2021 CES: Under the Hood with Prof. Amnon Shashua
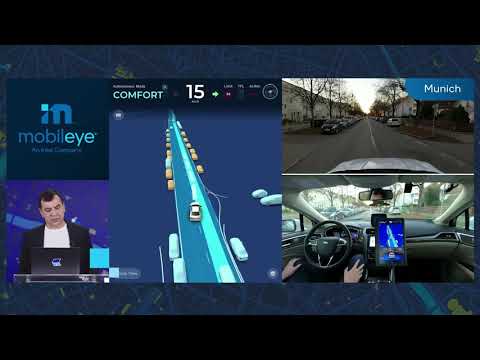
- Welcome to Mobileye's annual Under the Hood session. So this time we're doing it virtual. Normally we do it at the CES. I'm going to go into a status update of course, but every year I choose a topic or two and I kind of deep dive into those topics. This year, I'm going to choose a topic of maps. I think after five years of development, we have reached a game-changing status that I want to share with you, and also about the active sensors, radars and LiDARs.
We have exposed in the past that we are working on those active sensors, but I'll shed more light, and also what is the motivation of doing that to begin with. So let's begin. So first, just to remind kind of to set a baseline. What is our business pillars? So we have driving assist, which goes from simple front facing camera up to a 360 multi-camera setting with very, very advanced customer functions. From the the basic your end cup functionalities up to hands-free driving under a level two setting in which a driver is responsible. So this is a huge pillar for Mobileye.
The second pillar is all about the data we collect from crowdsource, the setting. We have now millions of miles being collected every day. What do we do with this data? It's not only powering our maps, but also creating a new data, creating a new data business. And then the full stack self-driving system. So self-driving system, it's the compute, the algorithms, the perception, the driving policy, the safety, the hardware and later into the future, also the active sensors.
And the self-driving system is the basis for building a mobility as a service business. So those are kind of the three main pillars. As an update, we finished 2020 with a 10% year on year growth in shipping IQ chips. Also our top line, more or less the same type of growth which is really very impressive in my mind, given that three months, we had a shutdown of auto production facilities.
So the year began in an alarming situation and ended quite well. Without the pandemic, we would be at a much higher growth, but still a 10% growth in a pandemic year is in my mind, very impressive. We had this year 37 new design wins.
And currently in parallel, we have 49 running production programs and the new design wins, they account for 36 million units over life. Above all the millions of units of previous programs. In terms of our portfolio, of the kind of the spectrum of product portfolios. So the basic is Silicon. We sell our IQ chip with the software embedded, the application software embedded.
This is how kind of legacy product position. We added a new product position, which is kind of a domain controller, the Silicon + PCB, this is for the more high end driving assist which has multi-camera setting like our supervision, which I'll say a few more words about it. That takes 11 cameras into two EyeQ5 chips. It's so advanced that we decided that it's better to build an entire subsystem which is a Silicon plus the PCB and not just the Silicon itself. Then the full stack self-driving system. It is the entire hardware around the self-driving system, and later including the active sensors.
And then through contract with car makers, the full stack self-driving vehicle. And then together with Moovit, the company we acquired half a year ago, going into mobility as a service, the customer facing portion of the mobility as a service. So this slide, I think, is one of the most critical slides in this presentation is what separates us from the crowd? And there are three, we call this the trinity of our approach. There are three components that are very unique to how Mobileye sees this world.
The first one is when we look at the driving assist and autonomous vehicle divide, we don't see this divide in terms of performance, in terms of customer functions. It's not that an AV can do more sophisticated customer functions than a driving assist. We see the divide in terms of meantime between failures. We can have a system that has a level four, level five capability while the driver is behind the steering wheel. That would be a level two. And we can have the same function but without a driver.
Obviously, if you remove the driver from the experience, the meantime between failure should be astronomically higher than the meantime between failure when a driver is there. So this kind of thinking drives us into this idea of redundancy. In order to reach those astronomical levels of meantime between failure, we build redundancies.
We build redundancies first in terms of different subsystems. We have a camera subsystem that does an end to end autonomous driving. And then we have a LiDAR radar subsystem without cameras, that does the same performance. Full end to end autonomous driving.
And then we put them together in order to create a kind of a product of the meantime between failures of the two system. In each subsystem, we also build a lot of redundancies. And I'll show you bits and pieces of this approach. So this is one unique way of thinking that is unique to Mobileye.
It's not that we take all the sensing modalities. Cameras, radars, LiDARs, and we feed them into what is called low level fusion into one computing box and output comes. The sensing state, we do a much harder work.
We kind of tie our hands. We take the cameras without the radars' and LiDARs' input and build a full sensing state. And then we do the same thing, but without the cameras, radar, LiDAR, but this tying our hands, pushes the envelope much, much further and allows us to build a system with a very, very high meantime between failure.
The second pillar is all about the high resolution maps, the high definition maps which we call AV maps. And this is going to be a focus of my presentation today. And here we have a unique approach which is a scalable geographic scalable approach in which everything is based only on crowdsourced data.
