Evaluation of Advanced Vehicle and Communication Technologies through Traffic Microsimulation
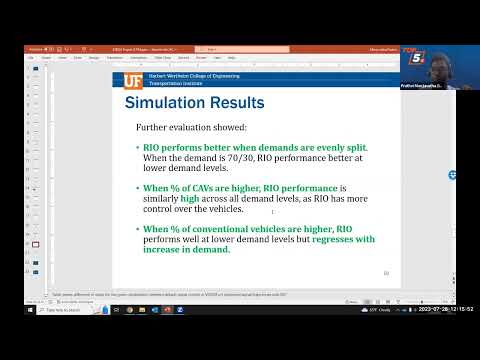
hello everyone this is prathway manjanata I am Pi for the project we are going to present today the topic today is evaluation of operational effects of connected and autonomous vehicles through micro simulation this stride project Builds on a previous tried project and I'm going to be talking about that and then after recap of that I'll introduce other topics this webinar in case you are new to this fried series of webinars is a part of the stride webinar series and stride is the usdot UTC for congestion mitigation which includes all the universities that you can see on your screen and University of Florida is the lead Institution for the Consortium for today's webinar this is going to be the flow as I mentioned I'm going to recap the previously developed simulation framework from the previous ride project and then the presentation is going to flow off with different upgrades so to speak we made with this framework first is going to be incorporation of signal and trajectory optimization that I am going to talk about and then Sheriff from Tennessee Tech is going to talk about vehicle Dynamics and then Dr Michael hunter from Georgia Tech is going to talk about incorporation of human-driven behavior of the human driven vehicle in the presence of Cavs or AVS um and and then we will go into the educational component with the results from the survey of practitioners and development of educational materials with Dr Hugo at Auburn with that so this is the UF team that that worked on the project thanks to um all the people who are part of this uh there were two students who graduated and became doctors after this project and with that this uh project Builds on an existing with Sims simulation framework that we developed a couple of years ago so when we started that work the idea was to evaluate with Sim capabilities and see how best we can model caves in a simulation framework using with Sim what we found was you know we can take uh take advantage of two functionalities that vcmop offers one is the com application programming interface and second is the external driver module the com API you know it is helpful to access all the network elements during the simulation whereas the external driver module it's very good in accurately implementing you know specified acceleration or or speeds for a given vehicle so with using a combination of the com API and the external driver model we came up with this framework for simulation where we said we capture all the traffic data using com API every simulations second or interval and and then we feed it into whatever the AV or CV logic one would like to run and then the acceleration for all the vehicles that are being controlled would be inputted to com API and then red into a text file where the EDM picks up the text file and implements that for those Vehicles every simulation time step so this is like the basic framework I forgot to mention in case anyone has any questions during the presentation please keep posting them on the Q a tab that you can see since we are four speakers we will try to dedicate 10 minutes for Q a at the end of the presentation and we'll try to answer them at the end so we started with this simulation framework and for this particular project that we are going to talk about today we had three different types of upgrades to this simulation framework when we completed this evaluation at the time we just used Navy model from the literature and then a CV model based on what PTV had and we and we ran this in conjunction with an emissions model that was developed based on the moves model by Georgia Tech so that was the previous project what we did in this project was we added the signal and trajectory optimization component we added the vehicle Dynamics component and we added the human behavior into this so first uh from UF I'm going to talk about how exactly we incorporated the signal and trajectory optimization into this framework so for this we picked up an optimization system that we are familiar with because we developed it here at uof so this is basically the signal on the trajectory optimization framework so there is a link to the published work if you are interested so essentially we developed this to be implemented in the field we have actually done some field testing with this framework as well the summary of this framework is basically there is the Sensor Fusion system that takes in all the information of vehicle arrival through different sensors once we have the vehicle arrival information we have something called as intersection control algorithm that will do the optimization and produce optimal signal plans as well as optimal trajectories so the optimal signal plan will be implemented at the signal controller and the optimal trajectories are the trajectory recommendations are sent to the Cavs so as you can see if we were to implement this in a simulation framework instead of the signal controller we would be sending this to uh the signal controller within the vision simulation framework and the optimum trajectories have to be implemented in vsim using the external driver module because of the reasons that I mentioned before so how would we go about you know implementing that so this is this is the this is how the modified or the updated simulation framework looks like so we still do use the