Automating Visual Inspections in Energy and Manufacturing with AI (Cloud Next '19)
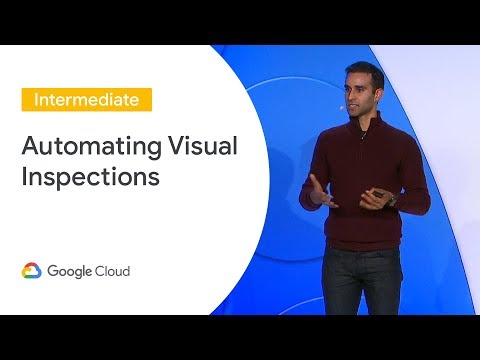
My. Name is Mandi 4-h and, I. Lead the industrial, AI initiative, for, Google cloud. Thank. You so much for joining, really. Delighted to have you here. At. Google we, believe that the goal of every, technology. Should. Be to enrich, our lives. To. Take our societies, our. Collective, humanity forward. And. Do. So in a responsible manner. So. We're constantly thinking of ways in which technology. And. Particularly, AI. Can. Help us realize this, bright, and promising future. So. We've been thinking how can we apply our advanced. Computer. Vision technology. For. Solving some of the very, hard. Incumbent. Problems, in the industrial. Sectors, and. How. Can we make these sectors. More. Efficient, and more. Sustainable. So. In the next 50, minutes we'll. Be talking about how, with, industrial. Inspection, AI, that. Is powered by the auto ml vision technology. Can. Help make industrial. Inspections, more, easier, faster. Accurate. And more. Importantly, more safer. And. We'll also look at how to, leading, companies, are applying this technology, to. The energy, and manufacturing, sector. So. Let's get started so. AI, hold great, promise for solving, some real, world. Problems. From. Detecting, glaucoma, with. Retinal, images, to. Processing, millions. Or even billions, of documents to understand, their content, to. Automatically. Moderating. Unsafe. And inappropriate. Content. We. Are applying this technology, across. All of these use case. But. We. Also recognize, that, developing. This technology, building. These custom, vision models is laborious. And it's hard. So. We wanted to enable. Even. The non programmers. To. Be able to tap into the power of AI and, that. Is precisely, why we, created, Auto ml. Vision. So. While our standard, ap eyes are a great, powerhouse, for, pre-trained. Models, on the, massive, google image, datasets. All. ML allows, you to train custom, models that are specific, to your industry needs. To your, use case needs, how. Do we do that so. In, a very simple, clean, UI. You. Are able to upload, the images. Labeled. Images if you're looking to classify, a problem. Or, you, can draw bounding, boxes at we as we take a look to, detect specific objects. Within those images. Once.
You've Done that, with. A click of a button you've got a model trained, and that, model. Can be used to. Detect, shark, species in this case or. You, can use that to detect defects. Anomalies. Breakage. In your. Specific. Industrial. Products. We. Already, seeing use. Cases, with. Wind. Turbine, degradation. Inspection. With. Outages, on solar, panel forms, or, failures. On electric, poles and we'll. Be looking into some of these examples in more detail shortly. At. This point I want to take a moment to talk about data, protection and, privacy. So. Your data sets your. Images, are your, images, all. Of, these custom, trained models, are. Used only on your use cases by, you, Google. Does not pull these images, into any common deposit, trees or use this across customers, so. Your data sets your. Images. We'll. Take a look at how. This. Technology can be applied for, aerial. Inspection in, wind turbines and then. An application of that on, the, production line in a, manufacturing company, but. Before I begin there we. Want to share Google's stance on the use of this technology. So. Google, cares deeply, that, it's technology, is used, for, creating a positive impact, in the world and in. That win Google, created, air principles, in last June, they. Set the standard, of the application, of these air technologies. And we. Abide, by these principles for. Any work, that involves AI and. Similarly. For the use of this technology and, for this product. We. Expect, that this technology, be applied in accordance. To the air principle, which prohibit, explicitly, the, use of this technology for any nefarious purposes. So. We'll now take a look at how. One. Of the leading, energy companies, in the world is applying. This technology, to. Create a brighter, and greener future for us all. Let's. Take a look at global yes, wind turbine, inspections. Not the biggest you know several times now. We really do have the technology, to, address the. Issue of carbon. Footprint greenhouse gases, from. The electric sector. Dey's, corporation, is one of the leaders in new, technologies, for renewables, and energy storage it's a fortune 500 company. Our mission, is accelerating. A safer, and greener energy future. Right. Now we have eight wind, farms each farm, has different.
