Leda Braga: Data science and its role in investment strategy
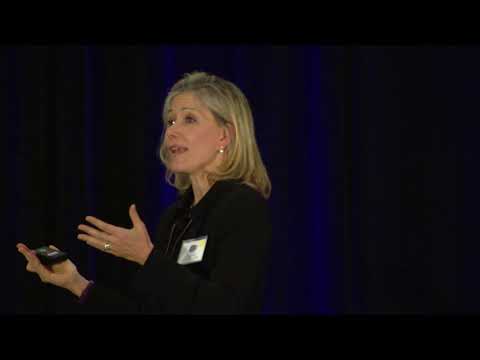
Thank. You good morning everyone thank you mango for the lovely, invitation Thank You Boonta Walter as well who who. Helped to invite me I don't know if winter is around I haven't seen him ah good. Yeah, so it's a fantastic opportunity to be here speaking, to you you know I I have a tendency, to try to rationalize, things. In my mind and I a few years ago I rationalized. Stanford. To myself and I thought Stanford. Is this place where, anything, is possible. And. If you and if you get a number of intellectually. Capable people, hard-working people to believe they are in a place where anything is possible that, is a very powerful thing to do and so to me it's, a pleasure it's a privilege, to be contributing, to this conference today and, you. Know in in preparing, for the conference, in in doing some homework I looked. At the previous speakers and the previous schedules, for the the other previous two conferences, and I realized that I'm the first financial industry. Speaker, in this conference, and. So, I'm gonna spend a little bit of time taking, you through what we do as investment, managers, that we are in a bit about our firm but, I I have, a feeling, that there's a reason, why they. Haven't been many financial industry. Speakers, it's because if, you, take us back to that percentage, that Margot was talking about the percentage, of women in the industry our bet with you the financial industry is right at the bottom it's. Going to be small single, digits I suspect. And so, you know that's something to change and hopefully in, today's, talk you'll get a feeling for what we do and how you can make a difference, in, data science in this industry and. So I. Also, realized. That a lot of the speakers here come from industry. Giants, like Google, Facebook, Intel. Microsoft, I, I, worked for an industry that is new to the conference, the financial, industry and for a much smaller company so I'm going to spend a couple minutes taking. You through who, I work for and what I do for a living. So. I as, you can see from the program I represent. A firm called systemic, investments. We. Are an alternative, asset. Manager, and, we, have been in charge of funds, systematic. Strategies, invest. And funds that. Go back to 2004. And so we have a long, track record I'm saying. We are an alternative. Investment. Manager, there's more to come on that in a minute, but basically out of the pool of people out there they manage, assets, that manage money for other people, the, alternative. Slice of that industry, is the, the state of the art the very advanced. Piece of the industry, we, are allowed to trade very, liberally, a lot of different instruments, we trade in and out of, securities. Very rapidly, very very, the turnover, is very high we, deploy a lot of leverage and so we are somewhat the, the leading edge of the investment, management industry. We, have about nine, billion dollars, under management in, our firm and we, are quite small in terms of staff we employ, a hundred and eight people across. Five locations, worldwide, but. We are diverse across. These 108 people we have 26. Nationalities. So that, is that is something to be to be proud of many, of us have PhDs, in in science and engineering impersonator. PhD in engineering and, I, was an academic for, several, years before I joined the financial, industry in the early 90s. There's. A little bit of a picture there is to put some faces to. The name so, that's who we are and and we in our mission statement we, openly, talk about employing, science, and technology, to, achieve returns. In investment, management now, then I thought you, know this is a data conference. Let, the data speak right, so I actually, computed, the word cloud on on. Three staff questions three questions, that we posed to staff. What. Do we do for a living and you can see the word cloud there we make money for clients pretty good, so. This is a 98, percent participation by, staff by the way so this is a good. Word cloud why. Do you work for systemic. Ah clearly. The environment, place, plays, a big part in the culture, and the, team and. Finally. Describe. The culture of systemic. Ax and, I'm very surprised by this but the word family comes up. And the. Culture, and excellence. Which is a value, of our company. So. So that's who we are so that's systematic, so we are an alternative asset. Managers they say there's going to be a little more about, this and I'm going to talk about systemic investment. Management so first of all let's, take a step back to think about what investment. Management is so. Investment, management is the professional, administration.