com interface to get all the traffic information on the vehicle arrival information every time step into our optimization module there are two types of optimizations that are happening one is the signal optimization and there is a charge directory optimization so the signal optimization is sent back into the com interface and to be implemented using with some signal controllers and then for the trajectory optimization we write it onto a text file that gets picked up by the external driver module so what are some of the challenges so to begin with to make sure that this particular framework is working we we just used a hundred percent Cavs to test as a proof of concept we use the same four legged intersection that we used in the previous project instead on and we to keep it simple we just used a two phase signal plan and then we try to compare the performance in terms of delay with with Sims signal plan versus an optimized signal plan this is what we had with the 100 Cav and so we had six different scenarios with increasing demand levels first three are evenly split 50 50 and uh next three are 70 30 with 800 1600 and 2400 vehicles per hour as the vehicle Network demand so in terms of simulation result for every single scenario we had an improvement in delayed so delay reduced in each of the scenarios with at least 30 improvements and then we saw more Improvement when the demand was evenly split over a 70 30 split so we said and and some of the problems we encountered are like the simulations runs very slowly sometimes at or even slower than real time and and then you know the reason is the way in which we send the information of the vehicle accelerations from the optimization module to the same is through it writing on a text file so that was the bottleneck that we found so the next step was to now Implement different combinations of Cavs CVS and conventional Vehicles so what are the challenges that we would face so there are a couple of challenges one was that with Rio because it was using a an assumed car following model for the leader I believe it was a Gibbs for a car following model it had like a certainty of where the leader vehicle is going to be whereas in this framework as long as it's a conventional vehicle uh the vehicle type is defined as a conventional vehicle with Sim controls that and there's no such certainty and the second thing is within the optimization module when the vehicle gets closer to the stop line so the optimization and the iterations stop and the the recommended trajectories get locked in this works very well as long as the vehicle is a Cav and it will implement the recommended trajectory exactly as it was prescribed but it doesn't happen so with convention and vehicles that are controlled by the Sim so the solutions were to you know keep reiterate keep re-estimating the departure times for those vehicles and then adjusting this in the network State and also not locking the for the conventional Vehicles not locking them with certain and trajectory no matter how close they were to the stop line of course this this meant more calculations and and further slowing of the simulation speed so to test this out we came up with 11 different vehicle mixes with different percentage of caves connected vehicles and conventional Vehicles so 11 vehicle mixes and four demand levels with from 400 to 1600 vehicles per hour and then two demand mixes one even mix of 50 50 and then one at 70 30. so this resulted in 88 different combinations and each combination we ran it for five times uh for multiple runs and I have some results to show here due to lack of time I'll be only showing like one set of results but I will highlight uh different findings from in a particular set of results so one thing that you notice on this table this table gives the delay difference between a visim operated signal control versus real or the signal optimization software operated signal control when you consider the average improvements across all the scenarios all the 88 combinations that I mentioned they're all positive that's good news there might be a negative in in a few scenarios that is reflected in the in the minimum improvements that you can see on the left side on the First Column so some of that is because what happens is you know some conventional Vehicles when they get stuck in a simulation let's say visim has this 60 second rule where by default those Vehicles disappear whereas in the optimization it doesn't so this is this discrepancy that is happening which is you know causing some bottlenecks and delay time is not improving so what we did was we re-ran some of those scenarios with different random seeds so that the vehicles that are stuck which are not removed artificially like with Sim less those are not counted in general what you can see is you know all the scenarios that are at the top of your table they're all like Cav heavy scenarios so the Cav heavy scenarios have better performance compared to other scenarios in general some of the other findings that we found when we try to redo this table with certain type of Demands or certain type of vehicle mixes what we saw was in general like we saw with Cav only case uh the signal optimization software works better when domains are eventually split and also like we saw with higher percentage of saves Rio performing better across all demand levels irrespective of the demand with higher percentage of caves whereas when the conventional