Are Emerging Technologies for Women? How to Become a Data Scientist Data Analyst or AI/ML Engineer?
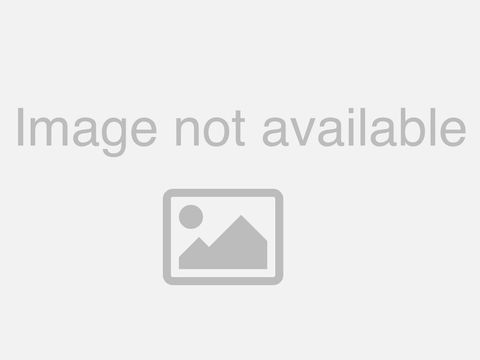
There, are many, opportunities for, women in emerging, technologies. Almost. All roles that you can think of the, data. Scientist, or a data analyst, right. Or a neuroscientist a male engineer all these roles are open, from the name and. The, field is very encouraging, towards women. Hey. Guys so. This Women's Day we have a very special video where, we're going to talk about are. The emerging, technologies, for. Women so. Emerging, technologies, we mean AI, ml data science, and data analytics, and to, discuss this we have some great. Springboard. Gurus. And mentors with us so, let's go ahead come. Let's meet our mentors, and gurus at Springwood, we, have Shira Smitha who is the data scientist, at g2 we. Have lavender who, is the founder, and machine, learning scientist. At, machine, learning interview calm, and, then we have Pavitra, of course with us who's, the staff research, scientist, at sweety welcome, girls thank. You for having us thank. You for everything thank you good so, before we get into the, discussion for, today please. Subscribe to, springboard India. YouTube channel for, more interesting, discussions. And insights. Into, machine. Learning data science, and data analytics ok so we are here to discuss on this special Women's, Day program, is, our the emerging technologies, like data science, machine. Learning and data analytics, you. Know for women so it'll be nice to get your views let's. Start with you thorough job well. Certainly, yes that's. Because so data science and data analytics or the new emerging, technologies that, are coming up are more, fun and. I think women shouldn't miss out on it it's. Not just math it's also about understanding, patterns and, intuitive, understanding. Of data and, I think women. Are not really well in these technologies. Interesting. Point, what about uterus with Orion totally conquered, and absolutely. It's for women it's for in fact everybody, who has a bend of curiosity, it's, for. A long period of time we didn't have something as interdisciplinary. As data science so, people with, backgrounds, like chemistry people with backgrounds, like economic. Statistics. Maths, computer, exit exit. Willing. And you know pushing these boundaries, because, and and, you know having more diverse, workforce means, you're thinking in a different manner because there is no one way of solving a problem in data science so when you have a diverse background the outcomes all these are better definitely. It's nice inside diverse background, a very nice yes, lavender you're the founder, and I. Think thoughts, coming from a founder I'm really, interested in hearing it, absolutely. I concur, with both. Of them that they. Are absolutely, for women. Women. Are you know users. Of technology. Where. You, know data science machine learning data analytics, are being applied and not. Having women take. Up these fields, and work, towards these, solutions we'll definitely you. Know create, a. Very. Different kind of a solution, so I think women, are absolutely needed, and women, are absolutely capable of taking up all these fields nice. So, we have diversity, we, have a founder. I think and she's also calling out for you women out there to come and start a company in data science okay please connect. With her and learn, more from her Pavitra. You, are with, a growing, company and I, think how, do you, see. More. Women joining, these kind of fields I mean you know what is your thought how did you how do we get them to come into, this field so, if, you see right like the last five, six years we have an e-commerce companies, my flip card and then we have companies like swiggy. I mean um one, day see Indian. Companies, right and the challenges, here are type words it's. Not just that you can take a solution, that works in US and put it in here it wouldn't work for us let's say for example the events we need like how do you find you. Need anybody execute, if you're, really hungry but when. Do you think you would come that, is actually some, few ml, models running he'll give you that kind of so, when the thing is about Tremont, coming, here is also about representation. Right, it's this that when. You're talking about diverse backgrounds, it's just not one perspective that matters, but for example and say are, we talking about gender bias so, those things come into picture and it's.
