Building Data Science Into Your Strategy
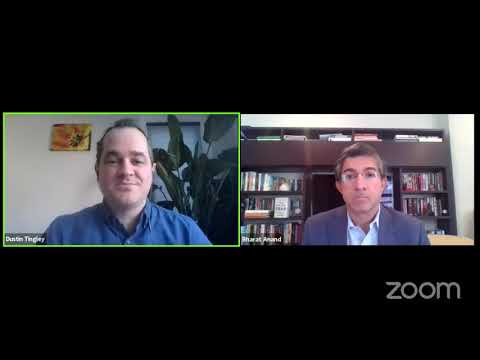
OK well welcome everyone. It's good to have you all join our live webinar this morning. And we have, we just wait about 15, 20 more seconds for everyone to join. It's a pleasure to be here with all of you. OK.
So my name is Bharat Anand. I'm a faculty member at Harvard Business School and also the Vise Provost for Advances and Learning at Harvard. And it's a pleasure to be joined this morning by my colleague Dustin Tingley. Hey everyone. I'm Dustin Tingley. I'm a Professor of Government at Harvard University.
And I also work to help direct a range of teaching and learning initiatives at the University. And finally, I'm a data scientist. So thanks for joining, Dustin, it's-- I'm looking forward to this conversation to talk about data science and the value in so many different arenas for individuals. But I have to say before we get into the conversation, I'm just excited about this new venture, in effect, that we're starting on, which is sort of thinking about big topics, quote unquote, as we see them today. Whether it's data science and digital transformation, whether it's health care leadership, whether it's climate change.
And we're really embarking on a new path now when we have obviously the HBSO platform, which many folks on this webinar have probably taken courses on. But also inviting faculty from across the University to create courses on this platform. And your course is going to be the first in this new domain so I would love for you to just describe a little bit about this course.
It's called Data Science Ready. Yeah, super exciting. Thanks Bharat. So Data Science Ready is a code and math free introduction to data science.
And so maybe it would be helpful to kind of think about the two main buckets of classes around data that are, for the most part out there. The first bucket would be classes like the amazing business analytics class offered by Harvard Business School online already. And those classes are largely more statistics oriented classes. They're going to help you think through how to do analysis and things like Excel.
And are very, very helpful. But they're very statistics oriented. Regression, distributions of data, these sorts of things. The other group of classes are your more standard data science classes that are going to be lots of math, lots of programming. They're really great courses, but they're really anchoring around how do you become a data scientist proper.
How do you code in R, how do you code in Python. Things like that. That's not what Data Science Ready is. So Data Science Ready is a course where we're trying to equip people to understand the data science landscape and be able to effectively work with data scientists or business analysts or others who are using data. So we get into questions about how do you make your data science team be doing things that are actually actionable. Or how do you think about protecting privacy in ways that, if you don't, you could face legal liability later.
Another big part about Data Science Ready is helping everyone see that so much data that is out there and that is being used by companies, governments, et cetera is not neat data that sits squarely in some sort of Excel spreadsheet and there's all numbers. Instead there's this huge range of data out there. Words, images, sounds things like that are all being leveraged and using advanced algorithms to do so. But it's not the sort of standard kind of Excel type setup. And then finally we actually spend a lot more time on cause and effect, right. And so I'll take an example.
Do putting ads on Google work? Right, that's a big question. Lots of people are spending lots of money putting ads up on Google. But it's actually kind of a tricky thing to evaluate.
So again, in Data Science Ready, we're really looking for ways to make sure that everyone within an organization understands the promise and perils of data science. And so we're really excited to offer this. But of course, this is just one class in a sort of a collection of classes that we're working on.
Bharat, what are some of the things that you're working on in that domain? Yeah, Dustin. So I know there's going to be I think, what do we have, 17 courses coming up in this digital success series. And yours is the first that we're launching at the University.
There's going to be several, both on the data side and the digital side. So I can speak a little bit to what's happening on the digital side, and then maybe you can offer some insight into what's following on the data side. On the digital side, well I'm sort of coming back to creating an online course after seven years.
And will be called most likely Digital Strategy or something to that effect. But the idea is what is it you need to know, at the very least, to be able to be successful in digital arenas. And you know I mean, I guess another way of saying it is what does it really take to be customer centric in a digital world.
And the examples are going to be fascinating. We're already starting conversations around these. Startups, establishing companies around the world.
