Clinical evidence for technological innovations: Alternative study designs
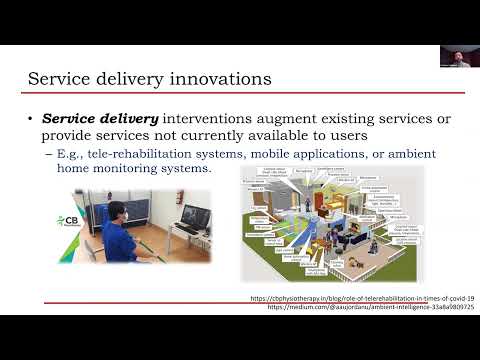
okay um so with all those housekeeping notes out of the way uh thanks for joining us again today um we're excited to to kick off our this is our last session in nl supports quantitative research design uh series so we have with us dr steven charnock he's an associate professor of biomedical engineering and he's jointly appointed to the faculty of engineering and applied science as well as the faculty of medicine at memorial university i hope i got that right um so dr chiarok i will pass it along to you to get started on your very interesting presentation wonderful thank you very much julia and thanks to holly for uh inviting me here today i hope everybody can hear me um i'm not up in my office as normal because i have a couple things going on in my home right now uh so i apologize i only have one screen which means it's going to be challenging for me to directly see the chat i have no problem with anybody jumping in if you have any questions though um or throwing something in the chat maybe julia if you're uh able to keep an eye on it maybe you can help if something is is critical or needs to be said right away i was going to say doctor i'll monitor the chat you focus on your presentation yeah absolutely thanks very much okay so as julia said i am uh stephen charnock i'm associate professor joint appointed to both the faculty of engineering uh in the faculty of medicine and engineering i am a computer engineer i also have an appointment to the discipline of emergency medicine and i'd like to say right off the bat happy tuesday when my kids pointed out to me today that uh the date is uh two two two uh two two and it's a tuesday so that's kind of an exciting thing for me uh really quick background about me um hold on it's nice screening yeah a little bit of quick background on me i'm a biomedical engineer i worked for 10 years in industry before i went back to do my phd in biomedical engineering at the university of toronto um what that really means for me is that i apply a lot of computer engineering or sort of technical uh research and design principles to healthcare applications that's sort of how i view it and this talk is really supposed to hopefully support some of the other talks you've seen which are ideally presenting some more traditional research designs the idea behind this is that if you are a technology or an innovation uh researcher or designer you may find that some of the gold standard approaches to collecting evidence to support your work in your research and your development may not actually be effective or appropriate so we'll talk uh especially toward the second half of the um of the presentation on what i mean by that and what alternative study designs exist out there for people who are are maybe looking at how an rct for example a randomized controlled trial is not appropriate for their research uh before that though i'll quickly talk a little bit about technology and healthcare and what that means talking about sort of current and future trends just to set the stage and introduce the idea of disruptive innovations uh which will set the stage for a brief discussion on current states of evidence in healthcare and particular randomized controlled trials and what the challenge is with implementing some of those uh study designs are with disruptive innovations and of course uh closing it out with a discussion specifically about research designs that are appropriate for those types of innovations um so this is something that you'll probably see a lot in the news these types of of uh publications are pretty common looking at really exciting healthcare technologies that every executive should be excited or new things in the future uh in my experience most of them are artificial intelligence based that seems to be a very common trend right now in research and in development so you see lots of buzzwords around things like artificial intelligence and blocked blockchain automated voice recognition voice searching chat bots virtual reality uh harvesting data deep learning um you know through everything like social media and through your email habits and things like that uh personalized and intelligent mobile apps all these kinds of things really uh to me it's it's just a sort of snapshot of how the world is viewing technology lots of exciting things out there but one of the things that i find is really interesting is that as much as um if you do a quick search on you know advanced healthcare technologies that are emerging or coming out um you you'll probably find that not not a lot of them are translating into actual practice so you know you see these images here of sort of intelligent user interfaces for surgeons or you know people using virtual reality and augmented reality to do things um but something seems to be happening year after year that are preventing these technologies from actually translating into uh health and healthcare applications and i think that this is common across many healthcare fields uh lots of people are coming up with new outcomes and new design targets and new features it's very very hard to change the current paradigm of healthcare technology is not immune to this but i think that there are some systemic issues specific to technology developers and technology researchers and in specific related to study designs that we're going to talk about today so when we think about technology-based interventions in this context i'm really speaking about technologies very very broadly technologies can be the most state-of-the-art artificial intelligence everything from absolutely you know intelligent environments that understand what's happening within them uh and again remember that these are are often in a bit of a dream world or or not necessarily in a real applied world but even conceptually these high-level highly intelligent solutions and interventions but also can go you know can go as simple as things like pill boxes and reminders and and you know environmental modifications uh you know being able to to accommodate different forms of disability so lots of frameworks exist um in in canada we have a we've adopted an iso standard uh that's that's generally accepted worldwide which is a relatively comprehensive framework looking at assistive and therapeutic interventions and so here we're getting a little bit more nuanced in terms of of how we frame the idea of a technological uh intervention specifically looking at things to help accommodate some sort of disability uh whether it be a an acute disability or a short-term impact of trauma or whether it's a more long-term chronic disability and within this uh within this taxonomy or this sort of classification interventions and specifically healthcare interventions are broken down into three categories either assistive therapeutic or service delivery augmentation i'm going to very briefly spend some time uh discussing what these mean because i think it's interesting to think about the fact that uh we're taking the issues uh associated with