vehicles are higher the performance is only good at lower demand levels so a mix of higher percentage of conventional vehicles and also higher demand levels is not a good recipe to get good improvements with with at least the signal optimization that we used so the conclusions from uf's work is you know the initial implementation of the signal optimization module is working with with the uh the the modified or the updated framework is working and we were able to successfully run with different types of or combinations of vehicle types the way uh in which we ran this whole system is very modular so if someone wants to use their own signal optimization algorithm they can use our existing with our modified framework and then they can plug in their own signal optimization framework we did observe a slower and slower simulation speeds as the scenarios get more complex the main reason being our accelerations are being written onto a text file that has to be read by the external driver module so we did try to investigate with whether we can do it in better ways uh due to uh you know constraints of time we couldn't explore it further and Georgia Tech helped us as well we try to see if we can use other functionalities that with Sim allows to see if we can use those functionalities to transfer this information about the vehicle accelerations from the optimization module to the ADM in a better way some some more work can be done on on this area so so that you know the computation is not a problem and finally we only considered vehicle demand and you know so in the next iteration of this framework we can include a pedestrian demand as well with that that I'm already a minute over my time so I will hand it over to Dr Sharif gavish from Tennessee Tech to talk about incorporation of vehicle Dynamics to the framework so hello everyone thank you Cody for this quick intro and this part of the project actually I'm going to discuss the incorporation of advanced vehicle Dynamics modeling for connected and autonomous vehicles with the micro simulation environment this part as food we mentioned was conducted by Tennessee Tech University under the East provision of Dr Armand and Dr Shireen the main the task mainly actually this task mainly actually focuses on enhancing the micro simulation performance by accounting for the vehicle Dynamics which will provide more accurate individual vehicle performance that can be incorporated with the micro simulation environment actually several parameters have been accounted for in this simulation modeling including driver characteristics the vehicle body for different cars and trucks as well as the power terrain operation environment as well as the operational modes the vehicle Dynamic model will provide distributions actually or like it will provide at the end the distributions and the performance distributions and curves for maximum acceleration maximum deceleration safe distance Gap as well as minimum safe time gaps that could be used within the micro simulation environment of this the main or life to this end Ransom or rank some rexim which is the Microsoft error like the vehicle Dynamic simulation tool used was used actually to model and simulate the vital dynamics of 10 different uh passenger vehicles as well as four different trucks uh it accounted for as I said different operational modes several drivers characteristics the vehicle power trails used in this simulation or within this simulation tool were derived from this provided website which is automobile slash catalog and the vehicle Dynamics have been tested using the US Open sex supplemented Federal test procedures for passenger cars as well as the cycle leave for trucks which are these two common or like well-known models for the performance for passenger cores as well as trucks this table over here it shows actually the different driver Behavior utilized within this simulation or like the vehicle simulation environment as well the simulated vehicles are presented here these are like the different 10 Vehicles as well as the four trucks in which the parameters used for the simulation where the width height weight the wheel radius drag coefficients uh initial and maximum engine speed as well as the provided down here so all of these were actually simulated within the rank Sim simulation vehicle simulation the dynamic environment and they obtained the actual like these different curves pinned from the previous mentioned website were factored in to effectively simulate these different vehicle vehicles or like the 10 Vehicles as well as the four trucks for sure validation and calibration for the developed vehicle Dynamic simulation were conducted in which the truck Sim and car Sim were used to validate the performance of the simulated Vehicles using the acceleration distance in which like replication we made sure that the values were replicated from the different traction and Carson modules in which several assumptions were utilized as provided here in this list in which we tested them also in different grades within the roadway profiles these are like some general conclusions and results obtained from the conducted vehicle simulation models in which like it was found that the maximum acceleration adjusted to the vehicle model and vehicle classification also the vehicle model vehicle classification and speed would affect the maximum deceleration and the minimum safe Gap distance is sensitive to the driving mode