Microsoft Power BI: Delivering business value with AI | BRK3212
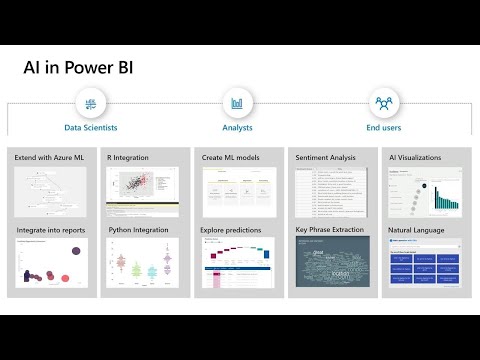
Hello. Everyone, thank, you so much for joining our session, looking at delivering business value with, a I my. Name is Justine Olynyk, I'm a program manager on the AI team I'm joined with my colleague Serena Stevens is also p.m. on the AI team and today. We wanted to give you firstly, an overview, of all the different AI capabilities. That we have in power bi we've. Recently had some critical announcements. And we've got some other new features that are coming out really really soon so, most of this session is really just gonna be very demo, driven we're gonna show you the new features that have just shipped and some of the new features that are shipping, basically, in about a week so, you're gonna get a sneak peek into that and generally. We just want to have you understand, you know what sorts of things for investing, in from an AIX perspective, and power bi and give, you an overview of what's coming. So. Just to start off with I wanted to start, by giving an overview of what the landscape, of AI features, looks like in power, bi and. We really are trying to cater towards a, whole host, of different personas, starting. All the way from our data scientists. To our analysts, and to, our end users and, really. The kinds of things that we're trying to light up for these users are very very, different if we, look at our data scientist persona to start off with we're, really not trying to compete with tools, like R or Python. We're not saying power bi is going to be the next Python we totally understand, why, data scientists, want to leverage those sorts of tools and we're, not expecting, them to replace those with power bi what, we would like to do though is provide an extensibility, platform, for those sorts of users so, if you do have R scripts if you have Python visuals, if you, want to connect to Python or R you, can absolutely do, that in power bi and so, what we're really trying to do is facilitate the, collaboration, between analysts. And data scientists, I actually, started, from the art world that was kind of what I did before I even touched power bi and, my first thought was well you know what art can do everything why do I even need power bi and then I started using power bi and firstly I realized I might have been slightly wrong but, secondly I realized also that I can actually do a lot more with, our power. Bi for, example if I plot a visual, I don't have to plot or, write up ten different scripts to cross filter by different things I can just click on power bi visuals, and the our script will automatically, update so there's a nice little touches that make it actually a really compelling experience, and makes.
It Easier for data scientists, to share their work with the business as well now. Moving along we also have within, the data scientist space extension, with Azure so. Of course you know we have Azure. Machine learning services. Which. Allow our, data scientists, to build machine learning models inside the Azure world and so, what we're trying to do over here is again, if you have your machine learning models already we, want to make it super easy for, you to be able to bring those in to power bi and just, leverage them so, when you're actually doing your data prep you're, gonna be able to see that, you can just connect to an Azure machine learning model, and call, it and basically map it to your data so, again that kind of seamless integration, is something that we're really looking out for now. If we move over to our analysts, they're the landscape is a little bit different we're, trying to provide our analysts, with a toolkit, of capabilities. That they can do in power bi so. We are shamelessly, stealing, from the Azure world, of course we want to collaborate but we also want to pick up that technology, and just, put it directly into, power bi and a nice user experience, so, if you do want to build machine learning models and power bi you, can absolutely do that we've, integrated automated. Ml which is a service that's built on top of Azure machine learning, directly. Into power bi so, you can very easily build, your machine learning models, we. Also have brought in cognitive services, so, making, it even easier we have these pre trained models that you can run as enrichments, as part. Of your data prep pipeline, so if you want to do things like sentiment, analysis or keyphrase extraction that's. Again a very very easy job in power bi you don't have to write any code. Finally. If we look at more of our end users, we're really trying to democratize these. Sorts of experiences, but. In ways that end users can consume them as well so for end users it's all about allowing them to explore their data interact. With their data and find insights quickly, and easily so. Here we're doing a lot of investments, in something we call AI visualizations, and, Serena's going to spend a lot of time showing you some cool demos around this where, we're really packaging, up some techniques, into, a visual our, analysts, know how to build visualizations our end users know how to consume them so, if we can sprinkle them with a little bit of intelligence we can make everyone's lives easier and also. This is where our natural language capabilities. Come in so, QA is a very important, part of power bi if. You've been following the announcements, just last, month we've given you an a completely, new facelift and introduced, a completely, new, set. Of capabilities that. Really make the QA experience. A, lot. More unique, and innovative than, what you've seen before and so, this is really allowing our end users to interact with their data in a really natural way.