There are no specialized vehicles collecting data in order to build the high resolution maps. Everything is done through crowdsource. It is a very, very difficult task. It took us five years of development.
Today, we passed a threshold in which all that development is becoming very very useful to our business. And this is really something very unique to Mobileye. The third component that's unique to Mobileye is all about safety. We understood that the meantime between failure of the perception system is not enough.
There's also the decision-making that the car needs to do and that's called the driving policy. And what would happen if the driving policy has a lapse of judgment? Because most human accidents occur because of lapse of judgment of the human driver. Now, obviously, we cannot allow computers to have lapse of judgment. So what do we do there? How do we define mathematically what it means to drive carefully? So this is something that we began in 2017. It's part of the fabric of our autonomous car. But more importantly, we're evangelizing it through regulatory bodies, industry players around the world with quite great success.
So those are kind of the three distinctive elements. So let's start with the camera only subsystem. So what you see on the left-hand side is kind of what you see in the trunk.
There is one computer to the left which holds two EyeQ5 chips. That's kind of the brain of the system. There is a PC on the right-hand side. It's a normal PC, nothing special about it, all what this PC is doing, will in a matter of weeks go into the EyeQ brain.
That's it. This is the hardware. In terms of sensing the cameras, we have seven long range cameras and four parking cameras.
And you see in the front, we have a 120 degrees, and then in 28 degrees. All cameras are eight megapixels. And then we have four corner cameras.
Two to the front, two to the rear, and then a rear camera, and then four parking cameras. Each is a wide field of view. 192 degrees at the side mirrors, front and rear bumper. So this gives us the most long range and short range vision perception. No radars, no LiDARs, right? So this is the camera only subsystem.
So, in the clip I'm showing you now for the next 15 seconds, let's see what this vehicle does. I'm going to show you kind of a clip running in Jerusalem, Munich and Detroit. We're expanding our testing. And this is now in Munich. Later, we are going to see Detroit.
This is Detroit. What you see here is complex driving in deep urban settings, and it's all done by the camera subsystem. So the perception, driving policy, control, maps, all done in the system. Now, this system also has internal redundancies. Before we go into the radar and LiDAR redundancies, internal, meaning if you have now multiple cameras, you can create another stream which we call ViDAR, kind of visual LiDAR, which builds a 3D map, instantaneous 3D map, and then uses kind of LiDAR type of algorithms in order to detect the road users. So this is what you see here.
We're starting from a still image. Just that you see the setup, and then you see the bounding boxes on all the road users around the host vehicle. The host vehicle here is in blue at the center, and then the host vehicle will start moving and the scene will start moving. So you see that all road users are detected. Basically, you see a bounding box around each road users through a separate stream, a separate algorithmic stream.
This is not the regular stream in which we take the image, the video, and then we identify cars and put a 3D bounding box around the car. This is a completely separate stream that is working independently from the classical stream that we built. So this is an example of internal redundancies that we build into the system. Now, what we have been doing in the past few months, which I believe is really game changing from... It's not only from a business perspective, but from the way driving assist is going to evolve, is to take this camera subsystem and productize it into driving assist. 'cause as I said, at the beginning with this Trinity of approaches, the difference between ADAS and AV in our mind is not the capability.
It's only the meantime between failure. The camera system can have a sufficient meantime between failure for a case in which the driver is responsible, kind of a level two system. But now it can do much more than a simple lane keeping assist. All what you have seen in the clip before, you can do in an ADAS system in which a driver is behind the steering wheel.
So this productization of the camera subsystem we call it supervision. Again, it's the 11 cameras around the car, two EyeQ5 chips. We provide the entire end to end stack in terms of the perception, driving policy, control, the mapping system and also parking capabilities. And it will be, the first productization is going to be with Geely. It's going to launch Q4 this year, 2021.
So we're not talking about something really futuristic. It's really around the corner. And this would influence the evolution of driving assist to a great degree. And from a business perspective, it's game changing because we are talking about something that is almost two orders of magnitude higher than the normal ASP radars.
So this is kind of a by-product of this true redundancy approach that we have for developing AV. The by-product is that we can immediately take it as low-hanging fruit in terms of driving assist. And here are some news clips about this relationship with Geely and how is it going to be launched later this year. So we expanded our footprint of the supervision. I've shown you a clip showing Israel, Detroit, Munich. The pandemic actually allowed us to be much more efficient.
So take, for example, Munich. In the pre-pandemic era, we would send 20, 30 of our best engineers to Munich to set up the vehicle, test, debug and so forth. We have only two people in Munich. Both of them are not engineers. They're field support employees, and we're all able in a matter of two, three weeks to set everything up remotely.
So it gave us a lot of confidence that we can scale much, much, much faster. So Munich is up and running. Detroit is almost up and running. Up and running, meaning that we invite our car OEM partners to test drive, to do many, many test drives of our vehicle. For example, in Munich, more than 300 test drives to customers have been done with this vehicle. Next, we are expanding to Tokyo, Shanghai, Paris and New York city.