Capacity, Starting. From 50. Turbines, up to 300, turrets they. Cover large spans, of geography, and land they're spread across hilltops. And mountain sides all. These. Turbines, needs, annual. Inspections. Originally. It could take up, to two weeks to, do one inspection, we partnered. With leading, drone, service, company measure, right. Now with drones we can do it in two days and this is safe and, quick. For. A wind turbine inspection, we go out with our pilots and what we're looking for is, cracks. Or defects things that may need to be prepared on a typical, inspection. We're coming back with 30,000, images spending, four weeks reviewing, images I don't think anyone's gonna argue that that the best use of a highly trained engineers, time how, do we speed, that up and how they make it 10x, more efficient, that's where machine learning and AI comes, in we've. Built a great and an solution, using Google class tools and platform with the auto amount vision tool we've trained it to detect. Damage we're, able to eliminate approximately. Half of the images from needing human review remaining. 50% of their time can, now be very focused on identifying that, damage and really, determining, the right course of action to immediate, it moving. From reviewing, images to training, machine learning models it's a much higher order employment, opportunity, for people and one where we're trying to develop on our team Google, cloud has, been a great partner there technology's, consistently, among the world leaders and I'm just a great partner to work with person-to-person. At. The end of the day we won't reach the cleaner energy, future without, advanced, tools like, machine, learning, technology. Will, allow the renewable energy to be cheaper than conventional ownership. Artificial, intelligence, robotics. This, is really where the future is all about. Please. Join me in welcoming Nico's, born from it yes. Thank, You Mandy and thank, you to the team that put that great video together. The. Power industry is enormous it. Touches all of our lives and the impacts are felt around the world the. Industry investments, are often quoted in the trillions of dollars the. Opportunities, for improvement, are often in the billions if not, tens, or even hundreds. Of billions of dollars. The. Industry is also going through significant, and profound change, renewable. Energy, is continuing. To fall dramatically, in price solar. Wind. And battery, energy storage are, not just possible or. Practical. The. Consumer, is also driving change they, are much more aware of both, the opportunities. And the costs, associated, with their energy use and the. Third megatrend, are the, new digital tools cloud. AI, and, many, others that, are changing the economics, of insight. I'm. Here today to share one story where. We've partnered, with Google to. Improve lives by, accelerating, a safer cleaner energy, future we. Call this our vision, or aerial, intelligence, platform. First. A little bit about myself and, the company I work for I'm, Nick Osborne I'm the business leader focused, on understanding. And applying advanced. Analytic tools like, artificial intelligence, and machine learning to. Applied business cases. Jobs. Really quite simple I accelerate, coordinate, and facilitate the. Adoption of these new. Tools across the organization. AES. Is a global power company, were, headquartered in India in the United States, but. Operate in 15 countries around the world we've. Made a very significant, commitment, to reduce our carbon intensity, by 70% by the year 2030. To. Help us achieve this we've. Made some very significant. Investments, in new technologies, we're. The world leader in battery, energy storage using lithium-ion batteries, and we're. Also the largest owner of solar assessing, and in the it states, on. A personal, note it feels good to come home at the end of the day and know I'm working with a company that's, putting its money where its mouth is to, drive that change that is core to our mission. Applying. New technologies, is core, to how we operate our business our. Drone program, is considered, world leading in the end of in, the energy industry, we. Developed this program by partnering with measure, measure. Is a professional, drone services, organization, and the, measure ground control software is an, enterprise caliber.