So. What we want to start with is the, end user experience so, we're going to be showing you some of the demos for AI visualizations, and, natural language and with that I'm gonna pass over to Serena. Sorry. Okay. Cool Thanks so. Analyzing. Your data in Part VI can be a very time-consuming process and. Really. Getting to an, insight, that is truly useful can, be very tedious but, there's also a lot of things that we can do to. Make that process simpler. And faster and. That, is why we're introducing AI, visuals, into power bi so, ai visuals. Help. You to explore, your data and, just. Adding, a little bit of artificial, brain power to make things easier and faster. So. The, one time that might mean, that we help you iterate through. Trying. Out tens, of combinations, of inputs. The other time it might be getting, you an answer to, a question you have about your data in a, couple of seconds. So. Just to show, you a couple of examples I'm, looking I'm working on a supply, chain data. Set here so my company is producing products. In, the. Healthcare industry and I'm. Distributing, the products, and then finally, selling. Them to consumers. Now. Having. A lot of product, in stock is quite, expensive but also, the last thing I want to do I want. Is to run out of products, and for products. To be on backorder. So. This analysis, is to look into why, things, are in on backorder and how I can reduce, that. That number. So. First of all I'm going to start with the using, the key influencers, visual, and my. Question, here is what. Influence, is a product, to be on backorder. And. So. I'm just gonna add some. Factors. That. I want to explain by. And as, I'm adding these things you can see that, that. The visual is is returning. Starts, to return results and actually every time I'm adding effector a new. Regression. Model. Is being run and so, you, can see, the the. Visual update every time. Let's. Have a look at the results. So. The first thing that pops out is that.
Apparently. When, the demand for product, is volatile. It, it's way more likely, to be on backorder, now. That's not very surprising, because anything, that's volatile, is is hard to predict so. So. It's it's likely that we run out at some moment, I. Can. Also just click. Around in my report to see other demand, types so for, instance I'm, looking here at some, some. Different situations. And different amount. Types and as. I'm, now looking, at, intermittent. Demand something interesting, is is popping up again you saw the dividual, is recalculating. The. The. Influencers, and it's. Now showing that when. We're. Accurately, forecasting. The. Demand there, is actually, still, that, is actually a factor, that influences things, to be on backorder and that, doesn't make any sense to me because if we are able to forecast accurately, then, why would we run out of stock so this, is something I want to look into further. And, I'm. Gonna do a root, cause analysis, using. The decomposition, tree. So. With, the decomposition, tree I can. Have. A look at the percentage, on backorder in, in. The total, data set and then. I'm going to split up by different, categories. From, the, first part of this analysis, the thing I took away was that, I want, to look. At the the forecast, bias because. There was something going on with that accurate, forecast, and. Then. I was also looking at the demand types, so, these first two splits, are, easy to make and, you can easily see, like how that, percentage. On backorder is then distributed at over these categories, but. Now, I am I have a problem because from, this point I really want to know where, the bottleneck, is in my data so which what, is causing, that higher number of products, on backorder, for. Intermittent. Demand I. Can. Now split, by, different. Categories. To try it out so maybe there's a problem in a certain, region or, with, a certain shipment type but, I would have to go and try out all of these things and maybe even different combinations, of factors. So. Instead I'm just gonna ask RBI what. Is the highest value where. Where is the highest percentage. On backorder in my data it. Turns out that is in product type and specifically. In patient monitoring, so. I didn't have to go through all of that it was just done in one click let's. Do that again I'm just gonna. Again. Look for the high value and, now. Something. Interesting is, popping up you, can actually see that there's, one particular plant, it. Has a very high percentage on, backorder and so. Apparently. The problem. Is not that we cannot predict, whether, we're or. What the demand for something is gonna be but, there is just as planned I can't keep up with. Producing. Enough for this particular, demand. Now. I. There's, a lot, more I can do with the decomposition, tree so this was a very specific I, was, really looking for that path of the highest. Percentage. On backorder but I can also just. Explore, a little bit more of my data and, for. Instance look at the different, forecast. Category. Now. What you see happening here is. That. Because. I was asking, power bi to find me the highest value. It's. Now doing that again and and, it's seeing that this it it's not in the same categories, that these highest values are shown, so, while I change to a different forecast, category, it's now showing that, there is actually a specific, distribution. Center instead, of that plant that was the bottleneck, before. I. Can. So. So this really works like any other visual so I can also, use it to bookmark, certain, things so, for instance I can I've.