Of Various, securities, and other assets to achieve, specified. Return. Goals in specified, investment, goals for. Clients. What, so what what do we do we deploy, the capitals, of the world, right, and and. Where do these capitals, come from well there's a lot of big pools of money out there that need to be managed, you start with pension, funds so all of our pension money needs to be managed if, you think about insurance, companies insurance. Companies are in the business of collecting premium. And stashing. Up the money in preparation. For for. The need to pay out upon. Certain events, and so, there's money that the insurance companies, put aside needs to be managed. Sovereign. Wealth funds hold, the, wealth. Of various, countries and they need to be managed and, so each one of these clients will have different. Preferences for. Time, horizon. Commitments. That they have against, those monies that they need managed, so, some investors, have a longer, time horizon, than others some, investors, need a lot of return some. Investors, have restrictions, on what they can and cannot invest, and our job is then to to, organize, the portfolio's, that suit these investment, objectives, and we do that through funds, we set up funds and investors. Then come and invest, in funds, so that's what the the, investment, management industry, does, there's. About 80, trillion. Dollars, of, professionally. Managed money worldwide, 80. Trillion. Dollars, and in. How is this PI split. So. As I say to you that the majority of this money is managed, in a fairly, direct. And in, straightforward, way so. Much. Of this money is deployed in buying stock markets, I just buy the stock markets and I hope that, they will grant. Me the that I need or I buy, a portfolio. Of Treasuries. Of government. Bonds, and and, I hope that that will give me the return that I need the. Alternative. Asset. Management the alternative, slice, of the investment, management pie is about, 10%, of that 80 trillion so, 80 trillion is the big pie 10%. Of the 80 trillion about a trillion, is called, alternative. Asset, management and then, within the alternative. Slice there, are three, parts. There's real estate and infrastructure, private. Equity in hedge funds, so. Real estate infrastructure, private equity hedge, funds systematically. Lives in the third of the, eight trillion. Ten percent that is called hedge funds and as I say we are the state of the art we are the more innovative, part, of asset, management we trade, in and out of things with, with little restriction, with the ploy leverage, we. We. Have the freedom to to, innovate, and. So. The, activity. Itself in terms of how we deal technically. With the activity, there are two avenues of work one, is what, we call signal generation, and the other one is portfolio, construction so, every asset manager, will talk to you about signal, generation and portfolio construction signal. Generation is the process whereby with the side which, securities. Or which assets, we have a view on this, is a good buy this is a good sell this is this is going to go up this is going to go down and so, that is the forecasting. Part of the job then. The portfolio, construction, is once I've selected a, number of securities, that I'm interested, in trading and having positions, in then. How do i size, those positions how much of each and how, quickly do I go in and out of them and and, that is the bit of the exercise, where we really say well wait a minute what, kind of parameters am i working to here what kind of risk does my client require.
And What kind of time horizon, does the client have. So. So. So that that's what we do and and, the, the, game is in terms of data science. The, systematic. Approach to investment. Management is the one that, is really, like data science, right and and, the because we live in a world where all decision-making. Is becoming data-driven the. The press has really, become very interested, in systemic, asset management 10, years ago 15 years ago when I joined this side of the industry you wouldn't see any press on systemic, trading nowadays, you see it all the time there's headlines all the time now. If, you actually look, historically. The. The, the, big, famous investors. In the world behave. Like data scientists. I don't know if you care to to, truly, listen to what Warren Buffett had, to say I mean Warren Buffett is perhaps an, early, time data scientist. He's. A disciplined, man who looks at the data to make his decisions, and uses, the data and monitors, the data to support his ongoing decision, so so, I think you, know there is a lot of press there but, people are accepting. Systematic. Trading as a as a strategy. Now, what, what is it that stops systemic, trading becoming more widespread and. More dominant, I think, there's some amount of algorithm, aversion, so, what I'm saying is I'm gonna get to how we go about dealing, with data and the challenges, that we face but before that just to put it in context, just, telling you look, you know it's a very good approach we look at data to make our investment, decisions but there's a lot of algorithm, aversion, to to fight against, to battle with. So. Some, of the things that we hear I, like, systematic. But systematic. Funds they tend to be all the same well. I cannot really understand, the strategies, it's all rocket science, it's, black box stuff it's, less transparent. And discretionary, trading, and and, then you have to think discretionary, trading is the the, the part of investment, managers, where where the, the investment. Process is dominated, by human, decision-making without. The formality of data, science, necessarily, and so, you know one of the the slides that I've got here is just to say what does the data say so