We have kind of a caveat with New York city, 'cause right now it's not possible from a regulatory perspective to test drive hands-free in New York, but hopefully it will change in the next few months. Because we feel that New York city is a very, very interesting geography, driving culture, complexity to test. We want to test that in more difficult places, but New York city seems like something that we can handle. If we handled Jerusalem, we can handle New York city.
So now let's go to the next subsystem. So we want to achieve a level four. Level four, we need a much higher meantime between failure than one subsystem, the camera subsystem can provide. So here we're talking about just LiDARs and radars, no cameras. It's a non-trivial problem, even though, you know, almost all of our competitors, they are LiDAR-centric.
So they rely on LiDAR. But they're LiDAR-centric, but they have also cameras. And cameras is very, very important. The texture of the camera allows you to disambiguate all sorts of things that we are not doing, because we excluded cameras from this subsystem. So it makes lives a bit more difficult, but the most critical part, which makes it very, very difficult for us is the localization. Normally, in a LiDAR-centric system, the map comes with a cloud of points at every moment in time in order for the car to locate itself within the map.
Now, this cloud of points, which is carried at every time instance, is very, very heavy from a computational point of view, and we don't use it for our camera subsystem. In our camera subsystem, we are using our REM approach, which is based on detecting visual landmarks. So what we want in this camera LiDAR subsystem is to use the LiDARs and radars to identify the same landmarks that we have in our visual system, and localize the vehicle into the same REM map that we have with the computer vision. So it's a completely different approach and it adds complexity.
So here is a clip. Before I run the clip, because it's going to look exactly the same way like the computer vision system. So you see on the roof of the car you see the LiDARs, right? You see something kind of protruding on the roof of the car. So it's a different car. It's not the same car.
So you can see that is end-to-end. Also, in few seconds, you are going to see complex driving maneuvers, where with other road users, need to take unprotected turns. So everything is done, the same performance level as the camera subsystem that we have been showing and here there are no cameras at all.
So, this was kind of an update. Now I want to kind of deep dive. And I want to deep dive into two big areas. One is about the maps. We have announced our crowdsourced mapping idea back in 2015.
At that time there were two components to it. The first component is building a technology that you can put on every car that has the driving assist system. The driving assist system will not send images to the cloud because the bandwidth is too high and not to mention privacy issues and so forth, but detects all sorts of snippets. Lanes, landmarks. Information from the scene packs it into a 10 kilobyte per kilometer. So it's very, very low bandwidth and sends it to the cloud.
And we had established contracts at that time. It was with Volkswagen, with Nissan, with BMW, that their production car starting from 2018 will start sending us the data. So that was the big achievement at that time. And then we started working on taking that data that we receive and building a high definition map. And now I want to kind of deep dive. What does it mean? Why are we doing what we're doing and why it is a game changing? It's a game-changing to the entire field.
It's not only for supporting AV but also supporting a function like the supervision that I mentioned before. If you want to reach a good meantime between failure for the supervision, in which the driver's responsible, you still need this high definition map. So first, what's the motivation between a high resolution map? So theoretically, because human drivers can do it. So theoretically it's possible to detect and interpret all the roadway data, real-time, online from the car on board system. So we're talking about drivable paths. We're talking about lane priority.
Which lane has priority one over the other? Path delimiters, where are the curbs and so forth? Traffic light and lane association. Which lane is relevant to which traffic light? Crosswalk associations with traffic lights and with lanes. Stopping and yield points. Now, when you're taking an unprotected turn, where exactly you want to stop and wait for the oncoming traffic. You are approaching a higher priority route and you need to stop or slow down, what is the optimal point to do that? So all of this information, we as human drivers we learn how to do it, and we do it efficiently. Our claim is that making this work is possible, but making this work at the high meantime between failure, that's not realistic for the current state of AI.
The current state of AI can detect road users at fidelity approaching human perception, in terms of detecting pedestrians and vehicles and other road users. But understanding the roadway, and the details that I'm going to show you, in a real time system, you have a one shot to do it, maybe in the future, and I'm always optimistic about computer vision. Maybe in the future it can be done, but right now it's not realistic. So what will happen if you don't build high resolution maps, is that your meantime between failure would be low.
You'll fail, every 10 minutes, every 15 minutes. So the idea of the high resolution maps, is you prepare all this information in advance. And all actors in the AV space are using high definition maps. Even those that claim that they're not using high definition maps, they have some sort of map, because when we see the videos posted by people driving those vehicles, we see that there's some version of a map there. So what are the challenges? The challenges is first, you need to build them at scale.
If you're talking about a robotaxi at a certain city, it's okay. For now you don't need the scalability because you're going to test or provide a service in two or three cities, that's fine. In the future, you also need scale for robotaxi.