But there's follow on courses that are more vertically specialized as well. There's one one digital health, which is looking at digital transformation in the health care ecosystem, which I think just given the changes of the last nine months is fascinating. There's one on digital cities, which is looking at smart cities and sort of how do we think about, what is the impact of digital technologies on urban design planning. So those are some of the courses there.
And then we'll have courses in digital marketing and AI driven organizations and so on. But what's happening in the data side? Maybe you can explain a little bit. Yeah so I'm super excited about classes beyond Data Science Ready. So we've got Data Science For Business course that's going to be launching in a couple of months. And that course what it's doing is it's starting to help equip people to be doing a little bit of the programming. Getting their chops ready in things like R. But all
in a business context. So you'll be learning about some of the models, some of the algorithms that are being used in business today. But getting some actual programming jobs.
So that's called Data Science For Business. And that's going to be launching in a couple of months. We've got a course that is entirely around privacy in the different regulatory frameworks that go around that.
Which is just super important no matter the country that you're living or working in. Especially in this world where everything is spread out globally. So we're really excited about that.
And then another example is of course being taught by a very famous economist that's about how do you use big data for social good. And that's a really exciting class that especially as companies start to up their social responsibility game, they can start to think about how data might be used in a way that is really exciting. So if you haven't yet enrolled and paid to join the Data Science Ready offering that launches in a couple of days, please do. And absolutely applications are open for the next wave of data science that will be happening in April. So I'm looking forward to having all of you join the class at some point.
And there's a couple of questions already in the chat, Dustin, around when are these courses launching. So Dustin's course, I think the first launch happens this month. And as Dustin said, for those of you already filled out the application as of yet to pay, there's a couple of days more. The next one is in April. And each of these other courses is launching subsequently. So I think the Data Science For Business is launching in March.
And then roughly every month to two months, we'll be launching courses. So just look at the website for both HBSO and Harvard Online as it comes online. And we'll keep you informed. Dustin so just to take stock. So let's actually just talk about data science now.
What I'd like to do is maybe spend about 20 minutes or so, just chatting about sort of data science writ large, and how we think about the value for organizations and individuals. And then we can open up systematically to Q&A for the last 15 or 20 minutes. By the way before that, let me just make sure that chat is open. So I want to engage in a conversation with everyone through chat here.
And to do that, let me just have everyone maybe use chat to put in an example, your favorite example, of how a company is using data today. OK what's your favorite example of a company that's using data well. And what's the concrete way that it's using data that you have in mind.
OK. So if we can just, if you can put it to chat. And we'll have a look at sort of what folks are saying.
All right. So we're already getting some examples. And Dustin, so there's surveys obviously. There's a few already that are talking about Amazon and recommendations, and we'll come back to that. Netflix as well.
I love this, remote control towers for supply chain and ops. And I know you have some examples on that side of things. Amazon, Amazon, Google, credit. That's interesting. So those of you who remember Capital One from the Econ for Managers course. That's a really interesting example.
Mobile operators. Google, Netflix, Spotify, Amazon. So you see the usual suspects here, Dustin. And this is a great list.
By the way, hold on to your example, OK, because I want to come back, come back to this. But in the meantime, also if you can write down in chat. So these are all examples of use cases where companies are using data in particular ways.
Indicate whether you know where the companies are actually getting the data from. And how they're building the algorithms to be able to use this data. Just indicate that in chat. Or keep that in your mind. That will be, that's a question I want to come back to.
While I am I doing that Dustin, what's your, what are some of your examples of uses of data in organizations that you've seen as surprising. Yeah. Thanks Bharat. And thanks for all these great examples. I see things like Spotify.
We actually are going to talk a little bit about in the course about how Spotify makes recommendations about sound, about music, which is really interesting. So when I look out there, I mean a lot of times people focus on things like Facebook and how they make targeting advertising as possible or how Google enables any number of things. But there are just so many great examples out there. Here's a fun one. So Chick-fil-A. Chick-fil-A is an American sandwich company.
And it turns out that they have a super sophisticated data science operation that is built into the core of their operations. And they do lots of different things. But here's one of the projects they did. So they had a set of products that they would sell that aren't their main thing. You think about like little containers of milk or so on and so forth. That what would happen is that the cashier at the front of the store, any time that that would be ordered, they would have to go to the back of the store and grab it and then come back, right.
Because they didn't really have space in other places. And so they wanted to be able to, how do we reduce the amount of time their employees are just walking around. Because that's making customers wait in line. And so what they did is they did a study to essentially figure out what was the optimal size of a little micro refrigerator that they could keep up at the front that would reduce that amount of time.