Drone Operations, platform, through. This partnership we've, improved the cost safety. And performance, of our inspections. Another. Consideration is, that what we often hear about the threat of technology. Taking jobs or eliminating, jobs that's clearly not the case with, what we're seeing in our drone program, and many, other technologies, that we're exploring we. Now have over a hundred and seventy pilots, trained, in our organization, performing operations in over a hundred locations, around the world these. Are employees with tremendous value for our company for, their personal, advancement, and their broader career growth. Prior. To drones. These. Inspections were typically done manually, so, it was either someone climbing up the turbine and then, rappelling, down to inspect the blade or hiking. Around the turbine with a large, telephoto, lens trying, to capture an angle and trying to see if they could detect, damage. Neither. Of these were as effective, or as efficient, or, as safe as what we're able to do with drones. So. Using drones, we're. Now able to take that partial, inspection, that was taking two weeks of time and do a full inspection in, two days a much. Lower cost much, higher quality and a much safer manner. Tremendous. Improvement in efficiency and velocity, in our organization, but. There was one new workflow, we're. Now when we do a single turbine inspection, so single turbine has around 300 images when. We do an entire field this means we're coming back with 30,000. Or even, 60,000. Images this. Takes a lot of meticulous, and detail review. To. Complete, the inspection, work. So, we saw this as a great opportunity for artificial, intelligence and this. Is really where our partnership, with Google started to grow. To. Understand our journey towards AI you, need to understand with where where we started we started with an investment in talent we, sent two classes, of six people to Google's advanced to solution, lab for, intense, training and supervised, machine learning. This. Cohort, became, the foundation for our work in AI. Internally. We refer to this decision as a no regrets decision, meaning, that we were able to quickly move forward, make, this investment with, little or no hesitation. On our part, a, few, keys for ROI, is. One is don't, just send IT people, to this training a lot. Of the value from data science in general and. This program comes, from the mixture of expertise, and ideas that you get when you send multiple multiple. Types of people through the program, the. Second piece of advice and, this is maybe, a bit selfish on my part is, make. Sure you have a good commitment, to work on your projects, after, this training, we. Only sent high-performing. Individuals, to, the training and the risk with sending high-performing, individuals, is that they're going to get quickly pulled back into their day job and. That's definitely something we had to work through as an organization, so, this. Investment, set, the groundwork to, accelerate, our progress that, we were making as a company and is, another, example of, where new technologies are, increasing, opportunities, for our employees. So. From this foundation we got to work we. Went through a proof pilot, production, process, with, each step being a stage gate for further investment so. Starting with our proof we, built a custom, tensor. Flow model leveraging, the openly available inception. V3, vision. Model and it, worked we, were able to detect damage, but. It also showed, us where our shortcomings were, our. Data needed work and setting. Up the end-to-end platform, was going to be difficult and we were going to need some help. So. In speaking with Google, about our progress and our learnings. We. Discussed, the possibility of partnering, on a pilot phase, so. In the pilot phase we, are we are were labeling sorry, we, were using Google's. Data labeling, service and Google's. Auto mail vision tool to, really accelerate our efforts and boost, our efficiency, and again, it worked. False. Negatives. Were. Seen as a key business risk for our organization. So not detecting, damage is something that we weren't willing to accept, in our inspection process so. Using our most restrictive, precision, recall metrics, during. This pilot phase we were able to show that we could eliminate 30%. Of the images from needing any human review so. That four-week review, process, was now down to three works three. Weeks really, accelerating, our velocity, and our time to action time. To action has really become one, of those key metrics that we look at with this project. So. This gave us the commitment, our yeah. Commitment. And ability to move forward with our production environment.