different driving modes and the stable table down here provide like the different ranges that were obtained actually for the different parameter investigated four parameters the maximum acceleration deceleration safe distance Gap C distance Gap and save time gap for the different parameters like the different truck types car types different tested speeds as well as the driver characteristics and the different operated operational modes so this like would conclude generally the different distributions and the ranges that can be utilized within the micro simulation investment can be emptied to this micro simulation environments in order to simulate the different Vehicles accounting for the vehicle Dynamics so by this this would conclude this uh vehicle Dynamics port and I would hand it again to broadly thank you so much Sheriff so um those were the first two uh uh changes that we did to the framework um so the first is the signal and trajectory optimization and then the vehicle Dynamics uh the third one was uh to model human driven vehicle behavior in presence of caves so for that we have our doctors Michael hunter from an and angstrom and gain from Georgia Tech um all right thank you so yeah so so next we're going to talk a little bit about um considering the human driven vehicle side of the AV equation and the folks working on this project is myself and Dr gwyn's also on the call and jamesabay who was the student working on it has graduated so he successfully often some great things in Industry right now so just sort of a very quick background and I'm not going to get into all the different pieces of the literature but what you'll see is when you look at a lot of the AV modeling out there today the two most common customizations tend to be that you know the AVS are seem to be uh very cooperative and they tend to have a conservative Behavior but also that the human driven vehicles are usually assumed to be very Cooperative or that they'll Retreat a AV as if it's another you've been driven vehicle and that may not be true I think there's some some inclinations out in the literature we can look at maybe the way folks have been treating some of the mobility service Vehicles some of the test beds that have been going on with AVS there's a potential here that there there's going to be some aggressive behavior towards AV so that a driver in one vehicle May treat a car that's being driven by a person differently than it would treat another car that's being controlled through an autonomous as a final vehicle so we want to sort of try to explore some of that this builds off of this builds off of some initial efforts we had done using Sumo where we were looking at an off-ramp in that case and just trying to see what would happen if I had these aggressive vehicles uh in in the fleet and in that case in the this version as well they'll talk about here in a second we really break it up to three different vehicle types one is the autonomous vehicle which is assumed to be a cooperative and not a human driven vehicle which is also generally assumed to be Co-op and then an aggressive human driven vehicle so we break the human vehicles into two categories one more of a Cooperative that's the general assumption the most research today and the other is a more aggressive and in our version of aggressive we're doing two things one we have them Target the AVS so the aggressive human vehicle when it's trying to uh merge it will go as far down the main line as it can and to essentially cut off an AV and get its maximum advantage and then the other aggressive part is when it cuts off it will use very very small gaps and actually in the Vista inversion which we'll talk about it will use basically no Gap it'll just force its way right in front of a an AV and four set AP to hard brake if it needs to so that's what we're really trying to try to look at that impact when we did this for the Sumo off-ramp version we were able to show that we could do it I'm just going to cut it basically what you really take from this is this idea that this is a time space diagram trajectory that the aggressive vehicle gains quite a bit of Advantage right it's able to pass a lot of vehicles that are essentially exiting nicely on the off-ramp move way out right to the to the tip of the door and get itself off overall on the off ramp when you look at averages things don't seem to change almost all that much but what really changes is who's receiving the benefit who not so travel test versus a very bimodal with the aggressive Vehicles picking up right really reducing their travel time times because the penalty being paid by the the AVS and the and the just the regular human driven Vehicles because they're caught in that same traffic Stream So if they're caught behind a aggressive uh if they're caught behind an AV then it's being cut off well they're going to pay the same penalty so we decided to flip this around and look at okay so what would happen uh maybe some capacity impacts looking at an on-ramp situation so now we're going to have an auditoring and we're going to look at sort of a worse case we're really trying to kind of break out the parameters and kind of understand what the variables are so we look at a single Lane single Lane made line and also a single Lane ramp and then we're going to have the ramp essentially merge in we are going to do two sets of experiments here the first experiment assumes that there's no meter on the ramp