But if you're talking about supervision, in which you want to have this capability on production cars, driving assist cars at volumes of millions, they need to drive everywhere. So you need to have your high resolution maps at scale. And later when we're thinking about 2025 in which a self-driving system can reach the performance and the cost level for consumer cars, they also need to drive everywhere. You cannot tell them you can drive only in San Francisco.
You're only going to spend all that money and drive only in San Francisco hands-free. So maps at scale is an issue. Then how fresh is the map? You need to update it. Ideally, you want to update it in real time. The moment there is a change on the road, it has to be reflected in the map, ideally. Now, today maps are updated every month.
We want to be able to update it at a matter of minutes. So even if you're updated on a matter of a day, daily update, that's also groundbreaking compared to the way maps are being done today. And third, the accuracy.
In order to make use of those maps, you need to place the vehicle and other road users at a centimeter level accuracy. So this is also, and so GPS is not good enough. So what is the common approach of building high resolution maps? Now, we know about the common approach because we have a separate division, because it took us five years to develop these high definition maps using our crowdsource data. So in the meantime, we had a division that builds high definition maps in the common way in which we have a vehicle, a collection vehicle, with a Velodyne 360 degree LiDAR and cameras.
And we build high definition maps just like all our competitors know how to build high definition maps. So now I'm about to show you what what's wrong with it. So let's have a look at this clip here. So this is a high definition map that we built in an area around Jerusalem. Now, look how detailed and how accurate everything is there, in 3D.
And it's really over specified. You don't need this kind of global accuracy in order to support AV. That means a host vehicle needs to locate itself very, very accurate in the map, and a radius of about 200 meters around it.
All those other road users need to be located very accurate in the map, but something that is two miles away, I don't care about it. Why does it need to be located very accurately in a map? So this idea of a map that is centimeter level accurate at a global coordinate system is over specified. You don't need it. So this is one thing that is wrong. So existing high definition maps on one hand are over specified.
Second, they're under specified, in all but it's related to semantics. So we kind of divided the semantics into these five layers. Drivable paths, lane priority, association between traffic lights and crosswalks to lane association. Stopping and yield points and common speed. We know what the legal speed is? What is the common speed? If you want the car to drive like a human in an area, it at least needs to know what is the common speed.
What would be the maximum speed is a matter of negotiation with the regulatory body. Because humans drive above the legal speed, but at least it needs to know what is the common speed in order to drive in a way that doesn't obstruct traffic. Now, these semantic layers are very, very difficult to automate.
This is where the bulk of really, the non-scalability of building high definition maps come into. So take, for example, a drivable path. Now, if you look at this picture of the road, that there are no lane marks. So how would you know that it's a two-way street in both areas? It's kind of tricky. If you look at the priority, on the left-hand side, you have a no turn on red. On the right-hand side, there's a sign telling you that after you stop, you can turn on red.
Then on the row below, you are not supposed to turn left on green and on the side, on the other one, you can turn on green after you yield. So understanding the priorities, lane priorities and association with traffic lights is tricky. And then if you look at traffic lights and their association with lanes and crosswalks, it's a zoo out there. Just look at these pictures.
These are from different territories from geographies in the world. It's very, very complicated. The fact that humans can do it doesn't mean it's easy.
It's very, very complicated. So this is where lots of manual work is being put into building a high definition map. And then stopping and yield point. Now, theoretically, if you know everything about the scene in 3D, you can calculate based on viewing range what is the best point to stop in order to see what is relevant to the traffic and then continue.
The picture here shows you that in order to do that, you have an occlusion. A building is occluding the viewing range. Now, if the map doesn't have information about this building, you'll not be able to calculate exactly what is the optimal stopping point before taking a turn there. So it means that you're placing more and more requirements on this poor map.
Not only the roads and the semantic information but now where are all the buildings located? The height of the buildings. Are they translucent, not translucent and so forth and so forth? So you see, it becomes unwieldy. And then, common speed.
You see here kind of a bar of... The color coding is speed color coding. We know what the legal speed is, and we can calculate what should be the maximum speed based on curvature of the road and so forth but it's not enough. There are all sorts of cultural factors in determining speed of driving, especially in deep urban. So this is also very useful information in order to create a smooth driving experience.
So this is why we're not calling what we do a high definition map. We're calling it an AV map, autonomous vehicle map. So it supports kind of three areas. One is scalability, and that's where the crowdsource comes in. Second is accuracy, but accuracy where it matters.
As I said before, accuracy matters locally. Where I am located and about 200 meter radius around me where other things are located, relative to the stationary elements of the world. What happens 10 miles away doesn't matter. So there is to focus on accuracy, but where it matters. So it's kind of, it's not global, it's kind of local-global accuracy or semi global accuracy. And then the detailed semantic features find a way to fill in all the semantic layers.
The example that I showed before, automatically just using the crowdsourced data. And the crowdsourced data, I remind you, are not images. It's a 10 kilobyte per kilometer data, snippets of data. So the REM technology is kind of, we call RSD the road segment data.
This 10 kilobyte per kilometer, we call this harvesting. So this data is being sent to the cloud. Then there is this automatic map creation that you have been working on it for the past five years.