Created Two bookmarks, here where, I can either look at the, high risk which is the highest path or, I look, at the low risk which is looking, for the lowest path so this way I can really prepare that for my users publish, it out and users can interact and play with it if. I want people to. To. Explore. Their data with users. That consume, my report to explore this data but not change the, actual categories, I can, also just lock. These for for, the consumption stage. Okay. So I want to. Explore. My data a little bit further and. I'm going to use the new Q&A visual, to do that. There. Have the cue a visual, on on the page here and it's. Already showing me some suggestions of questions that I can look into, so. For, instance I can, look. At the, let's. Say top. Plans by product, availability, and, we'll just return in app or I can start typing my own question. Now. With, this new release of the of, QA and. The whole sort of revamp, we, really put a lot of attention into. Not. Only the. The. Access in the product so you can now create the visual and access it easily through, there but also the search UI and making, improvements to what, you see while you're typing to, really help you get, to the right questions and, and and. Find your answers. Okay. So I'm just gonna say I'm looking, for. Backorder. Amount. By. Area. And. Now, you can see something interesting happening, here so. Area, is. Red. Underlined, so, apparently it's not in my data model there's no where. Where I defined, what area is or what. A number, by area, but. Still I'm getting results and I'm, getting the amount of backorder, by region. And, so what. We did here is we integrated with, being. An office, to basically. Gather all their, information. About, synonyms, and put. That all in QA so, we. So, that's how we kind of have all these. Additional. Synonyms. That we can detect without you, having, to define them. Now. Is that any other visual all this this. Visual is just interactive, so as soon as I have my result I can go and interact. With it in my report. That's. Ask another question I'm, gonna I want to look at the. Percentage, out of. Stock. By. Product, type. And. Again. You see something happening here there, is a, problem with the percentage out of stock so while in my daily conversations. I know that. Percentage. Out of stock is exactly the same as percentage, on, backorder, but apparently that is not how it is represented in, the data so. So. I'm not getting an answer. Luckily. If. I just select this out, of stock I can, now go, and easily, define that so. When. I do that I'm getting into the into. The Q&A, setup. Experience, and this, has allowed me to wade. Through this easy UI just, add as many synonyms as I want, and just fix, all of the things that are not understanding, Q&A. So. I'm gonna submit this question, and I'm. Gonna say I actually want to define the. Whole that, whole section of percentage, out of stock as being exactly, the same as percentage, on backorder, so. I'm submitting that and. Then. It I can actually use, Q&A, to teach, Q&A, so, now I'm going to say. Percentage. Them out of stock is the same thing as percentage. On backorder and. I, can save that to my model as, I. Save it you see that now percentage, out of stock is actually. Understood, by Q&A and if, I go back into the report. I should, probably. Fix. The product type as well. Now. You can see that the results are kind of coming, back and in just a few seconds I added that synonym, to Q&A and it can now use it. Now. There's a lot more that I can do here so I. Can. There's only so far I can go as the, report, builder in thinking, about what. Users, might ask and what things I might want to ask add, a synonyms but what. Would be really useful is, to know what people are actually asking so as soon as I, publish. My report, and people are interacting with it what are the kind of questions they are asking and what are the things that are not understood, now. You can see exactly that here in a Q&A tooling so I've published this report people, are asking questions and I can see them what. Is going wrong here and I can actually just. Easily, edit. Them as well, so. In the same way I can now say factory. Might. Be the exact, same thing as a plant and. I can save that here.