And so that's just an example. This is a fast food company that's highly leveraging data. Another example Bharat that comes to mind is a company called Stitch Fix. Stitch Fix is a company that is making recommendations to people about their clothing.
And Lord knows my wife wants to enroll me in a lifetime membership for this sort of thing. But it's really neat. Not only are they recommending things like clothing, which are going to be super hard. It's not like the type of thing where you have a lot of data about books or search record, search engine history, et cetera. But they went a step further. How do you match an individual client with the warehouses that should be serving them.
Because if the warehouse that has the clothing that that person would want is like super far away, then that's a problem. And so they're optimizing matching you as a customer to the actual warehouses that then would be your optimal supplier. And they've really baked in data science throughout their entire operation.
They have a really cool blog, as well, that talked about all the different ways that a clothing company is using data science. So those are just two fun examples that I've come across that are really exciting. That's actually neat, Dustin.
I mean it's interesting. That Stitch Fix example actually reminds me a little bit of Netflix, in terms of what they were doing way back when they were in the DVD business. What is interesting is they have like these 40 plus fulfillment centers around the country. And each center actually stored a different stack of DVDs based on local tastes. But one of the things that was interesting was when Netflix gave us recommendations on what you might like. It wasn't just based on your purchase history.
It was also based on whether the DVD is stocked in the fulfillment center that is closest to you. So they could get it to you fast. And I remember teaching this case once and one of my students said, God I feel cheated. I thought they were giving these recommendations based on what suits me best. But that's a really neat example. By the way we're seeing some great comments in chat.
So I want to pick up on one question, which is, like we're seeing in chat, data can come from various different sources, like you were saying earlier as well. Right, it can come from purchase histories, spending habits, search, claims data, all kinds of things. And then you have the output, which is how is data actually being used to help customers or suppliers. But in between, there's sort of a black box, right.
Which is how do we take this data and use algorithms to then make sense of it. And you know I think it's fair to say that most of us sitting in organizations don't really know what exactly that algorithm is, right. And in fact, as we talk about machine learning, it gets even more obscure. So my question for you is, let's say there was an algorithm that helped you make better decisions.
Like hiring or pricing or recommendations. But you didn't know why the algorithm was actually making the recommendations it was making. Should you use it? Wow.
That's a great question. Yeah so we've got an algorithm. It's making, quote unquote better suggestions or whatever for you. But you have no clue why it's making those recommendations. And look, this is a great question, because this is actually very common. This is, you will get vendors that will essentially sell black boxes.
And then the people that use those things don't really know what's going on. That's a good question. So you know, first of all, let me just maybe visit some themes about from the Data Science Ready that might kind of weigh in on that.
I think the first is it's really important to be aware of the full range of data that can be fed into these algorithms. So just going back to your comment about like what are the inputs. And the thing that we're seeing today is that range of inputs that are coming in and feeding these algorithms has immense scope. We already talked about text, search history, what you might sound like, even, what you might look like.
But notice that when we're then really expanding out the breadth of data that can be used as an input, we then have to be really careful about some things. So for example, what if this algorithm is actually introducing bias. And that bias is coming from the way that the algorithm was trained, on such that it's creating racial bias. Or it's creating bias based in sexism, et cetera. And so you really have to be aware of that. And if you've got this like black box going on, it's going to be kind of difficult, right.
And so I'd be a little bit-- I think we just have to be aware of that. I'll give you a quick example from actually a colleague of mine. She once typed in her name, she's an African-American computer scientist. Typed in her name into Google. And she would get bail bond ads.
And she was sitting with a reporter where this happened, and the reporter was like, hey, wait a second why are there bail bond ads coming up for you? Were you ever arrested? And she was like, I swear I've never been arrested. It turns out that the search engine was basically serving up disproportionate numbers of bail bond ads when names that were more traditionally African-American sounding were being populated. Now the people who designed that algorithm weren't intending for that to happen, right. But nevertheless it did, and they had to do something about it.
And that's not the sort of thing you want your company to have to deal with. So I think just in Data Science Ready it's just important to think about the range of data, as well as whether what the algorithm is doing is going to introduce bias. But you know what. That doesn't really answer your question. Yeah I was just going to say, I want you to answer the question. Oh and by the way in that example, I mean that example was very troubling at the time, right.
And our colleague, I mean this is a famous example, Latanya Sweeney actually turned it into a big research agenda as well. But I want for you to answer the question, would you use it? Yeah, so should you use it? OK so, I'm going to still dodge a question a little bit because it's a big question. It has lots of facets to it.