So. Our production environment is a scalable, platform for. Us to label images train. New models and manage, those models in production, we're. Still iterating, and refining on this model but we're again showing some very promising, results we're. Now showing that we can eliminate 50%, of, the images from needing any human review and the, remaining 50% of the images are now categorized, and classified, by type of damage further. Improving. Our time to action and focusing, our engineers, on the most important, and most critical, types of damage. So. Going, back to data one. Of the things that we had learned about early on was, that our, data all we had a lot of data that was not at the quality, or level of consistency we needed for machine learning so. Working with measure we, developed in nine category, classification. Of damage this. Includes things like cracks gel, coat damage. Different, types of delamination and splitting as well, as some non damage categories, like serial, numbers lightning, protection points, stickers, and whatnot so we. Also worked with Google's, data labeling team to. Iterate and walk through many, many. Edge cases, of. Different types of damage that are out there we, started with a series of batches, small, in size doing. A full and complete review, of all the labels that were coming back. But as the quality of labeling improved and our batch sizes improved, we've moved towards a sample basis. We. Also needed to develop a platform. To. Manage the labeling effort model training prediction, process, working. With Google we. Identified, clear object to be a local GCP partner to. Help us architect, and develop, our platform, using, the latest thinking and cloud and serverless, tools available from Google clear. Object, has been a great partner and work, to quickly develop this platform for us the. Platform leverages. Auto ml for, our core modeling, engine, cloud. Storage and cloud SQL for, our image repository and metadata as well. As cloud functions, and app engine for to, manage our interactions, and orchestrations. So. Now that we have this platform we're, continuing, to improve on, the model or, we're also looking to expand, its use we're. Looking at new business cases, solar. Transmission. Infrastructure, and even safety, as well. As looking at new inspection modalities. For. Example infrared, and even lidar, we're. Also looking at pushing the model to the edge or in. This case the drone so. I'm really excited to hear about what LG is going to be sharing next. Energy. Is a trillion dollar business it impacts. Lives in every day in every country around the world the, challenge, and the real-world impact, are huge if. You're interested in working with or for, company, that, is improving, lives by accelerating, a safer, cleaner energy. Future please. Come talk to me mandeep. Thank. You very much make for that great presentation. So. We saw how Auto ml version can. Be used for, visual inspections, to. Make them more easy, faster. Accurate. And safer. In. Speaking, with lot of experts, from the industry we. Learned that there are some specific requirements, for manufacturing. Use case a lot. Of a time this, data, sits. On, premise. There's. Latency, requirements and. Most. Of the image and data sets are in a format that requires, it to be processed on the edge devices, this. Be a mobile phone this, be an edge TPU, a CPU. Or a GPU. So. With our Auto ml, version on edge, solution. You're. Able to take your custom trained model and then. Download, them in an, edge device and you. Can run those inferences, from. Your edge devices. I think. You'd much rather see. That in action and, hear, directly from a manufacturing, company which, has deployed these models, on the production line so. It's a great pleasure for me to invite mr. soon book leave from LG and share, more about this initiative mr., Lee a. Good. Afternoon everyone. I'm. Very thank, you for your attention to our previous. Presentation. My. Name is Tom Oakley and the vice-president.
Of AI, and, picked a business, unit at LG's, Janice. It. Seems there, are many Isis, fascists, in our audience today I. Think. If. You are like me I expect. We share many great hopes to apply, AI, tulear, word assertions I. Also. Hope this, short, overview our collaboration. With. Google Auto ml will help you all in, your AI work. Today. We'll. Be looking at how, additional, send Coogler has successfully collaborated. On hey I immediately, commission, technologies, and how. We have, been apply, our leisure to pigeon. Inspection. Systems, and several. Manufacturing. Solutions. Let's. Begin with a, little background over, jeez Janice I think, you may know the name of LG group but. You don't know about it what, kinds. Of companies, in the energy, group so, I want to introduce some companies we. Have LG Electronics, which produces the television, and refrigerator, and we. Have LG Display a produces world reading, or LED panel and the. LG Innotech, produces, a camera model so, I think a half of you the have already the, LG no text camera in, your cell phone oh sorry. Smartphone, and. LG. Chemical produces, electric. Battery is another and world reading company under, age group so you may know that almost. All the LG. Group company is working, in the manufacturing industry, as. LG sentences, supplies. The IT solutions. For. The LG group affiliates, and other companies, the. Working, in the manufacturing, industry we. Are constantly, working on how. To best, apply a high, technology. To improve, the manufacturing, processes. And. We. All know that it can be really. Challenged to use the big data and AI technology. To ensure product. Quality. On. A largest, scale production, this. Is where our discussion. Of a Google or ml comes, in today. Edition. Has started, working with the Google team l in. The summer of last year. We. Started, our collaboration. After seeing the Google was, achieving, in their, immediate recognition. Technology. Because. We thought Google or ml could, help to improve vision, inspection. For. LG, production processes, and, to. Our great satisfaction, our. Collaboration. Has been a success. Okay. Before we, work, with Google ultramel, actually. We had already, developed. Our own in-house. AI system, a photos.