And. I can even see it got a nice preview. Of the result over here, now. As I'm adding all these terms I have this list. Of my term so you can manage them it can throw, away things it can edit it and then what. As soon as I update my report, in the service every, consumer, of my report can benefit from this so. That's. Just a little bit of things about a. Couple, of the new aiv, that we have, in power bi awesome. Thank, you so much Serena, so. To switch gears a little bit we're, gonna be now moving more towards our analyst persona and, we're, gonna be focusing on to the capabilities, in power bi which is about building your machine learning models and applying, enrichments, on top of your data so. Out of curiosity just as a show of hands how many people here have built machine learning models before in, any tool ok, handful, and just raise, your hands loud and clear for those who have never built a machine learning model before in their lives, awesome. That is what I was hoping to see so, we're gonna do a little bit of a crash course and, machine learning today, so we're, gonna do a demo but we're also gonna go a little bit in depth about how you actually would build your first machine learning model in a tool like power bi so. With that I'm gonna jump into, let's, hope I can get the screens right this time, nope. Clearly I can't. The. Numbers are all very close together so. Ok. Ok, ok 6 there we go, yes. Perfect, ok, so we're gonna go through scenario, where imagine, you are running a cloud service and. You're. Very interested, in how, your customers are, perceiving, the, product, that you're offering them so. We have a bunch of new customers who have joined the service recently, and, we, have built, a basic report about, maybe customers, in terms of which regions they're coming from what's their role whether their interests but, what we really want to do is we're going to add some predictive, analytics, to this so, we want to understand, which of these customers, are likely to in the, future be unhappy, with our service now. We don't know much about these customers, maybe the abusive been using this for a couple, of weeks but, what we do have is we have historical, data about customers who, have been using the service for a little bit longer, and we've already let's imagine that we sent them out a survey they filled it out and they've already told us whether they're unhappy. Or not and that's, one of the first key things for machine learning is, you, need to have your historical, data ready so machine learning it's, not magic it. Can't predict the future unless it has a good quality data to start off with so, we need to collect some data where we already know some things about our customers, the, data has to be same sort of data that you'll be using on your new customers, to do your predictions, so, if at the time of training you, for example know that there might be an admin or consumer, or publisher, that. Sounds like a good field to use because, you probably already know that for your new customers, having. A field like maybe, you, know that they've been using the service, for three months might not be the best kind of feature because, you, don't know maybe at the time of when you're sending out the survey you know how long have these new customers been, using it so it might be a little, bit of a skewed feature so the first thing to think about is what. Is my historical data where, I already know the true outcome whether they're happy or unhappy and is that data the same as it's gonna be for my new customers. So. Over here I'm in data flows for those of you not familiar of data flows this, is just like power query and the power bi desktop where you can prepare earlier data but. This is kind of the enterprise version of that where, you can create these ETL, jobs that are scheduled, where, power bi handles or your functional dependencies. So this is kind of the enterprise story for a reusable, data prep but we're not gonna be spending too much time on this but. The one of the nice things about data flows is you can build machine learning models on top of them and just have them as part of your data prep hype line so. Here I've already got an entity, with historical, customer, feedback so. What you can see here is we've got some data about. You. Know all of these different customers, have been using the service for a while we've, got how long they've been with us they've maybe we've got complete weather they've completed tutorial, or not how. Many tickets they have open subscription, type and so on and, what. I can do is you'll see this little brain icon, over here with a lightning bolt it's like super, smart brain and I, can add a machine learning model to this and then.
We Go into the installation process of the automated ml model so. What we've done is we've provided a very nice user interface that makes it simple to leverage this automated, emmaus service that exists, inside asher. Now. The first step i need to do is i need to figure out what. Is the field that i want to predict so, again this is my historical data so i already know this and I, have very. Luckily. A field in my data model that, is a binary field it's either true. Not and it, tells me whether my end-users. Gave me a high rating or a low rating okay. And, I'm gonna click Next, now. Power, bi is gonna take a couple of seconds to take a look at this data that I provided it with and it's, actually going to recommend what sort of model I should build so, again we try we're trying to simplify the process a little bit power, bi take a look at the data it figured out we have two outcomes it's either true or false, high or low and so it's. Recommended, a binary prediction, model if I want to select a different model I can do that these are the sorts of models we provide so, you can build either a binary prediction, which is two outcomes a general. Classification model. That might be if I have multiple, categories so. Instead. Of something that's just high or low maybe. I would have wanted to split out my survey, results, into let's. Say scores, from one to five but I want to classify them as separate groups or, maybe I have you know a high medium low score, or something like that so, this is like multiple groups and then, you can see a regression, problem here is completely grayed out I can't even select it because this. Is only for numerical, data so if I'm trying to let's say estimated house price that. Would be a regression, problem because, it's a continuous, scale and so here because it's a true or false I can't even select a regression option, I'm. Gonna go with power bi is recommendation. I'm gonna go with a binary prediction. And. Here I need to focus on what, it is that I'm interested, in predicting so.