So I think it's important, first and foremost, to actually evaluate the claim that the algorithm was actually helpful for you. You know you get a lot of vendors and others that will come in and say, hey, this improves what you're doing. But when you actually look underneath the hood and ask for evidence for that, you get a lot of like well we're using complicated neural networks or something. It's like no no no no.
That's the algorithm. I want to see the performance. So I think it's first you have to actually evaluate and say, does this provide performance. OK. And so let's just stipulate that it does.
I think then, the heuristic I like to use is would I ever have to explain the decision to someone, OK. And so there are certain applications. So for example, the design of microchips or the sort of artificial intelligence that goes behind self-driving cars.
You know what. If the self-driving car doesn't cause, crashes then I'm sort of OK with it, and I don't really care all that much about that black box. But if it's ever something where I might be called on to explain a decision, which quite frankly, is very common, I think about hiring, thinking about pricing. Then you're running into trouble. Because your explanation can't be, well, my black box gave me something.
And so I think it's just that level of interpretability and that level of explanation is going to be really important then. That's really helpful Dustin. And we're getting some great comments in chat, both about this notion of can you explain it to someone else, depending on what the use case is. As well as the biases that creep in oftentimes.
I'm noticing one from Tommaso Pandula, who was actually one of the learners who took Economics For Managers. It's great to see you, Tommaso. It's great to see you, Tommaso, and many others as well. So just to stay on this for one, for a little bit more.
So if I'm a consumer of data. Let's say I'm a manager in an organization, right, I have many of my colleagues who are data analysts. They come to me and they say, we've got this awesome algorithm that's going to generate whatever recommendations and other outputs that might be helpful. Does the course talk about sort of what kinds of ways I might be able to sort of approach this particular question about when or when not to have more or less faith in the data of the algorithm? Yeah so a couple of the units definitely give some insight here.
So I mentioned before that we talk a little bit more about cause and effect than a normal kind of data science course. And I think that part of the purpose of that is these algorithms, this black box, it might just be surfacing tons and tons of correlations. And those correlations are actually correlated with success. But then, you're not learning what's driving success.
Like what is the causal thing that you and your organization could change in order to then have that broader impact. And so it might be that this algorithm is increasing your performance by 10%. But if you actually understood why it was doing that, and then you as a manager that knows your business that knows your industry, says, oh well these are the things that we then might change in order to reorg how consumers are coming into our website, et cetera, you might then receive a return on investment of 5 times that. So I think attacking that is really, really important. It's a good point Dustin about the cause and effect. You and I have talked about this in the past.
And sort of, if you have a causal problem with 1,000 data points, that cause and effect problem is not going to go away with 10,000 or a million data points. Big data doesn't solve that problem. Big data doesn't solve that problem, that's right.
Yeah, that's great to hear. So what are in your minds some of the biggest traps that companies fall into when they're trying to be more data oriented? Yeah so I think there are a couple of traps. I'd say the biggest trap is people way too early getting super excited about collecting and processing huge volumes of data, just because we can.
Right. The cloud came along and now and all these technologies. And it's just like, yeah give me the data, give me the data.
But what's the purpose, right. And so a big part of the course is helping people to think about how do you define a problem that then is answerable with data science type tools. And we actually give some examples, some real examples in the course, about where organizations made huge investments and the output of those investments were not things that were actually actionable, and hence, they didn't solve the problem that they were trying to solve in the first place. I'd say the second common mistake, and this actually goes to a point that a couple of people have made in the chat. Another big mistake I find is that firms will separate the data scientists and all the sort of quantity type people like me and they'll put them over here in this silo.
And so what does that do. That means that the actual managers and decision makers, they don't understand how those operations work at a high level. And of course like Data Science Ready is designed to help them understand that better. But you're siloing these people.
And so that means oftentimes that they don't even understand the company that they work for. I once was out in the Bay Area at a large company and talking to the chief data scientist. And he's like, look, I simply don't understand how data science plugs into the rest of the organization other than my producing these reports and hoping that they carry some weight.
And so that's what I call a one sided communication. But it's actually two sided. And the two sided piece is really important because the data scientist that we have in our organizations, and I direct a data science team and this is a problem there, too at times. They need to understand business and economics.
And so there's a two sided thing that sort of because we're siloing these folks over here, the managers and the decision makers don't really understand how data science works and its promise and perils. And so they don't ask really good questions to begin with. And the data scientists themselves don't understand the industry. And so I've got a dream that which is basically every data scientist has to take your class Bharat, Economics For Managers.