the second experiment we're going to put in meter present to essentially sort of mitigate see if we can mitigate some of the effects we saw early on and the experiments are going to be very directed at capacity so so we're putting way more Demand on this thing uh the demand coming from both the ramp and on the main line then we can know it handles because what we want to see is how much traffic can you push through the ramp Junction uh to do this as I mentioned again now we're in the vissim world now so we're on the platform that we've been talking about yeah we have an algorithm to make this work we've got our a B's HCBS and ahdvs and the AVS and hdvs are generally similar in terms of how we're modeling them or their Cooperative AV ahdvs right we're not using any Cooperative behavior and miss them and we're targeting so essentially what's going to happen here is an hdv will seek to maximize its Advantage because it's far down the ramp as it can and then cut off in AV this takes as proof that you already mentioned there's a few components here that we have to work with we're doing the component object modeling external driver model which would prove sheet mentions you got to use the com the com because it's basically capable of looking at all the attributes you can get vehicle speed lane position type such as the objects of the mode of your system simulation your component object model although it does have limitations it can read most objects but it cannot write to most objects and the limitations are greater when you're in runtime for instance we can't force emerge say right now change lanes to do that you have to use your external driver model which as you've seen in the driver module is very much about having uh this is what people typically if you want to do your own custom car following model you would use an external driver model we've also added in a user-defined attribute user defined attribute is kind of a newer Vista feature that allows you to create your own attribute which we do we use it as a flag so we use com to do all the targeting once the targeting is all set uh and it's time to merge then we change that user defined attribute that will turn a flag and then EDM say oh my turn and EDM will force to merge the thing behind all this that is somewhat important is that you can't do this by just changing the vehicle parameters I know for instance if this if you know has a has an AV that only works if you're staying if you're living within the basic underlying car falling model you want to just calibrate it here we're really trying to change the behavior we're saying we want to Target you do that you start getting into all these things so like we said there's two basic scenarios in one scenario all the ram vehicles are human driven Cooperative the other scenario they're all aggressive not very cooperative and what you're going to see when you get into that is that you have aggressive vehicles and when there's no AVS the main line will keep running just fine it's this is this yellow line it's a total vehicles or through the junction but once I even have just two percent AV just a few AVS what will happen is this gray line here is the ramp and the ramp basically takes over the instead of the main line getting much traffic through the ramp basically gets all this traffic through because I have these aggressive AVS aggressive uh human driven vehicles and they're cutting off the AVS and even if there's only a couple percent they essentially just toward these giant platoons of aggressive vehicles and they the same AV might be cut off by a hundred aggressive Eagles just go in and going and going in now we know we've kind of built this to be sort of a worst case but it's this idea that you kind of get stuck that that AV basically kind of gets stuck and they just keep getting cut off and if I move this traffic where it's not aggressive vehicles in the ramp basically as we're modeling and most of the work today then you'll see that doesn't happen and that there's more of a balance down here and that that the main line still keeps its traffic moving and the ramp is putting some on as well but there's more of a balance but if people get aggressive so here's sort of another way to look at this solid is aggressive the dotted is not aggressive the aggressive does increase overall Junction capacity so that's what this this is your overall Junction capacity which kind of makes sense because the aggressive are basically creating some so much smaller gaps so you're going to get a little more traffic in but what you're going to see is solid line aggressive dotted line not aggressive on the main line that even a very very low percentage that main line is going to get pushed way down and the ramp is going to push a lot more so this is the ramp traffic I go from no wave East I don't get much RAM traffic onto just two percent I get a ton more traffic on from the ramp but I've essentially shut down the main line which is this solid line that the main line does not able to process more traffic but if I look at the ramp again I can push ramp vehicles through when they're not aggressive I'll actually get quite a few quite a few fewer vehicles on the ram so same deal you're going to see this endless trade-off uh part of that like I mentioned is we also counted like how many vehicles were cut in front of a single uh AV so when there's only two percent AVS out there cars regressive get a lot of cars just