And then there's the localization. Given that the map is in the car or an area, a relevant area is in the car, and given what the cameras see out there, how do you locate the car into the map at the required accuracy? Which is a centimeter level accuracy locally. So here is kind of an example. On the left hand side is the harvesting. I'll show you a clip of harvesting in a moment.
And then we start cloud-based. Now, everything I'm talking about is done in the cloud. Data is being sent to the cloud.
So the first thing that we need to do, the data arrives at a GPS level accuracy, because every car has a GPS. We're not talking about differential GPS, just normal GPS. So you need to take that data where the accuracy is a GPS level accuracy, and you need to align the data coming from hundreds, thousands of cars.
Each car is seeing the world kind of slightly differently from a different angle, and not all the landmarks are detected by each car. There could be occlusions, all sorts of things that could affect what is being detected, and then align them at a very, very high accuracy. And then the third part is what we call modeling and semantics. After we aligned everything, how do we go and figure out where the drivable paths, the traffic light, lane association, the crosswalks and so forth and so forth.
All those examples of semantics. So here's the example of harvesting. So all what is kind of color coded in yellow or overlaid in yellow, are stuff that the camera picks from the scene and sends to the cloud. So again, the camera is not sending images to the cloud. It's is detecting lane marks and landmarks and trying to understand the drivable path.
It won't do a perfect job in understanding the drivable path, but we figure that maybe one car would not do a perfect job on understanding the drivable path, but hundreds of cars passing, they could create a much, much better understanding of where is a drivable path? Much more than a single car. So we call that data RSD, road segment data, 10 kilobyte per kilometer sent to the cloud. So here on the left, you have about 300 drives, 300 different cars in an area. And you see that the accuracy, it's very, very difficult to understand where are the drivable paths from this information? So through a process of alignment, which is a complex process, we're able to take this data and figure out where the drivable path, where the path delimiters, where are the lanes, all aligned at the centimeter level accuracy? So this is one stage. So here's an example.
On the left-hand side is kind of the input GPS accuracy. On the right hand side is a zoom in. So the blue dots are the fragmented lanes.
So this is kind of lane information, fragmented lane. The magenta dots are the drop points of the drivable path. So every second we have a drop point. And you see that these drop points are all over. It's very, very... It's almost impossible to see that you have here two drivable paths.
And then the red dots are the path delimiters, the road edges. Now, after alignment, you see that now the blue points, which is the lane, are fully aligned, over 300 drives. You see the magenta points are now, these are the drivable paths.
So now you clearly see two drivable paths and you see the road edges. So this alignment is all geometrical, and it's tricky because each type of information has a different error model. It's a bit tricky. Now, we do the modeling process.
So what we want to do, we want to go from the left-hand side. Here we have kind of example of a roundabout, to the right right-hand side. On the right hand side, we see the lines are the drivable paths. We see the pedestrian walking zones. Now, just simply clustering and doing a spline, a fitting and so forth, gives us the picture on the upper left.
We see it's not perfect. And then over time, we develop all sorts of deep networks, techniques using high definition maps as training data in order to train this modeling approach that eventually gives us something that is very close to perfection. So let's now try to figure out how crowdsourcing... So all what I showed right now is all about the geometry. Now, let's look into the semantic.
So again, we have this picture in which we want to figure out the drivable paths, they're now lanes. So you see on the right-hand side of it, you see now we can put the correct drivable paths because we have information from crowds. People driving along those two drivable paths. On the right-hand side, you see a complicated area. And this complicated area, you see all the drivable path coming from crowdsource.
So we can take something quite complicated and automatically, you know, build and interpret the entire picture of what is going on there. Let's look at now on the left-hand side. Left-hand side, we have association between traffic lights and lanes. The drivable path. The drivable paths are in yellow here.
And the circles are the traffic lights. And you see it's quite complicated. We circled just one of them, but you see on the top and then on the bottom, there are many traffic lights and the association between which lane, which drivable path is relevant to which traffic light is quite complicated. And all of this is done, inferred automatically.
At the center, you see kind of a yield sign. Where exactly is the stopping point when you have a yield sign? We can get that from the crowd. And on the right hand side, we have a crosswalk association. So when you have a crosswalk, pedestrian crosswalk, we want to associate with lanes, which lane is relevant, but we also want to associate with which traffic light, because it determines priority. If it's a red light for the pedestrian, then the car has priority.
Of course, if the pedestrian jumps in, the car needs to stop, but in terms of priority, it's important to know whether there's a green light for the pedestrian or a red light for the pedestrian. And we need to know this kind of association. Further, we can do a kind of a path priority on the left-hand side. Understanding kind of the frequency of stops, in each path tells us which lane has priority to another line of force. We're talking about a situation in which there's no traffic sign that tells us that we have a priority.
When there's a traffic sign, it is simple, but there are many situations where there are no traffic signs that will tell you who has priority. And this can be inferred from crowd. At the center is, where it's kind of the optimal point to stop before taking a turn.