healthcare and kind of breaking them down into different sort of perspectives on how we view the end users and one of the things that i'd really like to emphasize as a latent theme uh underpinning this this entire discussion here um is is that the idea of disability is often much more complicated than traditional study designs in my opinion anyways uh than traditional study that designs accommodate for so the idea of disability can be viewed in terms of sort of a functional or performance based impact on a person's life so assistive interventions are specifically designed to support some sort of functional activity or some sort of functional uh performance deficit so a prime example would be a traditional wheelchair or memory aids like you see in the middle image but can be much more sophisticated in terms of the wheelchair on the left here uh which is a more intelligent automated wheelchair that has some capacity to be able to independently navigate and interact with the user so you can see that this is not just uh something like an intelligent wheelchair chair is not just tackling issues related to physical um disability but also perhaps cognitive disability or or you know some more nuanced social interaction components associated with disability um the the device on the right is a commercially available memory aid which is not just using a smarting but also in some ways passively interacting with the environment and understanding the appropriateness of timing and the appropriateness of inter interacting with the user so for example if a person is watching a tv show and the medication or you know something you're trying to prompt them to remember um is not critical to be happening at that particular time then these intelligent devices can understand a little bit more about the context of what's happening around them and perhaps for example delay a reminder um therapeutic uh interventions or therapeutic innovations i often in this lecture or in this discussion are going to be i'm going to be interchanging innovations and interventions because i'm speaking specifically about these you know modern technological innovations that are healthcare interventions so from a therapeutic perspective these technologies can really be focusing on on not so much a functional loss but on on restoring or improving um a loss of functionality that is is perceived to be able to come back so this is sort of the idea behind therapy um on the left side you'll see what what is a robotic exoskeleton that actually has the ability to offer propulsion for uh gate support and loss of lower limb control helps support balance and and can support things like falling so in this case for example somebody who has lost uh entire lower limb functionality but still has neurological control over those limbs uh in some measurable way can actually use something like these exoskeletons to be able to move around um virtual reality in the in the middle image here has been used for all kinds of things particularly within cognitive domains so you see the image on the right overcoming phobias of spiders or uh you know flying or feeling as though you're being you know uh i can't think of what the word is but trapped within some sort of space uh or even you know traditional therapies associated with ptsd or relationship disorders uh alcohol abuse um all kinds of really interesting uses of these technologies for therapeutic inventions uh interventions but as i've said sort of earlier on and i'll continue to reinforce we don't really see a lot of these entering mainstream um mainstream healthcare uh practice and so again i think that one of the big issues with this is is the way that we evaluate uh and obtain clinical evidence to support their effectiveness or their efficacy as we'll talk about shortly uh and then also some of these interventions these technological innovations that we see really becoming mainstream specifically in the research world not but also in terms of some smaller startups are are interventions that are targeting service delivery so in this case we're not even necessarily looking at the patient in terms of a disability however we want to classify or qualify or quantify disability or a therapy in terms of restoring some sort of loss functionality but specifically looking at the process of service delivery so tele rehabilitation is probably the most publicly uh publicly well-known and sort of successful technological innovation that affects service delivery in this case you're using some sort of technology for telecommunication to support either rehabilitation or patient doctor connections supporting rural and remote locations television rehabilitation has really uh in many ways almost crossed the boundary but i think that i would argue in many cases we haven't seen that also translating into more modern forms of healthcare delivery although interestingly as i'll probably mention a couple times throughout this webinar um the introduction of the global pandemic has really seen uh an interesting pressure put on the use of of technology and i think this is a prime example we're using a virtual a virtual meeting room to be able to share information uh anybody who's tried to reach out to a family doctor if you're fortunate enough to have a family doctor you've possibly met your family doctor by phone or if you've had some sort of physical condition like a skin condition you've maybe had the opportunity to snap some photos and upload them so that your family doctor or specialist can see them so you know we are seeing some penetration of these technologies into mainstream you know health and health care but you know other things are mobile applications or ambient home monitoring systems this image on the right is intentionally cluttered and it's showing the idea of how advanced some of these technologies can be in this case we can see what would be some sort of smart environment that is instrumented with all kinds of different forms of technology um and user interaction capabilities so whether microphones or water sensors uh present sensors in showers and surveillance cameras but home control systems that are not only interacting um actively and passively with the inhabitants of the room who might have the disability or the whether cognitive or physical or social or otherwise but also the caregivers and the users and uh you know broader implications to connecting with uh health care service providers as well um so you know obviously i could spend you know quite a bit of time talking about lots and lots of modern interventions that are under different forms of research and development um but the idea of translating some of these really really cool uh in my opinion anyways really really cool uh interventions and innovations to you know more mainstream healthcare are really challenging these interventions can be extremely complex both in terms of sort of a more pragmatic perspective on how if you think about this intelligent environment somebody has to install and maintain that environment and also complex in terms of the technology itself technology tends to have a