if, I'm here defending, the, data science. Driven approach. To investment management is, that a superior, approach is that an inferior, approach how does that compare. To the history, of asset management which up to now has been predominantly. Discretionary. And. So this is a study I'm coaching, a study by by a set of appears in the industry that, that computed. The returns of over. Nine thousand, hedge funds from. One of the key industry, databases, the the hedge fund research database, and those, returns spanned the period an almost twenty year long period, between 1996. And 2014. And, and. You can see that the results there what these guys did was they picked up the nine thousand funds and they use some natural language processing algorithm. To classify them, as. Discretionary. Systematic. Using, the description, given in the database, and then they computed, averages, and some statistics, on it and you can see that they ended up with four groups, systematic. Macro, funds discretionary. Macro funds systematic. Equity in discretionary equity. And. You can see that the returns, the average return, and this is excess return this is a return, over the the risk-free rate the. Average. Return, is similar, across all four, categories and then, another, exercise, that they did was to try to explain. These returns, using common, factors, and common. Factors have to be. Securities. Or types of portfolios, that are easy there are obvious, that are easy to deploy, and so, they they did a bit of analysis, on that a bit of regression, there to try to explain, how much to. Assess, how much of the returns of each category could be explained, by simple factors. And again. Then what you're left with once you've explained, some of the returns using the simple factors we're going to call alpha, we're gonna call skill, we're gonna say look this is really what these funds have delivered, and so there's an alpha, row. There and by the time you've accounted for the easy. To explain, piece of the return the, returns look even more similar, and then. Finally if you normalize, by the return, volatility. So how much risk did I take in stomaching, those returns, then. The, ratio at the bottom is quite comparable, so. So what is this slide actually here, to say the slide is here just to give you the message that the, systematic, approach to investment. Judgment is about the same maybe a little bit better than, the discretionary, approach so so, there's a lot of future. Promise. In in this approach. And. So it's okay so later you tell me that you, you work for this company it's state-of-the-art, investment. Management you're about deploying the pools of capital of, the world and and, you tell me that up to now the world has been predominantly doing.
That Job in a discretionary, manner by, opinions, and by people looking at data in a in a perhaps, in a less objective, way so. So what is the difference then what is the difference between this systematic. Investment, management approach, in the, more traditional. Discretionary. Approach well. The this graph is about this this difference and the first thing that I need to say is that this difference is going away huh the two approaches, are merging and and, in future everybody, will talk about the, systemic, approach because because, the discretionary, guys have realized that that, they are at an information disadvantage. So, they're trying to join us and and, integrate, their, their world with ours but, but in any case historically, this is the main difference the, level of diversification, so. The if you listed, all the trading opportunities. In in order of trade conviction. The. Discretionary, guys tend to trade to the right of us systematic. Folk so, that means that they have fewer. Trades on in higher, conviction. On their trading, we. By contrast, have lots, of trades on and not, so much conviction, on each trade that we have and, and. That is the the main difference now what is the implication, of that you, know I I this, is a highly. Science. Oriented forum. Here so I don't need to explain this to you but I can tell you that that, most people out there don't think about these things I used to ask this question to, motivate, the thinking about diversification, I used to say to people listen, you know if, I tell you that you're gonna toss a coin for your life and, if, you get heads you survive if you get tails you. Die and I, offer you one throw on a very. Highly, biased coin, towards heads because actually I'm nice and I want you to live or I. Offer you a truckload, of throws, on a less. Biased coin, we, to undo you take and. Most people think that the highly biased queen but that's not the answer you guys are technical you know that so, so the game here is this how bias does the coin have to be towards. Heads to, achieve a positive outcome, to achieve on average, more heads than tails, say. With 80% probability and, the, answer is if you have a lot of throws of that coin then, you only need a little bit of bias right because you've got simple limit working for you so, so, that is the game that we play the systemic, crowd tends to have a more, diverse set. Of trades, on and and. We don't focus quite so much on getting each trade right, so. In other words by diversifying, the choice of trade opportunity. The, systematic, approach makes the investment process less. Reliant, on the. Random nature of forecasting. And more. Reliant on the risk control, in the portfolio construction okay. That is that's the key insight now having said that if you think about investment, management the, glamorous, part of investment management is forecasting, this is what gets people the headlines like if you call a market right if you if you if you call, a big event that that is what is glamorous.
