individual, stories to try and engage people who. Are not necessarily engaged. With the news with, the politics of the United States and I, must confess we did first think about creating the Mallo meter but, then Morocco suggested, that we might run out of time. It. Might not be, so. Why. We want you to think of a way this, is a way of engaging the, emotions, of readers and perhaps. What our news organizations. That say about the Trump Amida to, its readers in terms of trust and transparency. So. I suggest, the media company needs to be upfront about. Two things two. Key words one is intention. Why are they making it and the other is accountability. Who. Is responsible, for it just. Leave those two thoughts with you the, next project. One. Of the kind of holy grails of machine learning is the idea of automated, fact checking and. I've had a very little bit to do with this and up on screen is the thing called claim Buster again you can download that and the. The. Idea of. That is that we. Can use natural language processing to. Find out the, machine can tell us essentially when a politician, is lying.
There's. A lot of problems with that because as we probably all immediately, guess that politicians. That. Machines don't really do very well with satire or nuance and such like but. It'd be an amazing, thing if you could just have automated, fact check links. But. Actually that's not this talk but if you if, you, would like to look up. Claim. Buster you. Will notice that I managed to persuade claim. Buster to put. In hand side, from. So this is a project. Based in University of Texas and what. Claim, Buster puts out everyday through. This NLP. It, recognizes. In, different, shades of blue the likelihood, of a politician, making, a factual statement as. I say this is not automated fact-checking. But. What if along the way we. Could harness similar, technologies. Similar. Deep learning things to the, cause of recognizing. And exposing. Commonly, deployed fallacies. So. The types of fallacies, that find, their ways into your news feed and and they're common, in news media and rich which are really their, building blocks of fake news and then. What if we could use that information to create a a tool that, would enable early, high school students, say to, develop, better critical, thinking skills so, that they could identify and. Avoid, unsound. Or an erroneous, reasoning. And that's, the goal of the straw man project, and I should very much like to pause at this point and recognize, that the. Straw man is primarily, the work of Gabrielle. Jakub who was a honor, student, in Fast and also the, to the wonderful, people are sitting in this room Simon, 9 and the wonderful speaker, next to come cursor kita now. These fine people are, hunting down the fallacies, and will drive the creation and testing of a. Fallacy. Busting, machine and as, I say my overarching, goal is to get the fallacy. Into. The New South Wales schools in the New South Wales border, studies has already, interested. But to do that I think. Again we must play with the emotions, of people and, I, want to create I think, we might need to gamify this, idea and we might need to create an avatar to enable, high school students to interact with it so, I think the straw man the, hay of the straw man should become a she. And we've, all heard of Khaleesi. The. Mother of dragons where, I'm keen, to think about felici. The Slayer of fallacies. Anyway. So, can we create a hero's. Journey towards, truth using, deep, learning I think we can there. Is much more to be done on both projects. But. They really gives us a chance to think about trust, and transparency. As. You probably know trusting, journalism, as a is at a record-low, in. This country and many others and if, getting it back is going to be a very, hard job, because. Especially among young people they can't recall the time when you, simply, trusted something because it, was the brand that you saw every morning on your, coffee table or whatever. But. I do think it's interesting that there, was a survey at last week that showed that people are more likely to, trust what. They see than. What they read so I think there's a capacity, to use deep learning again. To produce video. And, other forms of visuals that may enhance, trust especially among young people. About, five or six years ago I brought the fact-checking, service PolitiFact to Australia, and I still think and the idea was that could, put fact-checking, be used to, restore, the truth-telling, aspect, of journalism, and I still think that fact-checking, has a role to play but. I think the real problem with fact-checking, is that, it didn't actually answer. This emotional, quotient and. I think this was a missed opportunity, because. Even though we showed the workings behind our fact checks and we cited lots of references we, needed to be even more transparent about what we were doing and why we were doing it we need to be more responsive, to our readers needs and so, we could talk, really about our intention, and our accountability, and there's.