bit of a stigma you have to come from a sort of science technology engineering or math background to be able to consider yourself a tech person um i do a lot of work with healthcare providers who are adamantly clear even though they might be more technologically advanced than even here some of my graduate students that they're not tech people so there are interesting boundaries between uh the translation of technology to market um but more specifically a lot of these interventions are very nuanced and customized to the to the users that they're intended for um so by nature of targeting diverse users that brings into question the relevance or the applicability of some of our um methods like i'll talk about in the next couple slides like randomized control trials where we're looking for relatively strong control over the populations where we're doing research on we want very homogeneous populations and you know in a very pure sense a lot of the experimental study designs that i'm i'm presuming have been covered in different venues although like i said i will briefly go over them these diverse uh these diverse experimental study designs that we can rely on whether they be experimental or observational in nature are ultimately trying to reduce the uh population that we're doing research on into a very homogeneous uniform uh group that we can look at and and you know literally attempt to only modify one single variable which would be the intervention uh so that we can do a strong statistical approach to measuring change but you know users in healthcare applications are are not homogeneous they're very heterogeneous heterogeneous and diverse um and as are the environments that they're living in and you know operating in so these innovations really uh are struggling to strive and are struggling to survive and thrive uh in these environments where rigorous control is being imposed on on the generation of research evidence um also from a societal perspective uh whether it be from the users of these technologies or the clinicians who are are using them or the caregivers or other stakeholders who are supporting them there's often resistance to new technologies of various forms and we're going to stay a little bit away from that i think we could do multiple lectures on on why technologies are resisted in terms of acceptance and adoption but mostly what i would really like to focus on is how we can translate these technologies from these early developmental stages into later stage sort of clinical applications through the acquisition of rigorous evidence and i think that the the concept of rigorous evidence is what i'm really calling into question here what rigorous evidence means and particularly in relation to study designs uh in clinical applications because the truth is if we're going to see something translate into clinical application we have to be very sure that we do have appropriate and rigorous clinical evidence because you know at the end of the day lives may be on the line so these technologies these innovations uh a term emerged uh quite a while ago probably in the early uh uh two thousand 2000 circa 2005 2010 this idea of a disruptive innovation um and the idea of disruption is really that this idea that we have current paradigms that are in operation and they tend to be well uh established so we see that for example in gender studies and we see that in race and ethnicity studies as well it's very very hard to disrupt the the dominant paradigm and the same is uh true for healthcare applications and the same is also true for technologies in general so disruption is really a process in this context anyways a disruption is a process where you can consider how a smaller company whether it be you know an actual business or whether it be a researcher like myself who will and almost all cases have fewer resources uh whether that be you know just access to funding or you know uh human hours to do development how can a company who is smaller and has fewer resources actually disrupt or change the since uh this established our incumbent business uh or process and so this this uh this image on the right is a little bit clutter and cluttered in the way that it's displaying the information but the idea is that um the incumbent businesses of the incumbent paradigms are generally focused on improving products and services for their most demanding customers so whether that be in a healthcare application where you are looking at the the you know the clinical makeup of people coming in to emerge and saying you know we recognize that renal issues are you know a huge proportion of our our population so we're targeting all of our resources and services toward the the dominant um you know issues that we face and this is common both from a business perspective where we're trying to generally accommodate the largest customer base or the most profitable customer base the same the same is true for technology developers who you know even if it's a new innovative technology it eventually ends up becoming a technology that satisfies the larger population and when we think about injury when we think about disability and we think about healthcare it doesn't mean that a large portion of people don't have non-dominant issues it just means that there are often groups of people particularly in local ecosystems that are struggling with common issues and so um you know entrance proved to be disruptive to these technological and and healthcare uh incumbent forces by successfully targeting these overlooked segments how do we target these smaller populations that are understudied and are under serviced and even in in situations like newfoundland that could be as simple a concept as targeting rural populations our rural population is proportionally very large but our rural population is also very very diverse and and one solution doesn't apply to all of them so in many cases we have large health care centers that that uh cater to our urban populations but what happens about these technolo what happens to these technological innovations that are targeting these rural populations so the traction comes from being disruption the traction for these companies comes from being able to talk with those smaller more nuanced issues and and populations and i argue that one of the things that is preventing translation of these technologies to broader market is what we consider acceptable um clinical evidence so we know that many of these startup companies we see lots of them emerging in st john's over the recent years they really want to build an evidence base for their products and that's become clear i am currently working with i think four startup companies through through various uh direct means in an attempt to be able to be able to develop both clinical and technological evidence that will support products um that they're developing so that they can now seek for example additional funding or start to gain traction in the healthcare community so these innovations within healthcare all innovations within healthcare are necessarily held to a very high standard but these you know