In My case I'm actually interested, in when high rating is false because, I'm interested in knowing when, my users are gonna be unhappy and, so. What I'm gonna do is I'm gonna give this a label which means maybe risk and this might mean a no risk okay. Now. The final step of building the machine learning model is giving the, machine learning model of data to learn from so again not magic I have a label which, in this case is true or false and I have all of the features that could maybe maybe. Predict whether. Something, is going to be a high rating or a low rating so. Let's imagine for a second the completed, tutorial, is a very very important feature maybe. Everyone, who has completed a tutorial, you. Know knows how to use the service perfectly, and so they're all super happy and everyone who hasn't thinks. The service sucks and so, what's going to happen, the scenes is every time the machine learning model sees completed. Tutorial, it's gonna look at what the label is and it's gonna over time learn okay every time I see this is yes I see. That there's no risk and so, that's something you know so you need to provide lots of examples, for a machine learning model to be able to learn from so. Here I power, bi has already done an automatic, selection for me I can change the selection around if I want maybe, we don't want to use tenure because that might be a misleading factor, maybe. I do want to include mobile so. Again I can change and tweak this around if I wish to do so you. Know there's certain types of features you probably don't want to be using you, can see we have let's say first name last name and email generally. With machine learning you want to provide something that has lots of examples, to learn from if I have like a customer, ID we, have customer IDs one and someone's, happy customer, ID is two someone's, unhappy, we're, not gonna learn anything from it because guess what every customer ID is gonna be unique so we need some examples, that the model can learn from you, know across time, ok. So, now that everything, is ready I can, give my model a name and I, can basically go. Ahead and train this model and there's, gonna be a couple of things that happen behind the scenes when I actually train this model first. Of all ultimate, adamm al is gonna look through all the features that I provided it with so, when I see features I mean all of these factors. We just gave the model and it's, gonna do its own feature selection, so it's gonna actually figure out ok out of this list that you provided me which, ones can I feel like I can, meaning completely learn something, from and, then, I can even create its own features, so, imagine, for a second, that you actually had some sort of verbatim, from the customers, maybe you have some. Sort of comments, that they provided, if. You just provide us with a long text string what awesome ated amal's gonna do is it's, gonna break up that text for, and pick, out all the different words that the machine learning model can learn from so.
Imagine You know someone wrote hey this is a great service I'm very happy, chances. Are someone else is not gonna write that word forward so if I just look at the whole text individually, again the ML model won't learn anything because every. Text field is gonna be unique but, if it splits it out into different words and maybe it splits out the word happy, chances. Are other people use the word happy and we can learn and when people say happy there. Is no risk they give us good readings right. So we, can do more in a richer data to learn more from it another, example is if you give us the date field, maybe. The complete date isn't important, but if we split it out into a month or into, a day of the week that, might matter as well, so. That's the first step we're gonna do feature selection and feature synthesis, then. We're gonna do the algorithm selection, so also mated amel has a whole bunch, of different algorithms available, in its library and it's gonna iterate through them and it's gonna figure out which algorithm, gives me the best results and it's, also going to tweak all those algorithms, with different parameters, so, you can adjust different things and that's, called hyper parameter, tuning and it's. Gonna do this iteratively to, figure out what is the best model that it can possibly build, for me and so, that process takes a while we, usually do, about twenty to thirty iterations, although. You can adjust the time if you want to just do a prototype you just want to train for five minutes you, can do that too but what. Basically happens, at this point is once. The model finishes training, so imagine I hit save and train we waited for I mean a couple of minutes or an hour or so I would, basically let's, jump back actually I could. View my different machine learning models here and the machine learning models tab and I, could view my training, report, so. This is the training report we have generated for this customer, classifier, model, and what. You can see over here is, a bunch of different metrics, that can help me evaluate whether, this model is a good model or a bad model, now.