So we see how, where did other drivers stop? On the right-hand side, you see, if I want to take an unprotected left turn, where's the optimal point to stop in order to wait for the oncoming traffic and then take a turn? It's also quite tricky. Again, we get this from the crowd. And this is the common speed. The color codes are our speed, our velocities. What is the common speed in this particular territory, in this particular country, in this particular road type? How fast people on average go.
This is very, very important in order to create a good and smooth driving experience. So today we have about Eight million kilometers of road being sent every day.Over six car makers. In 2024, it's going to be one billion kilometers of roads being sent daily. So we are really on our way to map the entire planet. Map is not the right word, 'cause we don't have street names and things like that. So, it's not a replacement of a navigation map.
It's kind of the high resolution information that allows the car to interpret the road. I wish we could have a different word for a map. It's not a map.
So this was about our game technology and and how game changing it is. It allows us to do geographic scalability, using very, very low cost information coming from cars. 10 kilobyte per kilometer. Sending this to the cloud is really almost zero cost. And all the technology in the cloud to build those high definition maps in order to again have a geographic scalability to support level four driving and to support the supervision, to support level two plus level two, very, very advanced driving assist, given that we have in advance the information about the roadway, especially in the complicated, in the urban settings. The second deep dive area that I want to get into, is about the radars and LiDARs.
So we have two subsystems, camera subsystem, radar, LiDAR subsystem. Now there are many LiDAR suppliers, there are many radar suppliers. Why do we think that we need to get into development of readers and LiDARs? So, first let me explain that. So for 2022, which is a year from now, we are all set. We have the best of class time of flight LiDARs from Luminar. Our vehicle has a 360 degree coverage of Luminar LiDARs.
And then we have stock radars. Again, 360 degree coverage of stock radars. And the LiDARs, the Luminar LiDARs, and the radars that we have, the stock radars that we have are sufficient to create a sensing state as I showed you in one of the clips before, we can do an end-to-end driving with a very, very high meantime between failure of each subsystem. Now, when we're thinking about 2025, we want to achieve two things in 2025.
So 2025, we want to reach this level of consumer AV. Now, there are two vectors here. One vector is cost. We want to think how to reduce costs significantly. Second vector is operational design domain.
We to get closer to level five. So we want to do two things. Be better and be cheaper, right? So it's kind of contradictory. So when we look at front-facing, front-facing, we want to have a three-way redundancy.
We want to have radar as a standalone system, LiDAR as a standalone system, and of course the camera as a standalone systems. So we want more from the radar, because radars today don't have the resolution and don't have other, don't have the dynamic range in order to be a standalone system in a complex setting. And we want more from the LiDARs, which I'll mention why we want more from the LiDARs. Besides front facing, we don't want LiDARs. We just want radars. But existing radars would not be able to do it.
Radars alone will not be able to create a sensing state in complex situations. So we need much, much better radars. So we're building kind of a novel thinking in which we believe, that the radar evolution has much more way to go. In order to build something, these are imaging radars, which I'll detail in a moment. That could be a standalone system. So this is really very, very bold thinking.
Now, why is that important? It's important because the difference between radars and LiDARs in terms of cost is an order of magnitude. No matter what people tell you about how to reduce the cost of LiDAR, the radar is 10 times lower than that. And we're building LiDARs. So I know exactly the cost of the LiDAR is everything. All what you're hearing about, LiDARs would be 10 for a penny or something like that. That's fake.
LiDARs are about 10 times more expensive than radars. So if we want to do a kind of a drastic cost reduction, we need to take radars and push the envelope much, much further with radars. So this is why we decided that through Intel, we have kind of the knowhow. Mobileye doesn't have the knowhow, but Intel has.
So through Intel, have the knowhow to build kind of the cutting edge of radars and cutting edge of LiDAR. So let's start with radars. So we call this a software defined imaging radar.
So one thing to say about radars, which is very non-intuitive to someone who's not an engineer or is not fluent with radar technology, that unlike cameras and LiDARs, a point in the world manifests itself, not in a point in the image, as a single point in the image, but all over the place. So if you look at the picture above, picture above is what you see from the camera. And we see here four targets. And the picture below is kind of the radar signature. And each target, each physical target has what is called side lobes, has echoes.
Because each point in the scene manifests itself in multiple points in the image. And being able to separate those echoes and leave only the true targets is very, very tricky. So it requires, it's not only increasing the resolution but increasing the dynamic range, increasing what is called side lobe levels, the SLL. Increasing the accuracy of the data in order to be able to treat the image from the radar as something useful, just like an image from a LiDAR or an image from a camera. So there are two goals here. One is increase the angular and vertical resolution.
That is kind of a no brainer. We want much higher resolution, because we want to put a contour around the object. We want to be able to detect small objects.
So we need much, much higher resolution than today's radars. But the second part is a bit more tricky. We want to increase what's called the probability of detection. So this is increasing considerably the dynamic range of the sensor and the SLL of the sensor. So here is what we want to do.