It's It's it's data. Science is also just not about you, know, biasing. Us with just one lis one solution, it's also we're looking at different, perspectives and when, women come into this field those different perspectives amplified. Interesting. What, about uterus method do you feel how, can we motivate more, women to come into this field I think. You. Have to approach it very differently, because. What. Women. Always feel like you know they're scared of coding, tests, they're, scared that they are not at power of you know how, I am evaluated. So we change how we evaluate women. Or you. Know for per se anybody from a problem-solving, point of view versus, you know just looking. Giving them a coding test to do away with their fears then. We are looking at them in a different way we are putting them in an environment which, is easier, for them to even get started so I think it's for. Us to revise, the pipeline so it's not like a pipeline problem, it's how we approach, that pipeline that's the issue and obviously. We as companies, have a lot of lots of biases, so can we. Improvise. Our policies, better can we have removed work culture you, know providing flexibility, to women at. The end of the day it should be like what they bring to the table not, you know where what they are or who they are so, I think that's what matters, so you review them for who they are. So. Since. You are running, a company and I'm sure that everybody here is talking about gender. Gender diversity, and you know that's the hot topic of discussion, in a lot of technology. Companies and we see that and people are trying to change it right what, is it that you do at your end you know to, motivate. Women saying that hey come join us and you know these are the benefits you get or hey come and join these kind of you. Know companies, come and join data. Science, machine learning, field. So what, is it that you think, you. Should do what you're doing right now which, motivates, them to come in join me right, I, think. The. First and foremost thing is to help, them and understand, that you know it's possible for, them to do. Whatever. They want to do right, by, showing them good role models, so being, a female founder for example is, you, know give. The message that it's a female friendly company here right. Beyond. That I think one. Of the points that was mentioned by Childress Mehta having. Flexibility. And remote, work culture. Is actually, very helpful I think for women because lot of women actually drop off for very talented actually. Who. Have actually done fantastic, things I actually drop off from the workforce when. They have kids when they go through my turn ad and.
Giving. That flexibility, makes a huge difference so I, just want to add one point here, so this, is something that I've heard from little women too and it, really helps when. Gender. Sensitization is. Given. That kind of a vintage in a company because, what. Happens is sometimes I've. Heard, this as. A feedback, given to women not, just you, know not. Just in the data science community, but recruits across saying, you, come across as someone as aggressive. Right. Yeah but there is a distinct. Distinction. Because, there is a difference between being assertive and, there's a difference between being aggressive. So, women, have perceived, to be of deserve when they are actually just being assertive, and then you are a data scientist, it's not like you know you just have one module to complete right, you have two different the data you take feedback at the same time you also have to it's also, being you know open, enough and taking it feedback at the same time also starts the saying okay, this might work on this might not work so as. Far as a whole I see data science community, as such if it has to grow we, need allies. Main. Allies allies. Who are our managers, who, are working, with us you know more generous and. Rather than you, know it's. About enabling women. Especially. But, this is money that I've seen her community, so. Like I was saying there's a recent survey which says that there are only 35, percent of women who, actually come into you know we science or the engineering, fields and then, currently, we have only 15 to 22 percent women. In the data science and AML, data analytics, field. So what I would really want to ask you girls is you, know what really inspired, you to. Come into this field and then, how has your journey been so. Just, whether let's start with you I, think. I have, no been happier I would, never have been happier doing anything else about from this so, I started. In data science and I, started because I didn't. Have any idea, what I wanted to do but I wanted to do something different like you know everybody wants to do something different so I had that thought. And I just came. To Grace, Hopper celebration for. Women in computing, okay and I met a lady there and I just, pursued, that lady why don't you teach him why, didn't you I want to just learn something and. That was my first break and you know first starting point and I got my first job offer post that and, probably. I had what, worked for me was I had been, persistent, and I was the only data scientist at that time in my company, for, the next three jobs actually the, only data scientist and now.
When I see it's the things have quality change it's like almost equal, or almost near equal, number. Of male, female percentage, which is like I see there is an improvement but a lot can be done at. The, same time I think women should be bold to, ask questions. Most, people are afraid or scared to ask they don't ask for a salary they think that I'm ok with this because, they are judged, based on the last salaries, right so, ideally. Most company shouldn't even look at salaries, if you are right person for the job you should just you know take that offer so most, people don't negotiate so. Women should have, know. How to negotiate through an offer because they deserve it and they shouldn't question themselves that, I am not good enough so, I think those. Two, attitude, change can bring a lot, of women into this, field and it's a very accepting, field because there. Are lot of lots, of opportunity. To wear multiple hats and, to end this community. Basically so I think I love that about my job nice ask, questions, be persistent, yes. Well, now you've been I'm. Sure you had a long journey as, machine. Learning engineer. And scientist, and then you found it a company so it's interesting for me to understand, and know how, did you start and, you know what, are the challenges that you faced and how. Do you make it through all that right, so, my. First introduction. To data science, it was never called data science at that time was when I did my Master's back in University, of Utah so, I was working on statistical, models for, computer graphics so. I was using machine learning but it was never called that I mean it was called machine learning but that's. When I got to know about it it was for computer graphics so, then, I worked at Amazon again. I was actually using some machine, learning algorithms, but it, was never called data science it was very low key so, I was an engineer there and I was actually implementing, all these algorithms and, no word about it right. So, then. I was in Myntra, for some time and in Mobe again, there, were a lot of interesting, challenges about. Ad. Targeting that. Can be solved with machine learning and I was looking at all that and at that point I felt I wanted to go deeper, into it, now. There are so many resources out there to, actually go learn data. Science machine learning in. Depth but, at that time even. Coursera, was not there it was just starting so, I. Decided. To do a PhD at that time so I joined the Indian Institute of Science so. I did a PhD at Indian Institute of Science and then joined, Amazon, again as a machine, learning scientist and researcher right. So there. I worked again on many. Interesting, problems and. I. Felt. At that point that, there, were so many people out there who want to actually become data scientists, but, who, do not know how, it, actually. What. The journey is to become a data scientist and how, to actually crack the interviews yeah, so I started, this company machine learning EUCOM mais non actually.