Of You familiar with the manufacturing, process you, will like clear, recognize. That the picture. The left of the screen, is. The typical, visual, inspection, system, that, reliance, relies. On the, human, operators. While. Many, production, lines can't have a camera, and IOT. Sensors. And other detection, technologies. But. Non. While. The many production, lines can use camera but it is still hard to find the rear defect. Efficiently. Sometimes. Non defective, product, open. Misjudged as defective. Because. Of minor factors, like a small, dust particles or, low. Resolution. Images, and. It is still more, effective. Rely. On people, to complete visual inspections. And. While. People, get, better the Ridgid that. Monotony, of a visual inspection, made. By workers also lead, to many. Errors as well. To. Solve this problem, indigenous. Made. A tradition. We. Moved from the traditional. Visual, inspection. The left, image II you can see, to. The AI inspection. System shown on the right. I'm. Sure many of you also working, on the inspection. Technologies, so you will, be familiar. The. Trier and era Mossad. We. Need to improve our system. With, artificial, intelligence. Anyway. Within. Our with. Our. In-house, system, we. Increase the accuracy and, performance and, even. Improved, our process, speed. And efficiency it means that we could reduce our the, operation, costs, or zone. With. Our in-house. AI system. We. Were able to apply to, over the, three production, lines only. In age group, some. Of these include, the first, picture. As you can see we, could improve the defect detection in. LCD. And LED, panels. And. Under. The middle of the picture we. Could remove in, pretties. From. The optical, Trillium and. Even. Improving the quality control, for the production, so, about automotive, efflux, can. Be made with, our in-house. AI system. But. Even. With this, improvement. Our. System, wasn't, working optimally. Because. It still requires, a lot of time and effort. To. Perform well. And. Now, I. Will. Talk about a little about the downside, of this. System. As. It's often the case with. The success, we. Also ran. Into some obstacles as. We. Expanded. The application, of our AI pigeon, inspection, into. Other area. We. Have experienced. A shortage of skilled AI. Developers. It, is very hard to hire the good AI, developers. For, the company they're located in South, Korea. So. It. Is very hard times when, the one, a I developers. Leave our company, the. Parry impetus is so big to our company. So. And. While, we designed the AI model, they. Need to spend a lot of time, and effort to achieve high. Performance. Additionally. As, we, develop, the model using. Service located at the production site the. Compressed, T of architecture, has been increased, so. It is hard to be served. So. Now, we. Require. The process to sentry design and this. Treat the model to the edgy and to. Centrally, control, the the performance of the deployed. Model in, one, integrity. System. Collaboration. With Google has. Been a critical, to. Find the solution, to these problems. The. Performance. Of Google, ultramel, has. Been truly. Exciting. Even. Though our, the. AIS person doesn't, like it. One. Of the key areas we. Need to improve in, our system. Was. Our. Productivity. In terms of the. Moral development time. As. You can see in the diagram on, the left our. Top, arrow. Bar. Shows it took roughly seven, days to. Complete, our model, before using ultramen, but.