And every manager has to take my class Data Science ready. And that's going to that sort of thing to get that two way communication that I think is just it's just really important. And I oftentimes see organizations, government, industry failing in that regard. Yeah that's a cute example, Dustin, but I think the broader point you're making is actually an important one, which is it's not just a question of hiring the smartest data scientists.
But it's also what's the lines of communication that bridge the work that comes out of that with the rest of the org, right. I apologize, there's some background noise going on here with my printer. But let's just stay on this for a second, and by the way, my own thoughts of this are that this question of problem definition is a really important one, right. Because the data is only going to be as good as the problem that you're starting with.
If you define the problem as, I want to figure out like what's the optimal color of my product. That's great. You can use data science.
But that's a very different question, and perhaps far narrower, than saying how do I build deep relationships with customers, right. And I've seen this an organization, that's sort of the data is only good as the problem you're starting with. And that's an important question.
The third one that I typically have seen is sort of this, I wouldn't say confusion, but sort of blurring the lines between what I would call data advantage and competitive advantage. Which is organizations often tend to think that data is the Holy Grail, right, someone said in the chat. It's sort of going to solve all our problems. But it doesn't solve the need for coming up with a good strategy in the first place. And I want to just ask you this question, which is, I mean I spent most of my life talking about strategy and digital strategy and data strategy. But how can individuals, organizations, consumers be more strategic about data science.
What are some of the things that come to mind for you? Yeah so I think there's, maybe my first comment is a little bit less strategic and a little bit more tactical. And that's to say that I think there are lots of ways that companies can engage with data science without going full bore into super big data, super advanced machine learning, artificial intelligence, and so on and so forth. And it's really more operational things. I'll give an example, which is that the manual process of people updating spreadsheets and then doing some sort of analysis, then copying and pasting some sort of plot into a Microsoft Word document or PowerPoint slide, then delivering those slides to someone when they read it. That entire process, which is a huge part of many business processes, a lot of data science principles are around how do you automate that.
Right. How do you automate not just the collection or sensing of data. How do you automate the detection of anomalies, such that you're getting quality data in, or you can be more confident you're getting quality data in.
And then how do you automate the actual analytics that go around that. And then how do you automate the generation of visualization, et cetera, that comes out of it. And so that's like a very small pipe line.
And it does not require this sort of huge jump in order. So I think from a tactical perspective you can just think about, hey we can get into this data science space without having to hire 30 people. Now some companies need to do that.
They're dealing with higher throughput data. You're going to need a team. You're going to need data engineers, not just data scientists. And so I think that's, you have to say, look, where are you in your competitive landscape to be doing things.
But there's a deeper question around strategy. And this is one of my favorite examples. We actually don't cover it in the class, but all of you here today get to hear about this.
So a couple of years ago, I was hanging out with a data scientist that works with a Major League, excuse me, a major basketball team in the NBA. And they are telling me about, OK, we've been doing all this analysis. And our recommendation is that our players should shoot more 3 pointers. And it was a super elaborate, very well done analysis. I mean the person is really smart, taking in lots of data. And so the recommendation is yeah, they should shoot more 3 pointers.
So for those of you who don't play basketball, that's just when you shoot the basketball further away from the rim, you get more points. So you should shoot more when you're further away from the basket. And so then I ask the question, I'm like, wait a second. What happens when the defense changes their strategy? Right? And so that's part of the thinking that you need to have in mind. Even if data is informing some sort of strategic choice that you make, you have to be mindful of the strategic context that you are competing with others in order to make that actually valuable.
And so you have to have examples like this, having taught corporate strategy for so many years. I love that example, Dustin, because I think it's pointing to something really general about competitive reaction more generally. And the very first case I wrote was on Capital One. This was like 20 plus years ago. A company that enters the credit card business. Basically uses what they call information based strategy, which was essentially data-based, to try and redefine how we did credit card pricing and so on and so forth.
But one of the things that I found interesting was they introduced this product in the early 90s called the balance transfer product. You can transfer all your balances to Capital One. And that was a way of customer acquisition and then retention. But it was also selecting on certain types of customers, risky customers. What is interesting is they were tracking the attrition rates and the risk profiles of customers very closely over time. And just when competitors started coming into the business and imitating that balance transfer product 18 months later, Capitol One saw this is not a good time, the risk profiles are getting pretty lousy and they got out, and some of these competitors actually blew up.
So it's a great example. The other one that I like to point to often is look at Netflix, right. They've had data as a capability for close to 20 years now. But what was interesting was when the studios started taking that content back, it was a change in strategy.