In The new version of this report that we just released last week we, actually gave you an overall score for the model so this number that, you see over here actually pops up on the first page because, some of the feedback we got is we want one number to evaluate how good the model is we need some sort of starting point so this is a pretty good starting point I can't. Tell you you know I often get asked well what is a good model what's a bad model that's, a very philosophical question. And it really depends, on the kind of business domain, that you're dealing with and if, you're doing maybe a marketing, campaign and you're trying to figure out which customers. To target maybe, it's okay if your model is 70% you. Know you're gonna get it about 70%, at a time if, you have, let's see a fraud, model, or something. In healthcare you probably want that accuracy, to be much much higher so, it's hard to kind of give a bulk work ballpark, figure of you. Know what's a good model but generally. Anything above if anything, under 50%. You're better off guessing, it would be like a good, benchmark to start with the. Training details this actually gives you a sneak peek under the hood of automated, amount so. Tells you what we've actually, gone. Ahead and done, so all those iterations, I was telling you about you. Can see that over here so you can see where the model started, and then something. Happened over here this was definitely not a good model but, you know you can see how it kind of progressed over time and, then, you can see the final score and the final algorithm, that was used now. If I scroll down you, can actually see all of the different parameters that were being used by in this case the pre-fitted soft, voting classifier, and. You can also see how we went ahead with data feature ization so which features were used and additional. Information like hey what do we do with missing, values or what kind of column. Type was it so, you have the full information of, all, the training that happened now. For, a binary, prediction. A very, important, kind. Of way. Of being, able to not. Only tell how well the model is performing, but. Another. Very important function is to determine, what your precision, and recall are so I'm gonna just spend two minutes on this I'm gonna go through, this at, a very high level but.
You. Can think of it this way if let's, use our example of customers. Who are at risk or no risk if, I was to let's say run a marketing campaign I could target every. Single customer, out. There and you know what every, customer who's actually at risk I would, target them because I've targeted everyone but I'll also be targeting lots of customers, who, are not at risk and so I'd be wasting a lot of money targeting. Let's say 10,000. Customers, when actually only let's say 3,000, at the merge risk now. I could go to the other extreme and I could send out my marketing campaign to no one you. Know what I wouldn't get anything wrong I wouldn't get any people who are actually not at risk I wouldn't target any of them but, I would also not target any of my people who are actually are at risk and so, this is where this recall and precision slider. Comes in where, you can optimize do, I want to target everyone. That's, on one hand or and. So generally. You wanna again, it really depends, on your business domain, of what it is that you want to optimize, for, often. If you're dealing with let's say a pro a fraud, kind of problem, you, probably don't. Want to miss out on any fraud so you want to be very precise you know or sir you want you want your recall to be good so you, want in that particular, case not to miss out on any fraud because maybe missing out on one fraud case could end up costing you millions of dollars whereas, checking a couple of fraud cases that aren't actually fraud isn't, gonna be that expensive but. Again it really depends on the type of problem that you're dealing with so. This is kind of allows you to determine, what that threshold, basically. Is between your precision and Yuriko so. As the final step I can apply this model to my new data so. Imagine, I've brought in my new. Customers, and, I, can actually then leverage, this model and use. It for customers, who I don't know whether, they're at risk or not yet they haven't filled out any survey but, I can essentially, try and predict which ones are gonna be at, risk and so. This, is going to allow me to maybe, more preemptively. Be able to target them with maybe a marketing. Campaign or maybe I want to give them a free month, of my service, or maybe I just want to follow up and understand you know what might be causing them some pain points so. Once I apply this model to my data what I'm gonna get is I'm. Going to get a new column. That's. Going to give me my, score. So, it's gonna tell me whether they're at risk or not depending. On where I set this probability, threshold. It's. Going to give me that label of at risk or not risk, so, for example I set it at 76%. Anyone. Who has a score above 76%. Will be at risk if I said it at 40% anyone. Who has a score higher than 40%. Will be at risk so, this, is gonna be determined by this threshold discourse themselves want to change but the label will and then. The final thing I'm gonna get is actually row, level explanations. For. Every single individual, so. Let's take a look at what that looks like I'm gonna move back to my original report and I'm. Gonna jump into this new. Report page we've discussed risk. Now. What you're gonna see on the, right-hand side is a table, that I've added which. Gives me the risk score for, every single individual. So it's row by row and I've, already ordered it to go from the highest risk score to the lowest so, firstly, I can maybe do something like top 10 customers at risk or top 100, and then, I can select one of these customers, and what.