On the left column is kind of the ecosystem standard. We want to move from radars that have 192 virtual channels. So you have 12 by 16 transmitters and receivers. When I'm talking about standard, I'm talking about really the very, very advanced standards. Most radars don't have this amount of transmitters and receivers.
Into something that's much more massive, which will have 2,304 virtual channels. So it will be based on 48 by 48 transceivers and receivers. Now, there are significant challenges from simply adding more and more transceivers and receivers, as computational complexity increases a lot. And I'll mention that in a moment.
Second thing that we want to do, is we want to increase this kind of the dynamic range of the side lobe levels from 25 DB carrier to about 40. Again, these numbers, it's not a linear relationship. It's logarithmic. So, it's night and day, basically. So that will enable us kind of two separate targets that are masked by other targets that have a much, much more powerful radar cross section, RCS.
It's both longitudinal and also in azmuth. Second, which is the most challenging, is going from 60 DB dynamic range to 100 DB dynamic range. So this will enable us to detect weak targets that are far away, that are masked by close targets that are much more, the RCS is much more stronger. So the picture below, you see this motorcycle inside a bounding box. This is the kind of situation we want to be able to handle.
We want our radar to be able to pick up this motorcycle even though there are many, many more powerful targets that have a much higher RCS signal. The paradigm shift in building this radar is divided into one, is this 48 by 48 transmitter versus a receiver gives us 2,300 channels. It creates a complexity burden to process all this data. If you do it kind of in a brute-force naive way, it's about 100 TeraOPS.
This is really significant. By building our own system on chip, by building, creating very, very advanced algorithms and approximations, we're able to bring it down to 11. So one, in order of magnitude in terms of computational complexity. So 11 TOPS in an SOC. So it's a big jump there in terms of what has been achieved.
Second, everything is digital. So, you know, the bandwidth is one gigahertz. The accuracy is 11 bits compared to ecosystem standard of 20 megahertz and eight bits. Working everything on the digital domains allows us to build filters that are much more advanced, much more accurate and powerful than what you can do in an analog domain. And this gives us both the higher dynamic range and also the accuracy to be able to create an image that can be useful as a LiDAR image or as a camera image. And let me show you some examples.
So in this clip, and then I'll take snapshots of this clip. You see on the right hand side, targets in an urban setting. Both stationary and moving targets. And there are also pedestrians out there. So let's have a look at this snapshot.
What you see on the left-hand side, you see a vehicle. That vehicle is moving, and you see pedestrians, the pedestrians are moving. Now, the vehicle has a much, much more stronger radar cross section than the pedestrians. On the right-hand side, you see the detection, kind of a bounding box.
Because we have high resolution, we can put a bounding box. Both at the vehicle and on the pedestrian. And we know also the direction of the pedestrians. Visually, you can hardly see that there are two pedestrians there.
So, here it's the power of the adding resolution. It is the power of the dynamic range, and it's the power of the side lobe levels that we were able to reduce them considerably. Here's another example. You have a vehicle, you have a pedestrian.
The vehicle would have masked the pedestrian because of the much, much higher RCS. And you have also the stationary vehicles there, which also adds complexity, not interfering and masking the pedestrian. Here is an example of the high dynamic range and the resolution. So we're talking about a tire without the rim.
Just the rubber of the tire being detected. 130 meters at high resolution, you'll see there's this red arrow consistently around it. And when we get closer, you see that this is a tire without the metal, just the rubber.
Now, this is important because we want non front-facing. We want three way redundancy. We want the radars also to be able to detect hazards and hazards could be low and small and far away. So this is about the radar. So all of this is targeting 2024, 2025 in terms of start of production. We have already samples, but again, this is futuristic.
It's not coming out tomorrow. Second is why are we building a LiDAR? So, the prevalent technology of LiDAR is time to flight LiDARs. So it gives you every point in space.
It's gives you its azmuth and also it's range. So it's kind of a 3D sensor. Time to flight sensor, gives a burst of a laser at every point. And then there is some kind of optical ways of moving this laser beam in order to create an image. If a frequency-modulation coherent wave is based on a different principle, in which you send a wave rather than a burst, this wave is chirped. So it kinds of a step, has a step form.
Now, the wave itself, the amount of energy at the peak is much, much lower than the amount of energy that you send in time to flight, but if you take the integration below the curve, you have much more energy than what you send in time of flight. Third, the second advantage, is you get also velocity. Just like with the radar. So now it's a 4D sensor. We also get velocity at every point.
So now if we look and what I showed here is an example of an article. FMCW LiDAR, game-changing for autonomous driving. So, it's being acknowledged, that kind of the next next frontier of LiDARs is the FMCW. But it's a very, very difficult technology and we have great advantages there that I'll show you in a moment. So the idea is that we're moving from 3D sampling of range, azmuth and elevation to 4D, having also the Doppler effect.