Which is producing their own content that gave rise to basically 10 plus billion dollars of cost that really turned Netflix around. It wasn't the data capability per se. That the data capability was great to layer on that. But it wasn't the first order issue. And so it's, I love this notion of trying to anticipate competitive reactions, but more generally thinking about strategies.
By the way the Q&A is blowing up. So maybe we can just take some questions. And there's a whole bunch of interesting ones here. I'll just start with something we've been talking about from Ozzie.
I'm looking at the intersection of data, AI, and health. These activities include applications to epidemiology, which are becoming increasingly important. The activity spans data science, business models, and so data seems to require a pretty broad understanding on how to be used to deliver this advantage, right.
And Ozzie, that's right. Some of this you'll see in Dustin's course. Some of this you'll see in follow on courses, including the Data Science For Business course and my course. So that's to come. How much data do you typically need Dustin to make good inferences from it? This is another question that's in the Q&A.
Yeah I oftentimes get the, like, how much data do I need a question. Either in my research or consulting. And it's a great question, but it's the wrong question, right. And so it's a great question don't get me wrong. But in some senses it's the wrong question. And you can think about that in a couple of different ways.
One is to just think about data quality. And we're going to spend early on in the class a fair bit of time going through examples of large companies using lots of data that just turned out to be not very reliable. Not actually addressing the thing that they thought it was addressing. And so every day of the week, I would go for higher quality but less data. Without a doubt. The other thing you have to be thinking a lot about is, this goes back to the kind of cause and effect discussion that Bharat and I are having.
Which is like there's this common phrase of you see an increase in ice cream sales correlating with an increase in murder. That correlation exists. Now why does that exist? Well it's getting hot outside, so more people are buying ice cream. And it's getting hot outside so people are getting mad at each other.
And so there's more violence. So it has nothing to do with ice cream, and of course, we know that. But it's really easy to just go through lots of examples like that on the surface sound right.
But if you dig into it there's just this major problem there. And big data doesn't solve that. The amount of data doesn't solve that. The other thing that I would just point out which is something that we'll learn just about the size of data. Which is especially when you get into the processing of non-standard types of data, like words or images or sound, you're going to get into a world where the actual size of the data is massive, because you're having to encode all of these different features about that. But that's where actually the value of algorithms becomes really important.
Because in some senses, these algorithms are just they're projecting a high dimensional space, like lots of complicatedness, down to something that's a lot simpler. And this is in the class, but I'll just give it as an example. The way Spotify works, and I'm not going to give away all the details, is that you turn sound into a spectrogram, which is literally just a picture.
And then you use techniques for analyzing pictures in order to differentiate different types of music. But that step is just taking lots of data and turning it into smaller and smaller amounts of data, which then, if you set up things right, is able to translate into an actionable decision, or something that a company can actually do. So there, the size is sort of irrelevant in the sense that it's actually taking lots of data, but you're then stinging it down to then get something that's useful. Yeah, that's good Dustin, By the way, a bunch of questions just about the logistics of the course. So let's just get, first of all, this is an asynchronous course, right? Someone is asking when are the class sessions, but it's really after your own time.
Can you just say a little bit about that? Yeah it's at your own time. I mean we're going to have you paced such that we want you keeping up with the course. Why do we want you to keep up with the course? Well part of the reason is that this is a course that is designed to let you be interacting with others asynchronously.
And so we just really want you to kind of keep up. But that essentially means that if you want to take an hour of me on Monday morning and another couple hours on Wednesday night or wherever you see it fitting into your schedule, by all means do that, right. So it's asynchronous in that sense. It's also just, it's also something that's designed in a way that really tries to suck you in. And this is something that the Harvard Business School online and Harvard Online is really working on to kind of differentiate ourselves. There are a lot of asynchronous courses out there that's just someone lecturing over some slides.
This is not that class. This is not Bharat's Economics For Managers class. This is not business analytics that some of you have taken. It is a highly tailored learning experience to really suck you in and get you excited about learning, rather than me just kind of giving you a PowerPoint lecture. So we use lots of examples, cases, ask probing questions. So it's part of that design that I think makes the asynchronous format in this context work.
Yeah, just say one line about the opening case or the opening story in the course. Sorry, say that again? Tell us what the opening story in the course is. So the opening story, and we started this about a year and a half ago, was about flu detection.