About It but but, but our approach is very robust, and very scalable, and very auditable, as well, okay. So having, said that and you're now pretty, sold on the idea that that, systematic, trading is very good it's very auditable, it's it's a great approach and it's going to grow to be the majority of the, assets and the management, are there then, let me get a bit more program I'm more practical, about what we actually do, on a, day to day basis, so in terms of signal, generation that's the forecasting. Problem right so then, we're trying to look for factors, that might tell us if a, particular, company, or a currency, or the stock market of a particular, country is going to do better or worse in the future so, we're trying to forecast, what, do we do we look at also two data price, and volume data, are key we always start with price and volume we, perform a lot of regressions, you know various, flavors, of regressions. Natural. Language processing is. A great discipline, for us because it enables, us to parse they. And, unformatted, in, unformatted, way so news, and events, and company. Filings, and and in general, press. Material. Occasionally. We work in the frequency, domain to try to assess a signal, generation and then, at the portfolio construction side, of things what do we do there there, once you've decided which securities, you have a view on how, much of each one do you wanna own how are you going to construct, that portfolio what. Are some of the techniques there we use volatility, estimation, techniques, the. The problem, of portfolio, construction. Is a constrained, optimization problem. So so, we deploy all the techniques there we. Do a lot of matrix, manipulation. Because you could think of the stock market as this multi-dimensional, world. Described, by all the stocks in your traded universe, and then. There's another problem, that we all deal with as well which is the problem of executing. The trade so once, you've decided you need to buy this many shares, then how do you go about buying them so, that you don't move the market and you achieve good execution prices. And I'll take you through that in a minute so now so let's, get concrete, now and let me take you through some examples, so. Execution. Algorithms. So. This is an area where data. Science, really rules and and, and I think execution. Algorithms, were the early part of data science, in in investment. Management because, everybody.
Everybody, Likes automated. Execution, everybody likes algorithmic, execution, in particular, the regulators, feel very. Comfortable. With it because they can check how, how things are being done and and and we, have a very, direct way to monitor, our participation, in the market so. There's a slide. There just to talk a little bit about how, you can go about executing, a, trade throughout the day so if, you've got a thousand, shares to buy you. Can do what we call a V wop execution. Of volume, weighted, average. Price execution. And so, what, you do then is you you take a little slice of every, bit of volume that goes through throughout, the day and you try to complete your transaction, while. Staying in parallel, with, the volume traded, and so, if you up is a very. Safe and a no-brainer, way to execute, but it doesn't really optimize. Any advantage, you might have of the information, of, intraday. Behavior, so what do we do we, study intraday. Volume trends, we try to establish whether volumes. Are higher in the morning if they're seasonality, in, the day and you try to time. Your flows to, take advantage, of that intraday. Seasonality, we. Also look, at the order, book information and, in the order book is this, a great. Big list of people, who want to buy in to sell and so, there's a bunch of people who want to buy and each one will prepare, to buy at a certain price and a bunch of people that are prepared to sell each one is prepared to sell at a certain price. Analyzing. The dynamics, of that order book like, how many people do I have in each side and do I have a lot of people wanting to sell and not a lot of people wanting to buy and other, people wanting to buy very spread out in price are they, arriving faster. Into the order book chatroom, than. The people that want to sell all that is very useful information, about the price, dynamics, of the day and we use all that as short-term. Signals, for execution. So. That's execution. Then, a couple of comments on how big, data can be used for, investment, I. Mean, here this is just a slide to to highlight, the fact that