Afterwards, We. Brought that down, to a mere, two two hours. With. Google Reutimann. The. Other area we need to improve was, the accuracy. Of our system. In. Addition to being faster. From. The diagram, on the right pictured. Google. Automates performance exceeded that of the, AI experts. In many, times our, test. Lizard showed the, average is six percent improvement, in, terms of performance we, can expect when, using a Google chairman, I think. While. We, have made, advances. Using. Google or ml and, integrating. That with our, visual inspection, we. Are still facing, several, challenges, in, many. Cases, we. Could not meet our clients. Requirement. And, you. Found that the, many of them comes from the low. Image, quality not, from the model, that we made with the Google. So. To. Solve this problem. We. Listen to launch it. Immediate. Pre-processing. Lizards. Team. The. Members, of this team. Spend. More time on. Exploratory. Data, analysis, and. Pre-processing. Data. And. Try how to try. Hard to augment. Data, for, getting better machine, learning models so. They became. To spend a lot of time, on, thinking, how. To the. Changing, the inspection, process itself. I. Estimate. Now, our. Members. Could use their time. And. Effort. For, more strategy. Work. Now. We. Are planning to expand, our. Business into, consulting. Services, so. We. Will provide expertise, to. Enhanced. In. To enhance overall, inspection. Processes, as a one-stop solution. We. Are hopeful, that we, will. See the first. Manufacturing. Visual inspection, area where humans. And a, I share. Areas the, least panzerotti, very optimally. Do. You agree. Ok. I, would. Like to announce that we have built. Integrated. AI a vision, inspection. Architecture. So. Our. System. And Google. Ultramel is connected. Seamlessly. With. This architecture we, will. Be able to maximize, humans. Capability. And. Utilization. Of Google. Or ml. This. Architecture. Starts, from the data, scientist past the bottom. They. Will ensure a major, quality. So. They. Will produce, a clear image and will send to the Google or travel and. Google. Tom a text a clear, image and produced, a a model, with, efficiency. And with effectiveness. Awesome. The. Morris will, be completely, managed. With. All the history data and, performance. Status and. Automated. Learning. Processes. With. This, architecture. The. Elegiggle is now, developed. Now can, developed, and managing thousands, of a aia morris simultaneously. In. Addition, to vision inspection, our. Goal is to expand the. Architecture, to the other the, manufacturing, use cases to, manage the whole factory. Equipment. Facilities. And. The. Safe things and so, on i think you, may think, however many use cases we, can expand this, instrument architecture. In the manufacturing, industry. To. This point. We. Have gone over how, collaboration, with google, Tramel has, improved our visual. Inspection. Systems. Now. Let's. Look at a to, the future. Based. On our AI, integration. Success with within, the edge group, we. Will keep going to be positioned, as leading, AI, visual, inspection, total, service provider so. We, recover from the, pre-processing, area. And we, will cover learning. The model and then, we will manage, all the Morris melt. With. Google, attainment. Whether. The cause of poor, inspection, quality is.
Motion. Running attainment. Over. The image, quality or, data. Labeling over. The operators. Themselves. Working. With Google ml we. Will strive to achieve our, goal of. 99.9, percent accuracy and. The. Leak Lake of. 0.001. Percent, under. All conditions. If. You were experiencing. The similar issues in your, industry. I, hope. That this, session could, be helpful, I really. Appreciate your attention and thank you for listening thank. You. Thank. You mr. Lee, so. The. Goal that mr., Lee shared about LG, is very much what we share for our product. And for our roadmap as well which, is to make our inferences, faster. Our. Interfaces. More, intuitive. And easier and our results more accurate. Within. Manufacturing we. Seeing many, more use cases beyond. Automotive. Beyond. Electronics. Into, the food into. Retail and many more categories, and we, are very excited to work on these new use cases with you we. Saw how a eye and, visual inspection, can be applied to the manufacturing. Use cases and we. Looked at how this can be applied for the aerial inspection use, cases. Beyond. The three use cases that we talked about on the aerial inspection side, we. Are also exploring, more work on the agriculture. Monitoring, and, construction. Site monitoring, as. Of. Today this technology, is available, to use in Bera. Please. Visit, cloud.google.com. Slash. Vision, to register your interest you. Can use the technology right away but. By registering, at this site, we. Are able to partner, with you and work, with you on our upcoming, releases and our, early access program, so. We look forward to hearing from you. Thank. You so much for joining us in this, shared vision and. We. Really look forward to working with you in creating a more brighter more, greener and more positive, future thank, you very much all.
2019-04-16 19:33