Plenty More things, to say about this whole thing but I think in the age of AI that. Is quite possible that we can when we build trust and we talk about responsibility. We, really need to talk about the role of the Machine and the, role of the human and, we should need to be talk about the the, who who does what when and why and who, takes the rap for, when things go wrong, thanks. For listening. Thank, you Peter though and of course, the point is that you can now grab these people and pin. Them to the floor of a coffee in, the, break that's coming up very shortly but. Kirsty Quito next, up. Kirsty is one of my colleagues and the connected intelligence centre who. Gets. And. Who understands, data and. I'm gonna do this in the educational, context, because well actually universities. They collect a lot of data. So. We've, been collecting data for four, years we've been collecting data for decades. Really, we've. Been, collecting data what all universities, collect data about students, satisfaction, we collect data breath grades, we, collect data about how. Students, traverse campus. Locations. We. Collect data about staff, what, staff are doing on campus lots, and lots of data but we've, always had. Very, isolated, siloed data so. If we've only really started as universities. They're really. Quite recent, history and what, universities, have started doing is they've started realizing, that actually. Date is really powerful we can do a lot with data, so. We can start helping students. To optimize, their learning, so. We. Work a lot in the field of learning analytics that kick and really. What we're doing with learning analytics is we're trying to use data to try and help students, to learn more effectively or. To help staff design. More. Cohesive, learning experiences, and to actually develop. Better teaching environments. So we can use data to really like change, the educational, landscape buy a better technology workout if the technology actually helps us at all. So. We're really starting to use data much more effectively. At universities. Than we ever used to and, there's, new data standards. Emerging, and new opportunities. To use data in education, so. Just. In the last three or four years we've actually seen two new data standards, emerge out of education, technology, X. A X API or experience, API and, aimers. Caliper and, these, are both new, data standards, that essentially, let us collect, data from ubiquitous. Mobile environments. So a lot of the educational, data that we've used to collect in the university, system has been very. It's. Been very isolated, and difficult. To join up and, very restricted, to for, example environments. Which are very managed, so for example data that's collected from a learning management system, and can't. Be maybe, using well the data, that's coming from our learning management system, about we, know that most of our students are all over the place they're using their mobile phones, they're. Using. Home. Computers, they're using a whole heap of quite wild environments, so, we've got new opportunities, emerging but, at the same time. There's. Challenges coming, as well, so. Right. At the time where universities, are working out that data. Is actually really valuable resource we, can do a lot with data we're, moving to cloud systems. And sometimes. We're losing the chance to actually control the data and to extract the data that we're fine so useful so we're making decisions in, multiple parts of the university, that are potentially, going to work, against each other and we need to start getting very strategic, about how we use data in the educational, system and all. Companies are facing these challenges it's not just universities. So. What I want to do today is. Think. A little bit about the questions, we're asking, in the university, context, because. There's a lot of talk about privacy. And. Privacy. Is important, privacy is very important, when it comes to Education data we do need to be very careful, with data and, we need to be very careful, that the right people have the data and that, the data is not misused, or abused or the wrong people don't get their hands on that data, but. There's other things we have to worry about as well and, I. Get worried sometimes that, we're not actually asking, the right questions, because. Actually what we tend to worry about is, privacy, and, we forget about things like access, and ownership, of data. So. Who. Gets access to. Educational. Data if. They do get access to it do they get it do. They even understand, it and, who. Owns that data where, does it sit and who's responsible for it and. I'd like to see us starting to shift our conversation. Along a little bit more into. Some of these more nuanced, questions, that we could be asking ourselves. So. One. Thing I'd like to point out here is this is actually a. During.
From A talk given by Audrey. Watters he's a big. Talker. In educational, technology, she, was asking questions about who owns educational, data at least five years ago according to this picture that I've got here, and. Yet the concept of data or ownership for the university system has not really managed, to transmit, into, a full-on. Conversation, so. Question. One who. Creates, educational. Data. And. Where. Are they creating, it. Students. Create educational, data. Staff. Members create educational, data. Where. Are they creating, it they're not often creating, it in our nicely controlled systems, so. I've had to change my picture because I've moved to UTS but, when I started doing this research I was, at QUT, and we. Had a beautiful new learning environment very, similar to the UTS beautiful. New learning environments, of e-learning. Services. Have turned on all of the technology, for a new star course we were running and my. Students, after I'd gotten them all into groups at the end of the first day of class. Signed. Over their Facebook accounts, to each other and. They weren't using the learning management system at, all. So, if you were, predicting. Maybe, student, success or trying. To develop ideas and models of what students were doing in your system and you were just using the learning management system data, then. You. Had very, bad data and, yet. We had an entire organizational. Unit that was trying to put in Student. Success off of the back of student, behavior in the learning management system, so. Who creates the data and where do they create it something, we need to be very aware of when we're thinking about learning and how we can actually optimize, learning or do it better but, who, gets access, to that educational, data is a question. We don't often ask. So. Is, it the strategic. Intelligence unit, at the organisation, our. Lecturers. Getting access to the data our, students getting access to the