when, it comes to exotic data, provision. There. Is a dynamic. In, the marketplace, whereby, the, first level processing, of all, this exotic, data, is typically, done by a small company and there's a lot of startups, in that field so people, that will deal with their processing, imagery. On a. Shopping. Mall car park activity. Or the. Shadows. Of reservoirs. Of oil. Or of gas to extract, information we don't tend to do that first pass information we, tend to buy that, pre-process, data but there's a lot of small, companies doing, that and we interact, with them all and, so, you know a couple of examples, of how we, can use big data in investment. So. Suppose I have the the following investment, thesis, large. Stock, moves are legitimate. When backed up by professional, participation. And they. Are ephemeral they are bound to disappear, if they are backed up by retail, participation. So that's the thesis right and so, I can then assess. Every, time a stock moves up by, a lot or down by a lot I can ask myself is that a legitimate move or is that a move that will disappear, and I should bet against, it well. One way to evaluate that, is to look at the activity on. Professional. And retail interfaces. And so, we tend to buy that data we buy it from technology, companies, some, of it we process ourselves some, of it we buy and we with, the post-processing, of, that data but, trying to assess, whether. The, retail has been active, entails, for example, monitoring. A number of wikipedia, pages and and. All the mapping, that goes through that you know if you're interested. In Apple stock which, Wikipedia, page are you likely to look at well, there's several that, will that, will relate to Apple stock and so all that data mapping, and and, in relationships. Between data needs, to be addressed, and so this is just a chart of an investment, return and you know the scale is not really relevant but this is just showing that that, applying, this investment, thesis using. Some, exotic, data to assess the, level of professional, activity, versus the level of retail activity does. Work that that does produce a. Gaining. Strategy, then. Another example. Classification. And grouping of companies, you, know there's lots of phenomena, out there that, that, stock, markets, do display, that have to do with groups of companies I mean in particular certain, groups, of companies tend to move together and, if a particular company deviates, from the group the the, price action tends, to be of mean reversion to, the, the.
Group The pact again and traditionally. You look at sector, classifications. To group, these companies sector. Classifications, are somewhat limited apart from anything because given. A sector, a company, either belongs, or doesn't, belong and so, an innovative. Way to do sector classification. Is to do natural, language processing on. That, based information, news, or company. Filings, and group, the companies according, to, how they describe themselves or, how they are spoken about and. So again this said this is a child a scale is not very relevant but this is just showing the same signal. Applied, on a traditional. Company grouping, in an, alternative. Company, grouping and you can see that the performance improves. So. Okay so. Systematic. Trading, use, of big data execution. Algorithms. Enhancing. Investment. Signals, using exotic, data the, other question that I often get is artificial intelligence how, is that changing the landscape and. Then, again the, dream there is that of autonomous, investing, right everybody thinks that you know with the learning algorithms, I can throw. All the data at the algorithms, and the algorithms will tell me how to make money well. Unfortunately the, biggest crime, the biggest sin. Of. Systematic. Trading is overfitting. And a, lot of these learning algorithms are very very, rich models, they are very rich in parameters and in structure, the, financial data is by contrast quite sparse, and limited, and if you think about. Financial. Stocks there's maybe four thousand, five thousand, investable, stocks to, a certain size and you, get one, price point. Per day in a daily time series you might get a tick level time, series of prices, but that, that investment, horizon, is quite tight not not quite so easy to to profit, from and, so. The. The idea that you can throw, the. Data at an algorithm and the algorithm, will manage money for you is still very far I think. The, the, more likely, scenario is the scenarios, that I've described, where an investment.