Popular Request from lot of people or, you know how to prepare for interviews. So. Hurt already has been long challenging. Lot of learning, and learning and then, relearning again what, about you pakistan has it been easy for you so i. Used. To be a software engineer I was working in Microsoft and, then I quit and I became a design to search and. Then I call it the innovation consulting, where I was a company, called as l1 and then, I had a started bug where, and you know what let's do a start-up as to a product so, I met my co-founder, and then we started. Working, on a I startup which was like you know creating videos that, non designers like us can do these videos easily so I was like ok fine now or never will still do low coding, and I think I Mossad but then I figured out that AI, was, much more interesting, and I started into computer vision so it, was more about a calling because it was about getting AI and design together and, the challenge, was immense, and it was all about understanding the concepts, and you know getting, the concepts, to enough tradition like that was the biggest challenge so I really loved doing that and when we got acquired by sweetie I could, see that what am I at learned during the startup phase I mean there's, nothing like being a start-up founder because, you have to wear several, hats, so. During, that phase I bought a lot and when I came in here I was like wow this, field is far far more interesting, and we have not more challenges here because swiggy the the challenge, that we face are very Indian challenges, and it's now needs, a lot of lateral thinking. So. It's, been an interesting journey hype it's been mostly a self rather was lecture stuff yes I think probably most. Of the folks in data science community, are self you have to be yeah it's actually right so I mean there's, always a thirst for knowledge right, and play ok this blog is going to stay in a state that involves, let's go listen to this person, and, you, know is, this something else that you're going to say so it was just like you know Natasha and for us yeah right it.
Was A really even wonderful, journey and I think the. Genasense community, also helped me a lot so, there people, I was learning, things with and I think there isn't something that you. Have to learn together, yes, otherwise, it's difficult, and in, that case again right, women. Helping women it's. Something that's very very important, and once, you see let's. Say I have. A VP. Who simply joined a woman, VPN, I just. See her and I get inspired right, a, woman, being there actually, inspires others just. Like you know she is a woman entrepreneur yeah. Like having. A lot of women in leadership roles. Says, a lot about a comfort zone and that's one of the reason I love my company that you see a lot of role models there so the lack of role models is, what we. Have, and, it needs to be addressed when, you see an example you want to be that person you want to take that journey right I think, that's really important, in it so what I hear is a lot of learning a lot of persistence. Women. Inspiring women you, know that's that's what I hear commonly, a tamale yeah so. It was interesting to hear how, you guys actually, became, reader scientists and machine learning scientists. Whatever, the ask next is what, opportunities. Exist in. These emerging technologies for. Women so when, do I start with you know, there. Are many, opportunities for, women in emerging, technologies. Almost. All roles that you can think of big, data, scientist, or a data analyst, right. Or any, scientist, I'm an engineer all these roles are open, for women and. The, field is very encouraging, towards, women right. So companies. Are actually taking, a lot of, care. These days to make sure that you. Know women. Are comfortable, in. Joining. The workforce and, also, in retaining them, right. And. I have personally seen women in many, different kinds of flows and different, levels. So. Right from a beginner to like a senior scientist, to a head of a data science team and, I. Think, that. Definitely answers, the question has the other so there are a lot of opportunities, available. What about your power I feel that you, know there are different opportunities available, in this emerging, technology, so it's, also about transitioning, from one field to another so, since. I had also done, something of that sort so one thing is that that you it's, okay to make mistakes and, you can give it a shot and try and see even this fits, you or not one. Thing that I could always say is that when you you. Could be a domain, expert in a field but, design in this again something, like you, can learn quite, easily and, then apply it in your field and you would be doing wonders with it you have like a lot more results that you can show and, it's like another tool that you can use the. Next thing is also about AI product, managers and I think, they play a huge role because they are to understand, AI the uncertainties. That come along with it and how do you also you know tie it to a business metric how are you going to push, the product forward etc, right so a product, managers they. They actually play in different fields and I think that's all the wonderful thing that you, know if you have, done a MBA and if. You do a data science course or if you're able to understand that why. Not be a, problem so what we are listening or what we are hearing here is that there's a lot of opportunities, available it's just that how you identify, and, go for it so, just go ahead and, find. Your space and you know just to each other. There's. Also another interesting, you, know article, that I'd read that when, there are lesser women you, know in technologies, the, product, that come out also you.