data that they themselves generated. And. This is an important question to be asking because quite. Often what we find is the people who can most understand, that data or the people who generated, that trace they. Understand, what they were doing when that trace was created. But, they can, potentially have a hard time to understand, the traces unless we treat them well and do a good job trying. To work out what in fact, is going on there. So. If. I, as. A lecturer, look, at this digital trace what. Do you think I can learn from that. So. The person, who. Was teaching the course could. Tell me a very good story about what was going on in that digital trace, she. Was using blogging. That's the mechanism for the students, to communicate, with each other when, do you think she hit the deadlines for the blog post. You. Can see it very clearly once you know what's going on until, you. Know what the pedagogy is behind that, data trace it's quite hard to interpret. But, the students, and the lecturers would understand, that trace very easily now this is a very easy digital, trace, to start interpreting but. Sometimes they can get much more complex. If. You get into things like topic analysis, then quite often the experts or the people who generated, the data can understand, those topics much more completely, than. Just. A straight computer scientist who's, maybe, running the models so the interpretation, of these digital traces is generally. Much easier to do when you're at the level of the person or the people called most closely associated with creating these digital traces, the. Thing is they can only do this when they have some training. It. Takes a lot to teach, students. And staff how to understand, data it's, a new way of thinking generally. And. You. Can get yourself into a whole heap of trouble if. You just give students, access, to data and, expect them to understand, what it's saying about themselves and. In fact they can misinterpret it, quite, profoundly. So. You, could end up for example if you have a student, who's from, a low socioeconomic, status. Sort, of first in family situation. Coming to university, and feeling like they don't belong. Deciding. That yes in fact they don't belong if, you tell them that they're at risk of failing because they haven't been showing up to their classes, so. With your analytics, and with your data you. Can generate a reality, which you're actually trying very, very hard to avoid if. You're not careful about what you do with your data, but. I would like to argue that we actually have a duty of care as a, caters to do this so. Just because it's difficult doesn't mean we shouldn't be doing it and the. Reason we need to be worrying about this is because, everyone. Is starting to form, this kind of analytics, on your data.
So. There's. A battle essentially. For control of data that's emerging, and, if. We send our students out into the world without. Teaching about, algorithms. And clustering and how different things are going to be used to analyze their digital traces we're. Leaving them grossly underprepared. For. Interacting, well in that world and we need to be very careful that we actually do provide them with the training they need. So. Here's, a couple of projects that we've, been working on it kick and. Different, other places over, the years so, one, way in which we try to generate an. Understanding. In our students, about the data traces, that they leave will. Be around, how, can I actually get data from all those ubiquitous, environments, where students learn how. Can I put, together how. Can I do some analytics on it and then give it back to those students, in ways that make sense so this is a project that was funded by the office of learning teaching. And, essentially what we do is we interface with social, media api's we store the day there in a standard format we do some analytics and then we give people back contextualized, and, contextualized. Analytics, that helps them to understand what they're doing but. There's a problem with that. If. You just go hey oh good a nice dashboard go and have a look at it things. Tend to go a little bit wrong. And. What you find is students. Don't tend to apply that knowledge back to themselves they, don't reflect on what it says about themselves, and they don't change their behavior patterns as a result and, it. Can be really hard to use so you need to be very careful, about the learning design and the scaffolding how, you link, those. Like, those, reports, and the analytics, to. The things you're trying to get students to do and that's, where analytics, really meets learning design and assessment, and the field has only. Really started to, acknowledge that really well that's becoming quite a theme in the field. Here's. Another thing that I'm thinking about lately which is sort of a scaling, up, of this first. Project I was talking about so. Personal. Data, educational. Data is, something that students will increasingly find, invaluable, when. They're trying to do things like claiming, competency. Returning. To university, when entire fields, of employment, dry up. Universities. Always have an enormous. Problem with recognition of prior learning and, we're, going to have a tsunami of people coming back to us who. Don't want to sit to an entire degree again, they. Want to claim just the extra bit of learning they need to go back out into the world again we, need to enable them to actually, show that they've got competency. And credit already that. They've gained in many, different places so. We're starting to think about well how could we develop architectures. That enable, students to aggregate, data from multiple places make, sense of it and then use it to claim recognition, conferencing, that's another project we're working on at the moment, so. Final, proper locations, because someone's about to hustle, me off the stage. Can. We create, a date data effect system if we're going to be ethical at UTS about how we treat data we need to be thinking about how we treat student, data how. Can we create like a data rate ecosystem. That enables, students to have. Control, and access and potentially, even ownership, of their educational, data I think. That's something we should be trying to do and. How. Can we convince. Employers, that. That's actually something very useful and, that. That data store the students, are harvesting, and collecting about their own competencies, integrants. Can. Actually then be used to claim that. Yes actually on expert in this thing and this is why you should be giving me a job when they go out into the world. Kirsti. Because, we're running a little overtime we're, just going to move, straight to our last presentation, last. But by no means least we've, got Joe, travaux here from t
2018-03-16 13:56