these clinical practices that are are common for generating um these high standards are largely emerging from pharmaceutical development so pharmaceutical companies uh and the randomized controlled trials specifically are considered the gold standard of evidence and these these trials though specifically were meant to try to isolate the impact of one of these pharmaceutical interventions uh or or you know modern developments and see if in isolation over large groups of homogeneous people we can measure some sort of impact using very rigorous and well-developed statistical methods unfortunately as we'll see on the next slide a lot of these approaches are relatively unsuitable for technology-based interventions um i argue in many ways that they're counterproductive to the goals of supporting people with disabilities or health care issues uh and you know counterproductive to creating benefits for society and i think that the prime example again within newfoundland is servicing rural populations where you know undoubtedly we have to service these populations but we are we are just constantly putting these solutions on the background um so what is evidence you know and and what is this idea you know evidence of what is it of efficacy or is it of effectiveness because efficacy is really looking at the performance of a single variable under a very controlled situation and effectiveness um you know strictly speaking in a healthcare context is looking at how well an intervention behaves under very real world pragmatic conditions so that you know the the goal of something like a randomized controlled trial is very very different than the goal of a much more pragmatic study design that's looking for how how well something works in a real world scenario so i'm going to briefly skip over this because i'm assuming everybody here is quite familiar with it um but just showing that the evidence pyramid uh you know is really focusing on this blue stripe in the middle which is you know or sorry the green the dark green stripe which is randomized controlled trial of the light blue stripe which is sometimes missing from the evidence period uh pyramid which is sort of this idea of taking randomized controlled trials and synthesizing these these data into you know best practice guidelines which tend to be a little bit softer but even above that we see things like systematic reviews and meta-analyses which are um generally trying to go out into the world uh find randomized control trials on specific topics and synthesize those data into larger sort of accumulations of randomized controlled trials everything from the light green down is sort of lower quality evidence within the evidence pyramid so non-randomized controlled trials randomization we'll see is uh really important cohort studies all the observational studies tend to be um considered a lot less uh reliable in terms of quality of evidence and then down at the very bottom we have expert opinion or as i like to call it eminence-based medicine where we have you know people who are relying on a long history of experience and information um to make decisions and often not even turning to what we would consider best practices toward the top of the pyramid um i'm going to take a quick pause just to take a look at the chat which looks like it's empty um but just in case anybody wanted to chime in and ask any questions or throw in any comments before i continue um yeah hi yeah can you can hear me yep i can hear you very well thank you yeah um i really enjoyed your presentation so far it's making a lot of sense to me and um uh maybe i'd wait till you're finished until i bring up some comments about the technology and how it's being used in some places uh describing how how you are describing the use of technology in uh in a controlled trial i i've got something that i'd like to share with you and the group about something that we're doing just started that will i think you would appreciate what we were doing about exercise in rehabilitation with a certain group of uh a certain group of patients so it is a like a a group and there's already been done uh certain uh trials previously okay and uh that are looking at advancing this but it's just a little tiny start in what you're talking about here right now absolutely yeah i would be really keen austin to uh to be able to hear what you say and maybe you know hopefully you continue thank you for the positive feedback so so far hopefully you continue to find it useful because you know when you think about rehabilitation settings in specific that's a really really challenging world where uh solutions have to be very specific to an individual so uh yeah absolutely i'm planning on leaving maybe 15 or 20 minutes at the end for some open discussion i think that would be wonderful uh and if you feel like at any point in time you want to jump in absolutely please feel free to uh to jump in no problem thank you yeah thank you too uh so randomized controlled trial i think most people are relatively familiar with it this is the gold standard approach for collecting and evaluating data and you know as austin will probably know if you complete an rct successfully and it's reviewed uh appropriately it's generally due to be really good evidence um some of the some of the neat and effective things about randomized control trials or rcts as i'll refer to them uh are randomization so that's an attempt to reduce all kinds of things like bias and and you know issues with you know uh the humans that are involved in in both the intervention and the people receiving the intervention blinding as well same idea so that people are not uh changing their actions based on what they're seeing happening um control and intervention groups so here you have ideally two groups that are 100 identical with the exception of one group receiving the intervention uh and the other not receiving the intervention this is a beautiful dream but in a rehabilitation center setting it's very very hard to have two people in a room together one getting an intervention and one not and the one who's not getting the intervention not knowing about it or you know not not receiving any intervention so we'll see that these are common themes that emerge in technological solutions um the nice thing about rcts though is because of the way that they're structured and developed over the years there's the rigorous statistical approaches that are uh are relevant to them and even even the con concept of reporting results in how you report rct results has been relatively strongly researched all kinds of guidelines i've included one here for anybody who's interested um and the entire structure of an rct is such that uh if you follow the rules and you conduct it properly your evidence is is and the contribution of what your research is and your findings are are relatively well understood and that's the the main take-home message of an rct nrct so within this idea of completing rcts there's a sort of larger picture of clinical trials how do you conduct clinical trials this is a well-published guideline so the first step is small