trying to cut in front of it or when they're not you're going to start to see some balance and that's going to balance out too as you get more and more AVS because it's essentially more and more targets to choose from uh so what we did do then in the end was we added a ramp meter just to see and I'm going to very quickly do that you'll see when you put the rent meter in the the total ramp Junctions to be saying that's a great meter buys you a little bit but the big difference is so this is the aggressive vehicles on the ramp where before without the rent meter they just took over and they shut down the main line now what happens is you're limiting the number of vehicles can get on that ramp so you're going to limit the number of vehicles that are able to cut off the AVS and you can control that and so now the main line this is going to flip it the main line actually will keep moving so and you can now reduce so where we had a no ramp meter your main line I'm getting shut down with a red meter you can now sort of Start Control those trade-offs and help the capacity as well we'll skip that I know I run a little logs I'm not going to get too much into this you really got to do this stuff with EDM and com so we actually have created some documents for instance we have a nice uh document that we're just wrapping up right now on how you would actually do something like this in EDM it's a little tutorial so if you want to do this kind of stuff it's very helpful that first time you do it's very painful so this sort of walks you through your initial application actually the biggest hang up on all this is you've got to have somebody know C plus plus and they're becoming fewer and far between we're not going to dig too much into that moment so what kind of walks away from this is it is really important that we start doing some of this kind of thing because if we have aggressive drivers taking advantage of ABS but the aggressive driving really does have a potential significantly impact both capacities and travel times but we're not capturing if we don't reflect the potential those kind of drivers and the impacts are not experienced equal right so so some vehicle types are really going to suffer where others don't so you might look at average you'd say oh well it's not that different it might be significantly different to depending on where somebody is in their experience AVS may be essentially getting shut down on the road because they're being bullied because they're being targeted so future impact we consideration we need to start realizing we might have to start putting out some mitigations like rent meters and other things simply to help control that to act as gates to avoid that and also when you're doing some of this modeling outside the box like the platform we've been talking about you're not going to get away with just changing parameters in in your wiedemann models you're going to have to look at how it handles to come look at how I handled CDM Alexa we also use the UDA which was which was kind of a creative way to sort of pass between the two of them this part of the the task this task six Urban and the Tennessee Tech has been working on tasks to develop a theory education material for transportation engineering course many for the civil engineering students this is what I'm going to present the results from here is we we conducted a national survey uh try to identify the critical clb topic for civil engineering curriculum those are the seven these transportation engineering course offered by I don't know many universities but this is what we offered here the over introduction to transportation engineering Transportation programming modeling High redesign Transportation safety system and traffic engineering traffic simulation and its so the through this class we like to add some lecture about cre to each of this class so that that is a purpose so so this material can be used by other professors at other universities so you don't have to spend similar amount of time by each faculty to develop a similar material so this is a series we conduct for the national survey we did the online National survey among Transportation educator and and also practitioners we received 48 response that's a result from a representative from 30 States 33 States uh include some faculty already uh integrated uh CRV in the class teaching and also some practitioner already hold some the workshop on the cre so actually we put these two group of response give them a little higher weight when we rank the topic so this is a serving results first we uh we identified a list of the potential topic put in the survey those potential topic is based on a literature review this compiled uh based on previous CRV study or some existing CV course already offered by different University and workshops so the survey was designed for actually three groups so if they are uh they say well the answer is transportation engineering educator already incorporate the CV in into that course they will be in a group of one so they will answer the question designed for them will be a slightly different than other group so we received the 20 responses from this group and the second group are the transported engineer Educators who have not yet included any saving material in their course I don't know the what what happened in your University but Auburn here I I we have not included any Cav Topic in those transportation engineering course so uh