This Waterful chart gives you the top is it, gives you what is actually increasing this particular, person's risk and what is decreasing it so, again I'm getting that row level insight, into what's going on with my data so. For James over here we can see that what happens to be increasing, his risk is the, fact he used the mobile app that seems to be not, working out for him the fact he has some tickets open the fact he's a premiere subscriber, and there's, a couple of things that are decreasing, his risk - so just his company size and so. I can all use, all this information to, see how the score, of 88, was essentially, derived so. Here you know you can basically start, using automated, ml to start blending, your, descriptive, analytics with, these sorts of predictive, insights from. A data flows perspective, you can just schedule, this and every time your data refreshes. It's just gonna be operationalized, and you're gonna get two new predictions, that, come in. Awesome. So with that I'm going to pass over to Sarina, to take a look at some of the cognitive services, and, your number seven okay. Okay. So so. Far we looked at how, you can use some. Of the AI infused. Visuals. To, get quicker to insights. How, to actually build your own machine learning model but, you can also leverage. Pre-trained, machine learning models in power bi and I want to show you a couple of examples of that. So, I'm looking I have a data set here that is, about rental. Properties in Seattle, so, vacation. Rentals people. That visit. Stay. For a week me, and then when, they leave they might leave a comment. And. And, you know about. How happy they are with their stay, so. I visualize. This data here I can, see, some things about you, know what what are the, priciest, neighborhoods, and with the best, occupancy, so, maybe if you want to make the most money you need to be have a loft in the industrial, district, and, I can see like the the, revenue, that that, is, generated, on an annual basis I can also see how many reviews, people leave, per, month but, because, those reviews, are just text, data I don't I can't. Get any more information out that right now. So, that's where I want to go into power, query and make, that data more useful. So. I'm gonna go to my, query, over here and let's.
Have A look at this data so the review table, has, some comments in it who. Left a review when, that, kind of stuff and. I. Want to make this text, data more. Useful and get. More out of it so I can. Use the text analytics. New, function, in power bi desktop to, do that so. Maybe you've already seen this we had these, AI. Insights, in. In. Data flows before so that it's it's it's, already available there but, this this month we're also releasing it in the power bi desktop. So. I'm just gonna select text, analytics, and. You'll see all the functions that are available to you so, both text analytics and vision, use. Use. Models that are pre-trained. Developed, by as your cognitive services but, you consume, them purely in power bi so. They, do run on your premium, on a premium capacity. But. You don't have to have an azure, subscription. To to, run them, now. The third button and you see the Azure machine learning is. Different so you don't need premium for that you, can just use. All them at your machine learning models that have been shared with you or that you've created yourself and, just, apply those on your data in power bi, but. I'm gonna look at text analytics for now so, I, can detect, the language of a text I can extract, the key phrases so, what topics are people. Talking about or I can score the sentiment, so I'm just going to start with that now. As you see I have a selection. Drop, down here for, my premium. Capacity, so, I can select the one I want this to run um and this is just to run it to. Run it on during, the, evaluation, in power query so once I published. A report it. Will use the workspace, and the capacity, that I actually published it to. Okay. So I selected, this core sentiment, I want, to have, get the sentiment, of this, text, or. The Commons column and I. Can put, in a language, code if I know what, language it in or. Otherwise probably, I will just detect. That for me. I'm. Just gonna apply that and as, any. Other transformation, in power query it's adding a new step over here in the applied steps so you see that it has applied, score sentiment, over here and I can remove or edit that. Just. As any other step and just. In a few seconds, I now have a new column with the. Sentiment, score per row, add. It as a number from going, from zero which is the most. Negative to, one which is most positive. And. I can start using, that in my reports. To. Analyze it. Now. I can, I have done the same thing for the. Key phrases so. I've looked at all the columns, all. The comments and then extracted, the key phrases and what. Key phrases actually, does is it it's, already sort, of unbefitting the, table, for you so it's, now creating, additional rows for each of. The, key phrases so you can directly use it in your report. So. Let's see what you can do with that so. I had this overview, but, now with, the, sentiment, that I have I, can, now start looking at some different things so. First, of all I can look at the average sentiment. And how that sentiment is progressing, over time so, is it are people you. Know getting more positive, or more negative is, there at some area that I need to keep. An eye on so. You can see for, instance also can look at what, areas. Have a, higher, percentage, of positive reviews and, what. Areas have a higher percentage, of negative. Reviews so if there's one area with a lot of negative reviews I might want to look. Into that and see what's happening there now. In. The same way. With. Just the. Same couple, of clicks I also did, an. Image tagging, on the, images, that hosts. Have with the with. Their listings, so, people taking take pictures of their home and create the listing. Of it and I'm. Interested to know. To. Look at like what is what kind of images, are. Maybe, related to like higher sentiment, and lower sentiment. Reviews. So. So. I'm going to just filter on the, negative. Reviews. Over here and I've, listed, what, the most common tags are so, you obviously there's, a lot of pictures about on, indoor because people are taking, pictures.