group testing you include a small number of participants again though these participants are are considered to be relatively homogeneous in terms of all of their characteristics except for uh the intervention they're going to we're not going to receive um initial testing is really about safety and looking at you know adverse effects is anything going to hurt or harm cause harm to people as we ramp up our studies um so you know arguably the groups that are involved in these early stage trials tend to be uh i use the term loosely but the worst of the worst people who are desperate for help um and looking for help so you know arguably there is a bit of bias already in this process um then you know we move to larger group testing where we're starting to transition as we move up move across this area we're really starting to move into uh more and more about evaluating the intervention itself and what the impact of the intervention is and toward the end we're now looking at how we can uh within post-market evaluation how we can roll this out broadly uh and you know arguably from a pharmaceutical perspective profitably uh across the continuum so the challenge with technology though is that these solutions that we develop and you know we think about exoskeletons and the cost of an exoskeleton we are never going to be able to produce hundreds of thousands of these devices to to you know complete a phase three clinical trial it's just not feasible uh in many cases to even have more than a few of these uh which are targeting very specific um use cases there are maybe not necessarily you know designed to go home with a person and you know when you think about pharmaceuticals whether you're taking five pills a day or you know one pill a day it doesn't really matter it's not taking up a lot of space or or time in a person's life whereas technological innovations aren't necessarily a lot more involved and nuanced so the weaknesses of rcts i'm not going to spend a lot of time on this because these are relatively well known and well published but basically time and cost are the two things that you hear a lot uh when you're rolling out a randomized controlled trial with 100 000 people across 20 different sites there's just a lot of coordination and effort that goes into that um but you know getting a little bit more uh you know relatable to to this this lecture is this idea of ethics so if you think about a pharmacological or you know pharmaceutical intervention the whole premise of a pharmaceutical trial is that we don't know whether it's going to do what we think it is uh in the case of a technological intervention especially from a startup company or from somebody who's building something they've done the work and they believe that this intervention is going to be good so if you have an intervention that is going to be helpful whether it be something even as primitive as a new cane that just reduces wrist and elbow strain why would you withhold it from somebody you know why why can't we not envision study designs where everybody gets it and then we work together as a group to refine and improve these things and this is very common in rehab and very common in in psychological uh interventions where you know we're developing things that we're quite sure are helpful um you know why would we withhold that from somebody and then this idea of conflict of interest so because our cts and i i don't want to get into this one too much but because rcts are are driven by you know pharmaceutical pharmaceutical type research rcts are almost always funded by pharmaceutical companies and so you know lots of reviews out there i i had one in my notes but i can't see it because i don't have two screens but over 500 our cts were included in a meta analysis that was combining them together and i think 300 of them had clear uh conflicts of interest because they were far they were funded by the pharmaceutical companies who were making the intervention but they were not disclosed so you know 60 of the uh you know supposedly blinded uh our cts were not necessarily free of conflict of interest and what we mean by conflict of interest is that the researchers scientists and stakeholders who are are conducting the randomized control trial have some potential benefit to gain out of a successful trial so my little image here on the on the bottom right shows you know about and ideally in society's perspective we want that boat to be going in the direction that is of benefit to society but sometimes individuals are paddling in the opposite direction or in another direction um so specifically thinking about innovations if we think about technology um you know some interventions like i said have obvious observable benefits i already talked about that one from an ethical perspective um but really the problem with rcts is that they're known for having findings that are are difficult to generalize to real-world contexts they're looking at large homogeneous groups um and then you know how do you apply that to an individual who's living you know in northern labrador when most of the participants were in you know a downtown urban london center um over reliance on group means overlooking valuable individual responses there's a an increasing push on understanding what individuals want to need rather than sort of pushing healthcare onto individuals talking about individuals specifically what their needs are um and and truthfully like i said within these rehabilitation settings specifically or other healthcare settings um populations aren't homogeneous there is it's almost impossible whether you say that you know a 43 year old man 5 foot 11 180 pounds and you have another you know 43 year old man 5 foot 11 180 pounds we're not the same person um those are just three factors that happen to be the same um and so the solutions are are suffering in many ways because of that um rcts only are measuring uh the impact over the period of the the trial uh in in many healthcare applications these are lifelong issues um the effects of the intervention and the uh issues associated with whatever the disability are uh or the you know the medical issue are are often lifelong and our cts don't accommodate for that cost and funding challenges what if you have a great idea but you just don't have money to do an rct um or the time or the resources to do it um and then i think number seven is one of the things that is nearest and dearest to me if you're working in sort of the the leading edge technical innovations technological innovations the technology is changing way faster than an rct a typical rcd is rct is approximately five years from start to publication of results we've seen this specifically through kovid where we've as a society somewhat accepted that we're going to bypass some of these processes so we get rapid results um but you know even fast within a covet context is two years three years for an rct within two or three years my entire technology platform has become obsolete um so you know in many ways i don't have time the the target is moving too fast but it doesn't mean the innovation isn't good