And whether you should set up an immunization clinic in a local school. Pre-COVID. And so we did all these interviews, it just totally pre-COVID. We did all these interviews, we have and we had this great story line that charts like how the modeling of the flu has emerged and helps us understand about data quality, how you structure data.
All connected to a decision that someone needs to make. We did all these interviews with top flight people, from different research institutes on all of this. And then COVID hit. And let's just say they couldn't return our calls. Yeah. So Dustin very quickly.
So there's a bunch of questions about data for use in the banking sector, data for use in health care. And just to say a word about that. So the cases of course straddle, I think, different sectors.
But in some sense what you're trying to do just like we do in many of the other courses is generalize to an order of thinking where you can apply that in any sector. These are not, some of the courses that will come in later on digital health and so on. They're more specialized. But this one, this one is a general course.
One question that just came up. And I want to get your thoughts on this. And I so, yeah. So no live virtual interaction. This is asynchronous. So this is an interesting question for, can you come up with better suggestions when we have very limited data availability, and the organization is in very early stages, like a start up.
The catch here is you have less data and the market is pretty new, but you want to be able to use data. How do you do that. Yeah so that's the context where people, I think, oftentimes undervalue the role, and this isn't something we really cover in this course.
So this is just an opinion. We tend to undervalue the role of very thoughtful and strategic interviews of other people. And the reason I say that is that in this sort of data obsessed world, we are sort of driven to like, OK, what's the big data set that I could download that will then help me answer this question. But many times, that sort of the received wisdom of that data set is sitting in the heads of other people that you can talk to, right. And so I think it's-- but when you do that, you have to be very mindful of all right well what incentive to they have to tell me this information, right.
So again, it's a very strategic process. The way you're thinking about it. Or sometimes you have to be mindful of the fact that people will make up anything just so that they think people are listening to them. So you're sort of BS detector needs to be on. But I think it's very valuable to sit and talk to people who might be advisors in that space.
And then I think you start to work up to OK what are the data sets that we should collect, given the problem that we're trying to solve. And it's that type of iterative early process that it's actually very fun. So just one logistical question, Richard Pitts. Hey, Richard. Asked is this available after April.
And the answer is yes. And can you just say a little bit about the pacing. How often will it be available this year.
Yes so about every three or four months we're going to have another wave. And lots of people are registering for the first one, and invite everyone to do that. But there will be another one in April. So on and so forth. And then we'll just continue to roll.
We really hope that it'll be exciting for you. And again, just to go back to one of the questions. If many times, I found that there are people who actually have some data science background, like they know some of these algorithms, and we'll think maybe this is too basic for me. But this is about critical thinking.
And I was trained in data science, too. I was trained in the math, I was trained in the program, and I was trained in the algorithms. I was never trained in how to be more critical thinker about the use of data. And so whether that you're coming from a little bit more of a technical background or you're someone who doesn't have that technical background. I think that this course is going to be helpful for those audiences. Now those of you who want to then focus on narrower industries, or narrow type of approaches, we're going to have courses coming up that will allow you to hit that.
But in some senses, this is helping to provide a foundation for both people coming from a technical background, but also the 90% of people who don't have that background that just want to get an introduction and are going to be in that more critical thinking type role within their organization. Yeah let me just stay on that for a second. I think this is one of the questions that came up in chat and we can talk about it a little bit.
I think this distinction you're making is a really important one, right. Because as you said right at the outset, this is not a coding course. You're not going to learn R and Python. But you're going to be doing a lot of critical thinking. And maybe we can just elaborate on that.
A, with respect to how is that different from the Data Science For Business course, and B, what is this type of critical thinking that you want to be able to instill in the audience. And who is that audience fundamentally. And I'll just preview that a little bit by I think several folks, it's great to see so many folks who've taken Econ For Managers.
I mean the reason we created that course was very simple, which is if you remember, it wasn't about training you in math or graphs. Which is I think the way we often teach economics. But really trying to get you to see sort of A, the big picture. But B, why this type of thinking is so powerful.
So if I was to say you know, that's the economic math, and that's the economic way of thinking. It was pretty basic on the economic math. But I hope pretty advanced, sophisticated on the economic way of thinking. And it seems to me from what you've done is the sort of parallel here. This course is basic on the actual coding, there's not much of that.
But really on the data thinking side of things, it's pretty sophisticated from what I've seen, so, but maybe you can add to that Dustin. Yeah no I mean, that's certainly the hope. One person's sophistication is another person's entry point.
But nonetheless. Yeah I think that's right. There are all sorts of things that aren't well known. I'll give you an example. We have a module that's about privacy.