This allows us to kind of instant heading the measurement, time to contact at the point level. And you can cluster data because now you have another measurement of velocity. You can cluster data much more efficiently. There's this issue of decay, which in time to flight is one over distance squared.
In FMCW, is only one over R because you are measuring different properties of the photons, kind of the magnetic field rather than the optical energy. So this gives us more range. We can have a better ODD for the 2025. And then sensitivity to interferences, we have, FMCW gives you a much, much higher immunity.
First, you are measuring only what you're emitting. You are not just measuring the particular wavelengths of photons that are coming back. And second is because of this wave that you are sending and not the burst, if you have retro reflectors they'll not create blooming effects like retro reflectors would create with time of flight sensors. And then being able also to create a density of two million points per second and 600 points per degree squared. Here are some examples on the top line.
The color coding is velocity. So it's not range, it's velocity. So you can have velocity information at a snapshot, just like with radars. The image below is just retro reflective. We can accurately detect the retro reflective, not have a blooming effect, because you're sending a wave and not a burst of light.
So, but what is unique about what Intel is doing is that Intel has special FABs. It's called silicon photonics FABs that are able to put active and passive laser elements on chip. And this is really game changing. And we call this photonic integrated circuit pick. So this is it. It has 184 vertical lines.
And then those vertical lines are kind of moved through optics But the chip itself gives you in a snapshot 184 lines, and then it is being moved optically. And having FABs that are able to do that, that's very, very rare. So this gives Intel a significant advantage in building these LiDARs.
So I'm now going back to update mode. So I did two deep dives on maps and sensors. RSS, just update. RSS is our kind of safety model. So RSS is based on the idea that we want to mathematically define what it means to drive carefully.
What is the dividing line between reckless and carefulness in a mathematical way? How do humans judge this? We make assumptions then we take the worst case. Given assumptions, what those assumptions would be how do we parameterize those assumptions so that we can then engage with regulatory bodies on those assumptions and reach an agreement? What are we allowed to assume? What we are not allowed to assume, or what set of parameters should we put there? And then we take the worst case assumption. And in that way, we can prove that if all cars behave in this way, there'll never be accidents. Of course, we have human drivers there out there. So we can prove that we will never cause an accident. This is kind of the idea.
And what is really non-obvious about all of this, is that we really were able to reduce the experience of driving, into five major rules. And it's kind of a generating function of all the experience of driving. So in terms of where we are in evangelizing it, we have an IEEE program chaired by Intel, whose role is to standardize a model like RSS. It won't be called RSS, of course, but it will be like RSS. ISO also is now creating an effort around the same principles.
The US Department of Transportation has already been writing about RSS and it's important. The UK Law Commission also is acknowledging the importance of RSS and how it should be integrated into laws that will enable to remove the driver from the driving experience. Last is the Mobility-as-a-Service progress. So we're not just building a self-driving system.
We want also to build a business around it. And when we look at the world, we have public transport operators and then we have transportation network companies like Uber and Lyft of the world, and both of those, in the future, they want, they aspire to automate, to remove the driver from the experience. And this is where we want to come in, we and move it together.
Is to build different models. First, a vehicle as a service, ride as a service and then mobility as a service around our joint technology. Few words about Moovit. It is the biggest kind of trip planner, around 900 plus, more than 950 million users, active in 3,400 cities and 112 countries. It has lots of transportation data, which is very, very important in order to create kind of a full experience.
You want to drive from point to point. Some of it could be with a robotaxi. Some of it could be through a scooter. Some of it could be through other public transport, could be a train. All this information is part of Moovit's data, which allows us to integrate and then create a very, very good experience of mobility as a service. And those are the layers.
The self-driving system is only layer number one, which is what Mobileye is doing. We are working with car makers to integrate it into cars for robotaxi. So that's a self-driving vehicle. And then all the three other layers, the tele ops, the fleet optimization, the control center, mobility intelligence and then the payment and the user experience are all under the responsibility of Moovit. And we're very deep into the integration.
In terms of deployment, we have deployment in Israel. 2022 together with a joint venture with Volkswagen. We have deployment schedule in France with two largest European PTOs. Testing is starting next month. We have a deployment plan in Daegu city in South Korea.
Testing is commencing middle of this year. And we have a collaboration with WILLER Group and also PTO in Japan. The idea is to launch in Osaka 2023, and we will expand more. So I think this is all what I had to say. Again, regular update and deep dive on to what I believe are really game changing activities that we have been working on in the past few years.
But I reached a point in which it makes sense to start sharing with the market and with everyone here who's watching this. One is about the crowdsourced mapping. Why do we need it? Why are we doing it? What is important about the geographic scalability? It's not only for autonomous driving, but also for driving assist. And why are we developing radars? Why are we developing LiDARs? Again, this is long, forward-looking, 2025, not just what we're doing 2021. We would like 2025 to be the year in which we can start giving the experience of people buying a car and sitting in the back seat whenever they want and have the drive everywhere.
not just in one particular location. Thank you.
2021-01-14 12:39