Of The indoors and the rooms and all of that but you can also see. What tags, are related to low sentiment, comments, and some. Things that are that. Are popping out here is that are, like. The garden, and, plant. And. Also maybe Dec I can, I'm gonna select. And. As. I do that you can see that some there's, some listings. Popping up that have, where. People, say it was a very old house it was construction, it, wasn't, it wasn't great and so, maybe. I need to have, a look at you, know when people are posting, pictures of the outside of their house maybe. There's something wrong with the inside or you, know that's, some maybe some clue that I can dive, a little bit deeper in. Now. I could. Also use this, as an input so the sentiment, as an input for a. Key. Influencer, analysis. So here I've tried out a couple of things and it's. Getting. Pretty interesting, results so you can for instance see that when cleaning. Free. Cleaning. Fee is higher than $200. That. Seems to be an influencer, of a, descent, event to be negative, so, you, know maybe if people are having. To spend a lot of extra cost there may be more you, know expect also expecting, more and being more critical. So. Now just from that single column of reviews. That people left, I was able to look, at all these different angles and, see and, see what what people are thinking, and and maybe, get. Better at, understanding what, makes my, the. P my renter is happy. So. I'm just gonna switch, through the slides, and. Just. Keep, a couple. Of slides here do you yeah. Just like it's a quick call out because this comes up quite, a lot as well what should I use altima Mel power, bi when the should I use it in a sure so. This is kind of like a quick cheat sheet for that LT ml in power bi is aimed. At a different or audience it's really aimed for a business analyst Asher, it's really for data scientists data engineer, the, skill set is also completely different in power bi you need to be familiar with things like data flows and power query you, don't need to write any code you saw the stuff the me and Serena we're doing didn't require a single line of code it's all point-and-click. And so, in, Azure the skill set is much more things like Python you're gonna have to be actually writing Python code also. You may, want to consider where's the model actually living so in alto ml the model is operationalized. And lives, in power bi in the future we are working on some functionality, to let you export it out into a Jupiter notebook so it could live outside of power bi, but. Generally, if you want creasing that's really multi-purpose. And you're going to be calling the web service from different places you may want to consider asher and finally. Again from a licensing. Perspective for.
Power, Bi its power bi premium or power bi embedded. And. Ultimatum. Ellen Tauscher is a sure subscription. And. Similarly for for, cognitive services so. The. This is also really designed for the. Or. The power bi integration, is really for the analysts, that are working in power bi that, doesn't need an azure subscription, but it does run on your premium, capacity. So. And. You can and you can at any moment, determine. What capacity you want to you want to select there so same, as far as your machine learning you don't need any to, write any code just, use it out of the box and and apply it to your data. So. The. Main thing that we wanted to show you today. Is just. How we're using AI, to enable. All sorts of users in power bi to. Basically. Do more in. Less time so how. Can how can we help people to find insights. Quicker, and using. Ways that are very natural, to them by by clicking or by using, natural language. And. Also, give analysts, the opportunity, to extend. Their work with machine, learning models either by building them or by using the, out-of-the-box, models. So kind of bridging the gap between, maybe. These really. Busy. Data scientists, the, science, teams that don't have time, to, work. On every model that the analyst is, is asking, for but, for the analysts who go, and try that out themselves and, find, out what the relevant questions are and and just search. For those answers. So. That's. Gonna be basically. The end of our session we, have a lot of documentation, on. Tutorials. On how to get started on these features the. Latest power bi desktop. Released. Next week is going to have these. All. Of these visuals, and the, earth inside. A preview. Feature. And. Then if you want to get in touch and if you have. Any, use. Cases you want to share with us or questions, or, suggestions. Please reach out we're. Very, open to to. Talk so. Thank you very much thank you.
2020-01-19 17:58