Right. And we actually have people, have learners help to design a privacy policy. Now most people, they don't even think about that, right. They just kind of click through go, Oh the lawyers do that, right. But this is a fast moving area. And so it's not something that has anything per se to do with coding or a particular neural network so on and so forth.
And we even get up to a point where we talk about some examples about de-identify data. Well it turns out that you can pretty easily de-- take data that has been de-identified and identify people that are in that data set. And so this has been done with things like medical records.
That's like, that's not something that has anything to do necessarily like an algorithm. It's instead a wake up call to think about how should we think about how to protect privacy and data in a way that oftentimes gets forgotten. And quite frankly, it's been forgotten in ways that then leads to large legal bills.
We also are wrestling with some philosophical things. Like what happens when recommendations that you make are helpful, but they get one of your consumers in trouble, right. And how do you anticipate those sorts of things as part of your, as like full on part of your business process. Rather than getting into the weeds about we use a reinforcement learning or convolutional neural network or whatever else. These are things that I think everyone needs to be thinking about.
Because there could be cases where someone could pull a flag and say, hey, let's stop and think about this. And we want to be surfacing those things. Otherwise it just leads to a hassle and legal bills later if you don't start to think about some of those things.
Thanks Dustin, that's really helpful. Sorry go ahead. Yeah I also think it's important that we're getting everyone on the same page.
So we don't talk about things like OK this is the cloud. This is what different computer languages are. This is how libraries fit into that. This is how, these are the sorts of things that libraries do. And so all of a sudden, everyone's going to hopefully have more of a common language.
And I think that's going to be also really helpful for then having that sort of higher level type of thinking within an organization. Yeah this two way communication, I think, that you keep coming back to I think is really important. Because in some sense, I was speaking to someone recently. And they said you know we have all these online courses and coding and stats which are great for the 2000 or so people in our org who are going to be data analysts or be data scientists.
But we have another 50,000 who need to be able to understand data. And you know that's not that's not a coding class or stats class. We need to, I mean the types of issues I think you're talking about I find fascinating. Because it's about would you use this algorithm? How do you think about the bias problem? How do you think about the privacy problem? How do you think about the problem definition? How do you think about, those are the kinds of questions I think that to some extent are our first order, right, for organizations.
We're over time and there's several questions coming in. What I would say is on the logistics of Dustin's course, if you can go to the HBSO website. There's going to be information there that's frequently updated. And there's also a site Harvard Online, which we're setting up which is also going to talk about these broader sets of courses.
Dustin, just let me just close with one last question. And this came in through the pre webinar Q&A. What's, it's often really challenging to present data in a fun, accessible, informative way. And I just wanted to get your sense, what's the, what's the neatest example you've seen of ways to present data in a fun way that you've encountered.
Yeah no that's great. You know, I'm really into taking data that is measured over time and then overlaying it on some other thing like geography. Right because you are representing then the sort of temporal flow. You might have made an intervention somewhere. So you want to see if a change happens. But then you might want to ask well wait a second did my intervention work in one place but not in another right.
And a company that is just going gangbusters with that type of visualization work in order to present results is actually Uber. They have a whole division within Uber that is about the visualization and communication of results. And you can Google data visualization and Uber and read all about it. But they're just doing a really good job of thoughtfully combining those different streams of data over time and over space along with other metrics, such that you just get a really clean insight from that visualization.
And it helps identify, hey why are our sales going this way in this region. So on and so forth. And so that's just one example, but it's a the whole field of visualization that's out there.
Yeah no I think if you search great data visualization examples, there's so many interesting ones out there. That's a neat one. Anyway. Thanks Dustin, this was fun to just chat about data science more generally but also the specifics of your course. Which I've already I've started taking it, and I'm really looking forward to finishing it. And it's great to see everyone in the session with your questions, but also great to see former learners.
Hopefully this session provided some useful information about what's to come. And as I said, there's many more courses coming down the pike through the series that will keep you closely updated on. Great to see everyone, and thanks Dustin. Yeah we're super excited. And just to let everyone know, just due to all the huge amount of interest that we've seen today.
One of the things we've actually done is reopen the applications. If you want to jump onto the January wave, happy to have you. And some of you might take it in April and in future courses.
So really excited to help the learning experience. Wonderful that's actually great to hear that we've reopened applications for a few days. So that's wonderful. Well thank you again Dustin. Thanks, everyone.
Have a wonderful rest of the day, and be well wherever you are. Thanks, everyone. Cheers.
2021-02-09 19:01