Thesis Is derived from economic, observation. And then, we look at the data to enrich the, expression, of that investment thesis into, and to make it work and. Then. Finally, I wanted to talk to you about ESG. Investing, and I I think, I hope I can leave you with a good message on this I mean, ESG, is a is, a big, topic it's, coming to - everybody's, mindset. Yes, G stands for environment. Social and governance, considerations. In investment, and. More. And more people. Are becoming aware that how they deploy their. Monies which companies, they invest in, sends, a message about what they think is sustainable, what they think is right what they think is proper governance, in, particular, the UN, has published, years, ago, their global, goals and from. The UN's global, goals the, principles. For responsible, investing. Came out. Systematic. Is a signatory, to the UN PRI. And what, do we do in that manner, several. Things that we do so for example in. The in the in in, the terms of screening, so that's the first step of ESG, investment, is to say look, I am an investor, I want you to build a portfolio for me but I don't want you to include stocks that do certain things I don't want you to include tobacco, or I don't want you to include gambling. Stocks in the. Mix so, then what we can do with the systematic, approach is we. Can actually rebalance. The portfolio to. Compensate, for those exclusions, and then we can perform the exclusions, on a systematic, basis then. In terms of alpha, generation. The. The the practice. Of finding. Companies. That behave well that have good governance, should, give you some alpha, sources. And then finally, impact, so, can you let the companies, know that you're investing, more in them because, they have good ESG. Practices. And. So then perhaps, the most important, slide of this presentation I, was watching, some. Of Margo's, videos about the conference, and she was saying something that Maria said this morning as well that, you know you you want to join the data science, community, and make a difference because. So many important, decisions, are being made on, a data-driven, basis. So, this 80 trillion. Dollars, of professionally, managed assets in the world, diversity. Plays a big part in, that you need to be at that table and I just wanted to quote for you a. Statistic. That I found that, in. 2015. Following, the Paris climate. Meeting. The two years that followed the Paris climate meeting saw a 55%. Annualized. Growth rate, in assets. Deployed, under a sustainable. Mandate, so. Now, we have, a lot of diversity, coming, to the fore through ESG, investment, and through disclosure, rules, from. The the, various regulators, so, what is that going to do to to, the investment, landscape and, then finally, a couple found, final, slides something, to say in this forum if you're contemplating a career in investment management if you like data science, exotic, data and and. In looking for investment, management it, would appear that the data supports, a good, track, record for women so the. The. Data. Based providers that do, analysis. On female run funds report. An out performance, I have to say the sample, is quite small and the time scale is short but but, it's a positive, but. It's it's. A positive message. Anyway, and. Then finally just a close I just want you to go home remembering. That systemic, investment, management is data, science. Applied, to investment, think, of warren buffett as a beta scientist, from now on and this. Approach is at least on a par with the. Human approach but perhaps more, scalable and more disciplined, there's. A large element of randomness in markets, relatively, sparse data so, learning algorithms, have limited use you have to really watch out for overfitting, overfitting, is a big risk, 80. Plus, trillion. Dollars of managed assets globally.
So, This, bit of data science, has a lot of power if you want to change the world you've, got 80 trillion, dollars there and to, change, the world with and ESG, investing, is the future so let's I try to join forces in shaping it and. That's what I had. We. Have just. Two, minutes or so for some questions, so while. You're thinking about a question let me start. It off first, of all I just wanted, to say I love that statistic, on women, outperforming. Men because, it just shows you how far chable data is, I mean you can always put it in the way that read if you choose. Have. You thought about high-frequency. Trading, or you have any any thoughts. On that it's very popular yes so so we do high-frequency trading, in the context, of executing. Trades so. So so, if you decide that you need us to buy a thousand, shares then, going, to market entails, looking. At tick level data we've had some success, of neural nets in that application but. But limited, so far the, the problem with high-frequency trading is the capacity. So 80 trillion dollars is a very large amount of money I mean institutional. Investors have very large tickets, and the, the horizon, for investment, needs to be longer when he. Says ok question, yeah, thank. You for talk I really enjoyed it I have, a question do you feel like the. Business. Insight, or, financial. Background are. Prerequisite. In your area, or you feel the data science part is more of a heavy lifting, compared. To, the. Business. Insight itself, I don't see you can be resolved ah. Good. So. Look. I I'm. An engineer, by background I. I, was, never particularly, interested. In financial markets, you know I'll be honest about it I was interested in I was, an academic you know why I had, my position, and I was teaching away and doing research I, I. Think you know as part of the job of course you do become aware but but you know you you. As a good professional, you want to understand. The environment that you're in and so I've done, plenty of studying of you, know microeconomics and and in news. And and, and other bits that affect markets, but but in answer to your question if, I'm anything to go by then no you, know I think a passion, for data science, and and an, interest in making a difference again, I think you, know there's something to think about is this look, you know if you want to change the world, Park your money in the right places and if you think about Investment Management as this, activity.
Whereby, The pools, of capital of. The world get directed, gosh. That is so powerful and if that, is going to become completely, data-driven overtime, then, you can't miss that opportunity you've, got to join in and and and, then have your say great. Well thanks very much leader.
2018-04-17 10:27