so what can we do well you know this whole idea of changing how we view things and acknowledging some of these things and we'll see as we turn to uh the literature that some of these solutions have already been here and it's not you know these are not necessarily new solutions this is just new ways of looking um at specific solutions i'm gonna go a little bit faster because we're already over 30 minutes in um so one of the things here is you know consider developing these uh or adopting these different development models that are used uh predominantly around the world both in business and in technology developments that are non-healthcare related but you know think of ways that we can apply these to healthcare innovations so specifically for the for the rest of this presentation we're going to be existing what within one of these phases we'll start with phase one which is really about how you go from idea to a prototype phase two is the idea of taking a prototype uh that you have some confidence in and moving forward on it uh you know as quickly as you can and phase three is kind of the idea of uh you know a bit more permanence in your type of solution but it's intentionally a circle it's not that linear model this is constantly uh iterating over and over again and one of the things that i realize i didn't include on this is that users are necessarily whether they be the the patients or the clinicians or the other stakeholders who are involved everybody is is involved in this process all the time so this is no longer a linear process of you know phase one two three four uh and out the door it's just a constant iterative process of collecting data refining revising uh with everybody who is involved in it understanding that this is a shared approach to taking an intervention that is not mature uh and clinical evidence which doesn't exist in kind of slowly bumping all of them up at the same time so starting in phase one uh again i'm going to skip a lot of this technical stuff but phase one is really about idea to prototype and then if you think about completing phase three and feeding back into phase one again uh we're always taking a new idea to a new prototype there you're never at the end you never cross the finish line there is always a new prototype that's coming out as new technologies come out as new information and new evidence come out as you get feedback from individuals and situations so primary outputs here we're really looking at just starting to document things and get things pen to paper but but more than that really engaging users so how do we figure out who the users are who the relevant stakeholders are and understanding the whole delivery process as early as we can um so study designs we you know i've set the stage for rcts being inappropriate really at this stage this is a whole different way of looking at it and in many ways if you think back to the evidence pyramid one of the things that i forgot to mention is you will see that qualitative research doesn't even exist qualitative meaning sort of the non-numerical collection of evidence and information often is perceived as interviews and focus groups and speaking to people uh individual and hearing their lived experiences and what their perspectives are um so here there's another that this sort of technology experience and technology evaluation doesn't exist on the evidence pyramid as well um but the idea here is how do you take your innovation your technological innovation and and evaluate it against technical or performance requirements and the biggest question here is what are technical and performance requirements so um one of the limitations i think technology developers face is that technical requirements tend to be very very literal in definition whether it be you know uptime or downtime or crashes or you know things like that but technical uh you know technical and performance requirements can also include user experiences and so you know these technical evaluations become very very important early on in terms of understanding a user's path through the experience and where within that path through an experience with an innovation or an intervention where within that path things went right and where things went wrong so that we can refine our solution so to do this study designs that are appropriate for these early phases are of course qualitative research i am a strong proponent of qualitative research as being one of the highest forms um of clinical evidence uh so we're collecting analyzing non-numerical data really looking at individuals and understanding their reality their social reality their physical reality their perspectives on health and health care and this is why i believe things like rcts are very very limited in you know their perceived evidence and their value of their evidence because there's no discussion within an rct of how the humans using the intervention actually feel outside of the primary clinical outcomes also though lots of room for quantitative research here uh but most of the quantitative research in these early phase and quantitative meaning numerical the traditional uh experimental and observational studies that we've we've become relatively familiar with um descriptive studies again this idea of user user experiences and correlational studies so not necessarily looking for a cause and effect type of understanding but really a fact-finding mission a needs assessment i've heard holly a couple times quite quite often when i talk to holly she's saying these assessments um and this is really understanding what user needs are and what what factors are impacting these technologies that we're developing um and then mixed methods approaches are really just combinations of everything more than one method rather than committing to an rct as the um the premium form of collecting evidence but you know thinking more broadly about you know using multiple forms of data collection uh typically though the term mixed methods is referring to uh combined qualitative research with some other uh less some other quantitative approach that is lower in the evidence pyramid so whether it be cohort studies or um you know case studies or or surveys or things like that one of the things that probably a lot of people who who would watch this aren't familiar with our study design called a single subject research design design so the idea behind an ssrd or a single subject design is that people can also serve as their own controls so we think about a randomized control trial where we have uh you know one group of people who are all homogenous in terms of whatever factors we're stratifying them by uh we divide into a control group which doesn't receive the intervention in a group that does receive the intervention um there are study designs ssrds where a person serves as their own control so you you know you look at a person uh and study them and and collect actual empirical qualitative and quantitative data before an intervention uh apply your intervention study that person and collect data during the intervention and then you know remove the intervention