Know Kind, of become, biased so, I want to understand, from your chourus method is that if. There are more women in emerging technologies will, that help you. Know the technology. That, are built also, how does that affect the gender equality. Definitely. I mean really, a good question because, representation. Inclusion, and diversity matters, a lot in tech, and especially, in a place like a data science. Community. The reason being we, look, for diverse perspectives, while, solving any problem, any challenge, no matter what the. Rule looks like so, the. Kind of empathy. Or the kind of you know very diverse. Thought. Process of perspective, that women bring, to the table that, cannot be challenged, for and I think that that, is what drives the best results. Out of a company so most of the companies, who are doing really well, today. You. Can talk of the big Google's. Facebook's, or Amazon's of the world a company, like g2 so. We, they. Are do early you know care up care about you know the how the diversity how, the representation. Is because. You see the results and it's, right in front of you is there we have stats to prove it so we do the analysis, so I definitely think that having, a gender balance, definitely. Makes, you. Know it's I think, after some time like I think we were talking about this. Some time back about. The code that Sheryl Sandberg. Spoke. About that after, some time there won't be female, leaders but there, will only be leaders so I think that makes more sense and that would happen, eventually all. Of us are in it together so. We are saying that there are opportunities. It. Will also lend to. More equality, at. Workplace, and of course we're. Just requesting. More women, to go and you know try. The emerging, technologies. So. This Women's Day we are actually encouraging, a lot of girls young girls and, women out there you. Know to come and make a career and the emerging technologies, so, I think it would be a good time now for me to ask your girls if, there are any tips and advice you would want to keep the women as how you can make a career in emerging tech. Like. Great, question or everything like this is something I would have told. Myself when, I was growing up that. Be. Courageous. Be. Always ready to ask questions, know what you do not know because awareness. Of yourself goes. A long way so that and always. Be relentless, because in the beginning this field might look overwhelming, but, reaching out to a lot of people, consistently. Helps. A lot because, 90%. Of the people will never respond, to you and that 10% is, your key to your next career move so be. Open. To reaching out network, with networking with people. And you. Know like be connected always look for role models here. All of us today have a journey so, if you need help, and advice look, up two mentors like us like me you also have role models to, look up or fall for, this career transition, so be, it I think this. Community, needs women that's. Lavanya. Let's hear it from you any tips, and advice for women how. Can they make a career and the emerging technologies, right, I. Would say women, need to be very proactive with, what they want they need to understand what they want and they should not hesitate to ask whoever. It is because. Sometimes. People, assume a lot of things so. We want men to be sensitised, but, that's not always the case right so, you need to be very. Open. And you. Need to go and ask what you want second. Thing is I think find. Your champion. Find. Your role model and find your metric cannot. Emphasize. Enough. So. Yes mentor, Pavitra, let's. Hear it from you what's your advice ai, is a new electricity that's, what I'm going G said right and very, soon it's going to be like people, who know I have people, who don't know anyway so, it it's.
Going To be like data science is going to be like kind of a fundamental. Learning for most everyone and I think we shouldn't miss this train and. It's. It's okay to make mistakes it's, it's. Okay to start learning I know it's a little daunting, but let's say for example you're learning writing for the first time it's okay just keep at it right. You will crack it and this is not a race that is between, you and someone else it's actually a race with, yourself, if. You it's more like you know having fun and learning and, that's what matters because it's at the end of the day it's also about fulfilling, what you want to do and, I think in that case especially women, have seen that you know they mean they actually do what they really, like doing, this, is amazing. I. Think. Eventually they will not have any women data scientist. I. Think. We are saying that there are opportunities is. Just that you keep at it ask questions. Find your mint and. You know don't be afraid to make a mistake yeah. So, thank you all for a very interesting. And beautiful. You. Know discussion, a lot of insights there, also. I feel, that you know it brings us to the end of our discussion for, today and, I think I'd like to close it by a very. Beautiful quote, by Estelle order she said you know I didn't get here by just wishing for it and hoping. For it I actually reached, where at least by, working on it so I think that. Goes for all the women out there in emerging, technologies, it's, so wishing you all a very happy Women's, Day. Wishing. You all a very happy when. Enjoy yours out there happy Women's Day. Happy. Women's Day to everybody out there thank. You for. Watching this video please like, it and please subscribe to, springboard, India, youtube channel thank you.
2020-03-08 17:55