that's it we've got a 16 response for the group of Two and a group of three we received the 12 response for their practitioner some day dot and some consulting firm they are they they already uh conduct some Workshop related to Siri so so we'll ask them to rank the topic for example this is example rank in theory topic for the course name for example introduction to transportation engineering course what what kind of the uh topic you think it was about Coe we should cover in this kind of intro class so so this is an example question what are the Cav level automation Civic control concept registration overview clb pilot deployment and that's that's each group is in each group they give a ranking for them and we give a weight for each group then we got the weighted average and based on weight average we can rank the topic for for the class one two three for example uh for the course this intro class we select uh top three ranking the topic to include in uh in in that class So eventually we are based on the severe results we identify those topics for each of this class for the urban University team we developed the material for first four class Tennessee Tech developer for the traffic engineering traffic simulation and yes cause so that those are the topics for each different class the format for the eventually for the course material has been developed for each transportation engineering course uh we include uh the material for one lecture is about 60 to 70 minutes class around the 35 40 the PowerPoint slides each slides content you have a you have a student notes you have a instructor notes and all the reference so and also we developed a class assignment example question and those class I brief up this project finish we can upload the course material to an online linked website and you can anybody want to use that can can download for to customize for your teaching purpose so that's my presentation I'm going to turn over to Dr Sharif from University tennis from Tennessee Tech to share his part of three course material he developed again as Dr said this was like collaboration between the two universities and my part actually When developing the connected autonomous vehicle education materials was mainly so it was mainly focusing on developing course materials for these three courses traffic engineering analysis as well as its and traffic simulation as Dr Hugo mentioned again in this task civil transportation engineering educational materials accounting for the effect of connected and autonomous vehicle Technologies on different Transportation Fields were developed and the focus again was to develop and provide the appropriate knowledge for engineering students about connected autonomous vehicles and their influence on the different Transportation Fields actually as he also mentioned this would help in supporting the Kev testing and deployment enhance the update current transportation engineering curricula key Pace with the evolving Automotive Technologies and provide the knowledge for engineering students and the main deliverables as usual it's gonna be like course notes handouts assignments project courses and so on the main sources and differences used in this airport actually were extracted from the Federal Highway Administration Publications the Society of Automotive Engineers Publications as well as reference manuals at the highway capacity manual manual learn from traffic control devices as well as published research manuscripts first for the traffic engineering analysis several aspects have been covered for this specific course including the Kev impact on infrastructure traffic flow and vulnerable Road users the course also presents what are the different limitations associated associated or associated with the care of deployment and testing as well as what are the current practices for caref Technologies and what are the current regulations and recommendations and specifications out there in manuals or result of several task force committees as well for the again for the traffic engineering analysis course or the infrastructure marking signs and signals were investigating the effect of Kev on these different track and control devices were highlighted um in which the CAF Technologies uh were investigated or like provide information how they can visualize the surrounding environment to operate safely and efficiently what are the different challenges with the chaos Technologies for example the low uh sun or like layer or maybe mental ass or non-uniformity of the different marketing signs provided across the U.S what about how they are going to perform in adverse weather conditions several challenges like this would highlighted as well as different recommendations provided for example from the National Committee on traffic control devices in which they have like this specific task force to investigate what kind of limiting or what kind of enhancement that needs to be provided for the current uh traffic control devices moving to the traffic flow what is the effect of connected autonomous vehicles on calculating the capacity of our roadways on how to calculate the level of service and so on the latest Highway capacity manual actually highlights specific sections within the whole manual actually on how to account for the presence of connected autonomous vehicles having these new tables for the adjustment factors of the calves and finally within this course I discussed
2023-10-02 08:29