and see if they go back to some sort of baseline performance and then you know maybe apply it a second time so uh a couple of strong things about the study design are that we're using repeated measurement over a period of time within the baseline group you may say for example that we're going to follow a person for one week then we're going to you know use our intervention follow them for another week remove the intervention for a week and we're going to sample them three times a day so that we have this idea of repeated measurement and the strength of this is that we're overcoming some of the statistical limitations of individual single uh measures so the nice thing here is that we can look at how different individual people respond and we can conduct these single subject designs across multiple people uh and synthesize those data rather than you know treating every group as one homogeneous group we're treating people as individuals and seeing if we can figure it through the combination of these mixed methods what the impact is being caused by so here you know we have just just more for a reference if anybody wants to look back on this different designs within series design is basically looking at just one person over time between series designs a series being a baseline and an intervention um it doesn't even have to be one intervention here uh you know baseline a uh the the a phase is just a normal um you know a normal day in a person's life b1 is uh you know pure tutoring and b2 is a second form of peer tutoring uh so it's within series if it's the same intervention it's a between series if they're two separate interventions uh combined series can be a mix and match of these but the neat thing is these are all the same people so days across the bottom if you look at baseline there's a relatively standard performance if you look at baseline two you see that there's a relatively high measurement and perhaps that's a learning effect a person who you know is aware that they have an intervention happening and they're maybe behaving slightly differently but over time presumably people return to their normal you know their normal behavior and so if you see something like this uh you can analyze it traditionally for single study design statistical methods are not overly rigorous but you're looking at a visual analysis and you're saying at the baseline phases after a you know a return to baseline two it looks like a person's operating at 10 to 20 percent whereas with the intervention they're operating at 70 to 85 so there's you know some some strong data here showing that this intervention is actually um you know having some sort of impact on a person's life so you know through some of those early phases and these single subject research designs have been you know have been uh you know in the literature for at least 40 or 50 years so they're around they're just not generally viewed or even covered in research methods uh lectures um so phase two now you think you have a good project you've run some single subject research design some different approaches now you're looking to refine your intervention you're probably doing this as we'll see through some of the later slides you're often in a technology perspective continuous continuously refining so you're not just holding your intervention stable you're actually continuously updating based on user feedback and in many ways why wouldn't you be if you if you're conducting an rct and you see early on that the results are not good you end up canceling the study right why not refine the study and you know so there are more modern designs that we'll talk about like step towards designs where we actually have the ability to uh iterate and you know this is a reflection of how our cts even are sort of adapting some of these single subject research design methodological uh approaches so you know phase two we're really looking at just better understanding users and making a better product that and we're shifting a little bit away from evaluating the technology now and we're switching now more to the evaluation of the intervention itself so uh just really here various modifications on different single subject research designs the randomized control then of one trials um here you have randomization introduced so you know we talked about rcts briefly where you're randomized to a controller versus an intervention group um here i talked about a standard ssrd being an abab design so uh baseline intervention baseline intervention you can randomize that for example so make it a baseline then a baseline an intervention intervention based on intervention any combination of these and you you will decide this and you'll decide your randomization scheme based on what your feedback is from your users so if your users are saying oh it was so obvious when i stopped using the intervention and that's why there was a learning portion i hope you guys can see me because i just got a notification that my intranet was briefly disconnected can anybody hear me can i get a quick confirmation that i can still i can i can hear and see yeah thank you okay all good excellent um so you know alternating interventions so rapid alter uh alternation i had mentioned in a single subject research design that uh you know one of the things that we do multiple measures within each phase uh sometimes for example that can introduce some different forms of bias in both the user and in the researchers so maybe instead of you know five or ten measures within each phase only go for one measure and rapidly switching between intervention and and uh non-intervention it's uh you know far fewer within phase measures so here you see you know two different interventions or you know back and cool i guess on the bottom there's no intervention in the top of some intervention or vice versa um ideally you'll be able to see some sort of difference between uh you know between the intervention and non-intervention portion again though the the caveat here is that the participants are serving as their own control and so you would be doing multiple trials at the same time with different people but collecting individual data and synthesizing at the end of the trial multiple baseline non-concurrent and concurrent designs are again just uh the idea that you can so a current design would be running the same intervention strategy on the same individual at the same time a non-concurrent recognizes that in some cases um you just don't have the population available and so you know if you only have if you're working uh on some sort of condition that is relatively rare uh you can only conduct an rct for the most part if you're doing it all at the same time if you stretch your your time window too far then methodologically your rct becomes weaker but you may not have a choice so do you just throw away a technological intervention that could be good because you can't do it at the same time or can we think differently about how we collect data also group based designs like rct ish designs are not uh it's not that you can't do them with technologies just that you may not be able to do them um so interrupted time series design you're basically uh just looking at uh you know a person over a longer period of time so now recognizing that these interventions sometimes you know just doing a one-week intervention is not enough maybe you're going to follow a person for years over time and you're going to you know collect a lot of uh data on that person initially and then introduce the intervention continue collecting data and then you know remove the intervention and follow up so it's basically a single subject research design over a long period of time if you do this over different people it increases the the robustness but again the cost and time requirements and resource requirements go up um just quickly taking a look at the time so we're at 12 51 i'm going to go a little bit quicker but trust me we're almost at the end um one group pre and post test so here uh before you introduce an introduction you see in the cartoon on the bottom right uh you issue some sort of pre-test survey often is what we would do we would do either like a validated clinical tests or some sort of questionnaire to understand what a person is like a baseline rather than just external measurements but actually hearing their words and their understanding of what their baseline is like uh introducing some sort of intervention and then doing the exact same test or some modified version of that test post intervention so you get a difference between pre and post where you're actually measuring your own external variables as well as you know soliciting information from a participant directly non-equivalent pre-test and protest process is the same thing just that sometimes you might not have access to you know all the groups that you would want so you're basically allowing people uh who do and don't have the issue to participate in the study uh an example of that would be for example if you had voluntary participation in um you know you think about an educational concept where i'm teaching a class and i have a voluntary uh tutorial so people don't have to come i can do a pre-pre-examination of people's knowledge uh just after a before a lecture after a lecture and then after the tutorial and then maybe after an exam and i could compare the two groups but they're not equivalent because i'm allowing people to naturally select versus um randomization so some of the strengths of randomization are that you are trying to remove some of the bias but some of the advantages of not randomizing your is that you're actually allowing people in the real world context to be able to uh you know share their opinions and then of course stepped wedge trial designs which are our cts where instead of doing it all at once you're intentionally ahead of time determining how you're going to stagger your intervention amongst different groups in many cases there are variants of step-to-edge designs where if you find your intervention is not working as well as you can you can change it and in this way you can see what happens when you change it on on the impact of your pre and post groups um so i'm going to go a lot faster here because i'd like to give some time for uh austin and anybody else to say something um this is again just uh uh you know more phase three we're now looking at things that are more and more um about how well the intervention is working but the big difference in phase three here from a technology developer is we're putting less emphasis now on the impact of the intervention and much more emphasis on the experiences of the users themselves um so tracker trials are a relatively broad encompassing term where we're acknowledging that something in the environment is changing whether it's the technology and the intervention itself whether it's the people or whether it's the challenge so covet as a prime example you'll see a lot of uh variants of tracker trials out there for for covid research where they're they're perceived as systematic reviews or reviews but really it's about aggregating information and comparing apples to orange on purpose understanding that the world has changed the intervention has changed the demographics of the people have changed um and so you know the statistical rigor disappears in favor of some of the less statistically rigorous approaches that we've already talked about um but recognizing that we don't have to always control everything um so the benefits are that we don't have to wait for interventions to be fully developed and we're actually allowing for these changes to be taking place um and then i think the last big one that i'll talk about are pragmatic trials so really um uh these explanatory trials that most uh rcts are are out there for is trying to look at the impact of the intervention specifically on a very very small number of outcome measures pragmatic trials are the exact opposite of that i shouldn't say the exact opposite but are very different from that in the sense that they're really looking at effectiveness so what are the usual conditions that these devices are being used or these innovations or these interventions um as opposed to looking at whether you know the intervention is working under optimal conditions um so again these pragmatic trials tend to be more of an encompassing term but there are true pragmatic trial designs uh trying to stay away from being a research methods webinar i'm not going to go into too much detail but you know understand that in practice most trials do have a pragmatic and an explanatory aspect but for me the the big take-home message is that the explanatory aspects are the ones that are are given all the credit uh and the credibility for success um whereas the pragmatic outcomes tend to be secondary outcome measures within uh these trials so really really quickly what's the difference um explanatory being the more uh exploratory or experimental in design have strict eligibility criteria versus pragmatic which include anybody um experimental interventions everything is very controlled i guess uh the take home message is the intervention uh what the practitioner does both with the experiment and with the comparison are very very strict um and very very highly controlled where whereas in a pragmatic trial it's the opposite you're allowing people to do what they want you're giving an intervention to somebody for example and if they never use it they never use it that's a valid result that's something that we're interested in it would be more interesting to know why they didn't use it than to force them to use it and report on why they didn't like it for example um so you know with an explanatory everything is rigid everything is defined a priori everything is strict the outcomes are very very specific compliance is very strict and the outcomes are analyzed specifically for the primary outcomes uh pragmatic trials in comparison tend to be a lot more loose and no specific follow-up your solicited participate if you don't participate that's that's in some ways taken as feedback directly as well um so it's kind of interesting to see that so in summary my take home mes
2022-03-03 05:27